AI Models Tackle Real Freelance Projects
OpenAI's SWE-Lancer: Testing AI in the Real World of Software Engineering
Last updated:

Edited By
Mackenzie Ferguson
AI Tools Researcher & Implementation Consultant
OpenAI has unveiled SWE-Lancer, an innovative benchmark assessing AI models against real-world software engineering tasks sourced from Upwork. With over 1,400 tasks collectively valued at $1 million, SWE-Lancer evaluates AI capability in practical software development scenarios.
Introduction to SWE-Lancer
SWE-Lancer represents a significant leap forward in the evaluation of AI's capabilities within the realm of software engineering. Developed by OpenAI, it serves as an unprecedented benchmark, assessing AI models using real-world tasks sourced from Upwork. The benchmark encompasses over 1,400 tasks, ranging from simple bug fixes to complex feature implementations, with a total potential earning value of $1 million. This rigorous assessment framework not only gauges the practical software development skills of AI models but also challenges them with projects that demand a comprehensive understanding of the software development lifecycle. By utilizing actual freelance tasks, SWE-Lancer provides a unique perspective on how AI can perform in a live engineering environment, complete with the challenges of management decisions and client interactions. The initiative highlights OpenAI's commitment to pushing the boundaries of what AI can achieve in professional settings, positioning SWE-Lancer as a pivotal tool in ongoing AI research and development. [For more details, click here](https://www.maginative.com/article/openais-new-benchmark-tests-ai-models-against-real-world-software-engineering-tasks/).
Claude 3.5 Sonnet: The Top Performer
Claude 3.5 Sonnet has distinguished itself as the frontrunner in OpenAI's innovative SWE-Lancer benchmark, a testament to its advanced capabilities in efficiently handling real-world software engineering tasks. This state-of-the-art model achieved remarkable success by securing approximately $400,000 in potential earnings, which accounts for a significant 40% of the total stakes presented in the test. Such accomplishments highlight the model's adeptness in agile and precise solutions demanded by freelance engineering projects, setting it apart from other AI contenders in the benchmark. By utilizing over 1,400 diverse tasks, ranging from fundamental bug fixes to comprehensive feature developments, SWE-Lancer convincingly demonstrates Claude 3.5 Sonnet's proficiency and potential in software engineering applications. More details on the benchmark can be found in the full article.
Learn to use AI like a Pro
Get the latest AI workflows to boost your productivity and business performance, delivered weekly by expert consultants. Enjoy step-by-step guides, weekly Q&A sessions, and full access to our AI workflow archive.
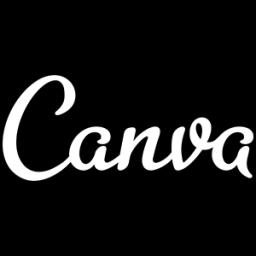
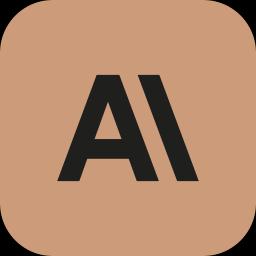
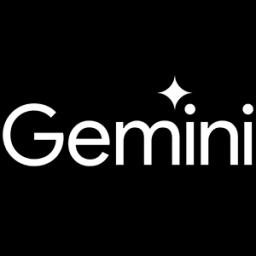
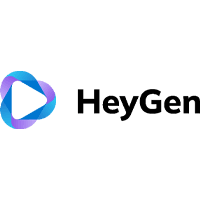
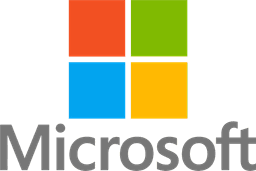
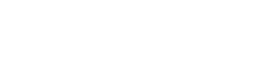
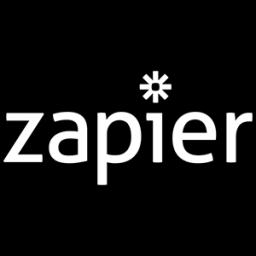
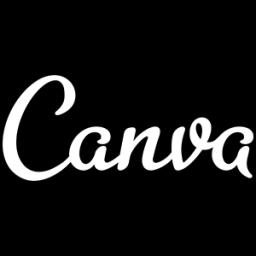
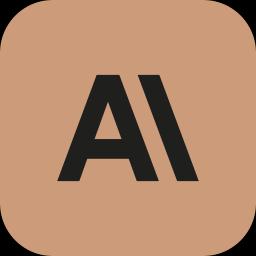
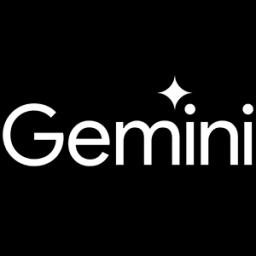
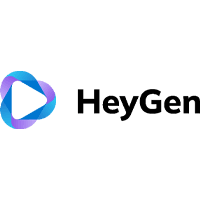
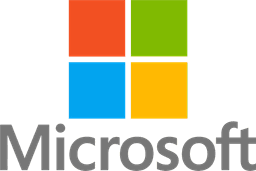
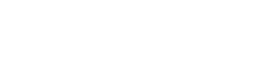
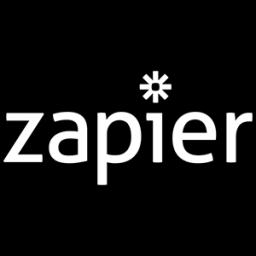
Despite Claude 3.5 Sonnet's impressive performance, which significantly surpassed many expectations, the findings disclosed under the SWE-Lancer initiative relayed a critical insight: AI, in its current sophistication, remains supplementary rather than substitutive in software development realms. The benchmark's comprehensive evaluations, designed to mimic real-world freelance environments on platforms like Upwork, rigorously tested AI's ability to autonomously address and manage intricate software lifecycle processes, including managerial decision-making tasks. However, the ultimate results underscored by Claude 3.5 Sonnet's performance, reiterate that these models, although advancing rapidly, still fall short of fully replicating human engineers' holistic problem-solving and creative thinking capabilities. Additional insights can be gathered from the benchmark analysis.
The development and success of Claude 3.5 Sonnet within the SWE-Lancer benchmark not only exemplify the AI's current strengths but also spotlight areas necessitating further advancements. The model's high earnings potential, quantified within the benchmark's framework, signifies a burgeoning opportunity for AI-enhanced productivity in software tasks, albeit with the caveat of essential human oversight. This benchmark iteration underscores the need for continuing adaptive learning and improved cognitive capabilities within AI systems to eventually parallel the nuanced understanding and decision-making proficiencies of professional engineers. The release of SWE-Lancer Diamond, offering a snapshot public dataset, aims to spur further academic and practical investigations into refining AI methodologies. More about the dataset and its implications can be explored here.
Real-World Applications and Limitations
The launch of OpenAI's SWE-Lancer benchmark marks a significant milestone in evaluating AI models on real-world software engineering tasks. This benchmark stands out due to its use of actual freelance projects from Upwork, which mirrors the entire software development lifecycle, including subtleties of management decisions. For instance, the benchmark's ability to capture over 1,400 tasks, involving complex intricacies from bug fixes to comprehensive feature implementations, provides a unique platform to assess AI's true capabilities in practical environments. This initiative not only tests the technical prowess of AI models but also scrutinizes their decision-making processes against human managers, offering a holistic view of AI's potential impact on the workforce.
Despite Claude 3.5 Sonnet achieving top performer status by capturing approximately 40% of potential earnings, the benchmark shows AI still lacks the ability to fully replace human software engineers. While these models excel in automation and repetitive task handling, they face considerable challenges in iterative development processes and complex problem-solving. This limitation emphasizes the current role of AI as an augmentative tool rather than a standalone solution. The SWE-Lancer results underscore a critical need for AI to develop more nuanced reasoning capabilities, akin to the unique insights and creative solutions humans provide in intricate engineering contexts.
Learn to use AI like a Pro
Get the latest AI workflows to boost your productivity and business performance, delivered weekly by expert consultants. Enjoy step-by-step guides, weekly Q&A sessions, and full access to our AI workflow archive.
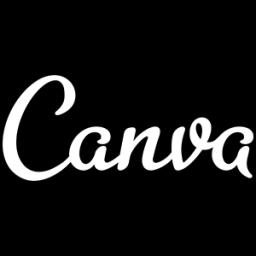
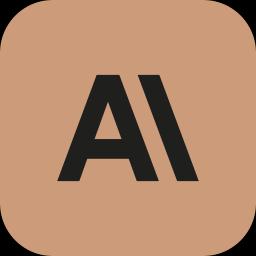
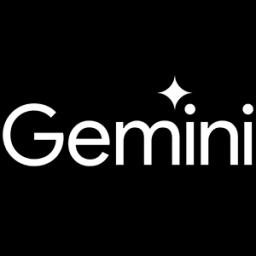
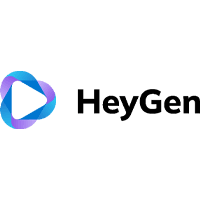
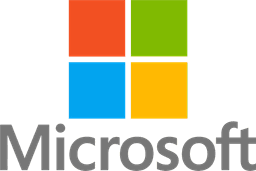
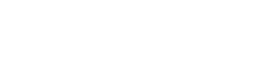
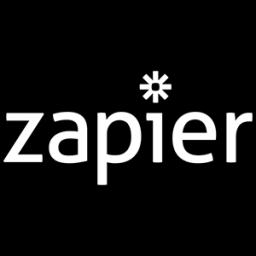
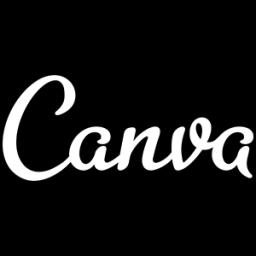
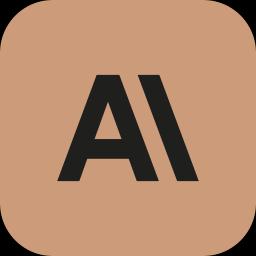
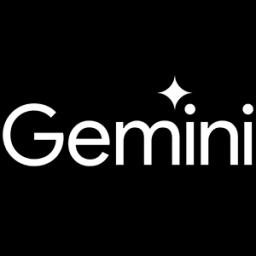
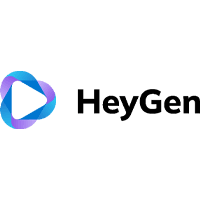
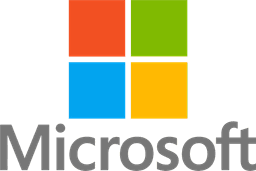
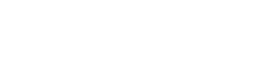
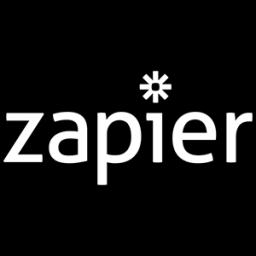
OpenAI's release of the SWE-Lancer Diamond, a subset of this benchmark’s dataset, is poised to accelerate research in AI-driven software engineering. This publicly released data, containing tasks valued at $500,800, aims to galvanize further exploration into how AI can be better integrated into the software development ecosystem. However, while the benchmark demonstrates promising synergies between AI and human work, it simultaneously highlights ongoing issues like potential data contamination during model training and the inherent biases within AI-generated solutions. Addressing these challenges is crucial for more robust and ethically sound AI applications in the future.
The implications of these findings are vast, suggesting a future where AI and human engineers work in tandem to enhance productivity and innovation in the software industry. This gradual integration is expected to foster economic growth through AI-augmented software development but comes with the responsibility of reshaping educational and professional training to equip the workforce with skills that complement AI technologies. The emergence of such AI tools and benchmarks signifies a transformative phase for the industry, requiring thoughtful policies on AI regulation, workforce adaptation, and ethical AI deployment.
SWE-Lancer Diamond: Advancing AI Research
The launch of SWE-Lancer Diamond marks a significant milestone in the field of AI research, especially in practical software engineering scenarios. As described in a recent report by OpenAI, this benchmark is based on real-world freelance software engineering tasks, promising to evaluate AI capabilities in authentic work environments. SWE-Lancer Diamond is designed to foster further investigation into how AI can support software development, offering insights by releasing a public dataset containing tasks valued at $500,800. This scenario provides researchers with extensive data to explore AI contributions to software engineering, potentially leading to the development of more sophisticated AI models capable of complex problem-solving.
The emergence of SWE-Lancer Diamond underscores AI's evolving role in augmenting human capabilities in software development. With over 1,400 tasks ranging from simple bug fixes to complete feature implementations, detailed in the OpenAI announcement, researchers can deeply analyze AI's potential in streamlining and enhancing coding efficiency. Interestingly, the dataset also allows AI models to be tested against managerial decisions akin to those made by Upwork hiring managers, offering a comprehensive assessment of AI's soft skills in decision-making.
One of the key findings from the SWE-Lancer benchmarks is the impressive performance of the Claude 3.5 Sonnet model. As noted in the OpenAI's findings, Claude 3.5 Sonnet achieved roughly $400,000 in earnings from the potential $1 million pool, highlighting its capability to handle various software engineering tasks effectively. However, the benchmark also reveals that AI models are not ready to replace human engineers entirely, as they still require advancements to match the nuanced understanding humans bring to engineering challenges. This finding reinforces the need for continuous research to enhance AI's problem-solving capabilities in software development contexts.
The development and release of SWE-Lancer Diamond aim to encourage increased collaboration between AI tools and human experts in software engineering. As OpenAI reports, the tasks covered reflect the full software development lifecycle, offering unique opportunities to analyze AI's performance in managing end-to-end processes in a realistic business environment. By bridging this gap, SWE-Lancer fosters a deeper understanding of how AI can contribute positively to productivity and innovation in the tech industry. This new benchmark is a strategic move by OpenAI to accelerate AI research, empower developers with more robust tools, and pave the way for future advancements in AI-aided software engineering tasks.
Learn to use AI like a Pro
Get the latest AI workflows to boost your productivity and business performance, delivered weekly by expert consultants. Enjoy step-by-step guides, weekly Q&A sessions, and full access to our AI workflow archive.
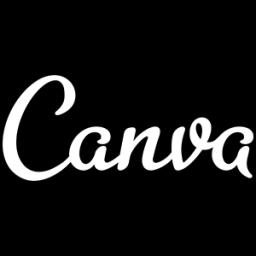
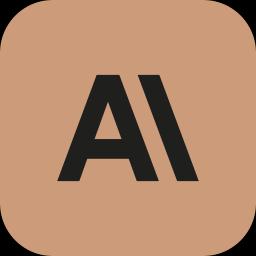
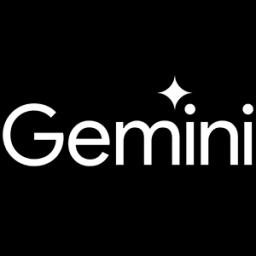
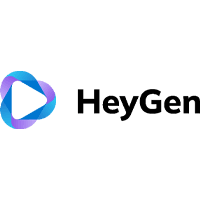
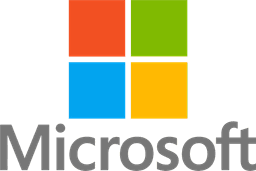
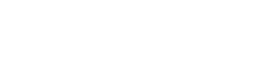
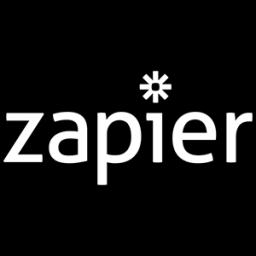
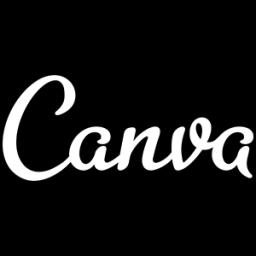
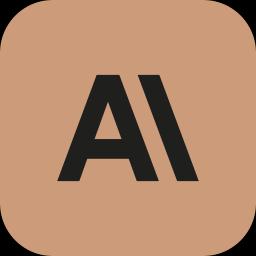
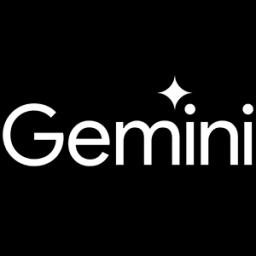
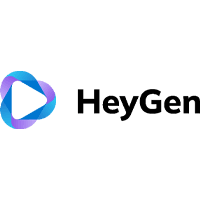
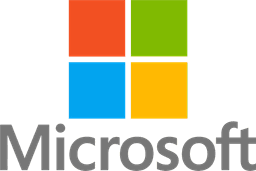
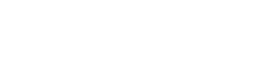
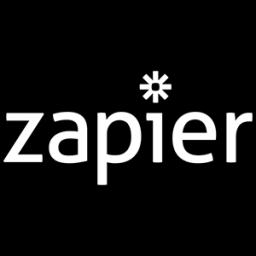
The Role of AI in Software Engineering
Artificial Intelligence (AI) is increasingly becoming a pivotal force in software engineering, demonstrating capabilities that were once considered exclusive to human expertise. One significant development is OpenAI's release of the SWE-Lancer benchmark, which evaluates AI models on real-world software engineering tasks sourced from Upwork, offering insights into how AI can tackle diverse job requirements within the industry. This benchmark, capturing over 1,400 tasks with a potential earning of $1 million, showcases AI's potential in handling practical software development projects, ranging from bug fixes to entire feature implementations (source).
Despite these advancements, AI is not yet ready to replace human engineers. The top-performing AI model in the SWE-Lancer tests, Claude 3.5 Sonnet, was responsible for approximately 40% of the potential earnings, illustrating that while AI can contribute significantly, it still lags behind human capabilities (source). This gap is particularly evident in complex project management and decision-making scenarios where human intuition and experience are paramount.
Furthermore, leading AI experts assert that while systems like Claude 3.5 may show proficiency in specific areas like code localization and performing quick fixes, they struggle with diagnosing and solving more intricate software problems. As a result, the current state of AI in software engineering is more suited to augmenting human efforts rather than replacing them, reinforcing humans' role in critical thinking and nuanced problem-solving tasks (source).
The introduction of AI technologies such as Microsoft’s Developer Copilot, Google's AlphaCode 2, Amazon's CodeWhisperer, and GitHub’s AI Security Copilot, further illustrates the growing integration of AI in development environments. These tools are designed to enhance productivity, provide robust security features, and optimize the coding process across various programming languages and platforms, effectively complementing human developers and enabling them to focus on more strategic aspects of software design (source), (source), (source), (source).
Public and professional reactions to benchmarks like SWE-Lancer highlight both the optimism around AI's capabilities and skepticism regarding its limitations. Community feedback emphasizes the need for further refinement in AI's ability to comprehend and integrate contextual nuances in large-scale software applications. Concerns about data contamination and bias in model training accentuate the ongoing challenges that need addressing for AI to achieve greater trust and reliability in engineering circles (source).
In summary, while AI is undoubtedly transforming software engineering by automating routine and repetitive tasks, it is the symbiotic relationship between human ingenuity and machine efficiency that will drive the industry's future. As AI technologies continue to evolve, there's a distinct need for educational enhancements and policy developments to ensure a balanced and ethical approach to AI integration in software development and beyond (source).
Learn to use AI like a Pro
Get the latest AI workflows to boost your productivity and business performance, delivered weekly by expert consultants. Enjoy step-by-step guides, weekly Q&A sessions, and full access to our AI workflow archive.
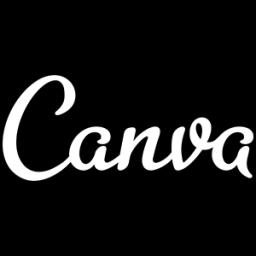
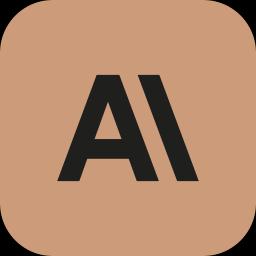
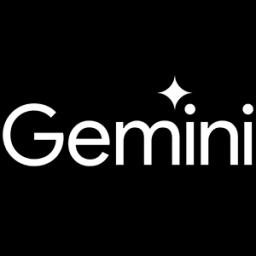
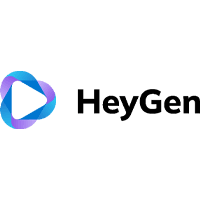
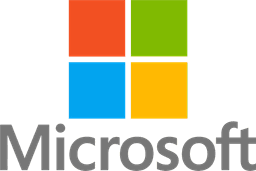
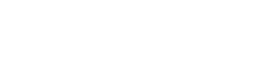
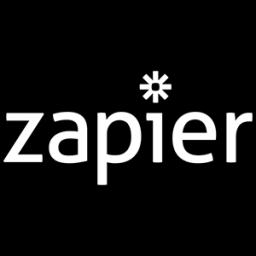
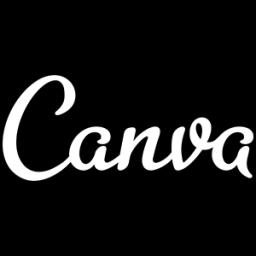
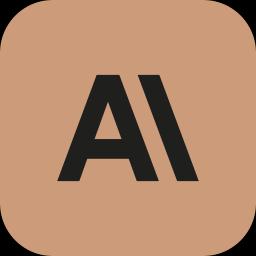
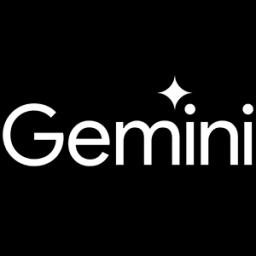
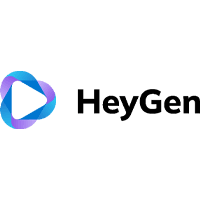
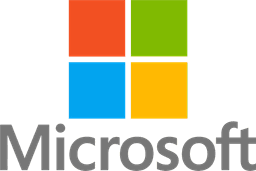
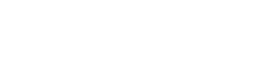
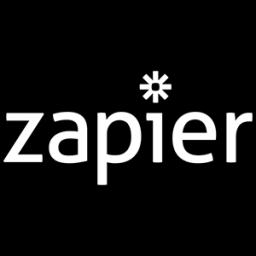
Public and Expert Reactions
The introduction of OpenAI's SWE-Lancer benchmark has elicited diverse reactions from both the public and experts alike. Many experts find the benchmark's approach commendable as it evaluates AI models against actual software engineering tasks sourced from platforms like Upwork, encapsulating real-world challenges. Such an innovative benchmark allows for a comprehensive assessment of AI capabilities in the software development domain. A specific highlight was Claude 3.5 Sonnet emerging as the top performer, achieving around 40% of the potential $1 million earnings, sparking discussions on AI's evolving role in the industry .
Despite the impressive performance of AI models like Claude 3.5 Sonnet, experts and developers are cautious about overestimating AI's current capabilities in the field of software engineering. Discussions on platforms such as Hacker News reveal that while the AI's task success rate was notable, it also exposed significant limitations, such as AI's inability to fully match the nuanced problem-solving and context-awareness required by human engineers in real-world scenarios .
The public reactions were a mix of excitement and skepticism. On social media and forums, users appreciated the progress but also voiced apprehensions regarding the potential challenges in implementing AI fully in software engineering tasks. Concerns about the benchmark’s methodology, possible data contamination during AI training, and the adequacy of evaluation metrics were notably highlighted by users .
Experts have acknowledged the SWE-Lancer Diamond dataset release as a positive step towards encouraging further research and innovation in AI technology. However, they stress the need for improved AI reasoning capabilities to adequately handle complex, large-scale projects that require a deeper contextual understanding, similar to human engineers. The dialogue is increasingly revolving around how AI can augment current workflows rather than replace them .
Overall, the SWE-Lancer benchmark represents a significant leap in the assessment of AI in software engineering, setting a new standard for evaluating AI’s efficacy in practical applications. Yet, the expert consensus remains that AI will continue to function more as an auxiliary tool within engineering teams rather than as a standalone replacement for human developers. This transition urges industries to adapt by reshaping training and workforce development programs to make the most of AI-assisted productivity enhancements .
Future Implications and Workforce Adaptation
The advent of benchmarks like OpenAI's SWE-Lancer signals a transformative period in software engineering, where the lines between AI capabilities and human expertise blur. Such benchmarks, which rigorously test models like Claude 3.5 Sonnet, hint at a potential economic ecosystem where AI augments human work, capturing a notable portion of earnings in tasks that range from bug fixes to elaborate feature developments. As highlighted [here](https://www.maginative.com/article/openais-new-benchmark-tests-ai-models-against-real-world-software-engineering-tasks/), Claude 3.5 emerged as the frontrunner, demonstrating the capacity of AI to undertake specific segments of software engineering effectively. However, full replacement of human engineers remains an unachieved benchmark, as AI models are yet to navigate the complexities inherent in holistic software development processes.
Learn to use AI like a Pro
Get the latest AI workflows to boost your productivity and business performance, delivered weekly by expert consultants. Enjoy step-by-step guides, weekly Q&A sessions, and full access to our AI workflow archive.
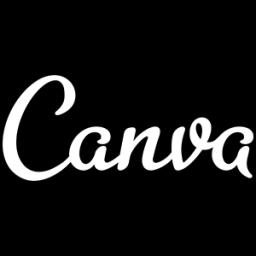
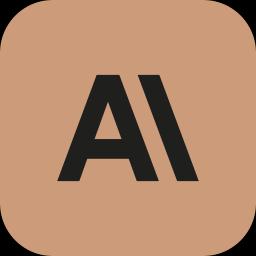
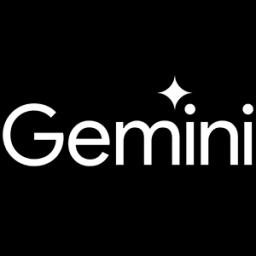
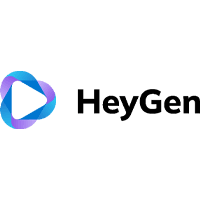
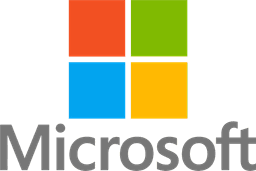
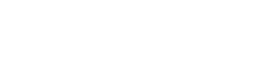
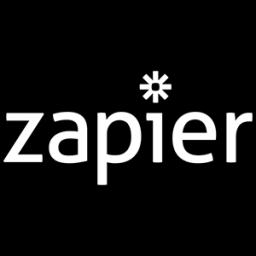
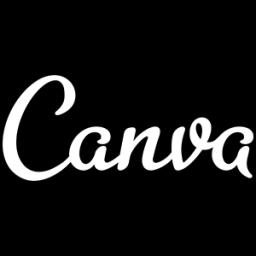
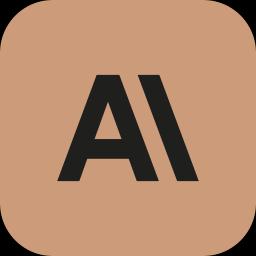
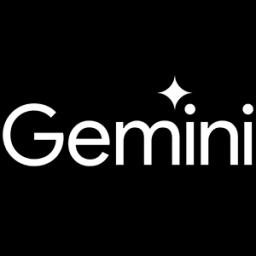
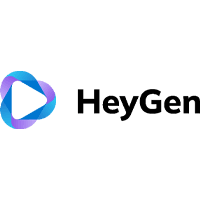
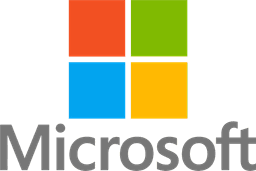
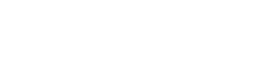
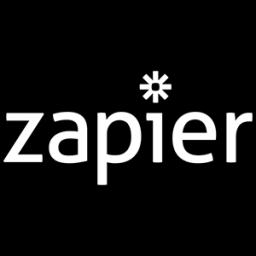
As AI becomes enmeshed in software engineering, the workforce faces imperative adaptation. The skills landscape is evolving, necessitating an embrace of AI-centric education and training platforms that equip current and future engineers with the acumen to work synergistically with AI technologies. Upgrades in professional training curricula are not just optional but essential, aligning educational outcomes with the rapid technological advancements embodied by AI. Industry analysts envision a future where AI handles repetitive tasks, freeing human resources to delve into complex problem-solving domains and nurturing critical thinking capabilities, a sentiment echoed in recent industry analyses [8](https://opentools.ai/news/openais-latest-study-ai-can-fix-bugs-but-struggles-to-find-them).
The emergence of AI solutions in code writing and error detection showcases an unprecedented efficiency gain in software development cycles, as evidenced by tools like Microsoft's Copilot and Amazon's CodeWhisperer [1](https://techcrunch.com/2025/01/15/microsoft-copilot-developer-milestone) [3](https://aws.amazon.com/blogs/aws/codewhisperer-enterprise-launch). These innovations underscore the importance of preemptive workforce adaptation to seize new opportunities while mitigating the risks associated with workforce displacement. Public sector involvement, in terms of policies and regulatory frameworks, will be critical in this transition, ensuring that the benefits of AI integration in software engineering extend equitably across societal strata.
Future workforce adaptation will not solely be about technical skills; it will also involve ethical considerations and understanding AI's broader societal impacts. As AI models are integrated into more strategic roles within development cycles, human oversight becomes paramount [4](https://github.blog/2025-02-01-introducing-security-copilot). Governments and organizations must promote regulatory standards that address AI biases and ethical concerns, fostering a balanced ecosystem where AI complements human creativity and judgment. By leveraging initiatives like SWE-Lancer Diamond and promoting dialogue on ethical AI utilization, we can steer software engineering towards a future that respects both technological progression and human values.