Is the AI Boom Losing Momentum?
Is the AI Boom Slowing Down? Experts Warn of Data Scarcity Challenges
Last updated:

Edited By
Mackenzie Ferguson
AI Tools Researcher & Implementation Consultant
As the AI industry faces potential slowdowns due to data scarcity, experts like Google DeepMind's Demis Hassabis emphasize the diminishing returns in AI scaling laws. While companies such as Databricks and Nvidia continue heavy AI investments, alternative solutions like synthetic data generation are being explored but face hurdles in broader applications. This potential slowdown holds substantial implications for tech giants like Nvidia, which are deeply linked to the AI surge, and raises the need for new approaches to achieve human-level AI.
Introduction to AI Slowdown Concerns
The prospect of a slowdown in AI development due to data scarcity has emerged as a pressing concern within the tech industry. As AI models have expanded in capabilities, the demand for extensive and high-quality data has intensified. At the center of this issue are the 'scaling laws,' which describe how AI systems improve with more data. These laws are reaching their limits as the internet's reservoir of data begins to dry up. Industry leaders, such as Google's DeepMind CEO Demis Hassabis, have cautioned that diminishing returns are inevitable under current data constraints.
Despite these warnings, investment in AI technologies remains relentless. Giants like Nvidia and Databricks continue to pour resources into AI research and infrastructure, betting on the sector's future growth. The contradiction between the exponential increase in investments and the looming threat of an AI slowdown highlights the industry's complex relationship with data availability.
Learn to use AI like a Pro
Get the latest AI workflows to boost your productivity and business performance, delivered weekly by expert consultants. Enjoy step-by-step guides, weekly Q&A sessions, and full access to our AI workflow archive.
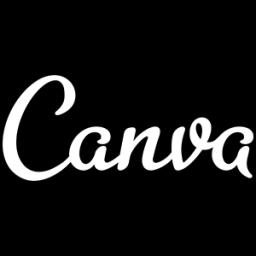
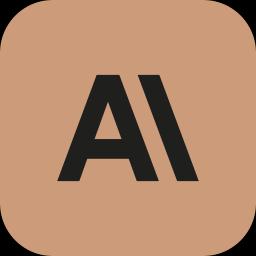
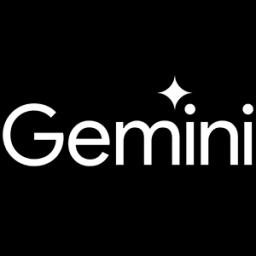
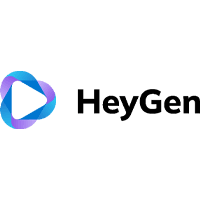
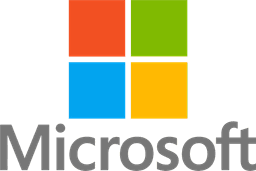
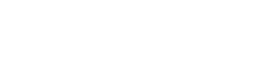
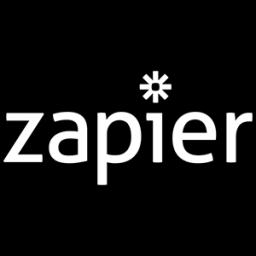
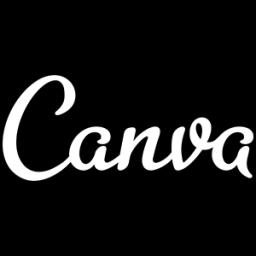
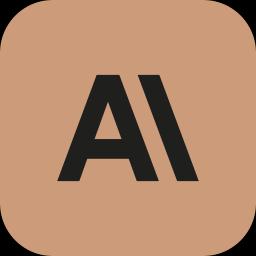
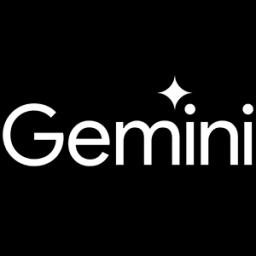
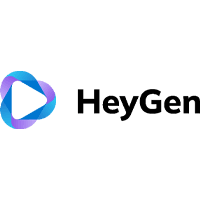
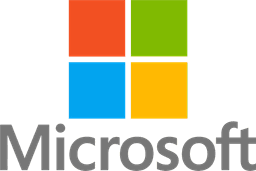
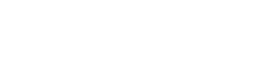
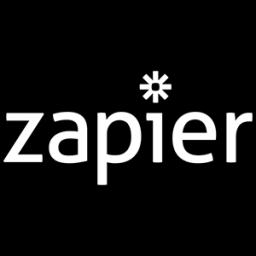
To address these challenges, some companies are turning to synthetic data solutions. This approach involves generating artificial datasets that can supplement or even replace traditional data-gathering methods. However, while synthetic data offers promising possibilities, its effectiveness is limited in complex fields where understanding nuanced human contexts is essential. Despite its limitations, synthetic data remains a key area of exploration, especially in sectors like autonomous vehicles, where companies like Nvidia are making strides in simulation technology.
Understanding AI Scaling Laws
AI scaling laws, which describe how AI models improve in performance with increasing quantities of data and computational resources, are facing new challenges as the availability of high-quality data diminishes. This concept is analogous to the process of education, where students improve their knowledge by reading more books. However, as the article highlights, experts like Google DeepMind's Demis Hassabis warn that we are reaching a point where the traditional scaling of AI models is yielding diminishing returns due to data scarcity.
The shortage of high-quality digital text needed for training large language models (LLMs) is causing concern among AI researchers and companies. Significant players like OpenAI and Google are encountering bottlenecks, threatening to decelerate the pace of AI advancements. This situation is compared to a looming bottleneck that could occur by 2028 if alternative solutions are not developed.
Synthetic data generation is one alternative being explored to counteract the scarcity of real-world data. By artificially creating data through techniques like trial and error, AI systems can continue to learn and improve. Despite this promise, synthetic data is not without limitations. It is particularly effective for generating data in fields with definitive solutions, such as mathematics, but struggles in more nuanced areas like the humanities where qualitative judgments are essential.
Learn to use AI like a Pro
Get the latest AI workflows to boost your productivity and business performance, delivered weekly by expert consultants. Enjoy step-by-step guides, weekly Q&A sessions, and full access to our AI workflow archive.
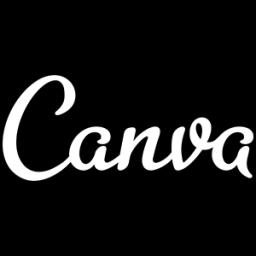
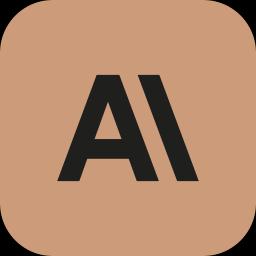
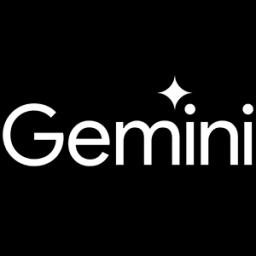
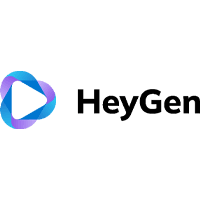
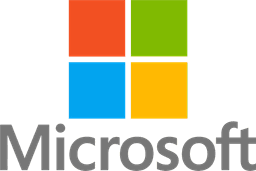
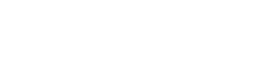
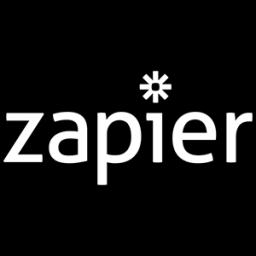
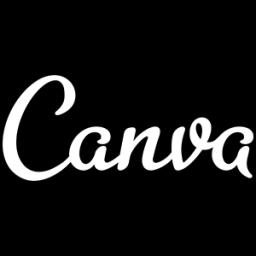
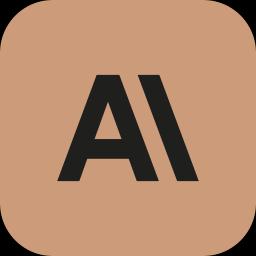
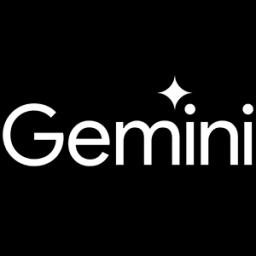
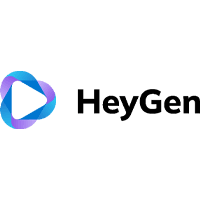
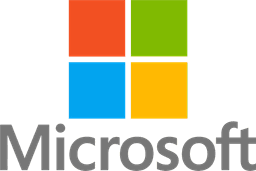
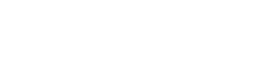
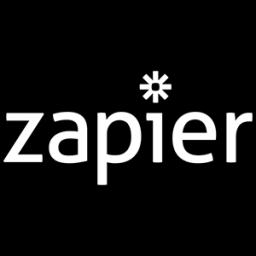
The implications of a slowdown in AI progress extend beyond technical spheres and impact economic valuations, particularly for companies heavily invested in the AI boom, like Nvidia. The potential deceleration could slow the development and release of new AI-powered applications and services, affecting productivity and economic growth.
Amidst these challenges, there are calls for innovation in AI research, focusing on more efficient data usage. Techniques such as few-shot learning and transfer learning offer promising results that require less data. Experts like Yann LeCun advocate shifting the focus away from simply accumulating more data towards developing systems capable of learning more efficiently with less.
Public reactions to these developments range from concern about the potential impact on innovation to skepticism about the genuineness of data scarcity as an issue. Privacy advocates view the slowdown as an opportunity to address ethical considerations regarding data collection, while others advocate for more research into synthetic data and alternative methods to overcome these challenges.
Data Scarcity Challenges in AI
Public reactions to the narrative of AI slowdown due to data scarcity are varied. Among tech enthusiasts and professionals, there is a palpable concern about the potential impact on innovation and the broader tech ecosystem. However, some express skepticism regarding the severity of these challenges, suspecting that narratives around data scarcity may be amplified by tech companies. On the flip side, privacy advocates may view this potential slowdown as a positive change, allowing for more measured and ethically aligned AI advancements. Calls for innovative solutions, such as synthetic data generation, resonate strongly, with discussions highlighting the need for new, creative methodologies to sustain AI progress in a data-constrained world.
As stakeholders navigate the complexities of data scarcity, several long-term strategies begin to emerge. The industry's focus appears to be shifting toward more efficient AI models capable of functioning with limited data. This transition could foster breakthroughs in synthetic data generation and novel AI paradigms that prioritize adaptability and robustness over sheer volume. Ultimately, the resolution of data scarcity challenges holds potential for reshaping both the means and goals of AI development, paving the way for advances that marry computational efficiency with human-like cognitive capabilities.
The Role of Synthetic Data in AI
The rapid development of artificial intelligence (AI) is seemingly on the edge of a slowdown due to an emerging issue of data scarcity. This scarcity of accessible internet data has triggered concerns among AI experts, including notable figures like Demis Hassabis of Google DeepMind. The apprehension revolves around the diminishing returns in 'scaling laws'—a principle that suggests AI models improve proportionally with more data. With the abundance of digital text dwindling, these models face limitations in performance enhancements, a sentiment echoed by the industry.
Learn to use AI like a Pro
Get the latest AI workflows to boost your productivity and business performance, delivered weekly by expert consultants. Enjoy step-by-step guides, weekly Q&A sessions, and full access to our AI workflow archive.
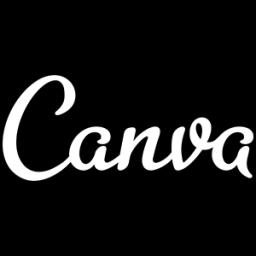
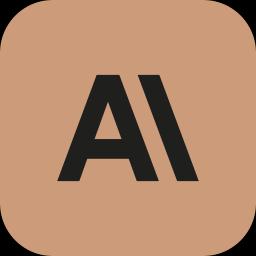
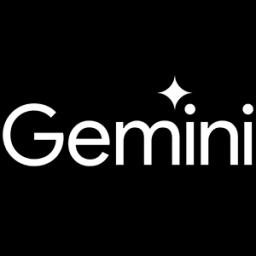
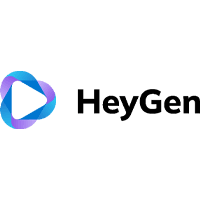
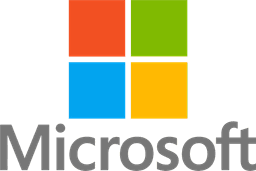
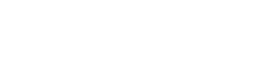
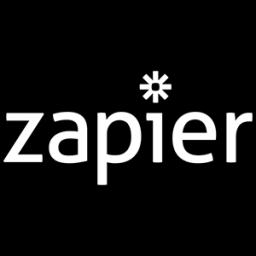
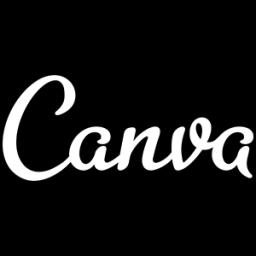
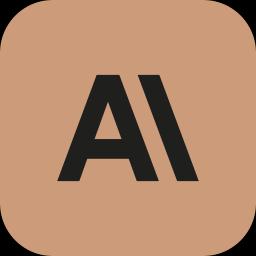
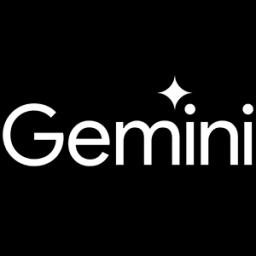
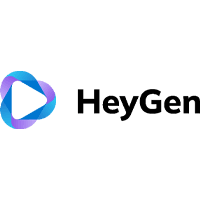
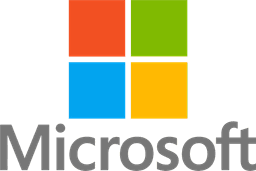
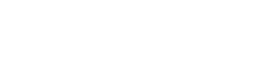
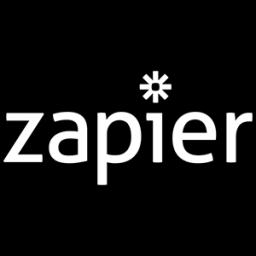
Despite these concerns, the AI industry continues to see significant investments, highlighted by companies such as Databricks and Nvidia. These organizations persist with substantial financial commitments in AI, driven by the expectation of sustaining progress. Meanwhile, there's a growing interest in synthetic data as an innovative alternative solution. Synthetic data is artificially generated rather than acquired from real-world instances, and it represents a potential solution to address the challenges posed by data scarcity. Yet, the utility of synthetic data remains confined, mainly effective in areas with definite right or wrong outcomes, like mathematics and programming, rather than more subjective fields such as the humanities.
The potential implications of an AI slowdown are far-reaching for tech companies, particularly those like Nvidia whose valuation is closely tied to the AI market's rapid growth. While optimism about overcoming these challenges exists, the industry is urged to develop new methodologies to reach human-level AI advancements. The article underscores that while synthetic data solutions are promising, the call for innovative techniques remains pressing to circumvent the growing potential for a slowdown.
The article reflects on potential future scenarios hinting at economic, social, and political ramifications. Economically, a slowdown might stifle AI-driven innovations, potentially affecting productivity and economic growth. Socially, sectors such as healthcare, education, and public service might experience delayed AI adoption due to these challenges. Politically, the issue could prompt a reevaluation of data governance policies and amplify geopolitical shifts among nations with larger data resources.
Experts offer varied opinions on the matter. Dr. Dario Amodei points out the necessity for high-quality data for AI progression, attributing current progress rates to the availability of such data. Conversely, Dr. Fei-Fei Li and Yann LeCun suggest the current data scarcity as an opportunity to invent more efficient AI models that can learn from less data, akin to human cognitive processes. Their perspectives suggest a forward-thinking approach toward overcoming the looming data shortage crisis.
Impacts of AI Slowdown on Tech Industry
The tech industry stands at a pivotal moment as experts warn of a potential slowdown in artificial intelligence (AI) development due to an impending data scarcity. Prominent figures such as Demis Hassabis from Google DeepMind have raised concerns about the effectiveness of scaling laws, which have traditionally propelled AI advancements by leveraging vast amounts of internet data. With this data becoming increasingly scarce, the trajectory of AI innovation could be significantly impacted.
Despite these warnings, notable companies like Databricks and Nvidia demonstrate resilience by continuing to channel substantial investments into AI. This indicates a bullish outlook towards overcoming current barriers and sustaining momentum in AI innovation. However, the question remains: will their efforts suffice in the face of diminishing returns attributed to data shortages?
Learn to use AI like a Pro
Get the latest AI workflows to boost your productivity and business performance, delivered weekly by expert consultants. Enjoy step-by-step guides, weekly Q&A sessions, and full access to our AI workflow archive.
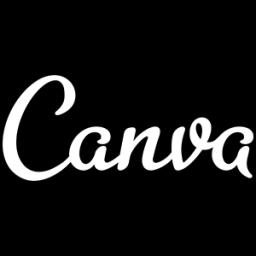
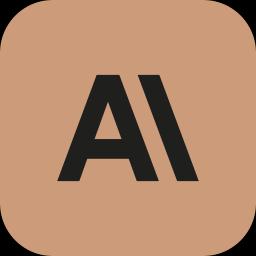
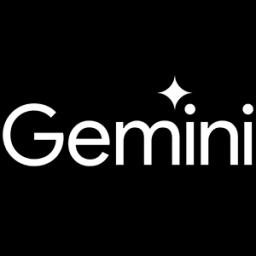
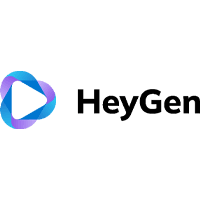
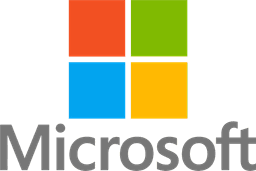
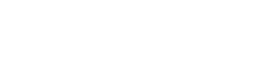
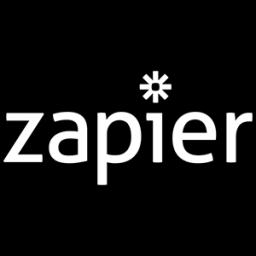
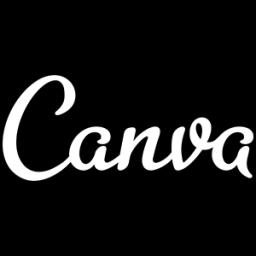
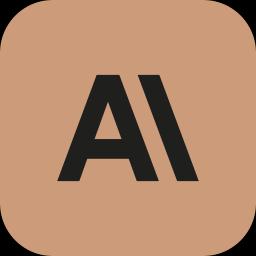
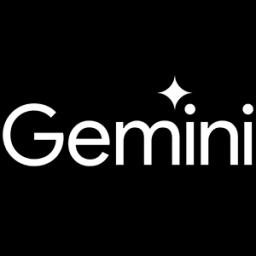
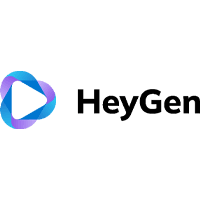
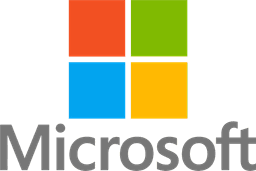
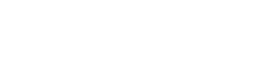
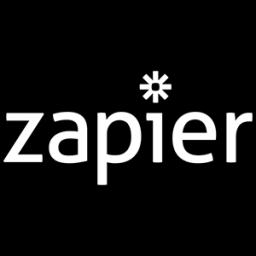
Exploring alternatives, the tech industry is venturing into synthetic data generation as a potential solution. While this approach offers promise, it is not without its challenges, particularly in domains demanding nuanced understanding like the humanities. Yet, in fields such as programming and mathematics, synthetic data has shown to be beneficial, reflecting the optimism some hold in these technological explorations.
The ramifications of an AI slowdown could be profound, especially for companies like Nvidia whose valuations are heavily linked to the AI boom. An industry-wide deceleration could influence everything from stock prices to innovation pipelines, fundamentally altering the competitive landscape.
While optimism persists among some experts—citing emergent techniques like few-shot and transfer learning—the pressing need for breakthroughs in data utilization underscores a transitional phase for AI. The ultimate ambition remains achieving human-level AI, which increasingly demands novel approaches and methodologies that transcend traditional data limitations.
Optimism and Alternatives for AI Future
The rapid pace of artificial intelligence development has brought about exciting possibilities and transformative technologies across various sectors. While the current trajectory suggests tremendous potential, concerns over an impending slowdown due to data scarcity are rising. The tech industry, dependent on vast amounts of data to train large language models (LLMs), faces diminishing returns as easy-to-access data is exhausted. However, this challenge presents an opportunity to explore alternative solutions and ignite optimism for a resilient AI future.
Prominent figures in the AI community, like Google DeepMind's Demis Hassabis, have voiced apprehensions about the limitations posed by scaling laws. These laws correlate AI model improvement with increased data availability, and their effectiveness dwindles when faced with a scarcity of high-quality digital text. Despite these concerns, major companies such as Databricks and Nvidia are not deterred; instead, they are doubling down on AI investments. Such organizations are crucial players in propelling the field to overcome current hurdles.
One promising avenue to address data scarcity is the use of synthetic data generation. This innovative approach involves creating artificial data that AI models can use to learn, similar to how children learn through playing and problem-solving. Though not without its own limitations—particularly in domains where nuanced human experiences are essential—synthetic data holds significant promise in fields with clear parameters, such as mathematics or software engineering. Notably, NVIDIA is already leveraging synthetic data for developing autonomous vehicle systems on its DRIVE Sim platform.
Learn to use AI like a Pro
Get the latest AI workflows to boost your productivity and business performance, delivered weekly by expert consultants. Enjoy step-by-step guides, weekly Q&A sessions, and full access to our AI workflow archive.
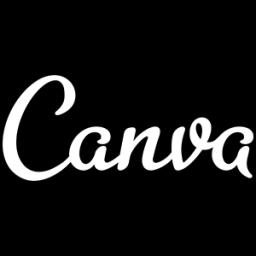
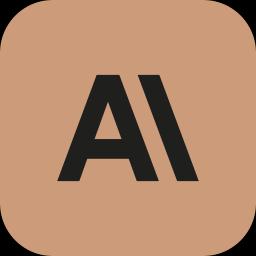
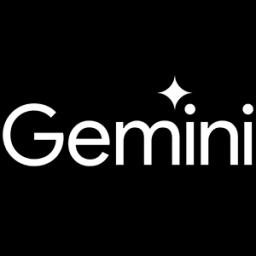
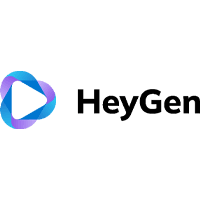
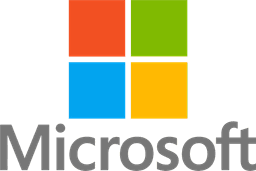
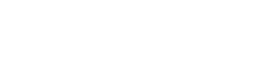
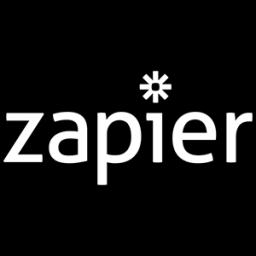
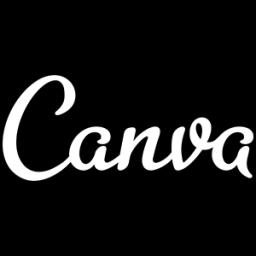
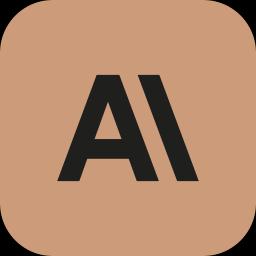
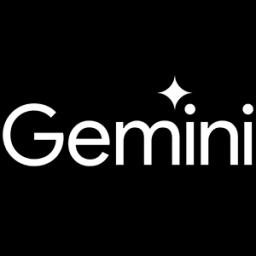
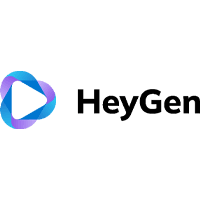
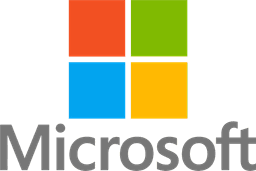
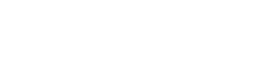
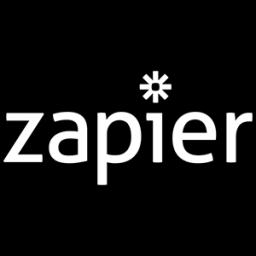
Optimism for the AI future is not just a matter of technological advancement but also involves a strategic shift in how AI learns. Dr. Fei-Fei Li of Stanford’s Human-Centered AI Institute suggests a positive strategy, advocating for the development of models that require less data through techniques like few-shot and transfer learning. Yann LeCun at Meta echoes this sentiment, envisioning AI systems that mirror human learning efficiencies rather than relying solely on data accumulation. This transition could revolutionize AI, making it more adaptable and efficient.
The broader societal context also plays a key role in the discourse around AI's future. While some stakeholders express concerns about slowing innovation and its economic impacts, others see this as a chance to emphasize ethical AI development. Privacy advocates may welcome the breakneck pace of progress slowing, providing room for discourse on data governance and ethical practices. AI might not only change in response to technological shifts but also due to evolving public priorities and regulatory landscapes.
Looking forward, optimism may be grounded in the belief that scarcity will drive innovation. This perspective sees constraints not as dead-ends but as catalysts for creativity, leading to groundbreaking advancements in AI models and their applications. The future could introduce sophisticated synthetic data solutions and invigorate international collaborations aiming for sustainable AI growth. With continued investment and research into efficient algorithms, AI's evolution may very well exemplify resilience and adaptability in the face of scarcity.
Public Reactions to AI Development Issues
The potential slowdown in AI development due to data scarcity has provoked various reactions from the public. Among tech enthusiasts and AI professionals, there is an evident concern about the adverse effects this could have on innovation and progress in artificial intelligence. These individuals worry that a lack of sufficient high-quality data could hinder developments that drive advancements in various technological fields and applications.
Conversely, there is a segment of the public that views the emphasis on data scarcity with skepticism. Some believe that the issue is exaggerated by tech companies to justify their extensive data collection practices. This perspective raises questions about the true impact of data scarcity and whether it might be a narrative propelled by industry giants for their benefit.
On a different note, privacy advocates and those apprehensive about the pace of AI advancements express relief at the potential slowdown. They see this as an opportunity to reevaluate the rapid, unchecked progress of AI technologies which could pose unchecked consequences if not properly regulated. This sentiment highlights a desire for a balanced approach to AI development that takes privacy and safety into consideration.
Learn to use AI like a Pro
Get the latest AI workflows to boost your productivity and business performance, delivered weekly by expert consultants. Enjoy step-by-step guides, weekly Q&A sessions, and full access to our AI workflow archive.
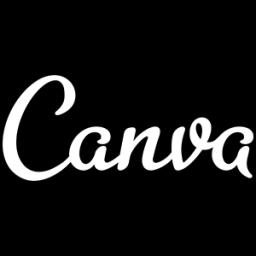
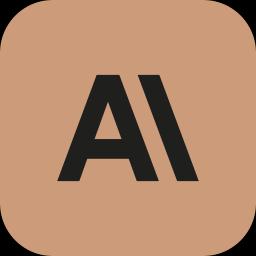
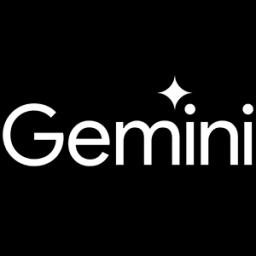
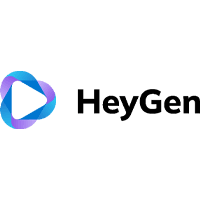
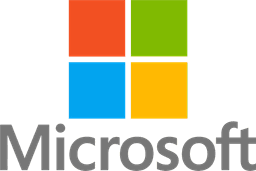
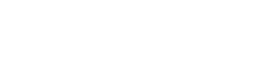
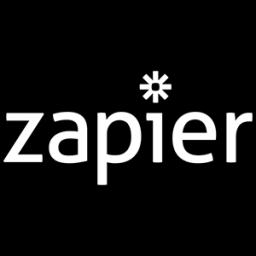
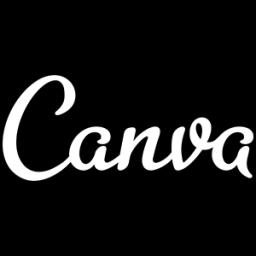
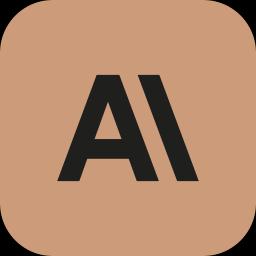
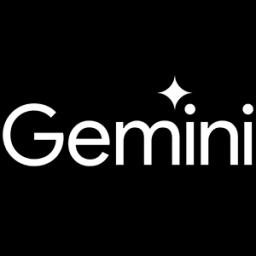
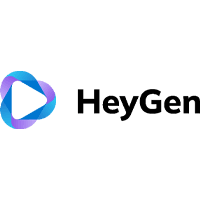
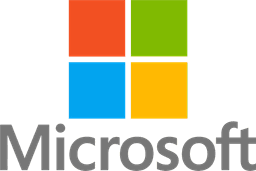
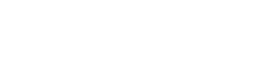
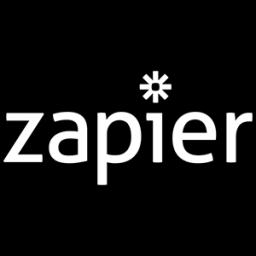
Moreover, there are calls for innovation and exploration of alternatives to overcome data challenges. Many advocate for more research into synthetic data generation and alternative training methodologies as solutions to data scarcity. This illustrates a growing demand for novel approaches that can sustain AI advancements without being heavily reliant on natural data sources.
The public reaction also includes ethical considerations surrounding AI development and data use. Discussions on social media and online platforms often emphasize the importance of balancing technological progress with ethical data practices and privacy regulations. There's a mutual understanding that data scarcity issues must be addressed with a conscientious approach to avoid ethical pitfalls in AI development.
In summary, the public's reactions to potential AI development slowdowns due to data scarcity are multifaceted. While concerns about stifling innovation are prevalent, there is also support for the pause as a chance to reflect on the ethical dimensions of AI growth and to push for diverse and innovative solutions. Mixed feelings about the role of synthetic data further underscore the complexities involved in navigating future AI advancements.
Future Implications of AI Slowdown
Artificial Intelligence (AI) has become a cornerstone of technological innovation, driving immense shifts across industries and transforming the way humans interact with machines. Yet, as the field has rapidly evolved, concerns over a potential slowdown due to data scarcity are gaining traction among experts and stakeholders. This slowdown highlights a critical intersection between technological advancement and resource limitations, offering both challenges and opportunities for future developments. It is essential to explore the implications this potential shift holds for the broader tech industry and its auxiliary domains.
One significant implication of an AI slowdown is its impact on the technology sector's economic landscape. AI has been a major driver of innovation and productivity, contributing to substantial gains in various market segments. Companies heavily invested in AI, such as Nvidia, which has capitalized significantly on the AI boom, may be compelled to reassess their value propositions. As the availability of high-quality data diminishes, these firms might face increased pressure to justify their market valuations and explore alternative avenues to sustain growth.
Moreover, the anticipated slowdown may prompt a reevaluation of AI investment strategies. Despite ongoing challenges, companies like Databricks and Nvidia continue to funnel substantial resources into AI research and infrastructure. This dedication could mark a pivot towards developing more efficient data utilization methods, thereby fostering innovations that counteract the diminishing returns from traditional scaling laws. This trend may also catalyze a surge in synthetic data generation, positioning it as a vital component of future AI models.
Learn to use AI like a Pro
Get the latest AI workflows to boost your productivity and business performance, delivered weekly by expert consultants. Enjoy step-by-step guides, weekly Q&A sessions, and full access to our AI workflow archive.
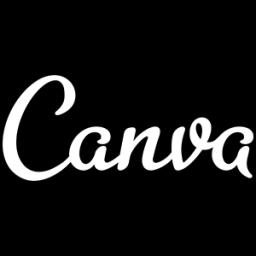
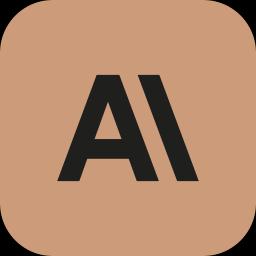
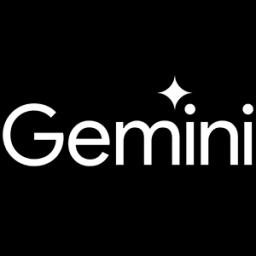
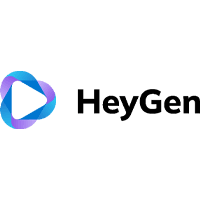
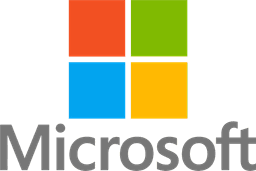
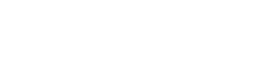
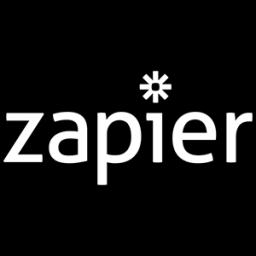
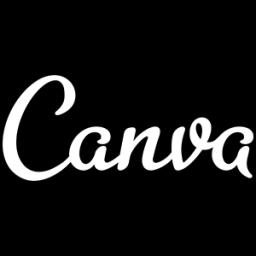
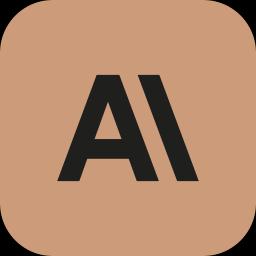
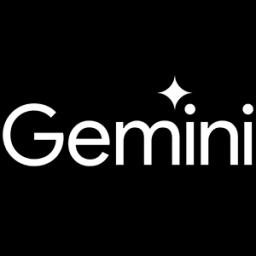
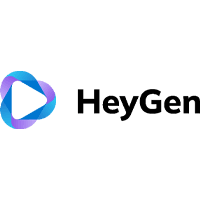
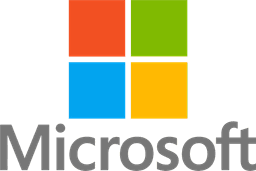
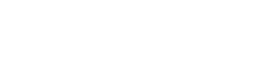
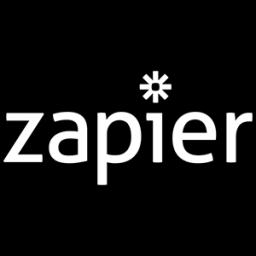
The social consequences of a slowdown in AI development extend beyond economic metrics. As AI applications permeate sectors such as healthcare, education, and public services, any stagnation in AI progress could slow advancements that promise to enhance public welfare. Additionally, the slowing pace might alleviate some societal concerns around AI, such as job displacement and ethical issues like algorithmic bias, leading to a more nuanced discourse on the balance between technological innovation and societal impact.
Politically, a slowdown prompts a reconsideration of data governance and regulatory frameworks. Countries with robust data reserves may emerge as leaders in AI, shifting geopolitical dynamics and prompting nations to strategize their data policies carefully. This environment could foster international collaborations aimed at overcoming data scarcity and promoting equitable advancements in AI technology, highlighting the need for unified global efforts to address these challenges.
In the long term, the challenges associated with data scarcity in AI could drive a paradigm shift within the industry. This shift may prioritize the development of algorithms that emphasize efficiency in data usage, reducing dependence on vast quantities of input data. Innovations in synthetic data generation could play a transformative role, enabling breakthroughs that redefine AI capabilities. As the industry adapts to these constraints, there is potential for developing more robust and sustainable AI systems that align with ethical and practical considerations, paving the way for the next wave of AI advancement.