AI Showdown
Battle of the Minds: Comparing GPT-4.1, GPT-4o, and o3 Models in Logic Puzzles
Last updated:

Edited By
Mackenzie Ferguson
AI Tools Researcher & Implementation Consultant
In a head-to-head comparison, OpenAI's GPT-4.1, GPT-4o, and o3 models tackle logic puzzles to determine which AI exhibits the most logical prowess. All models successfully solved the puzzles with GPT-4.1 praised for its clarity. Despite the strong performance across the board, users and experts weigh in on the practical differences.
Introduction to AI Model Evaluation
In the rapidly evolving field of artificial intelligence, model evaluation has emerged as a critical component of development and deployment processes. As AI systems become increasingly sophisticated, the methods used to assess their performance must also advance. Evaluating AI models involves not only comparing raw computational power and efficiency but also understanding how these models handle complex tasks such as logical reasoning, adaptability, and user interaction. A clear example of this is highlighted in a recent comparison of AI models conducted using logic puzzles. The evaluation process underscores the importance of selecting appropriate criteria and benchmarks that reflect an AI model's real-world application capabilities.
Logic-based evaluation offers a nuanced understanding of AI model performance by focusing on problem-solving ability, clarity of reasoning, and consistency in responses. As illustrated in a comparison study, different models such as GPT-4.1, GPT-4o, and o3 have been tested using a variety of logic puzzles to determine their strengths in logical reasoning. The findings reveal that while all models can solve these puzzles, each exhibits unique characteristics—GPT-4.1, for example, is recognized for its detailed explanations, enhancing its appeal for tasks requiring transparency and precision. This kind of evaluation provides valuable insights, allowing developers and users to choose models that best meet their specific needs.
Learn to use AI like a Pro
Get the latest AI workflows to boost your productivity and business performance, delivered weekly by expert consultants. Enjoy step-by-step guides, weekly Q&A sessions, and full access to our AI workflow archive.
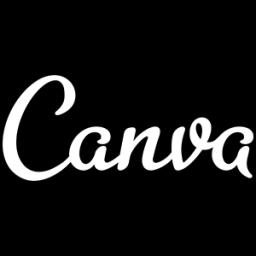
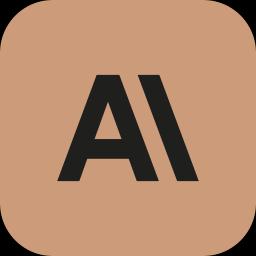
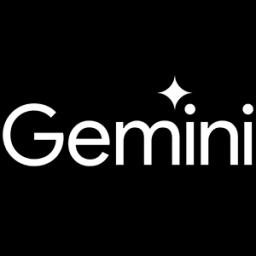
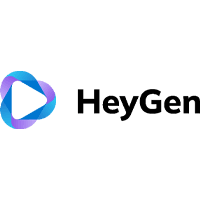
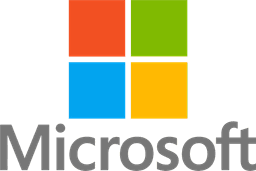
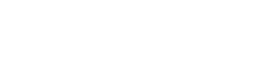
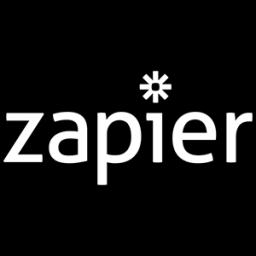
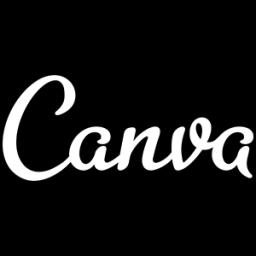
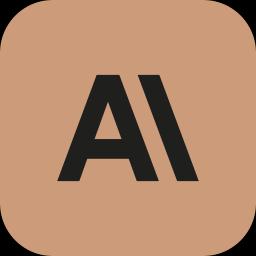
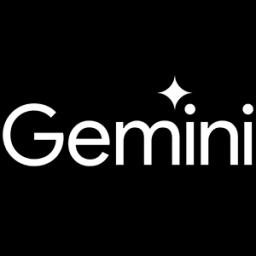
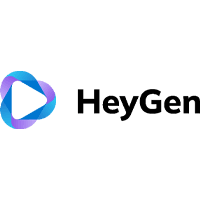
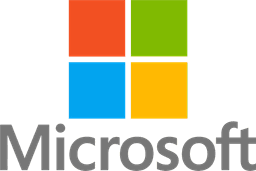
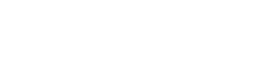
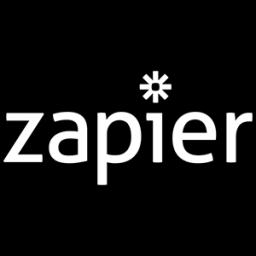
The growing emphasis on logical reasoning in AI model evaluation reflects broader trends within the technology landscape. With AI systems being integrated into more aspects of daily life and professional environments, stakeholders are increasingly demanding transparent and interpretable outputs. This demand aligns with ongoing efforts by AI developers to maintain transparency and safety in AI applications. OpenAI's recent commitment to frequent safety evaluations and transparency illustrates this shift. Evaluations using logic puzzles offer a versatile and accessible means of showcasing model capabilities, engaging both technical and non-technical audiences alike, thus reinforcing confidence in AI technology.
Moreover, the results of logic-based AI evaluations have far-reaching implications. As AI models demonstrate proficiency in solving complex logic puzzles, their potential applicability extends to various domains such as automated customer support, educational tools, and beyond. This potential not only underscores the transformative power of AI technologies but also raises important considerations about the future labor market, where AI could significantly alter workforce dynamics. As AI models continue to improve, continuously refined evaluation approaches will be crucial in ensuring they meet diverse industry demands while addressing ethical concerns and promoting equitable technological advancement.
Comparing GPT-4.1, GPT-4o, and o3: Key Differences
The comparison between GPT-4.1, GPT-4o, and o3 models highlights distinct characteristics that differentiate each in terms of logical reasoning capabilities. GPT-4.1 emphasizes a high standard in logical reasoning and coding, boasting an ability to provide detailed and clear explanations. This makes it particularly useful in scenarios where users require deep understanding or step-by-step problem solving. On the other hand, GPT-4o serves as the standard model and offers a balance of features. It lies in between by providing responses that are both concise and detailed, making it versatile for many tasks without an extreme focus. Lastly, the o3 model is crafted for complex reasoning, offering concise and direct answers that are efficient for experienced users who prefer brevity over detailed discourse. [Read more here](https://www.techradar.com/computing/artificial-intelligence/i-compared-chatgpt-4-1-to-o3-and-4o-to-find-the-most-logical-ai-model-the-result-seems-irrational).
Each model exhibits its unique strengths through specially designed logic puzzles. These puzzles, which include determining the fullness of a wine barrel and solving wordplay, serve as a robust medium to evaluate the logical prowess of AI models. GPT-4.1, in these exercises, is praised for articulating its reasoning process clearly, which can be particularly beneficial for users who require comprehensive guidance. In contrast, o3, with its focus on high-octane reasoning, manages to deliver accurate yet succinct solutions, suited for those who appreciate efficiency in communication. Meanwhile, GPT-4o strikes a balance by providing solutions that nod to both detail and succinctness, proving its versatility as a well-rounded model for a diverse range of logic-based tasks.
Learn to use AI like a Pro
Get the latest AI workflows to boost your productivity and business performance, delivered weekly by expert consultants. Enjoy step-by-step guides, weekly Q&A sessions, and full access to our AI workflow archive.
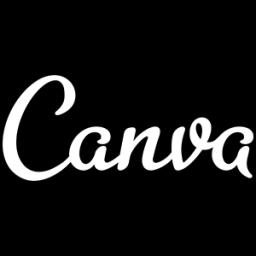
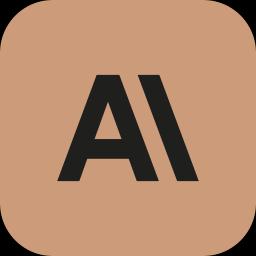
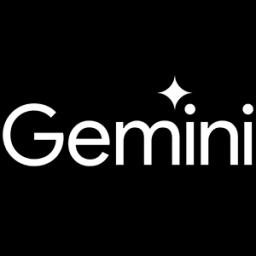
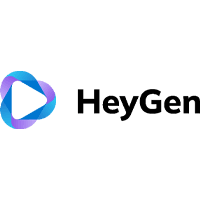
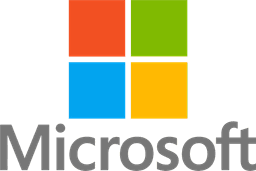
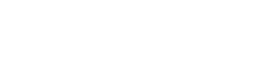
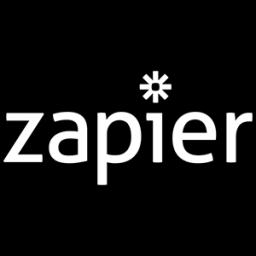
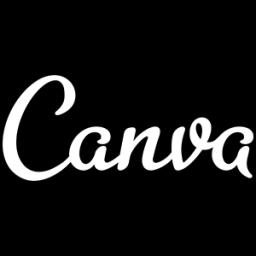
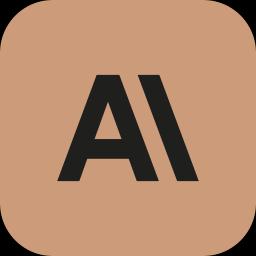
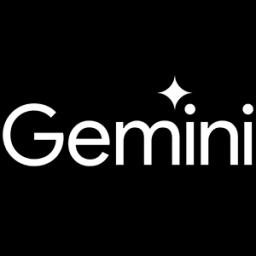
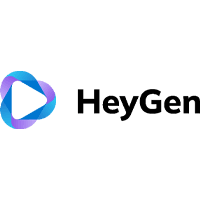
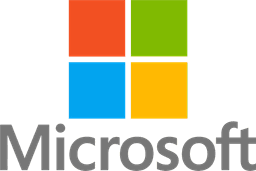
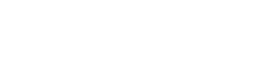
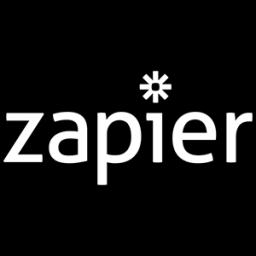
The public's reception of these AI models, particularly with the recent release of GPT-4.1, reflects both appreciation for advanced problem-solving capabilities and confusion over the sheer number of available models. For some, the distinction between models like GPT-4.1 and o3 is clear thanks to their specialized features, while others express frustration over the growing complexity and number of choices presented by OpenAI. This sentiment is echoed among tech enthusiasts and casual users alike, highlighting a need for clearer communication and guidance from OpenAI to help users navigate these offerings effectively. The article from [TechRadar](https://www.techradar.com/computing/artificial-intelligence/i-compared-chatgpt-4-1-to-o3-and-4o-to-find-the-most-logical-ai-model-the-result-seems-irrational) underscores the performance of these models, yet the surrounding discourse reveals the complication of multiple options in a rapidly evolving AI landscape.
Logic Puzzle Methodology in AI Testing
Logic puzzles have emerged as a novel methodology for assessing AI's cognitive abilities, especially within the realm of logical reasoning. Unlike traditional coding challenges, logic puzzles offer a more engaging and universally relatable means of evaluating an AI model's thinking processes. A recent comparison between OpenAI's GPT-4.1, GPT-4o, and o3 utilizes such puzzles to probe their reasoning capabilities . These puzzles are designed to mimic real-world reasoning tasks that human minds frequently tackle, making them an excellent tool for measuring AI adaptability in solving problems that require pattern recognition, deduction, and spatial understanding.
The comparison study highlighted how each AI model performed across different logic puzzles. These puzzles varied from finding a hidden cat, which involves intricate pattern recognition, to assessing the fullness of a wine barrel—a task requiring spatial intelligence and an understanding of basic physics principles. A wordplay question about the letter 'M' added another layer, testing the AI's ability to handle linguistic nuances . Such diversity in testing material ensures that the AI models are not just proficient in one type of logic task but can adapt to various logical reasoning scenarios, enhancing their utility in real-world applications.
An intriguing observation from the logic puzzle tests was the distinct approach taken by each AI model. GPT-4.1 stood out with its comprehensive and clear explanations, making it a preferred choice for users who value detailed reasoning. In contrast, the o3 model’s brevity in answers, while efficient, might not satisfy those who require more extensive explanations. GPT-4o, with its balanced approach, provides a middle ground, appealing to those who appreciate both conciseness and clarity . These differences underscore the importance of model selection based on specific user needs and preferences when applying AI to solve logic-based challenges.
The choice of logic puzzles over coding challenges is also a strategic decision aimed at broadening the appeal of AI evaluations. Logic puzzles do not require the user to have specialized technical knowledge, making the assessments accessible to a wider audience. This aspect not only makes AI testing more inclusive but also encourages public engagement in AI advancements. By emphasizing widely-appreciated cognitive tasks, the test becomes more about understanding and accessibility than technical prowess in coding . Such innovations in testing methodologies could serve to demystify AI technology and emphasize its practical benefits in everyday problem-solving.
Performance Analysis: Which AI Model Stands Out?
This comparison of AI models underscores a broader discussion in the AI community about the ease of selecting appropriate models for different tasks. The launch of GPT-4.1 added to the expanding lineup of models users must navigate, raising questions about the necessity of such variety. Critics and users alike express mixed reactions, noting the potential confusion caused by multiple available options. As reported by TechRadar, this proliferation of models might confuse those who are not deeply familiar with the intricacies of AI technology, despite the models' strong performance. This situation calls for improved communication and clearer guidelines from companies like OpenAI regarding model features and intended applications to aid users in making informed choices.
Learn to use AI like a Pro
Get the latest AI workflows to boost your productivity and business performance, delivered weekly by expert consultants. Enjoy step-by-step guides, weekly Q&A sessions, and full access to our AI workflow archive.
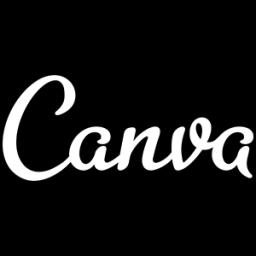
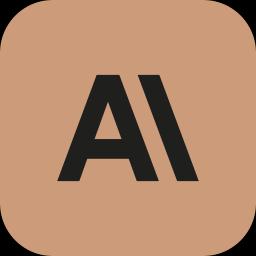
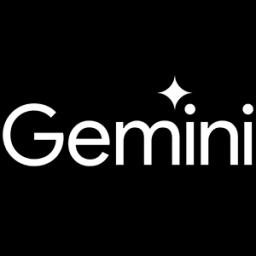
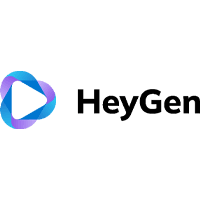
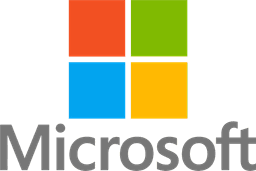
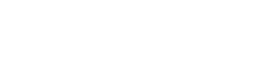
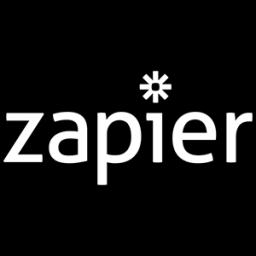
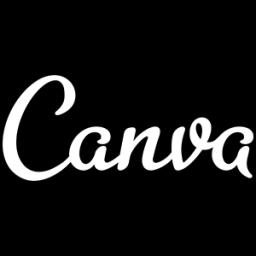
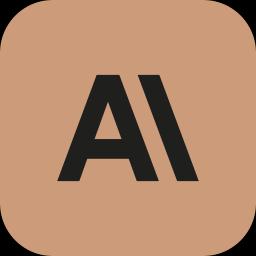
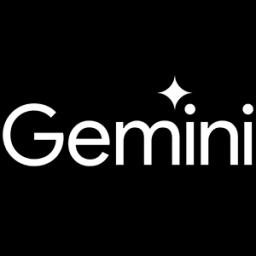
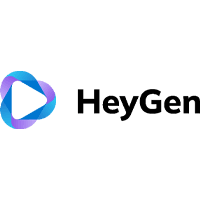
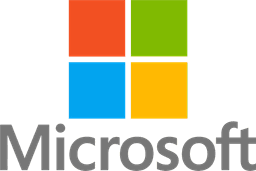
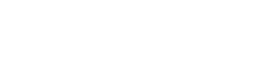
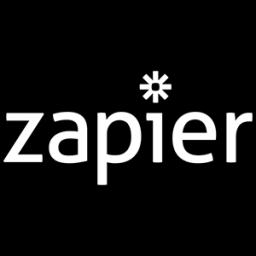
The Rationale Behind Choosing Logic Puzzles Over Coding Challenges
When analyzing the rationale behind preferring logic puzzles over coding challenges in AI testing, it's essential to focus on the broader applicability and engagement that logic puzzles offer. Many AI models, like GPT-4.1, have demonstrated their prowess in solving logic puzzles, which tend to be more universally relatable and less technically demanding than coding challenges. Logic puzzles, such as those used in recent tests—including the hidden cat puzzle and wine barrel conundrum—serve as a more accessible benchmark for diverse audiences, allowing for easier comparative evaluations of AI reasoning capabilities. Readers can explore the detailed comparison between GPT-4.1, GPT-4o, and o3's ability to tackle these puzzles in the TechRadar article.
Moreover, choosing logic puzzles aligns with the goal of enhancing the AI models' ability to mimic human-like reasoning. Logic-based tests not only assess pure computational capability but also evaluate how well models like OpenAI's iterations can interpret and solve problems that require nuanced understanding—a key aspect of human intelligence. Despite initial public confusion over the proliferation of different AI models, the consistency in problem-solving success across GPT-4.1, GPT-4o, and o3 using these puzzles underscores their efficacy in handling logic-based tasks. This approach provides a robust framework for future AI developments, fostering models that can integrate into everyday applications seamlessly. For a complete review of how different models performed in these puzzles, the article offers valuable insights.
Choosing logic puzzles over coding challenges is also a strategic decision that avoids the potential tedium associated with coding tasks. It ensures a wider appeal and engagement with both technical and non-technical audiences, which is crucial for public perception and acceptance. By focusing on logic puzzles, developers and researchers can highlight an AI's deductive reasoning skills without alienating non-coding readers. This broad appeal is significant as companies like OpenAI navigate public feedback and reactions, further explored in online discussions related to the diverse array of AI models available today (source).
Finally, logic puzzles as a testing medium signify a shift toward more cognitively demanding, yet broadly understandable, evaluation criteria. By embracing such challenges, AI models are better poised to achieve advances in fields requiring complex problem-solving skills, rather than niche programming tasks which may not effectively showcase an AI's potential to a lay audience. These puzzles test a model’s ability to not only provide answers but to do so with clarity and depth, characteristics that are increasingly in demand across various sectors. Through such an approach, the potential for AI integration into daily activities and decision-making processes becomes more tangible, opening avenues for discussions around ethical and social implications as outlined in various studies, including those on social AI impacts.
Public Perception and Reaction to AI Model Diversity
As the landscape of artificial intelligence continues to evolve, public perception of AI model diversity is critically shaped by the variety of available tools and their applications. OpenAI's GPT models, notably GPT-4.1, GPT-4o, and o3, showcase this diversity through their specialized capabilities in logic and reasoning. A recent comparison highlighted the relative strengths of these different models in solving logic puzzles, drawing attention to their nuanced differences and the resulting impact on user choice. Despite the improvements in AI performance, the vast selection can be bewildering for users, who sometimes feel overwhelmed by the options rather than empowered by them.
The reaction to AI model diversity is a blend of fascination and confusion. Users appreciate the clear, well-structured outputs of models like GPT-4.1 but often express dismay over the proliferation of models and the lack of guidance in selecting the one best suited for a particular task. This sentiment is amplified by discussions on platforms such as Reddit, where community members ponder the necessity of having multiple models when only the most effective ones could be prioritized. Such concerns highlight a pressing need for clearer communication from AI developers regarding the unique strengths and intended uses of each model, enhancing the public's ability to make informed decisions when engaging with AI technologies.
Learn to use AI like a Pro
Get the latest AI workflows to boost your productivity and business performance, delivered weekly by expert consultants. Enjoy step-by-step guides, weekly Q&A sessions, and full access to our AI workflow archive.
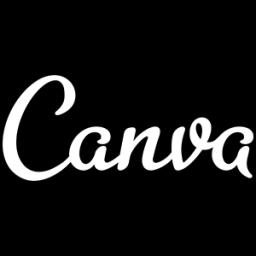
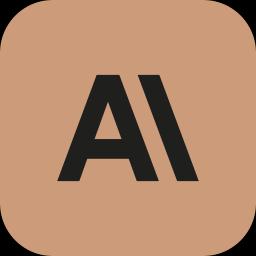
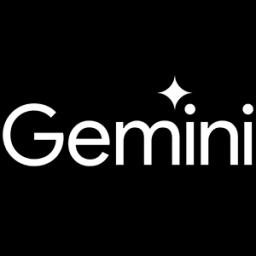
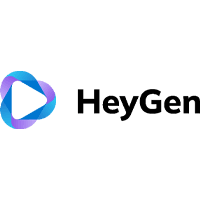
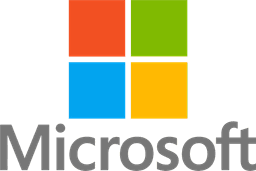
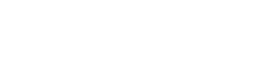
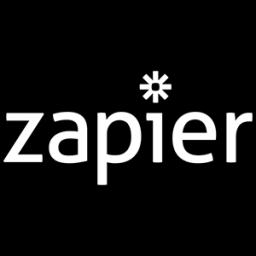
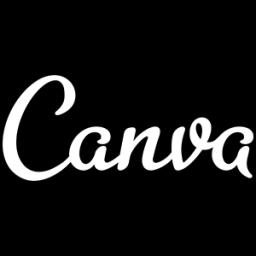
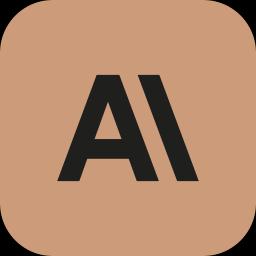
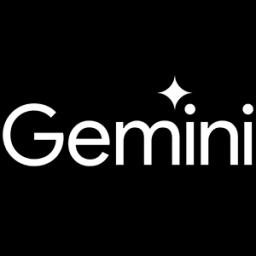
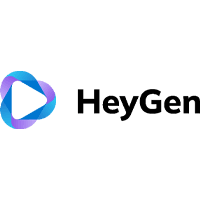
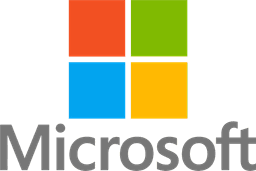
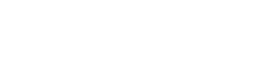
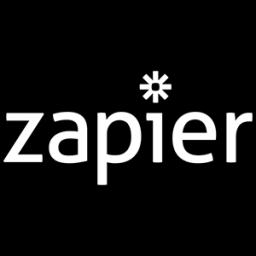
Moreover, the sheer number of available AI models reflects broader trends in technological development—where diversity is not just a feature, but a strategy for covering a wider array of user needs and industry applications. However, this approach may also inadvertently complicate the user experience. Reports of confusion among users, including professionals and academics, underline a potential barrier to effective AI adoption. For instance, the presence of nine distinct models available to ChatGPT Pro subscribers can complicate the decision-making process, leading to dissatisfaction and calls for streamlining options to enhance user accessibility.
Public reaction is also colored by the competitive nature of the AI industry, where rapid advancements and the introduction of new models are part of a broader innovation race. Within this context, the comparison of GPT-4.1, GPT-4o, and o3 serves not only as an exploration of technological capabilities but also as a commentary on the market dynamics and consumer expectations. While some users are drawn to the promise of cutting-edge technology, others feel a disconnect between the evolving capabilities of these models and their practical usability in everyday scenarios. This dichotomy underscores the complex relationship between AI development and public adoption, with implications for future AI governance and regulatory policies.
Future Implications of Advanced AI Model Capabilities
As we advance further into the realm of artificial intelligence, the capabilities of models like GPT-4.1, GPT-4o, and o3 are not only growing in sophistication but also expanding in their potential applications. These advancements hold significant promise for the future, particularly in how they might revolutionize industries and transform societal structures. The comparison of these models highlights their proficiency in logical reasoning, suggesting that they could automate complex tasks that were previously reliant on human intellect. This potential shift could lead to considerable economic growth, as increased automation and efficiency drive productivity gains across various sectors worldwide. For instance, companies might leverage these AI models to optimize workflows, decrease turnaround times, and innovate faster than ever before. As highlighted by experts, the economic potential of such innovations could be akin to a new productivity frontier, where AI augments human capabilities and accelerates developmental timelines for new technologies [2](https://www.mckinsey.com/capabilities/mckinsey-digital/our-insights/the-economic-potential-of-generative-ai-the-next-productivity-frontier).
On a social level, the enhanced capabilities of AI models could catalyze a profound transformation in the workforce. As AI takes over more logic-based tasks, human roles may evolve to involve more collaboration with these intelligent systems. This new dynamic could necessitate a reskilling of the workforce, as individuals adapt to roles that require them to work alongside AI, rather than compete against them. Moreover, ensuring that these advancements are accessible to all will be crucial in preventing a widening divide between those who can leverage AI for advancement and those who cannot. In this context, the imperative for policymakers will be to create inclusive frameworks that bridge the gap, promoting equitable access to AI technologies and preventing the exacerbation of existing socio-economic disparities [3](https://www.cbo.gov/publication/61147) [4](https://www.amacad.org/daedalus/ai-society).
Politically, the swift evolution of AI models like GPT-4.1 raises critical questions about governance and the geopolitical landscape. As these technologies become central to national economies and security, countries might find themselves in an intensifying race to harness AI’s full potential. This escalation could lead to geopolitical tensions, as nations vie for technological supremacy and navigate the complexities of AI governance. Establishing robust regulatory frameworks will be essential to guide the development and deployment of AI ethically and responsibly. These frameworks must ensure the safety, fairness, and transparency of AI systems to mitigate potential risks associated with rapid AI advancements. Furthermore, international cooperation will likely become more critical, as countries work together to create standards and protocols that address the challenges and opportunities presented by AI on a global scale [4](https://www.amacad.org/daedalus/ai-society).
Conclusion: Choosing the Right AI Model for Logic-Based Tasks
When considering the right AI model for tackling logic-based tasks, the choice can be quite nuanced as seen in the recent analyses of GPT-4.1, GPT-4o, and o3 models. These models have been evaluated under various logic puzzles, such as solving word plays and spatial reasoning challenges, with all three models proving capable. However, each model brings unique strengths to the table, potentially guiding different user preferences. GPT-4.1, for instance, was lauded for its clarity in explanation and logical consistency, qualities that might appeal to those who need reasoning transparency in tasks. On the other hand, the o3 model's concise and efficient problem-solving style could be preferred in environments where brevity and quick conclusions are valued. Despite the differences, it’s clear these models are robust options for logic-based tasks, as supported by extensive testing [link].
Learn to use AI like a Pro
Get the latest AI workflows to boost your productivity and business performance, delivered weekly by expert consultants. Enjoy step-by-step guides, weekly Q&A sessions, and full access to our AI workflow archive.
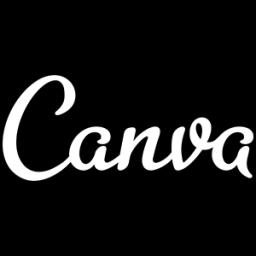
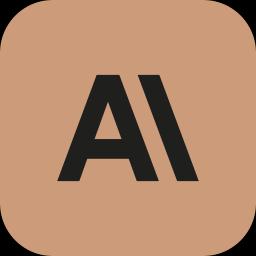
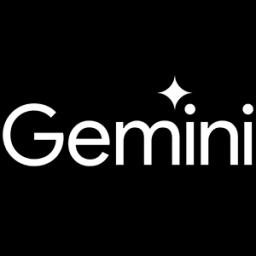
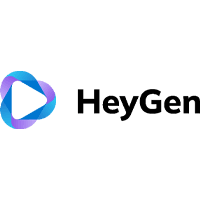
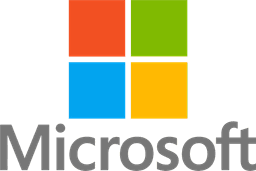
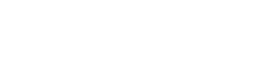
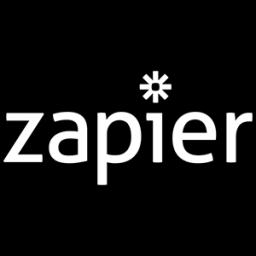
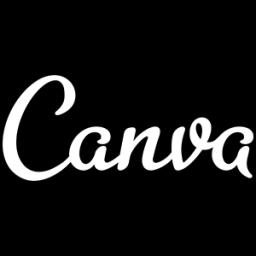
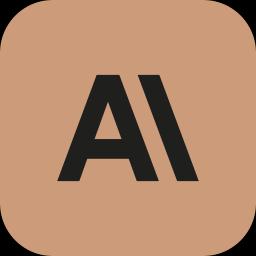
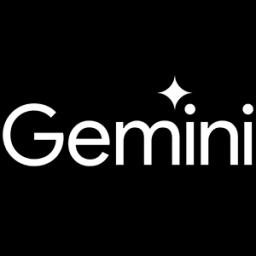
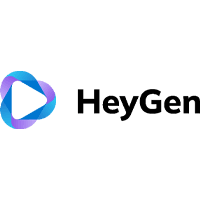
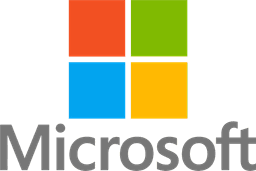
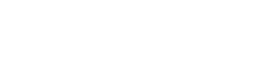
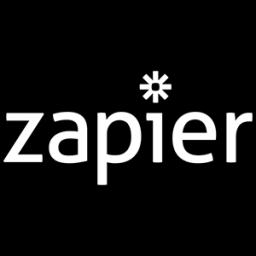
For organizations and individuals aiming to integrate AI into logic-heavy workflows, understanding the subtle distinctions between different AI models can greatly enhance effectiveness. GPT-4.1's strength in providing clear and logically sound explanations illustrates its suitability for educational environments or any scenario where understanding the "why" behind answers is crucial. Alternatively, o3’s design that leans towards rapid and brief responses might be more appropriate where swift decision-making is prioritized, such as in dynamic business environments. Overall, when selecting among powerful AI tools like GPT-4.1, GPT-4o, and o3, decisions should consider not just the logical problem-solving capacity, but also the specific communicative needs of the users involved [link].
Despite the proven capabilities of all three models in logic tasks, the plethora of AI options available may lead to decision fatigue among users. The current landscape for AI development is such that making an informed choice isn’t just about computational prowess; it also involves understanding the communication style and problem-solving nuances that best fit one's specific needs. Therefore, when engaging in the selection process, users should carefully consider factors such as the level of explanation required and the speed of response. This kind of tailored selection will not only optimize task performance but will also ensure that the AI implementation integrates seamlessly into the existing operational framework, enhancing overall productivity and user satisfaction [link].