When Machines and Humans Fail Differently
AI Mistakes vs. Human Errors: A Battle of Blunders?
Last updated:
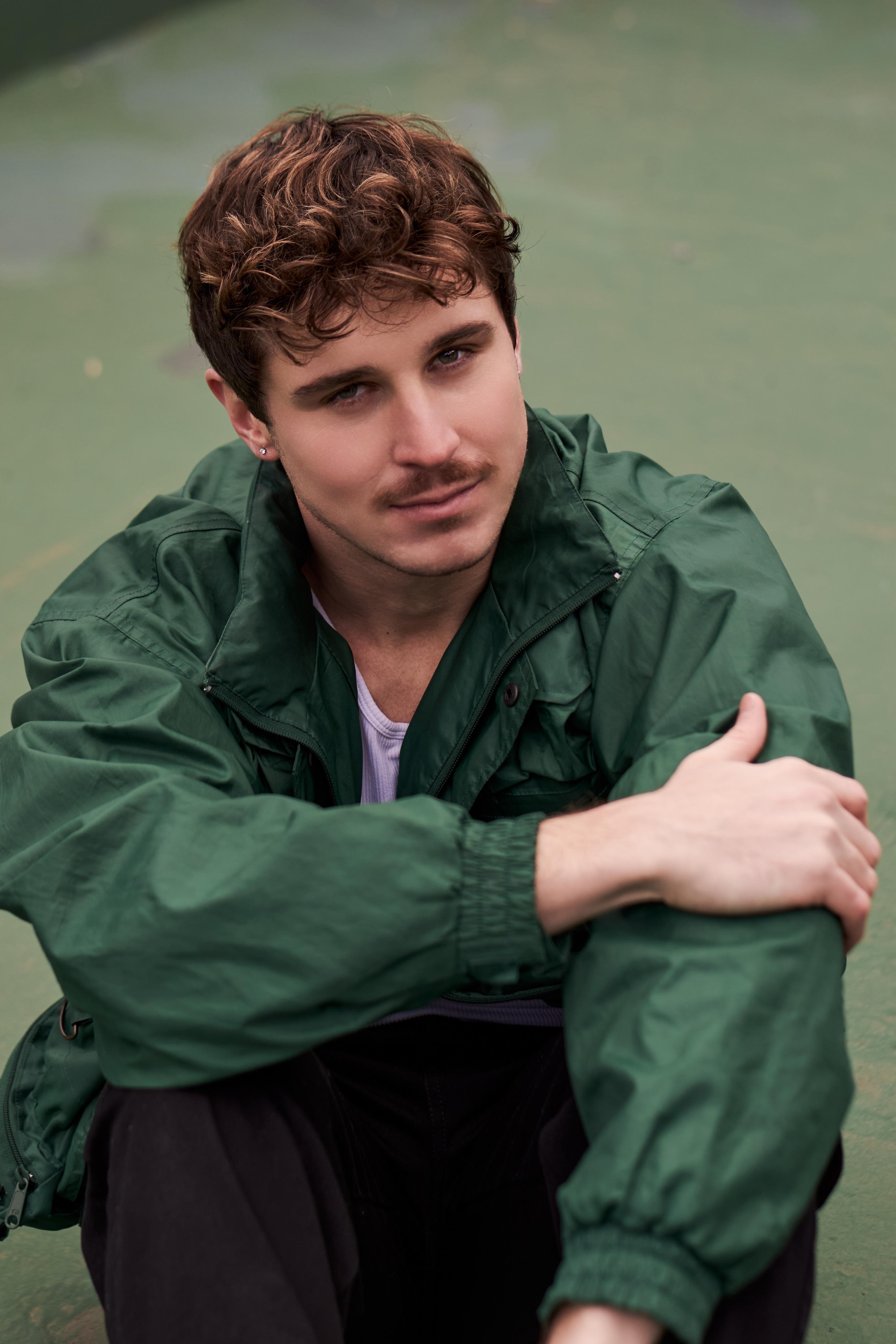
Edited By
Jacob Farrow
AI Tools Researcher & Implementation Consultant
Explore the intriguing differences between mistakes made by AI systems and human beings. From training data biases to contextual misunderstandings, this article delves into how AI and human errors contrast, and the implications for safety and policy. Experts share insights on modeling, predictability, and prevention strategies for these unique types of mistakes.
Introduction: Understanding AI vs Human Mistakes
Artificial Intelligence (AI) systems and humans have different ways of making mistakes, and understanding these differences is crucial for navigating the future of AI integration into society. AI mistakes often arise from biases in training data, limitations in algorithms, and rigid pattern matching, whereas human mistakes are typically related to misinterpretations of context, emotional interference, and errors in judgment. This fundamental distinction not only influences how errors are managed but also shapes the perception and trustworthiness of AI systems in various applications.
One of the primary concerns with AI mistakes is their potential scale and impact. Unlike human mistakes, which are usually isolated incidents, AI errors can propagate rapidly across systems and affect millions simultaneously. This scalability of errors can lead to widespread disruptions if not adequately addressed. However, the systematic nature of AI errors also allows for more predictable modeling and mitigation strategies, as highlighted by AI safety researcher David Winter, who notes the advantage of predictability in preventing and correcting AI errors.
Learn to use AI like a Pro
Get the latest AI workflows to boost your productivity and business performance, delivered weekly by expert consultants. Enjoy step-by-step guides, weekly Q&A sessions, and full access to our AI workflow archive.
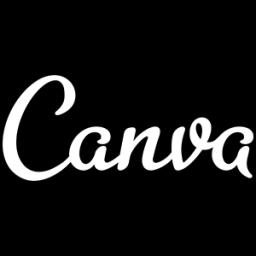
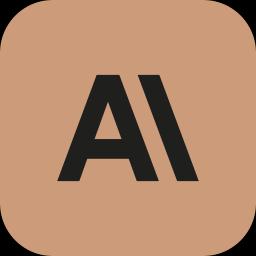
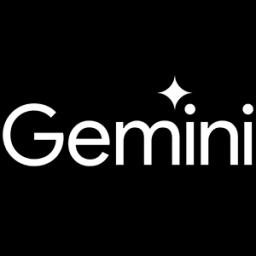
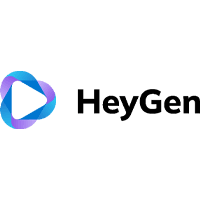
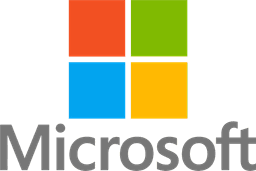
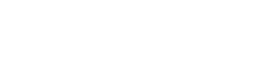
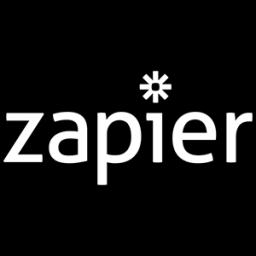
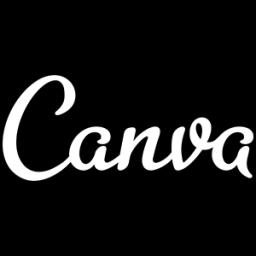
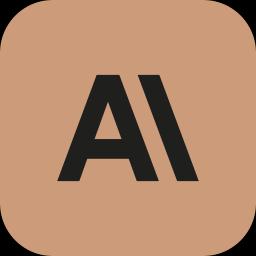
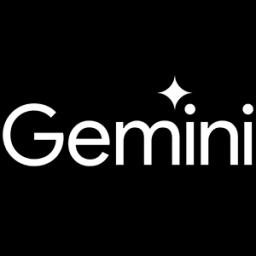
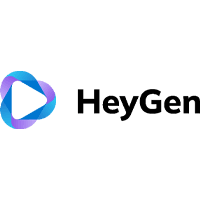
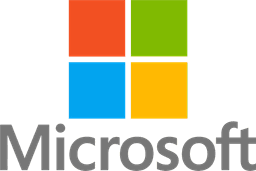
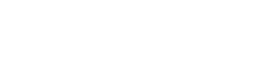
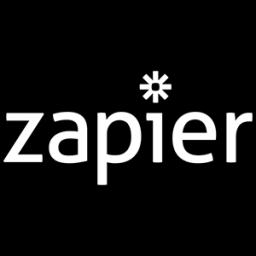
The Fundamental Differences in AI and Human Errors
Artificial Intelligence (AI) systems and humans operate fundamentally differently, which means their errors arise differently as well. AI errors often originate from issues within the algorithm or biases embedded in the training data. These systems are designed to detect patterns and anomalies based on data provided, which can lead to mistakes if the data is flawed or incomplete. On the other hand, human errors tend to result from complex factors such as emotional influences, contextual misunderstandings, or poor judgment. Thus, while AI errors might seem systematic, they lack the nuanced understanding that humans possess, leading to distinctly different error profiles.
Unlike humans who learn through experience and self-reflection over time, AI systems need structured intervention to learn from their mistakes. Human learning is inherently adaptive; experiences, mistakes, and reflections all contribute to an individual's personal and professional development. AI, however, requires corrected data or new algorithms to address and learn from any mistakes, implying a more rigid process that lacks the intuitive, experiential learning style characteristic of humans. This fundamental difference in learning from errors means humans can often preemptively adjust to avoid future mistakes, whereas AI requires explicit programming changes.
Understanding AI errors is crucial for ensuring the safety and reliability of AI systems. Given the systematic nature of AI mistakes, developers can implement more targeted and effective measures for preventing and mitigating these errors. Predictable and structured, AI errors allow for the design of safety measures that can be tested and refined over time. However, as AI systems become increasingly complex, the importance of designing comprehensive evaluation protocols becomes more significant to ensure these systems can operate safely without unintentionally causing harm in unpredictable scenarios. AI safety, therefore, hinges not only on technological advancements but also on ethical oversight and rigorous testing protocols.
Learning from Errors: AI vs Human Approaches
Artificial intelligence (AI) mistakes differ significantly from human errors in their origins and manifestations. While human mistakes often arise from contextual misunderstandings, emotional biases, or errors in judgment, AI errors are typically rooted in biases within training data, algorithmic limitations, or rigid pattern recognition processes. This fundamental difference underscores the need for distinct approaches in addressing errors by AI systems. Unlike humans, who learn from experience and reflection, AI systems require retraining and algorithmic adjustments to address their errors effectively.
Learn to use AI like a Pro
Get the latest AI workflows to boost your productivity and business performance, delivered weekly by expert consultants. Enjoy step-by-step guides, weekly Q&A sessions, and full access to our AI workflow archive.
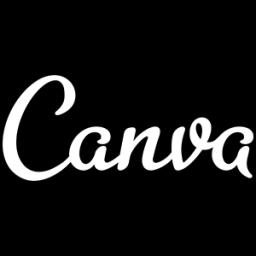
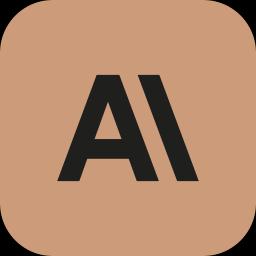
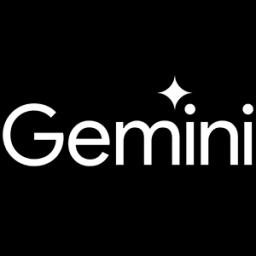
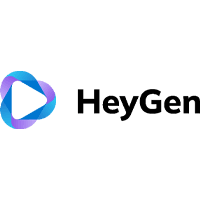
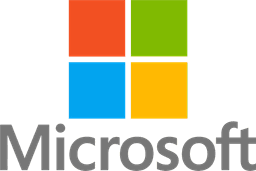
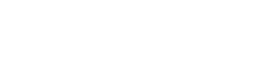
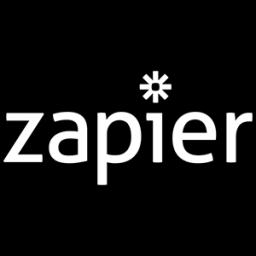
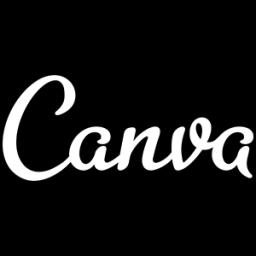
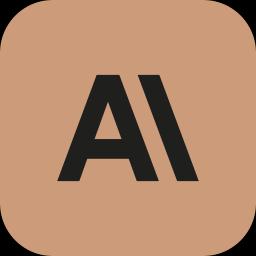
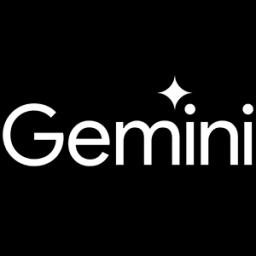
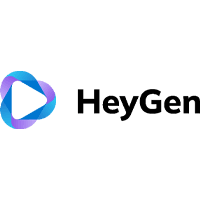
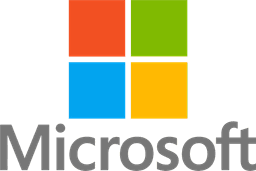
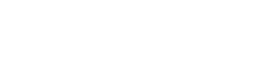
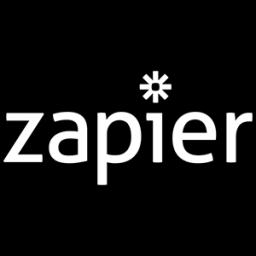
The ability of AI to learn from its mistakes is fundamentally different from human learning. While humans naturally learn through experiential understanding and reflection, AI requires explicit interventions such as retraining with corrected data and algorithm updates. This lack of intuitive learning means that AI systems cannot autonomously improve from errors without human-initiated processes. While these processes can be effective, they underscore a pivotal difference in how learning is facilitated in AI versus human cognition.
Understanding AI errors is crucial for the development of appropriate safety measures. Because AI errors can be systematic and predictable, they can often be modeled and mitigated more effectively than human errors. Nonetheless, the scale at which AI operates poses unique challenges; a single AI error can impact millions simultaneously, unlike individual human mistakes. Thus, addressing AI errors entails not only technological improvements but also the formulation of rigorous oversight mechanisms to ensure safety and reliability in AI applications.
Public perception of AI mistakes reflects a mix of concern and debate. A significant portion of the public views AI errors as more unsettling than human mistakes, possibly due to their potentially rapid escalation and impact. These perceptions contribute to a trust deficit, where people are more reluctant to rely on AI for critical decision-making. Meanwhile, discussions often highlight a divide between those who see AI errors as natural growing pains in technological advancement and those who perceive them as inherent flaws requiring critical attention. This discourse shapes the landscape of AI adoption and trust in society.
AI errors also pose substantial future implications across various sectors. Economically, industries may need to adjust insurance and liability frameworks to accommodate AI-specific errors, potentially fostering new markets for AI risk assessment and mitigation. Socially, AI is likely to influence trust dynamics and usage norms, prompting new standards around transparency and decision-making processes. Politically, the differential regulation and oversight of AI across regions may lead to international tensions, calling for harmonized global frameworks similar to those in aviation safety. These implications illustrate the broad impact and pervasive nature of AI error management.
AI Safety: Implications of Error Types
In the realm of artificial intelligence (AI), the nature of errors is distinct from human errors, fueled by AI's reliance on data and algorithms. The necessity to comprehend these disparities is paramount for ensuring AI systems' safety and reliability. Each type of error possesses unique characteristics that necessitate different mitigation strategies, underscoring the importance of understanding how AI operates differently from humans.
AI errors primarily stem from biased datasets, inadequate algorithms, and rigid pattern recognition, while human errors often arise from misinterpretations, emotions, and judgmental flaws. This divergence in error generation underscores the need for robust AI training and algorithmic refinement to minimize the negative impact of AI errors. AI does not learn from mistakes in the instinctive manner humans do, pointing to a critical area of improvement through iterative learning processes and algorithmic adjustments.
Learn to use AI like a Pro
Get the latest AI workflows to boost your productivity and business performance, delivered weekly by expert consultants. Enjoy step-by-step guides, weekly Q&A sessions, and full access to our AI workflow archive.
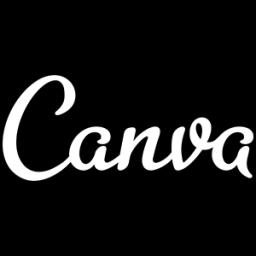
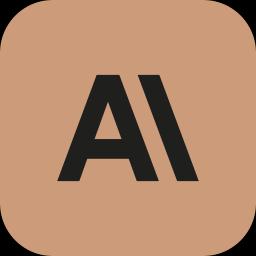
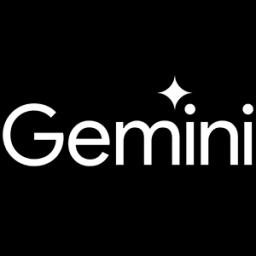
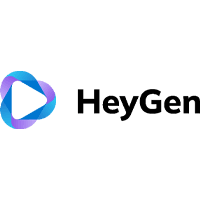
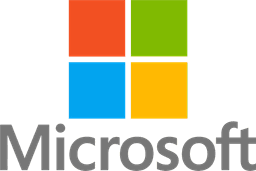
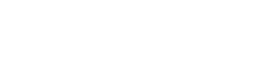
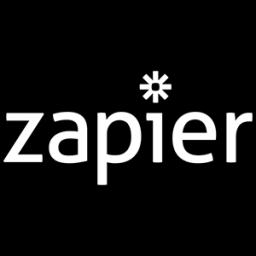
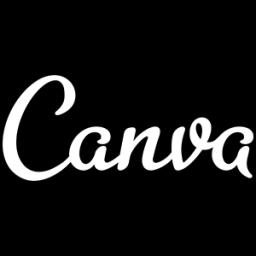
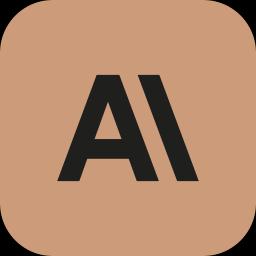
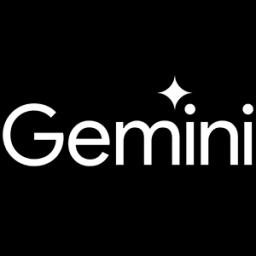
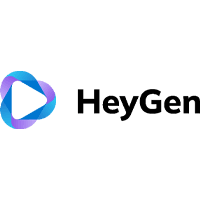
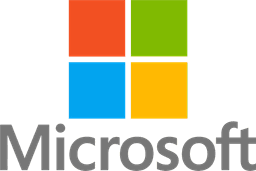
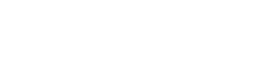
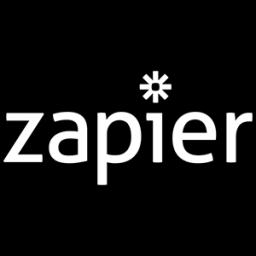
The implications of AI and human error differences are critical for developing effective safety measures. While AI errors can be systematically predictable, allowing for preemptive error detection and correction strategies, they also present the risk of scaling rapidly and affecting large populations at once. Human errors, on the other hand, are less predictable but generally more contained. Therefore, establishing comprehensive error management strategies tailored to the nature of AI is vital for enhancing system safety.
Recent global events underline the urgency of addressing AI safety. The EU AI Act's final approval signifies a significant move towards creating legally binding safety standards for AI, reflecting growing concerns over AI system errors. Simultaneously, investigations into Tesla's Autopilot and initiatives like Google DeepMind's safety frameworks demonstrate a proactive approach in rectifying AI errors and safeguarding users.
Expert opinions highlight that while AI errors are easier to model and mitigate due to their systematic nature, this predictability does not eliminate the seriousness of such errors. Diverse perspectives, such as those from Anthropic, warn about the potential unpredictability in advanced AI systems and advocate for research frameworks that consider both technical and societal safety aspects.
Public reactions reveal a broader concern about AI errors compared to human mistakes, not only due to their potential scale but also because of their perceived unnaturalness. This sentiment underscores a trust deficit in AI technologies for crucial decision-making, demanding greater transparency and error accountability from AI developers.
The future implications of AI error characteristics extend across economic, social, and political domains. Economically, AI error management could lead to the expansion of insurance markets tailored for AI-related risks. Socially, trust in AI is likely to become more selective, prompting demands for transparent AI processes. Politically, these issues could drive the establishment of standardized global AI safety regulations or even new political movements focusing on AI ethics and governance.
Expert Opinions on AI and Human Mistake Patterns
Artificial Intelligence (AI) and human errors manifest in fundamentally distinct ways, each influenced by intrinsic system properties and external contexts. AI mistakes are primarily driven by the data they are trained on, often reproducing hidden biases or reflecting algorithmic blind spots. Unlike humans, AI lacks the intuitive judgment and contextual awareness that guide human decisions, making its errors more artificially systematic rather than contextually misjudged. These differences underscore the necessity for targeted approaches in understanding and anticipating AI errors.
Learn to use AI like a Pro
Get the latest AI workflows to boost your productivity and business performance, delivered weekly by expert consultants. Enjoy step-by-step guides, weekly Q&A sessions, and full access to our AI workflow archive.
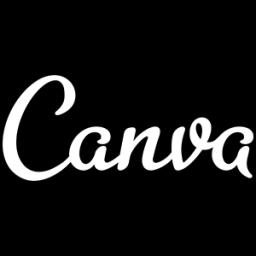
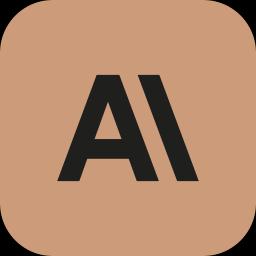
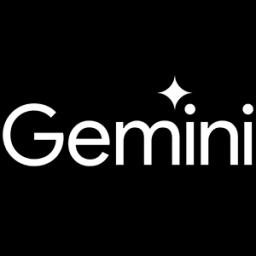
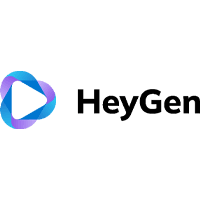
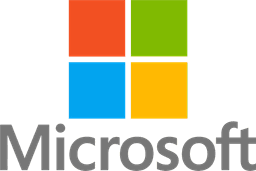
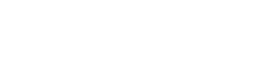
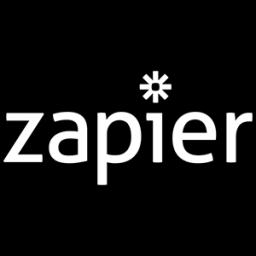
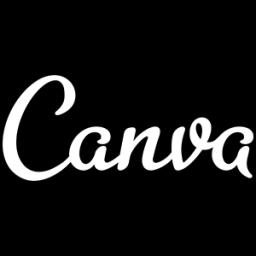
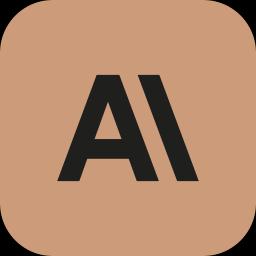
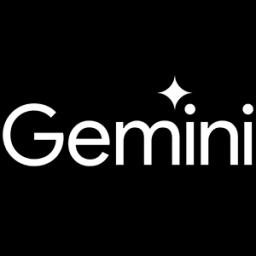
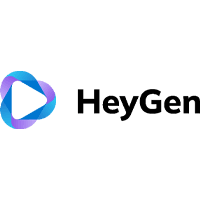
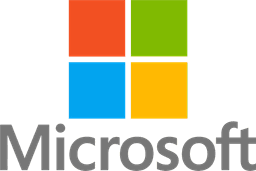
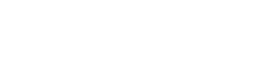
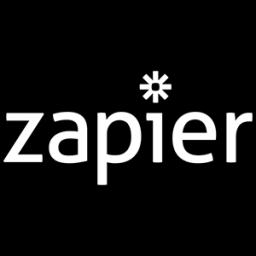
The capability of AI systems to learn from mistakes differs notably from human learning mechanisms. AI can indeed be retrained using corrected datasets and refined algorithms to minimize error recurrence. However, the learning process is not akin to the human experience, which involves reflection, emotional engagement, and cumulative wisdom. AI learning is inherently systematic, requiring explicit instruction and iteration rather than spontaneous adaptation.
These distinct modes of error and learning between AI and humans have profound implications for AI safety. Developing effective safety protocols necessitates a deep understanding of AI's error patterns to implement appropriate oversight measures. As AI continues to evolve, ensuring these systems are equipped to handle their unique error types safely is crucial, not only for their integration into society but also for maintaining public trust.
Expert opinions often converge on the predictability of AI errors as a potential advantage over the unpredictability of human mistakes. According to AI safety researcher David Winter, AI errors' systematic nature allows for precise modeling and error mitigation strategies, contrasting with the complex unpredictability of human errors. On the other hand, Anthropics' research team emphasizes the emerging risks associated with unexpectedly advancing AI systems, which could present sudden, unforeseen challenges despite the apparent predictability of their simpler counterparts.
Public reactions to AI errors versus human errors span a spectrum of concerns and perceptions. There exists a palpable anxiety regarding the potentially large-scale impact of AI errors, as they can propagate across systems with unprecedented speed and reach. Additionally, the lack of 'human-like' understanding in AI-induced mistakes often contributes to an underlying sense of distrust or unease, further amplified by the prevailing narrative of AI's unpredictability and unhuman nature.
Future implications of AI error patterns on society are multifaceted, impacting economic, social, and political domains. Economically, new frameworks around law, insurance, and risk assessment will emerge to address AI-specific challenges. Socially, as AI continues to integrate into daily life, it could either widen the gap between technology enthusiasts and skeptics or foster new collaborative norms. Politically, AI may become a focal point in regulatory debates, pushing towards international guidelines and standards that ensure balanced adoption and safety practices across regions.
Public Perception and Reaction to AI and Human Errors
The topic of AI errors versus human mistakes is increasingly drawing public interest, largely due to the distinct characteristics of errors made by each entity. AI errors often emerge from the biases entrenched in training data, limitations of algorithms, and rigid adherence to programmed patterns, which makes them fundamentally different from human errors that arise from contextual misinterpretations, emotional influences, and judgment errors.
Learn to use AI like a Pro
Get the latest AI workflows to boost your productivity and business performance, delivered weekly by expert consultants. Enjoy step-by-step guides, weekly Q&A sessions, and full access to our AI workflow archive.
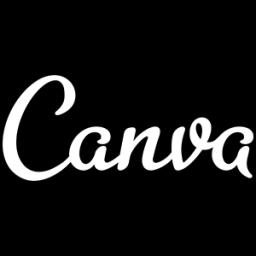
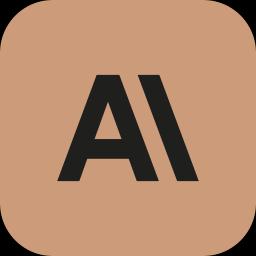
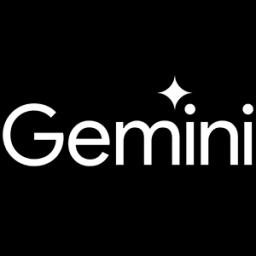
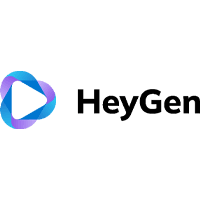
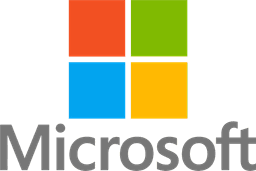
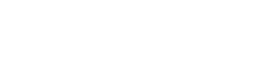
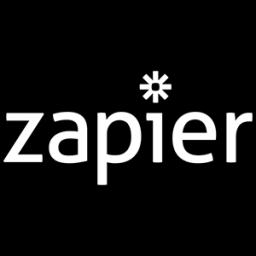
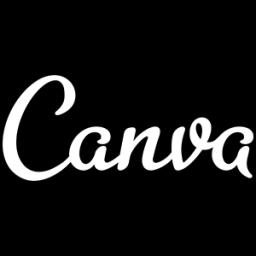
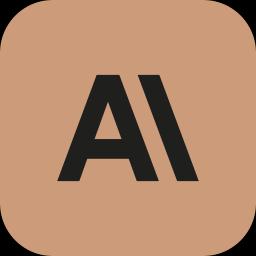
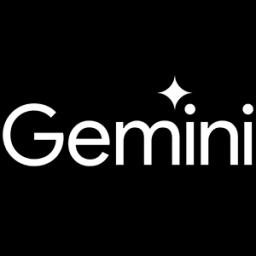
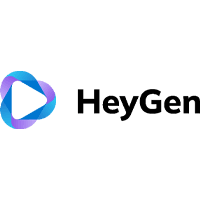
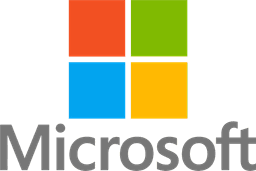
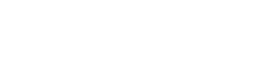
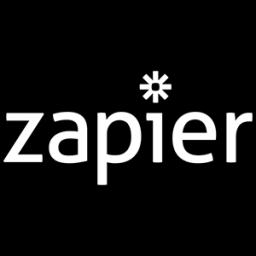
One major public concern surrounds the perception of AI errors as potentially more dangerous and unpredictable than human mistakes. This concern is amplified by the rapid scalability of AI errors, where a single mistake can impact millions, unlike human errors that typically affect only those directly involved. The rapid dissemination and amplification of AI errors through interconnected systems and devices mean that less time exists to identify and correct these issues before widespread impact.
Moreover, AI systems, while capable of retraining with corrected data, do not learn from mistakes in the intuitive manner humans do. They require external interventions to amend errors, whereas humans can reflect, adapt, and modify behavior autonomously based on experience. Such distinctions necessitate targeted safety measures and oversight mechanisms tailored to the unique nature of AI mistakes, crucial for ensuring the safety of AI deployments.
Public reactions reflect a mix of heightened anxieties and skeptical trust in AI. A general trust deficit exists, evidenced by reluctance in relying on AI for decision-making in critical contexts, stemming from fears of unanticipated errors and the "unnatural" feel of AI mistakes. Forums and social media often highlight these misgivings, sparking debates around AI's reliability and its potential to fully replace human decision-making processes.
The ongoing discourse also reveals a split between techno-enthusiasts who perceive AI errors as stepping stones for improvement and AI skeptics who view them as evidence of technology's inherent flaws. This division is not only fueling public dialogue but also influencing the trajectory of AI policy and research funding. As advanced AI systems continue to evolve, addressing these multifaceted public perceptions and reactions will be essential for balanced integration into society.
Future Implications of AI Mistakes in Society
Artificial Intelligence (AI) errors differ significantly from human errors, as highlighted by the title of the article, 'AI Mistakes Are Very Different Than Human Mistakes.' While the specific content of the article is inaccessible, the fundamental difference in error types sheds light on how each is formed. AI errors arise from biases in training data, limitations of algorithms, and strict adherence to patterns, compared to human mistakes which are often linked to contextual misunderstandings, emotions, and judgments. This distinction is vital in analyzing AI mistakes' future implications, especially concerning safety and societal integration.
One of the key questions involves whether AI can learn from its mistakes as humans do. While AI systems can be retrained with enhanced data and superior algorithms, they lack the intuitive learning process humans undergo through experience and reflection. For AI, new learning must be systematically integrated, unlike the more organic human learning curve. Thus, understanding the limitations in AI's learning capabilities is central to framing future oversight and learning adaptation systems.
Learn to use AI like a Pro
Get the latest AI workflows to boost your productivity and business performance, delivered weekly by expert consultants. Enjoy step-by-step guides, weekly Q&A sessions, and full access to our AI workflow archive.
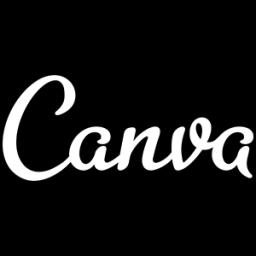
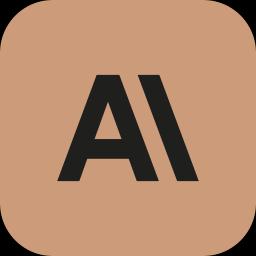
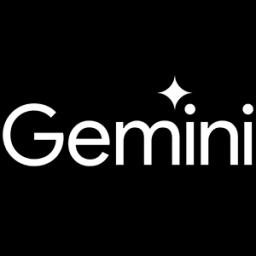
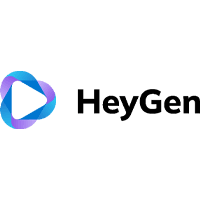
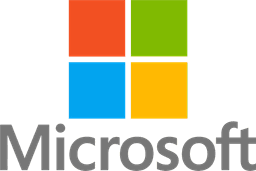
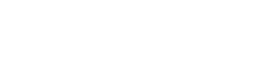
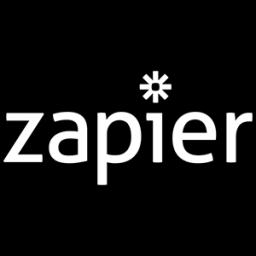
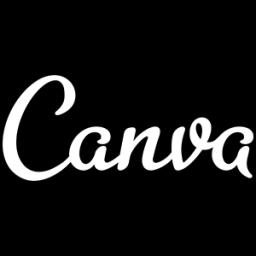
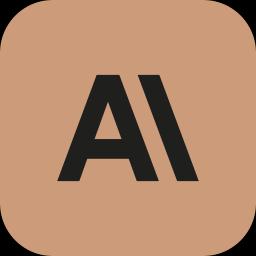
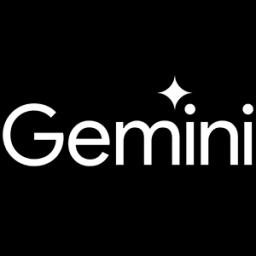
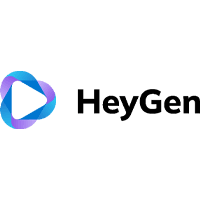
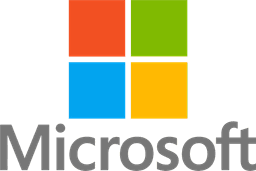
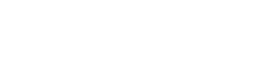
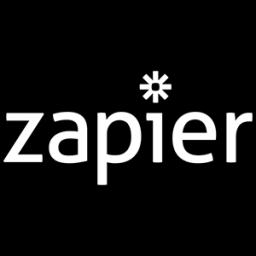
Another significant consideration is the implication of AI's error types on safety. Recognizing the inherent differences and potential risks associated with AI requires developing sophisticated safety measures and governance frameworks. Recent regulatory advancements such as the EU AI Act, and safety initiatives by leading AI organizations like DeepMind and OpenAI, underscore the collective effort to mitigate AI errors and enhance error-tracking capabilities. These steps are foundational for future AI integration into critical societal roles.
The societal acceptance and trust in AI systems hinge on these differences. Public reactions often highlight concerns over the unpredictability and scale at which AI errors can emerge. The perceived severity and 'unnatural' feel of AI errors amplify anxiety levels and foster a general trust deficit. Overcoming these challenges demands transparent mechanisms for AI decision-making and error reporting, promoting trust while fostering a balanced understanding of AI's role in augmenting human judgment.
AI's broader societal implications in the future will likely revolve around economic, social, and political spheres. Economically, the insurance and regulatory landscapes must adapt, providing for AI-specific risk assessments and compliance burdens. Socially, trust and adoption norms will evolve, potentially leading to polarized opinions regarding AI reliance in critical versus non-critical tasks. Politically, a drive for uniform global standards may arise, aligning international governance on AI safety and ethical usage limits.