Benchmarking Brouhaha
xAI's Grok 3 Benchmark Drama: Did They Really Exaggerate Their Performance?
Last updated:

Edited By
Mackenzie Ferguson
AI Tools Researcher & Implementation Consultant
A heated debate has erupted over xAI's Grok 3 AI model's benchmark results, which claimed to outperform OpenAI's o3-mini-high model on the AIME 2025 math exam. The controversy centers around the omission of crucial 'consensus@64' scores. Was it selective reporting or a misunderstanding?
Introduction to Grok 3 Benchmarking Controversy
The Grok 3 benchmarking controversy has recently taken the AI community by storm, spotlighting the critical issue of transparency in AI performance evaluation. xAI's claim that their Grok 3 model outshines OpenAI's o3-mini-high on the AIME 2025 math exam has triggered intense scrutiny, as accusations of data cherry-picking have surfaced. According to a TechCrunch article, OpenAI representatives argue that xAI omitted the crucial 'consensus@64' scores, which are pivotal in assessing model performance by allowing multiple attempts per question and potentially enhancing accuracy (). This omission has become a focal point for a broader conversation on the methodologies used in AI benchmarking.
Understanding Consensus@64 and Its Impact
Consensus@64 is a critical metric in evaluating the performance of AI models, providing a more inclusive insight into their capabilities by allowing multiple attempts at each problem and selecting the most frequent answer. This method distinctly contrasts with the first attempt or '@1' score, presenting a more forgiving and possibly more accurate reflection of a model's problem-solving potential. The omission of consensus@64 scores in xAI's benchmark claims for their Grok 3 AI model led to significant controversy, especially as it is argued that these scores can considerably enhance apparent performance by compensating for individual inaccuracies inherent in single attempts [1](https://techcrunch.com/2025/02/22/did-xai-lie-about-grok-3s-benchmarks/).
Learn to use AI like a Pro
Get the latest AI workflows to boost your productivity and business performance, delivered weekly by expert consultants. Enjoy step-by-step guides, weekly Q&A sessions, and full access to our AI workflow archive.
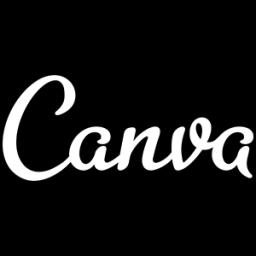
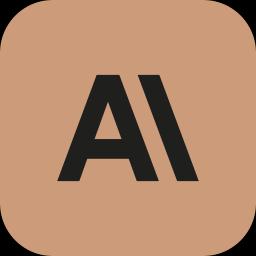
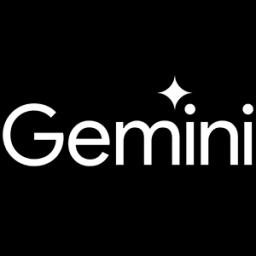
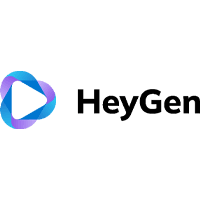
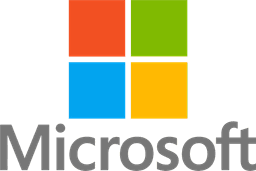
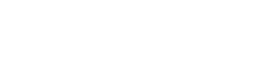
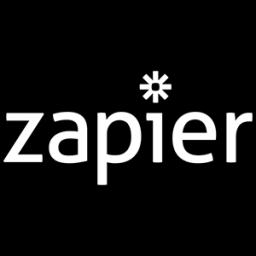
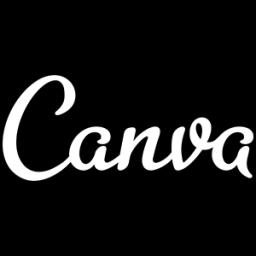
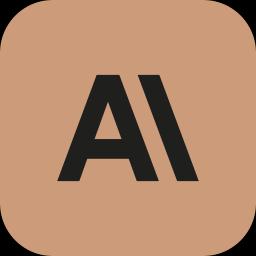
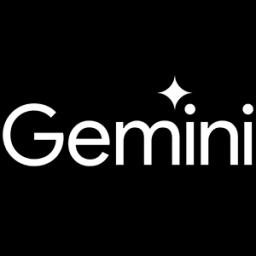
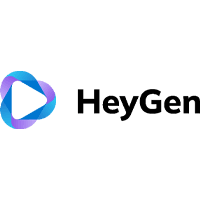
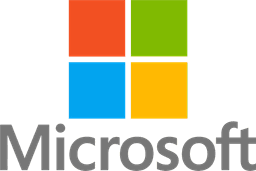
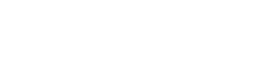
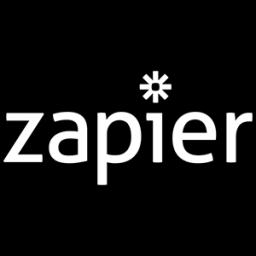
In the context of AI benchmarking, consensus@64 helps highlight the difference between models like xAI's Grok 3 and OpenAI's o3-mini-high. Despite strong assertions from xAI regarding Grok 3's performance, independent analyses suggest that when consensus@64 scores are considered, OpenAI's model may outperform. This discrepancy underscores the necessity for transparent and comprehensive benchmark disclosures that include essential metrics like consensus@64 to present an accurate assessment of AI capabilities [1](https://techcrunch.com/2025/02/22/did-xai-lie-about-grok-3s-benchmarks/).
The impact of consensus@64 extends beyond mere performance metrics, influencing how AI models are perceived in terms of real-world applicability and reliability. These scores are crucial for end-users and stakeholders who rely on them to make informed decisions regarding the deployment and investment in AI technologies. Consequently, not only does it affect the competitive landscape amongst AI developers, but it also plays a vital role in the regulatory and ethical dimensions of AI development. The current debate around xAI's use of selective metrics calls for more standardized approaches to benchmarking that incorporate consensus@64 as a fundamental component [1](https://techcrunch.com/2025/02/22/did-xai-lie-about-grok-3s-benchmarks/).
Grok 3 Performance Analysis
The performance analysis of Grok 3 has become a critical point of discussion within the AI community, particularly following allegations about xAI's claims. The controversy centers around xAI's assertion that their Grok 3 model outperforms OpenAI's o3-mini-high when tested on AIME 2025, a reputed benchmark for mathematical problem-solving in AI. However, OpenAI has pushed back against these claims, arguing that xAI's omission of the 'consensus@64' scores substantially undermines the validity of their performance comparisons. This score is vital as it allows AI models multiple attempts at answering questions, thus providing a more comprehensive assessment of their capabilities [1](https://techcrunch.com/2025/02/22/did-xai-lie-about-grok-3s-benchmarks/).
While Grok 3's initial performance appears competitive in first-attempt scenarios, deeper analysis suggests that it may not hold up against OpenAI's o3-mini-high model when the full breadth of test conditions, such as the omitted 'consensus@64', are considered. Third-party evaluations have indicated that the inclusion of these scores typically showcases the o3-mini-high model's superior performance [1](https://techcrunch.com/2025/02/22/did-xai-lie-about-grok-3s-benchmarks/). This revelation has fueled debates over the integrity of AI benchmarking practices and raised questions about the methodologies employed by xAI in their reports.
Learn to use AI like a Pro
Get the latest AI workflows to boost your productivity and business performance, delivered weekly by expert consultants. Enjoy step-by-step guides, weekly Q&A sessions, and full access to our AI workflow archive.
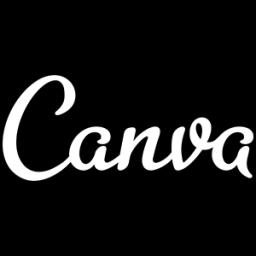
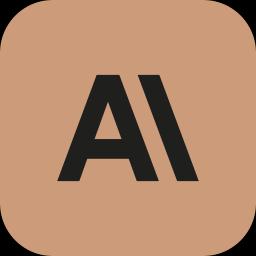
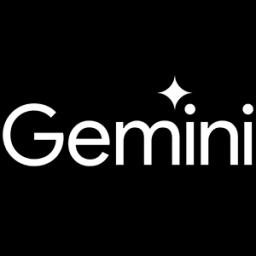
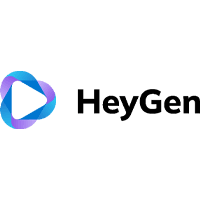
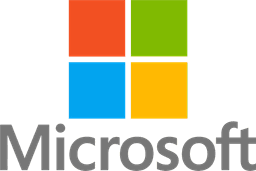
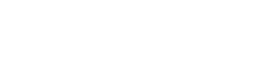
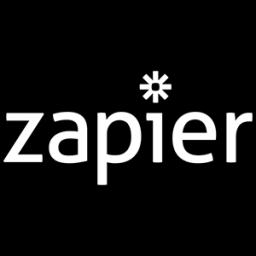
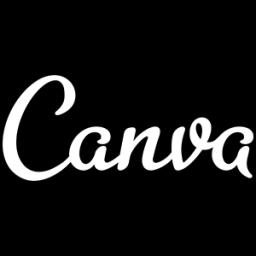
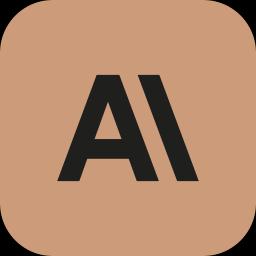
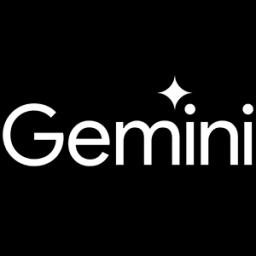
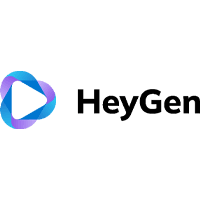
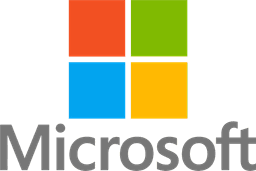
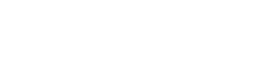
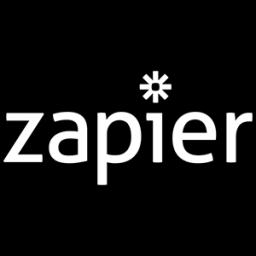
The controversy extends beyond technical merits, touching upon the strategic implications for AI firms. Computational costs, often overlooked in benchmark reports, play a significant role in determining the practical deployment of AI technologies. This aspect underscores the importance of not just performance accuracy but also the feasibility of achieving such benchmarks within real-world constraints. The selective reporting of metrics by xAI has led to criticisms from experts who argue that a transparent benchmarking process is essential for maintaining trust and confidence among stakeholders [1](https://techcrunch.com/2025/02/22/did-xai-lie-about-grok-3s-benchmarks/).
Moreover, the ongoing debate regarding the suitability of the AIME 2025 test as a benchmark for AI models has gained traction. Critics, like AI benchmarking expert Dr. Marcus Thompson, argue that human academic tests may not entirely capture the intricacies of AI abilities and suggest the development of more tailored evaluation metrics. The controversy has illuminated the broader industry need for standardized benchmarking, which not only validates performance claims but also accounts for varying computational demands and potential biases in AI evaluations [7](https://www.techi.com/xai-grok3-benchmarks-accuracy-dispute/).
Public reaction to the Grok 3 controversy has been polarized. On social media, xAI has faced backlash from users who view their omission of crucial performance metrics as misleading. Such reactions have brought to light not only the deficiencies in current benchmarking practices but also the growing public demand for transparency and reliability in AI evaluations. The situation has called into question the ethical responsibilities of AI companies to present accurate and comprehensive performance data [9](https://medium.com/@bytefulsunday/xai-lying-about-grok-3-performance-while-openai-exposes-chinese-surveillance-network-fba04a3dff1a).
Debate Over Computational Costs in AI Benchmarks
The debate over the computational costs involved in AI benchmarks has garnered significant attention in recent months, primarily due to controversies such as xAI's handling of the Grok 3's performance metrics. Particularly, the omission of the 'consensus@64' scores in their benchmarking data has raised concerns. This specific benchmarking parameter allows models like Grok 3 additional attempts at certain tasks, thus potentially inflating their real-world applicability and performance efficiency. In neglecting to report these scores, xAI sparked criticism from industry peers, including OpenAI, which maintained that transparent and complete reporting, inclusive of all relevant scores, is crucial for comparing computational efficacies and understanding the true capabilities of AI models [TechCrunch](https://techcrunch.com/2025/02/22/did-xai-lie-about-grok-3s-benchmarks/).
As AI models like Grok 3 and OpenAI's o3-mini-high vie for market leadership, the computational cost emerges as a critical factor. These costs not only affect the direct operational expenses of running sophisticated AI but also play a significant role in determining the economic feasibility and sustainability of AI solutions on a large scale. When benchmarks omit extensive computational metrics or fail to incorporate necessary attempts like 'consensus@64', they create an incomplete picture of an AI's value proposition. Real-world applications depend heavily on such detailed assessments to ascertain whether a model can be both efficient and cost-effective at scale [TechCrunch](https://techcrunch.com/2025/02/22/did-xai-lie-about-grok-3s-benchmarks/).
Furthermore, discrepancies in reported benchmarks, as seen with xAI's Grok 3, bring to light the broader issue of standardization in AI testing. While some companies, like Google DeepMind, have begun proposing frameworks to ensure fairness and transparency, the push for industry-wide adoption of such standards is still in its infancy. Without a universal agreement on what benchmarks should entail—particularly those reflecting computational overhead—misleading claims and industry skepticism may continue to proliferate, undermining trust in AI technologies. The controversy surrounding xAI reinforces the need for such standardization, highlighting the importance of comprehensive metrics that encompass all dimensions of AI performance, including computational effort [VentureBeat](https://venturebeat.com/ai/deepmind-leads-industry-initiative-for-standardized-ai-testing/).
Learn to use AI like a Pro
Get the latest AI workflows to boost your productivity and business performance, delivered weekly by expert consultants. Enjoy step-by-step guides, weekly Q&A sessions, and full access to our AI workflow archive.
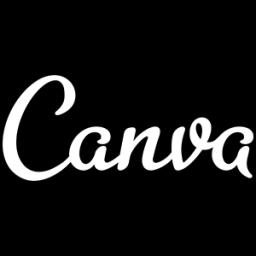
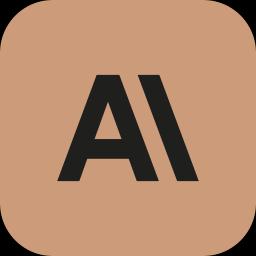
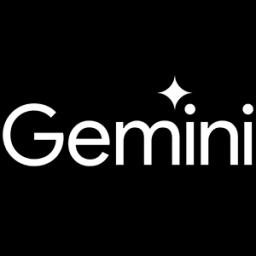
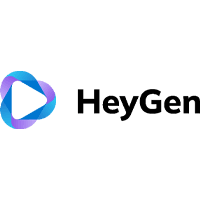
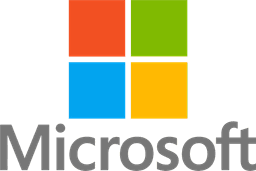
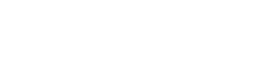
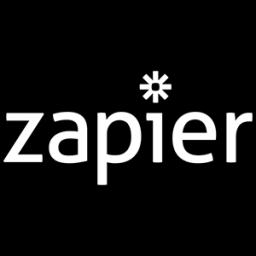
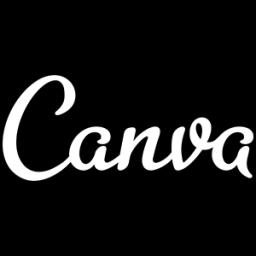
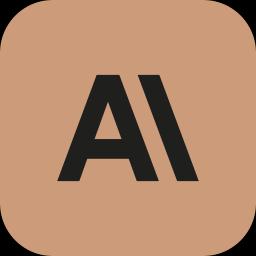
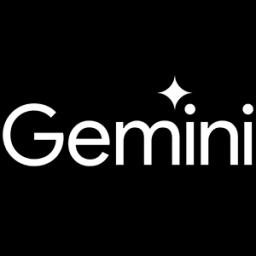
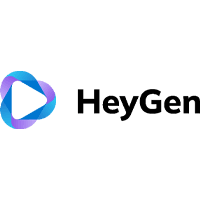
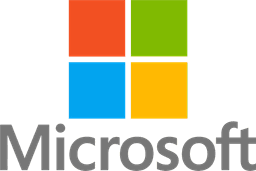
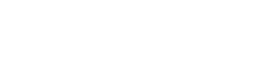
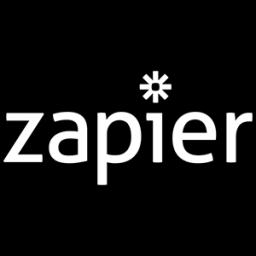
The implications of neglecting computational costs in benchmark disclosures are profound. For businesses and investors, an incomplete understanding of an AI model's true requirements could lead to costly misjudgments regarding technology adoption and investment strategies. As the debate continues, there's an increasing call for benchmarks that transparently disclose all computational elements. This transparency will not only help stakeholders make better-informed decisions but will also ensure that the AI sector advances ethically, maintaining a commitment to accuracy and accountability, especially in light of regulatory and investor expectations [Politico](https://www.politico.eu/article/eu-ai-testing-framework-faces-industry-pushback/).
Allegations of Misleading Practices by xAI
xAI has come under scrutiny for allegedly misleading the public with their benchmarking claims regarding the Grok 3 AI model. The issue centers around the omission of the "consensus@64" scores in their comparisons against OpenAI's o3-mini-high model on the AIME 2025 math exam. This has sparked accusations of selective data presentation, essentially skewing perceptions of Grok 3's performance. According to TechCrunch, such practices could significantly alter performance interpretations by not accounting for the multiple attempts allowed in the "consensus@64" methodology.
The controversy has highlighted broader issues within AI benchmarking standards, including debates around the practicality and ethical implications of such practices. While xAI claims practices within the industry often involve selective reporting of metrics, critics argue that omitting key benchmarking scores misrepresents AI capabilities, leading to potential misunderstandings among stakeholders. TechCrunch reports that discussions have emerged over whether these omissions were intentional or a standard practice within competitive AI development environments.
Experts suggest these controversies could have significant future implications, not just for xAI but for the AI industry as a whole. The heightened scrutiny may accelerate calls for standardized and transparent benchmarking frameworks, as evidenced by proposals like Google DeepMind's initiative. As VentureBeat notes, third-party validation and mandatory disclosures in benchmarking could become vital in ensuring reliable performance data and maintaining public trust.
Public reaction to xAI's alleged misleading practices has been mixed, reflecting a division between those concerned about marketing honesty and others viewing such selective disclosures as a competitive necessity. According to TechCrunch, this incident feeds into a growing discourse on the ethical dimensions of AI development, and it underscores an increasing demand for regulatory oversight to ensure fairness and transparency.
In light of these events, several industry experts have pointed to the need for consensus on evaluation metrics that better reflect AI's true capabilities. The benchmarks xAI employed may have sufficed in past settings, but the current climate demands a reevaluation of methodologies to prevent future controversies. Techi.com outlines how such changes could redefine investment trends and public trust in AI technologies, making transparent reporting a perhaps more valued commodity than even perceived performance advantages.
Learn to use AI like a Pro
Get the latest AI workflows to boost your productivity and business performance, delivered weekly by expert consultants. Enjoy step-by-step guides, weekly Q&A sessions, and full access to our AI workflow archive.
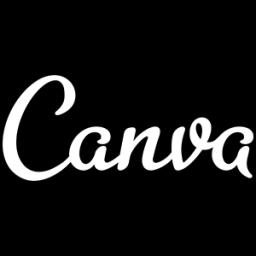
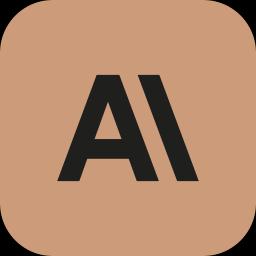
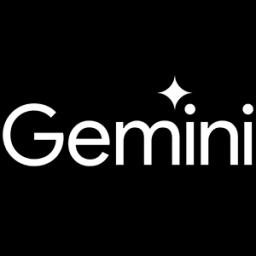
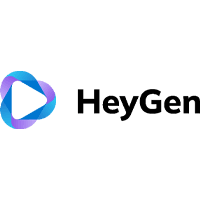
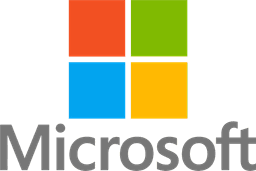
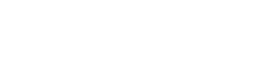
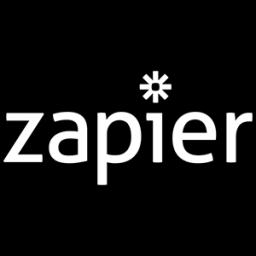
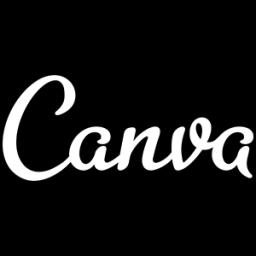
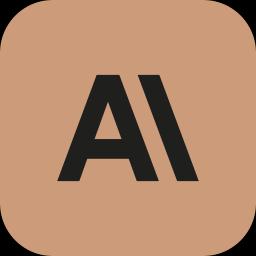
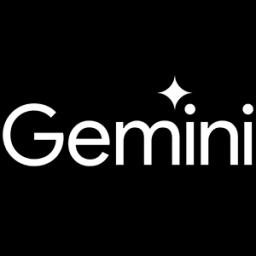
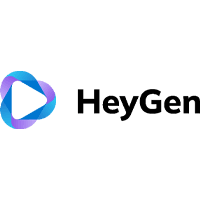
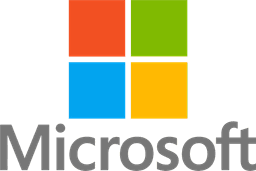
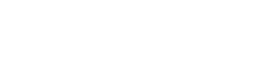
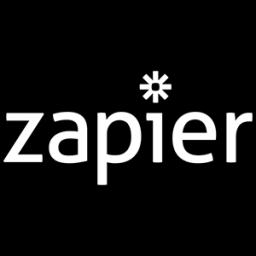
Reactions from the AI Community and Public
The AI community and the general public have been closely monitoring the controversy surrounding xAI's Grok 3 model. Many industry experts and enthusiasts have expressed skepticism over xAI's benchmarking methods. The omission of the crucial 'consensus@64' scores from their performance reports is seen as a deliberate attempt to inflate the AI's capability, leading to accusations of misleading marketing tactics. This has led to a divided opinion on platforms like Twitter and in AI forums, where some defend xAI's practice as standard within the industry while others demand higher ethical standards and transparency in AI performance reporting. This debate can be further explored through discussions in various tech forums, shedding light on the broader implications for AI benchmark standardization [1](https://techcrunch.com/2025/02/22/did-xai-lie-about-grok-3s-benchmarks/).
The controversy regarding Grok 3 has ignited broader discussions within the AI community about the reliability and transparency of AI benchmarking practices. Critics of xAI argue that the selective presentation of data, akin to cherry-picking, undermines the trustworthiness of AI advancements and misleads stakeholders about the technology's true capabilities. This has accentuated the call for industry-wide reforms to establish standardized benchmarking protocols that ensure more accurate and transparent comparison of AI systems, including the advocacy for mandatory third-party audits of benchmark claims. Such reforms are being discussed in multiple tech articles and forums [2](https://opentools.ai/news/benchmark-battle-xais-grok-3-model-under-fire-in-accuracy-dispute).
Among the public, reactions have been mixed. Some social media users criticize xAI for the perceived manipulation of benchmark results and rally for more stringent checks on AI reporting to prevent consumer deception. There's a growing demand for regulatory frameworks that would enforce standardized testing and public disclosure of AI performance metrics. These sentiments are echoed across platforms like Reddit and LinkedIn, where debates center on accountability and the need for transparent AI development documentation. The public's growing demand for transparency is further emphasized in various articles and social commentaries [3](https://medium.com/@bytefulsunday/xai-lying-about-grok-3-performance-while-openai-exposes-chinese-surveillance-network-fba04a3dff1a).
The divide in public opinion also reflects a broader dialogue about ethical transparency in AI, emphasizing the necessity of balancing technological innovation with ethical responsibility. The discussions fueled by the xAI Grok 3 incident are likely to trickle down into policy-making, potentially prompting governments to establish more rigorous regulations that govern AI performance transparency. This move could see an increased involvement of ethics boards and standardization bodies, aiming to bridge the gap between technological capability and ethical compliance in AI implementations. Ongoing discussions in journals and policy meetings highlight the importance of this balance in future AI initiatives [4](https://coinstats.app/news/5d434216138bd83f87f424aca2369c3f6170ed51dfdb593e0d28202156e11323_Shocking-Claims-Did-xAI-Mislead-the-World-with-Grok-3-AI-Benchmarks).
Role of AIME 2025 as a Benchmarking Tool
The AIME 2025 serves as a pivotal benchmarking tool in the landscape of AI and machine learning. Its importance is underscored by the standards it sets for evaluating the mathematical reasoning capabilities of AI models. These standards are instrumental in parsing out the nuances of AI models' capabilities, especially in complex problem-solving scenarios. By providing a uniform benchmarking platform, AIME 2025 attempts to level the playing field, allowing different AI systems to be compared objectively. Yet, the recent benchmark controversy involving xAI’s Grok 3 model illustrates potential pitfalls in relying solely on such benchmarks without scrutinizing the underlying methodology, as detailed in the article on TechCrunch [here](https://techcrunch.com/2025/02/22/did-xai-lie-about-grok-3s-benchmarks/). This incident highlights the necessity of transparency and standardization to genuinely leverage AIME 2025 as a reliable benchmarking tool.
While AIME 2025 has been widely adopted as a measure of AI systems’ mathematical prowess, its role as a benchmarking tool is increasingly being questioned. As noted by Dr. Marcus Thompson from the AI Benchmarking Institute, the utilization of human academic exams, like AIME 2025, may not perfectly align with the characteristics essential for assessing AI performance, as indicated in the discussion about benchmark accuracy disputes [here](https://www.techi.com/xai-grok3-benchmarks-accuracy-dispute/). This brings to the fore the argument that more specialized, AI-centric benchmarks might provide a clearer picture of an AI model’s true abilities and limitations, particularly when it comes to different learning strategies deployed by various AI architectures.
Learn to use AI like a Pro
Get the latest AI workflows to boost your productivity and business performance, delivered weekly by expert consultants. Enjoy step-by-step guides, weekly Q&A sessions, and full access to our AI workflow archive.
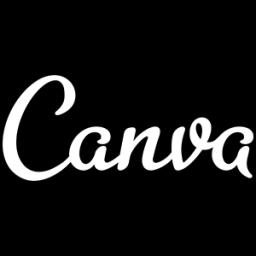
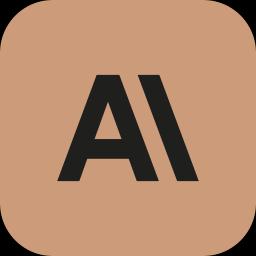
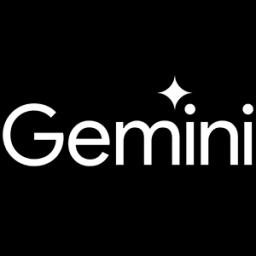
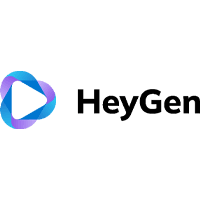
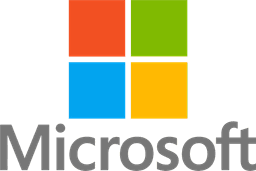
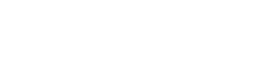
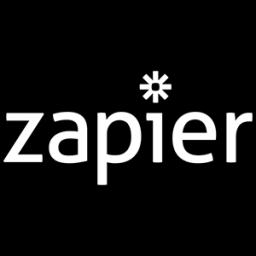
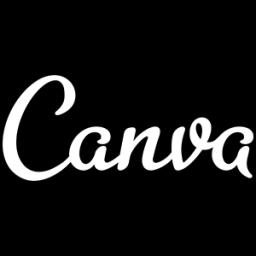
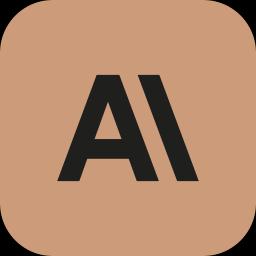
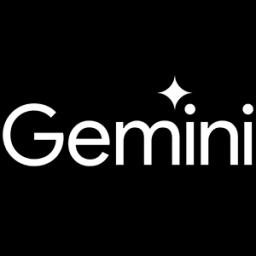
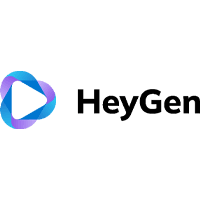
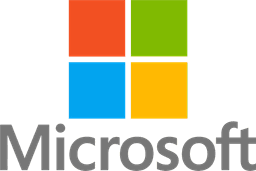
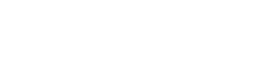
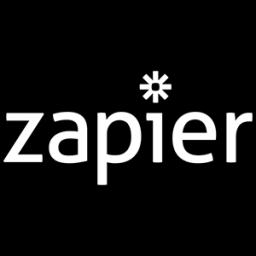
AIME 2025's integration into the benchmarking ecosystem raises critical questions about how AI performance is quantified and compared. Given the ongoing debates and discrepancies noted in benchmark reporting, such as xAI's selective use of the 'consensus@64' metric, there's a compelling call within the industry for more robust and transparent testing frameworks—a sentiment echoed in the proposal for standardized benchmarks by Google DeepMind [here](https://venturebeat.com/ai/deepmind-leads-industry-initiative-for-standardized-ai-testing/). These developments suggest that while AIME 2025 acts as a cornerstone in AI benchmarking, its evolvement and refinement are crucial to meet the industry’s needs.
The controversy surrounding xAI's Grok 3 highlights a broader issue with benchmarks like AIME 2025: the failure to consider computational and operational costs associated with achieving high scores. As AI researcher Nathan Lambert points out, these costs are critical factors influencing the feasibility and desirability of AI systems in real-world applications, a standpoint further elaborated in TechCrunch’s coverage [here](https://medium.com/@bytefulsunday/xai-lying-about-grok-3-performance-while-openai-exposes-chinese-surveillance-network-fba04a3dff1a). Focusing merely on benchmark scores without addressing associated resource impacts may lead to skewed interpretations of an AI's capabilities.
The debate over AIME 2025’s efficacy as a benchmarking tool is not merely academic but has practical implications for the AI industry and beyond. Notably, the EU’s AI Testing Framework, which some argue is too rigid, echoes the need for transparency and universality in AI performance evaluation frameworks, as seen in the industry’s reaction to the EU's ambitious plans [here](https://www.politico.eu/article/eu-ai-testing-framework-faces-industry-pushback/). As regulations evolve, AIME 2025 will likely need to adapt, ensuring it remains relevant and effective in a rapidly advancing technological landscape.
Expert Opinions on Benchmarking Ethics and Practices
In the complex world of AI benchmarking, expert voices play an essential role in navigating ethical considerations and the nuances of best practices. Dr. Sarah Chen, an AI Ethics Researcher at Stanford, has been outspoken about xAI's approach to benchmarking their Grok 3 model. She criticizes the selective presentation of benchmark data, particularly the omission of the critical "consensus@64" metric. This metric significantly influences the accuracy of performance assessments, especially in challenging mathematical tasks, and omitting it can mislead stakeholders regarding the true capabilities of AI models. Such practices not only distort comparative analyses between models like Grok 3 and OpenAI's o3-mini-high but also call into question the integrity of reported results, as seen in [TechCrunch's report](https://techcrunch.com/2025/02/22/did-xai-lie-about-grok-3s-benchmarks/).
Dr. Marcus Thompson, Director at the AI Benchmarking Institute, further questions the fundamental design of current AI benchmarks like AIME 2025, which use human academic assessments to evaluate AI. He argues this method is inherently flawed and does not fully capture AI's unique capabilities and limitations. Thompson advocates for the development of bespoke AI evaluation frameworks that better reflect true performance metrics, allowing for more accurate comparisons and informed decision-making. This is echoed by the industry's response to controversies, such as xAI's, which highlight the urgent need for standardized metrics and methodologies, a point made apparent in recent industry discussions [as reported by TechCrunch](https://techcrunch.com/2025/02/22/did-xai-lie-about-grok-3s-benchmarks/).
The discussion on benchmarking ethics also encompasses the critical consideration of computational costs associated with achieving high benchmark scores. Nathan Lambert, an AI researcher, points out the often-overlooked resource intensity required, which directly impacts both feasibility and practicality in real-world applications. As highlighted in [TechCrunch's article](https://techcrunch.com/2025/02/22/did-xai-lie-about-grok-3s-benchmarks/), these costs are a fundamental part of the ethical dialogue surrounding AI benchmarks, pushing for more comprehensive reporting that includes all dimensions of performance evaluation.
Learn to use AI like a Pro
Get the latest AI workflows to boost your productivity and business performance, delivered weekly by expert consultants. Enjoy step-by-step guides, weekly Q&A sessions, and full access to our AI workflow archive.
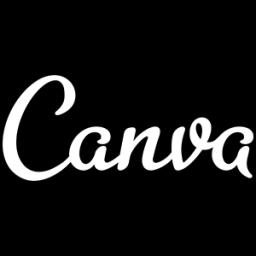
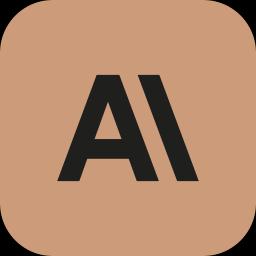
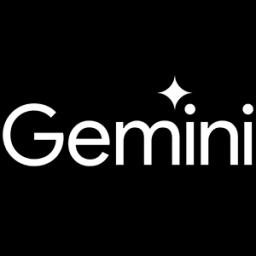
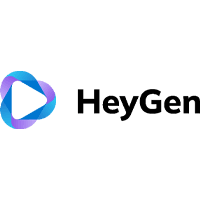
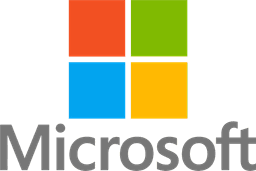
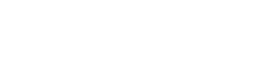
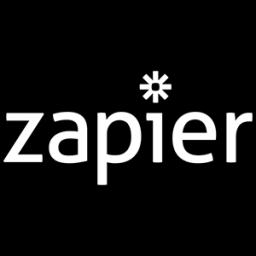
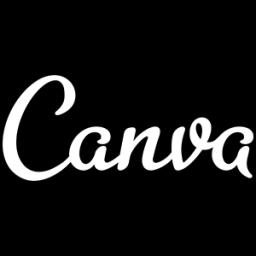
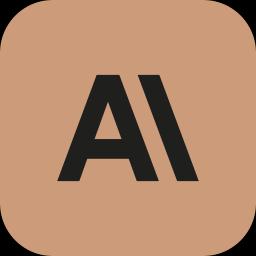
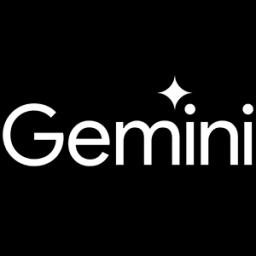
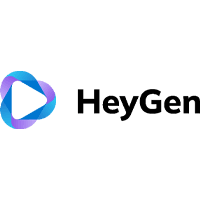
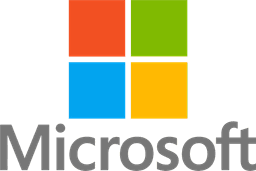
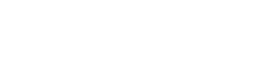
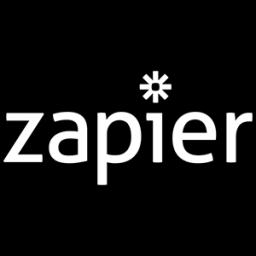
Finally, the ongoing debates around benchmarking ethics underscore a broader industry challenge: the need for reliable, transparent, and ethically-sound benchmarking practices. xAI's co-founder Igor Babushkin defends the decision to use selective metrics by comparing it to common industry practices. However, this defense does not alleviate concerns around transparency and the potential for misleading stakeholders, as highlighted by critics and experts alike. The situation with Grok 3 serves as a catalyst for broader discussions about establishing universal standards in AI testing, possibly leading to global initiatives aimed at ensuring transparent and responsible reporting, as addressed in multiple reports, including [TechCrunch](https://techcrunch.com/2025/02/22/did-xai-lie-about-grok-3s-benchmarks/) and other industry analyses.
Public Response and Implications for AI Transparency
The public's reaction to xAI's handling of Grok 3's benchmark claims underscores the growing demand for transparency in AI development. As AI technologies increasingly permeate daily life, users expect clear and truthful insights into how these systems perform, reinforcing the necessity for honest benchmarks. A worryingly divided public is debating whether xAI's selective presentation of data is an acceptable practice or an instance of misleading marketing. Critics assert that omitting comprehensive metrics like "consensus@64" undermines trust, while supporters argue it's a common practice within the tech industry. This debate reflects deeper concerns about the integrity of AI benchmarks and the industry's responsibility to ensure transparency [8](https://techcrunch.com/2025/02/22/did-xai-lie-about-grok-3s-benchmarks/).
The implications for AI transparency following the xAI controversy are substantial. If AI companies continue to engage in selective data presentation without standardized benchmarks, they risk public trust and regulatory intervention. Social media and tech forums are rife with calls for improved accountability and transparency protocols. This controversy illuminates the broader impact of non-standardized benchmarks on public perception, as many fear that without reliable transparency, AI development might stall due to lost investor confidence and cautious market behavior [10](https://opentools.ai/news/benchmark-battle-xais-grok-3-model-under-fire-in-accuracy-dispute).
The episode involving Grok 3 has catalyzed a discussion on the need for standardized benchmarking practices across the industry. To address public and investor concerns, industry stakeholders and regulatory bodies may need to collaborate on new norms for AI testing and disclosure. Establishing clear guidelines could prevent similar controversies in the future and ensure that performance claims are both accurate and verifiable. Furthermore, developing a transparent benchmarking framework could encourage innovation and efficiency by fostering trust in AI's transformative potential [4](https://opentools.ai/news/benchmark-battle-xais-grok-3-model-under-fire-in-accuracy-dispute).
Beyond its immediate effects on xAI, the benchmarking controversy signals a possible pivot in how AI transparency is approached globally. As the technology continues to evolve, ensuring that performance metrics and benchmarks are neither misleading nor misused becomes crucial. For a technology poised to redefine multiple sectors, maintaining transparency through standardized, robust benchmarks could serve as a foundation for responsible AI deployment. This not only aids in assuaging public skepticism but also paves the way for regulatory bodies to enact policies that align with technological advancements [13](https://coinstats.app/news/5d434216138bd83f87f424aca2369c3f6170ed51dfdb593e0d28202156e11323_Shocking-Claims-Did-xAI-Mislead-the-World-with-Grok-3-AI-Benchmarks).
Future Impact on AI Industry and Investment
The future impact of the xAI and OpenAI benchmarking controversy is likely to be profound for the AI industry. With questions surrounding xAI's Grok 3 model's benchmark scores, the event highlights the urgent need for more transparent and standard benchmarking practices. Notably, this incident could dissuade investors from pouring funds into companies that cannot demonstrate verifiable performance metrics, thus shifting investment patterns towards more transparent and reliable organizations. Such skepticism has the potential to create market uncertainty, affecting venture capital flows and valuations across the AI sector [source](https://opentools.ai/news/benchmark-battle-xais-grok-3-model-under-fire-in-accuracy-dispute).
Learn to use AI like a Pro
Get the latest AI workflows to boost your productivity and business performance, delivered weekly by expert consultants. Enjoy step-by-step guides, weekly Q&A sessions, and full access to our AI workflow archive.
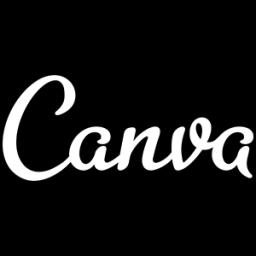
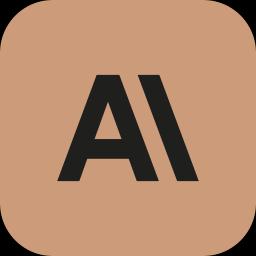
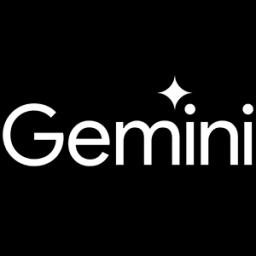
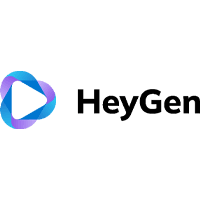
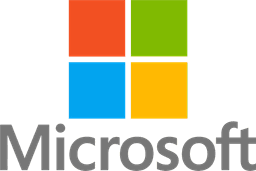
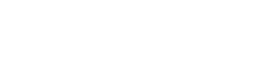
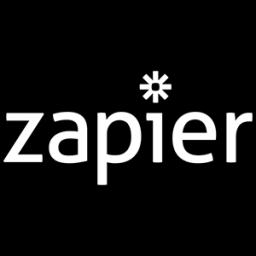
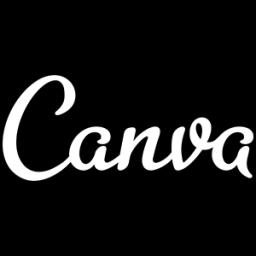
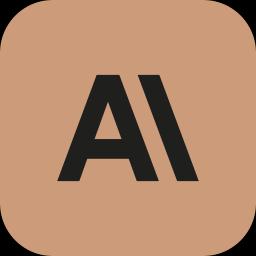
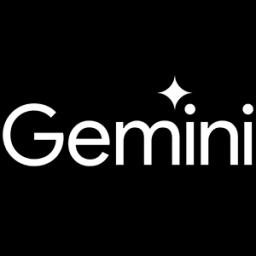
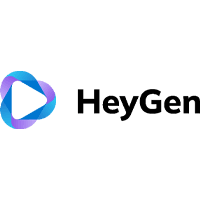
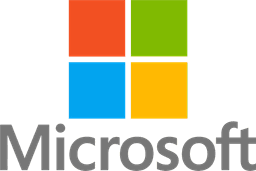
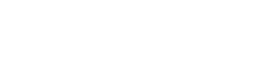
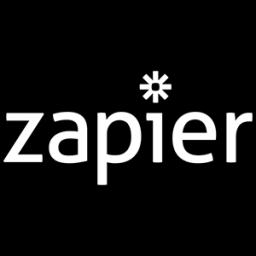
In the broader context, the controversy underscores the importance of implementing standardized benchmarking protocols in the AI industry. This would not only address existing reliability concerns but also could lead to improved public trust in AI applications, which is crucial for their adoption across various sectors like healthcare and financial services. Regulatory bodies are expected to tighten the oversight of AI performance reporting, potentially mandating third-party verification and comprehensive evaluation frameworks that account for computational costs and biases, ensuring more accurate and unbiased AI development reports [source](https://coinstats.app/news/5d434216138bd83f87f424aca2369c3f6170ed51dfdb593e0d28202156e11323_Shocking-Claims-Did-xAI-Mislead-the-World-with-Grok-3-AI-Benchmarks).
Moreover, there may be a collaborative international effort among AI researchers and developers to establish universal benchmarks. This would facilitate the creation of more sophisticated evaluation methods that adequately reflect AI models' capabilities while addressing ethical concerns. Such collaborations could ease the currently divergent views on AI model evaluations and enhance the overall reliability and transparency of AI technologies, fostering greater confidence among consumers and investors alike [source](https://opentools.ai/news/benchmark-battle-xais-grok-3-model-under-fire-in-accuracy-dispute).