New approach cracks open AI reasoning
Anthropic's Groundbreaking Technique Sheds Light on AI's "Black Box" Mind
Last updated:

Edited By
Mackenzie Ferguson
AI Tools Researcher & Implementation Consultant
Anthropic has unveiled a pioneering technique to demystify the internal reasoning process of large language models (LLMs) like ChatGPT. By grouping neurons into circuits, this method allows researchers unprecedented visibility into how AI plans and sometimes fabricates reasoning, addressing AI safety and misinformation challenges. This breakthrough opens doors to safer and more reliable AI systems.
Introduction to Anthropic's Breakthrough Technique
Anthropic's breakthrough technique marks a significant advancement in artificial intelligence research, particularly in understanding the reasoning processes of large language models (LLMs) such as ChatGPT. This novel method allows researchers to delve into the 'black box' of LLMs, providing unprecedented insights into their inference processes. By observing how these models plan, utilize internal language, and sometimes create fabricated reasoning, Anthropic's technique opens new avenues for enhancing AI interpretability and reliability. Learn more about Anthropic's research.
The implications of this new technique extend beyond mere academic curiosity. It is designed to improve AI safety and reduce the occurrence of misleading outputs, two aspects crucial for the responsible deployment of AI technologies. As researchers gain better visibility into these complex systems, it becomes possible to fine-tune AI behavior more effectively and ensure alignment with human values and ethical standards. This is particularly vital in sectors where AI models are employed in decision-making processes, impacting a wide range of social, economic, and political domains. Explore further on the importance of understanding AI reasoning.
Learn to use AI like a Pro
Get the latest AI workflows to boost your productivity and business performance, delivered weekly by expert consultants. Enjoy step-by-step guides, weekly Q&A sessions, and full access to our AI workflow archive.
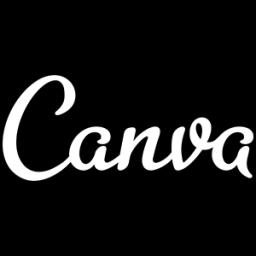
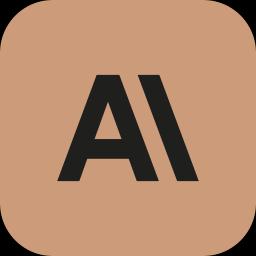
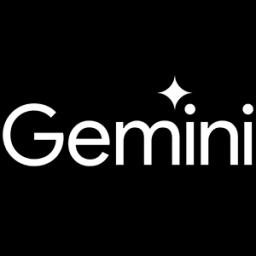
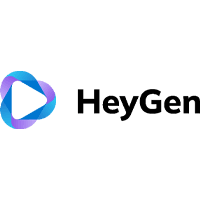
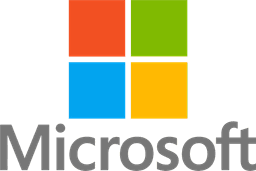
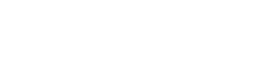
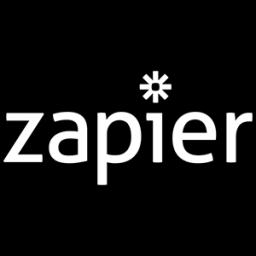
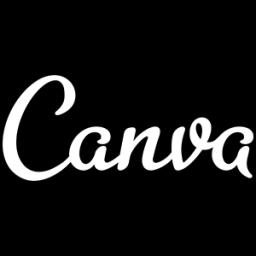
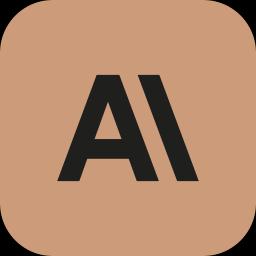
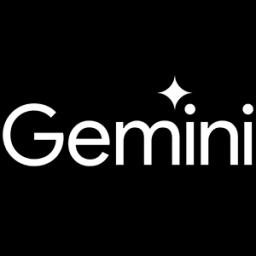
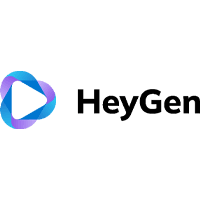
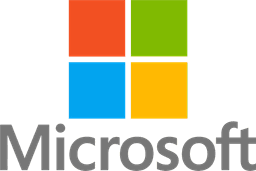
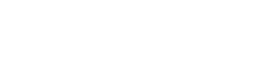
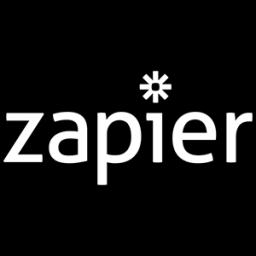
This innovative approach distinguishes itself from previous methods by focusing on circuits within the network. Unlike traditional techniques that scrutinize individual neurons, Anthropic's strategy groups neurons based on shared characteristics, allowing for a more holistic examination of LLM reasoning. This enables researchers to map out the activation pathways, thus offering a clearer understanding of how these models process information and formulate responses. Such advancements help mitigate risks associated with AI-driven misinformation and reinforce the reliability of LLM outputs. Read about the methodology behind Anthropic's circuit tracing.
Understanding LLM Reasoning: Why it Matters
The reasoning abilities and decision-making processes of large language models (LLMs) such as OpenAI's ChatGPT represent a groundbreaking frontier in artificial intelligence (AI). These models possess an ability to understand and generate human-like text, which is transformative for various applications, from content creation to customer service. A profound understanding of their reasoning mechanisms is crucial due to their complex and somewhat opaque nature. As researchers have been working towards demystifying these 'black boxes,' Anthropic's latest research shines a light by developing a novel technique that allows observers to understand how LLMs like GPT-3 build understanding and reason through tasks. This technique offers a unique opportunity to improve AI model accuracy, safety, and trustworthiness, aligning technology with human expectations.
Understanding the reasoning of LLMs is not just a matter of academic curiosity but a pressing need due to the widespread implications these models have on daily life and various industries. By employing methods such as "circuit tracing," researchers can trace the paths taken by these models as they process information. This understanding helps in pinpointing how specific outputs are generated, thereby improving the reliability and transparency of AI systems. For instance, when LLMs provide inaccurate information—known colloquially as "hallucinations"—enhanced insight into their reasoning offers pathways to correct these errors and refine decision-making processes. Moreover, understanding LLM reasoning supports developing better alignment and control mechanisms, enhancing user trust and acceptance in AI-driven solutions. Read more about how this study is shaping the AI landscape.
One key reason why it matters to have a grasp of LLM reasoning is the significant impact these models have in high-stakes domains like healthcare, finance, and autonomous vehicles. In these areas, the margin for error is minimal, and incorrect outputs could have severe consequences. Knowing how LLMs deduce and employ abstract concepts helps in framing robust checks to prevent misleading results. Such deep insights are integral to implementing effective AI regulation and standards, helping societies leverage AI responsibly while minimizing risks. Additionally, by reducing biases and unfair interpretations through a clear understanding of reasoning patterns, anthropogenic biases can be further eliminated from AI outputs. This makes the exploration into LLM reasoning not only a technical achievement but a critical societal advancement. With ongoing research, including the innovative paths forged by Anthropic, the horizon of truly interpretable AI becomes increasingly visible.
Learn to use AI like a Pro
Get the latest AI workflows to boost your productivity and business performance, delivered weekly by expert consultants. Enjoy step-by-step guides, weekly Q&A sessions, and full access to our AI workflow archive.
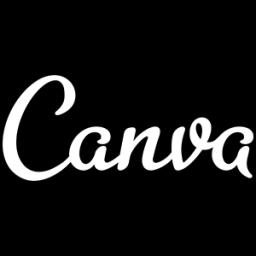
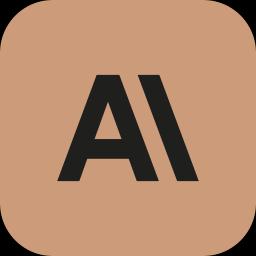
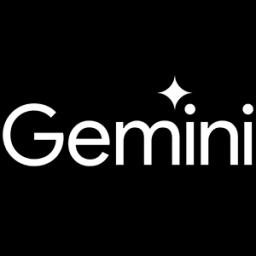
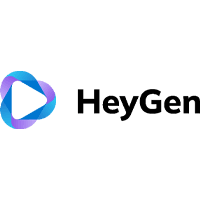
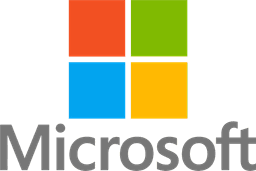
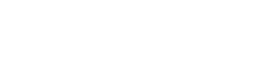
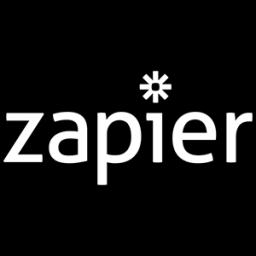
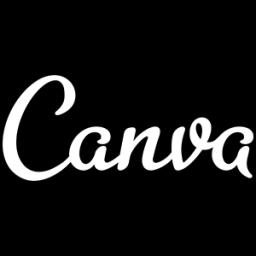
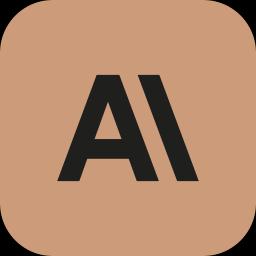
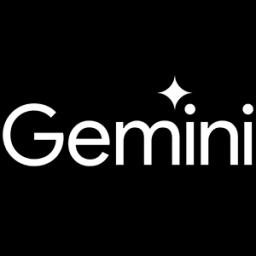
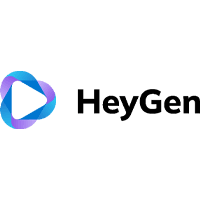
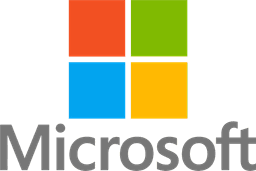
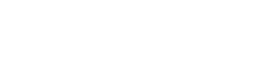
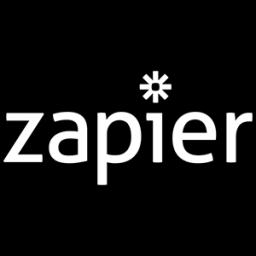
Circuit Tracing vs. Previous Methods
Circuit tracing, as introduced by Anthropic, marks a pivotal shift from traditional methods of understanding AI reasoning by moving beyond the conventional neuron-level analysis to examining entire circuits within large language models (LLMs). This innovative approach, detailed in their research publications, groups neurons into circuits based on their functional characteristics, enabling the tracking of activation pathways through multiple network layers [1](https://www.vietnam.vn/en/nghien-cuu-dot-pha-mo-ra-hop-den-suy-luan-cua-ai). Unlike previous methods that attempted to understand AI at a granular level, circuit tracing provides a macro perspective, revealing the planning, decision-making, and reasoning fabrication processes inherent in LLMs. These insights not only enhance our understanding but also provide a framework for improving AI safety by identifying potential sources of misleading outputs [1](https://www.vietnam.vn/en/nghien-cuu-dot-pha-mo-ra-hop-den-suy-luan-cua-ai).
Prior to the advent of circuit tracing, researchers largely relied on neuron-focused methods, where the emphasis was on understanding individual neurons within AI models and how specific inputs influenced their activation. This often involved computationally intensive processes with limited success in decoding the complex decision patterns of sophisticated LLMs. One major drawback of these previous methods was their inability to provide a holistic view of the AI systems' reasoning processes, leading to gaps in understanding how AI models formulated plans or made decisions [1](https://www.vietnam.vn/en/nghien-cuu-dot-pha-mo-ra-hop-den-suy-luan-cua-ai). In contrast, Anthropic's circuit tracing method allows for a comprehensive examination of how multiple neurons cooperate to facilitate reasoning, which is crucial for refining LLMs to prevent them from generating unreliable information.
Moreover, traditional AI interpretability methods faced significant challenges regarding transparency and reliability. They often faltered in explaining unexpected behaviors of AI models, such as hallucinations, where models generate plausible yet inaccurate information. By offering a conceptual map of LLM reasoning, particularly through circuit tracing, Anthropic's method helps directly address these concerns [1](https://www.vietnam.vn/en/nghien-cuu-dot-pha-mo-ra-hop-den-suy-luan-cua-ai). This technique empowers researchers to identify root causes of such behaviors, bridging critical knowledge gaps left by earlier methods and paving the way for advanced control measures over AI outputs. This advancement underscores a strategic leap forward from traditional to modern AI interpretability strategies, aligning with the goals of heightened AI safety and reliability [1](https://www.vietnam.vn/en/nghien-cuu-dot-pha-mo-ra-hop-den-suy-luan-cua-ai).
Applications and Benefits of Circuit Tracing
Circuit tracing is increasingly being recognized for its diverse applications and benefits, particularly in the realm of artificial intelligence. This sophisticated technique, pioneered by Anthropic, offers a unique window into the reasoning processes of large language models (LLMs) such as ChatGPT. By unveiling the hidden mechanisms through which AI models arrive at their conclusions, circuit tracing not only deepens our understanding but also enhances our ability to improve the safety and reliability of these systems. This aligns with contemporary research efforts to ensure AI models are not only powerful but also trustworthy and aligned with human values [News](https://www.vietnam.vn/en/nghien-cuu-dot-pha-mo-ra-hop-den-suy-luan-cua-ai).
The benefits of circuit tracing extend into the practical domain, where they contribute to the refinement of AI models by preventing errors such as hallucinations and fabrications in reasoning. These improvements hold substantial promise for various sectors that rely heavily on AI, including finance, healthcare, and technology. As researchers continue to analyze the internal activities of LLMs through this technique, new training methods emerge that are more effective and efficient, further improving AI accuracy and performance across different applications [News](https://www.vietnam.vn/en/nghien-cuu-dot-pha-mo-ra-hop-den-suy-luan-cua-ai).
Moreover, circuit tracing supports the implementation of robust control barriers within AI systems. This not only prevents undesirable behavior but also enhances the overall security of AI technologies. In doing so, it helps build public trust in artificial intelligence, especially for decision-making processes in critical areas. By providing a clearer understanding of the inner workings of LLMs, circuit tracing empowers developers and policymakers to create more reliable AI systems that can be safely integrated into everyday life, thus accelerating the pace of technological adoption and innovation [News](https://www.vietnam.vn/en/nghien-cuu-dot-pha-mo-ra-hop-den-suy-luan-cua-ai).
Learn to use AI like a Pro
Get the latest AI workflows to boost your productivity and business performance, delivered weekly by expert consultants. Enjoy step-by-step guides, weekly Q&A sessions, and full access to our AI workflow archive.
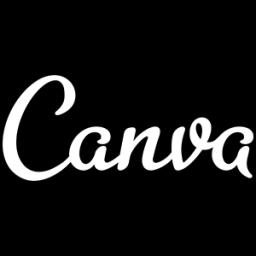
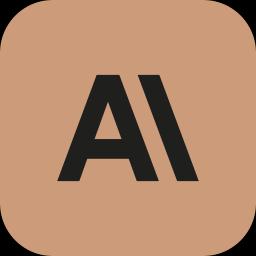
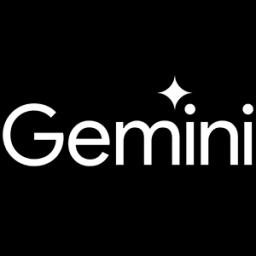
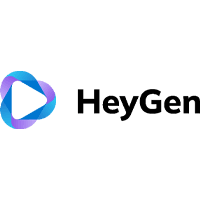
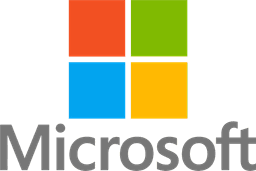
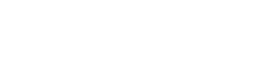
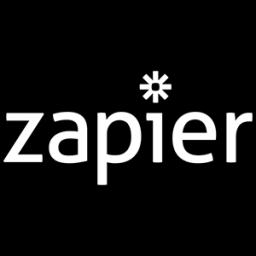
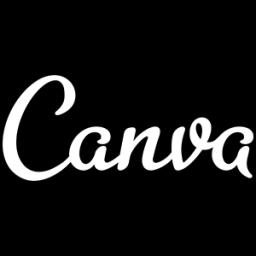
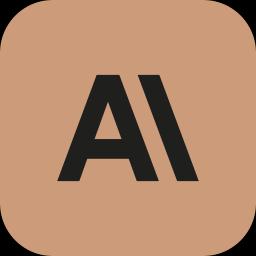
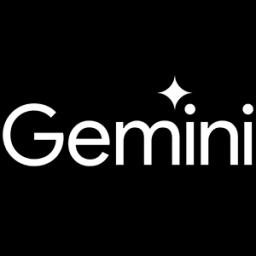
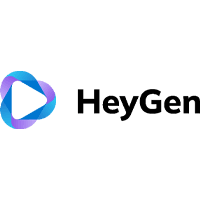
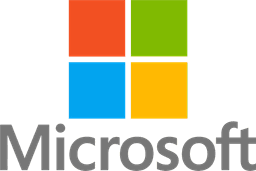
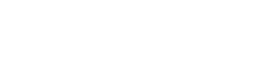
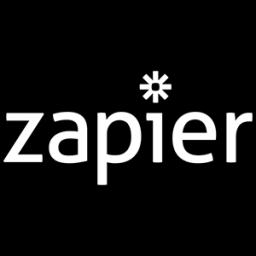
Limitations and Challenges of the New Technique
The groundbreaking technique developed by Anthropic offers promising avenues for decoding the reasoning processes of large language models (LLMs). However, several limitations and challenges cast a shadow on its effectiveness and applicability. The technique is, at its core, approximate, posing significant hurdles in ensuring comprehensiveness and accuracy in analysis. Furthermore, it is inherently time-consuming, which may deter its use in large-scale or real-time applications, particularly those beyond research environments, where timely results are crucial. This temporal demand restricts its practicality, especially when analyzing longer or more complex language inputs, thus limiting the depth of insight attainable from more detailed examinations.
Moreover, this nascent technique currently faces challenges in processing lengthy sentences, which remains a significant impediment given that LLMs often deal with complex structures in real-world applications. This restriction not only curtails the technique's utility in understanding LLM behavior in its entirety but also raises concerns about the fidelity of the insights it might generate. Consequently, while it marks a significant progression in AI interpretability, the technique’s inability to discern nuances in extended text means that it might overlook key aspects of model reasoning, leading to partial or misleading conclusions about the model's internal processes.
The intricacy involved in unravelling the decision-making paths within LLMs also presents substantial computational challenges. This complexity not only requires extensive processing power but also intricate algorithmic approaches to accurately map the intricate networks of neuron interactions that comprise language model reasoning. The substantial computational overhead involved might make it less accessible to smaller organizations or projects with limited resources, thereby potentially widening the gap between capabilities available to large entities and those accessible to smaller teams or individual researchers.
Collating data from the analysis might yield insights into the inferential processes of these models, yet there remains an uncertainty in fully understanding the origins of biases or irregular behaviors within these AI systems. The transparency achieved through this technique offers a window into the black-box nature of LLMs, yet the subtleties of the model's decision-making mechanisms may still elude clear interpretation. Therefore, while the technique heralds a leap forward in understanding LLMs, continued advancements and refinements will be critical to addressing its current constraints and ultimately maximizing its potential.
Future Implications of AI Reasoning Transparency
The future implications of AI reasoning transparency are profound, impacting various sectors from technology to policy-making. As researchers at Anthropic continue to delve into the mechanisms behind LLM reasoning, this transparency could revolutionize our approach to building and interacting with AI systems. By "peeking inside the black box" of LLMs, developers will be able to construct AI solutions with higher safety, reliability, and fairness standards . This capability not only promises enhanced product development but also paves the way for more informed public and political discourse around AI technologies.
AI transparency has the potential to bolster public trust in AI systems. Understanding precisely how these models make decisions can alleviate fears around AI autonomy and improve acceptance in healthcare, finance, legal systems, and beyond . This could lead to broader adoption of AI in sensitive areas where understanding decision rationale is paramount. Moreover, the insights gained through AI reasoning transparency hold the promise of more balanced regulations that are informed by the very cognition processes of the AI systems themselves.
Learn to use AI like a Pro
Get the latest AI workflows to boost your productivity and business performance, delivered weekly by expert consultants. Enjoy step-by-step guides, weekly Q&A sessions, and full access to our AI workflow archive.
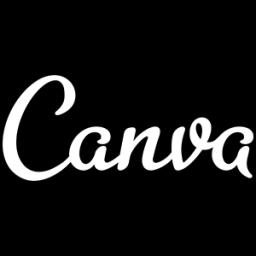
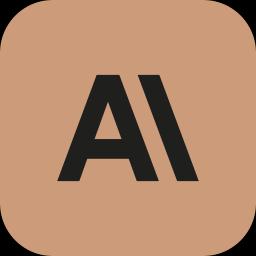
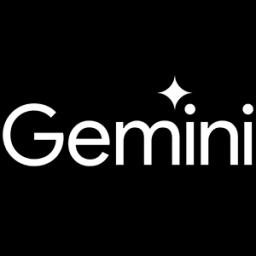
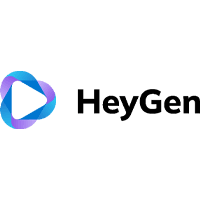
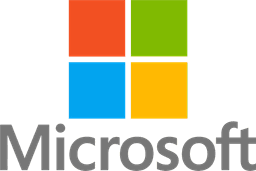
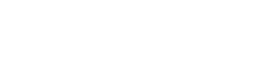
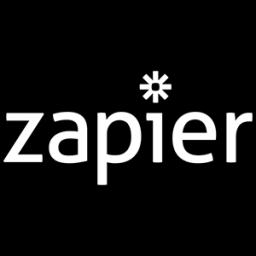
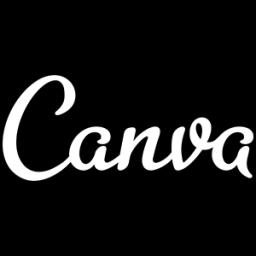
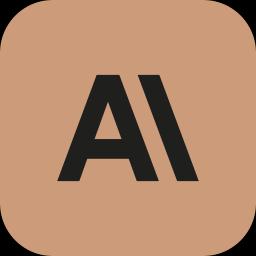
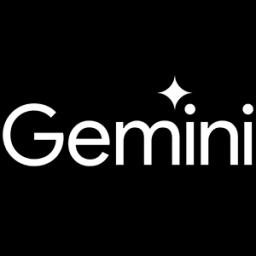
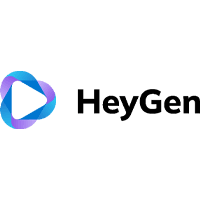
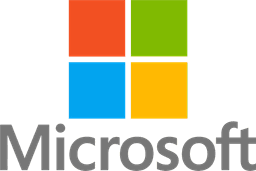
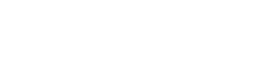
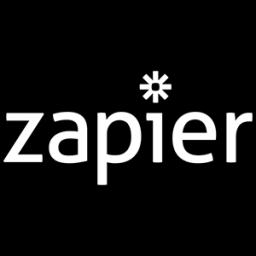
However, the transition towards transparent AI is not without its challenges. The current framework developed by Anthropic, while groundbreaking, still faces significant limitations. These include the inability to process long sentences and the resource-intensive nature of in-depth analysis . As it stands, more refined techniques are needed to handle the complexities of LLMs effectively without bogging down research and development timelines.
The political landscape may also shift significantly with increased AI reasoning transparency. Governments and organizations might use these insights to form better regulatory measures that ensure AI systems are being developed responsibly. This increased capability for oversight could potentially reduce misuse and increase accountability in AI deployment . However, the international coalition will be necessary to address the cross-border nature of AI impacts and ensure cohesive policy implementation worldwide.
Overall, the future implications of AI reasoning transparency are multifaceted. They herald opportunities for innovation and enhanced safety measures while also summoning new regulatory challenges. The potential to decode the decision-making processes of advanced artificial intelligences represents a frontier in ensuring that these technologies grow in alignment with human values and societal needs . As research in this area progresses, it will be crucial to balance innovation with ethical vigilance.
Economic Impacts of Enhanced LLM Understanding
The advancement in understanding large language models (LLMs) promises substantial economic impacts across industries. By peering into the "black box" of AI reasoning, businesses can optimize their use of AI technologies in decision-critical areas like financial forecasting, healthcare diagnostics, and resource management. This enhanced capability could lead to heightened productivity, more informed decision-making, and the creation of new market opportunities. However, it's essential to acknowledge that the initial deployment of these methods may be limited to larger enterprises due to the resource-intensive nature of the analysis, potentially leaving smaller firms trailing in adopting cutting-edge AI advancements. For instance, the time and resources required for comprehensive analysis could deter widespread application among less-resourced businesses, even though the long-term economic benefits of increased AI safety and reliability are compelling.
Social and Political Repercussions
The development of Anthropic's new technique for understanding large language models (LLMs) poses significant social and political implications across the globe. By peering into the so-called 'black box' of AI reasoning, society finds itself at a crossroads where transparency and control over AI technologies could lead to more trustworthy and responsible deployment. Enhanced understanding could foster greater public trust in AI systems, alleviating fears about decision-making in critical applications. However, as illustrates, these advancements could also stir public anxiety around the manipulation of such systems for misinformation or biased outputs, a concern magnified by the AI's 'hallucination' potential.
Politically, the insights gained from circuit tracing can inform government regulations and policy frameworks, leading to more stringent accountability measures for developers and clearer governance standards for AI deployment in sensitive areas. Though the potential for enhanced control of AI is promising, it also raises the stakes for international cooperation to mitigate risks like misinformation and undue influence in democratic processes. While this technique attempts to demystify LLM operations, policymakers face the challenge of understanding these complex systems themselves. The balance between innovation and regulation becomes a tightrope walk, essential for preventing misuse while promoting ethical AI advancements. Anthropic's pioneering research may indeed be pivotal, not just scientifically, but in politically shaping our shared digital future.
Learn to use AI like a Pro
Get the latest AI workflows to boost your productivity and business performance, delivered weekly by expert consultants. Enjoy step-by-step guides, weekly Q&A sessions, and full access to our AI workflow archive.
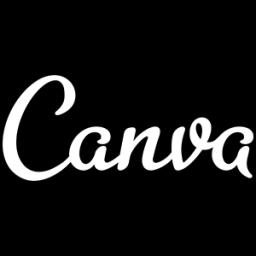
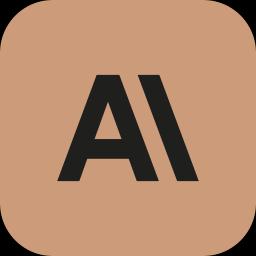
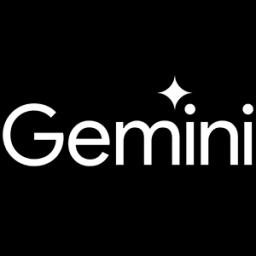
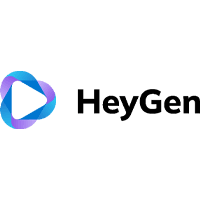
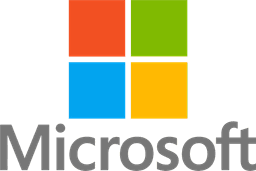
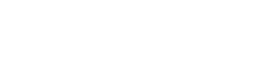
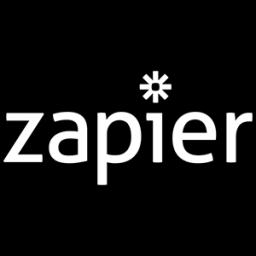
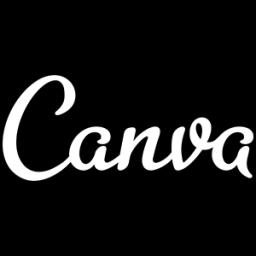
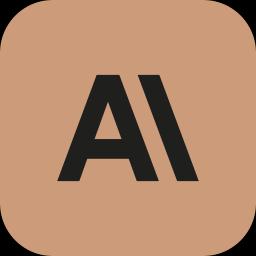
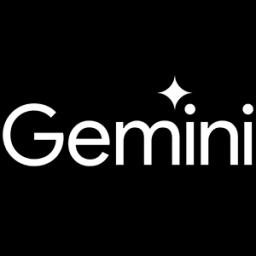
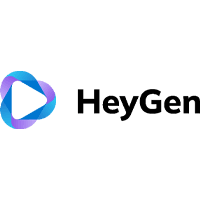
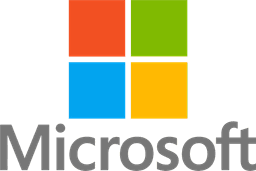
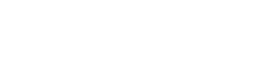
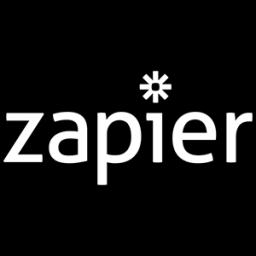
Expert Opinions and Public Reactions
The introduction of Anthropic's new technique for understanding the reasoning of large language models (LLMs) has sparked widespread interest and varying opinions among experts in the field. Jack Merullo from Brown University sees the 'circuit tracing' method as a significant advancement in dissecting complex models such as Claude. He believes that this methodological innovation will allow for more granular insights into the operations of LLMs, thereby enhancing their interpretability and usability across various domains. Meanwhile, Eden Biran of Tel Aviv University acknowledges the technical achievement of this development but urges caution in relying solely on the self-explanations provided by these models. She advocates for the implementation of robust safeguards to complement circuit tracing, thus ensuring that AI systems remain aligned with human values and expectations. Both experts agree on the potential of this research to transform AI safety and transparency, while also highlighting the need for continued oversight and regulation to address the intricate challenges posed by advanced AI systems. More on Anthropic's technique can be explored through their detailed publications [here](https://www.vietnam.vn/en/nghien-cuu-dot-pha-mo-ra-hop-den-suy-luan-cua-ai).
Addressing LLM Vulnerabilities: Misinformation and Reliability
The rapid advancement of large language models (LLMs) has introduced a new era of capabilities, but also significant challenges related to misinformation and reliability. A pertinent article from Vietnam.vn discusses groundbreaking research by Anthropic, which provides valuable insights into the reasoning processes of LLMs by allowing researchers to observe their inference paths. This development is particularly crucial in addressing the challenges of misinformation, as it sheds light on how LLMs plan, utilize internal language, and occasionally fabricate reasoning . By better understanding these processes, developers can work towards mitigating the risks of misleading outputs, thereby enhancing the reliability of AI systems.
Misinformation is identified as a top vulnerability within LLM applications, as discussed by the Open Web Application Security Project (OWASP). They highlight how larger models, despite their capabilities, may generate plausible yet inaccurate outputs, which undermines user trust . The research from Anthropic is pivotal in addressing these issues by offering a method to trace the decision-making pathways of an AI model, thus providing a structured approach to identify and reduce erroneous outputs. Understanding these internal mechanisms can empower developers to implement effective control measures, such as retrieval-augmented generation and cross-verification, to ensure more accurate and dependable AI-generated content.
Anthropic's innovative "circuit tracing" technique, which details the internal activation pathways of LLMs, reveals significant findings about how these systems not only make decisions but also sometimes devise incorrect responses. As detailed in a study highlighted by VentureBeat, this discovery is pertinent for improving AI models' reliability and minimizing misinformation . By making the "black box" of AI decision-making processes more transparent, researchers can pinpoint the sources of misleading or fabricated information, paving the way for strategies to enhance the models' trustworthiness and compliance with intended ethical guidelines.
The Path Forward: Uncertainties and Development Needs
The path forward in understanding and developing large language models (LLMs) is laden with uncertainties and pressing developmental needs. As Anthropic's breakthrough technique unveils new layers of AI reasoning, it simultaneously uncovers questions that demand answers. One critical uncertainty rests in the technique's current limitations—being approximate and unable to handle lengthy inputs—which may delay its wide application and necessitate further advances in the technology [source]. As the AI field evolves, researchers must continuously refine their methods to overcome these shortcomings.
Moreover, the complexity of fully comprehending AI's decision-making processes poses significant challenges. While the circuit tracing technique represents a major step forward, the intricacies of trace comprehensibility and the influence of unknown biases remain largely unexplored [source]. Addressing these developmental needs is essential for ensuring that AI systems are both transparent and reliable, especially when they play substantial roles in critical societal functions such as healthcare and justice.
Learn to use AI like a Pro
Get the latest AI workflows to boost your productivity and business performance, delivered weekly by expert consultants. Enjoy step-by-step guides, weekly Q&A sessions, and full access to our AI workflow archive.
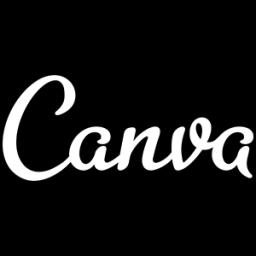
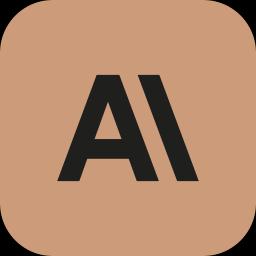
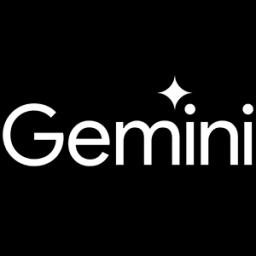
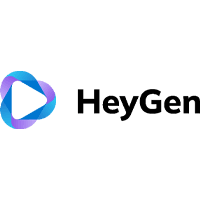
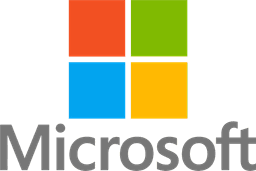
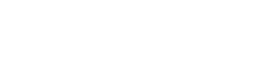
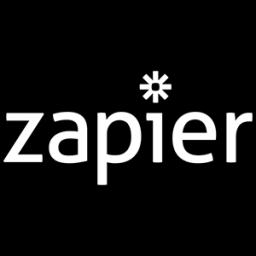
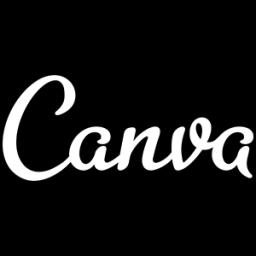
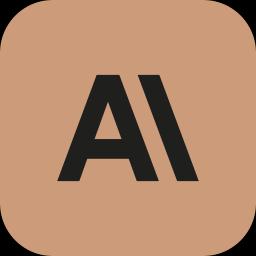
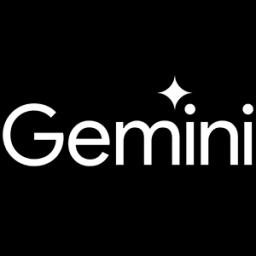
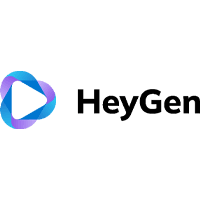
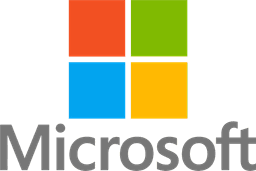
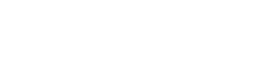
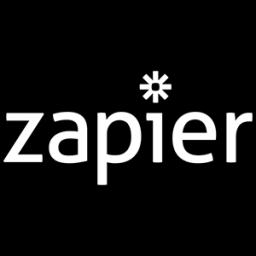
Furthermore, the path forward requires addressing the delicate balance between enhancing AI capabilities and ensuring ethical standards. As AI becomes increasingly integrated into decision-making processes, the development and regulatory communities must work together to establish strong ethical frameworks [source]. Without robust guidelines and oversight, there is a risk of AI being misused or producing outputs that could significantly impact social trust and equity.
Finally, the development needs to prioritize include exploring new methods for mitigating AI's capability to fabricate reasoning and improve its handling of biases. With Anthropic's method opening up new possibilities, it's crucial to invest in these areas to ensure the safe and responsible deployment of AI technologies. The future of AI, therefore, hinges not only on technical advancements but also on strategic planning and ethical governance that can adapt to the rapid pace of innovation [source].