Transforming Codebases with AI Magic
AI and Data Determinism: Revolutionizing Large-Scale Code Modernization
Last updated:

Edited By
Mackenzie Ferguson
AI Tools Researcher & Implementation Consultant
Dive into the world of AI-driven code modernization with Lossless Semantic Trees (LSTs) and deterministic tools. Learn how these innovations promise more efficient and accurate code transformations. Say goodbye to the limitations of Abstract Syntax Trees (ASTs) and embrace a future where AI-powered refactoring reigns supreme.
Introduction to Data Determinism and AI in Code Modernization
The intersection of data determinism and artificial intelligence (AI) plays a pivotal role in contemporary code modernization efforts. As organizations grapple with the complexities of upgrading and modifying extensive codebases, leveraging AI's analytical capabilities in conjunction with data determinism offers a transformative approach. One of the critical components in this framework is the use of high-quality, context-rich data, which empowers AI-driven code transformations. By employing Lossless Semantic Trees (LSTs), developers gain a superior alternative to traditional Abstract Syntax Trees (ASTs). This innovative perspective ensures more accurate and contextually aware code modifications that mitigate common errors associated with inadequate data representation.
The effectiveness of AI in code modernization is significantly enhanced by its synergy with deterministic tools such as OpenRewrite recipes. This collaboration enables autonomous code analysis, refactoring, and adaptation, fostering more efficient modernization processes. Integrating data determinism into AI workflows addresses the challenges posed by large-scale transformations, offering a structured methodology for dealing with dependencies and environmental contexts. By combining AI's reasoning capabilities with deterministic approaches, organizations can achieve precise and scalable outcomes in their modernization projects, as highlighted in the discussions from recent insights.
Learn to use AI like a Pro
Get the latest AI workflows to boost your productivity and business performance, delivered weekly by expert consultants. Enjoy step-by-step guides, weekly Q&A sessions, and full access to our AI workflow archive.
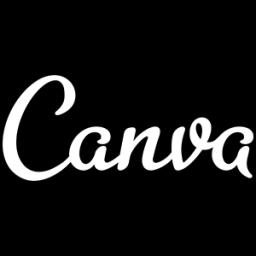
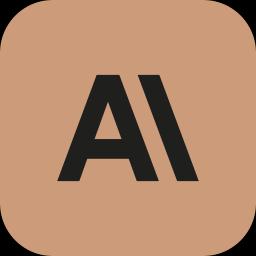
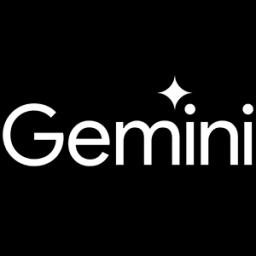
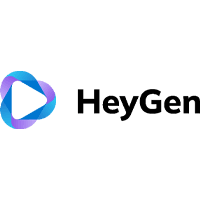
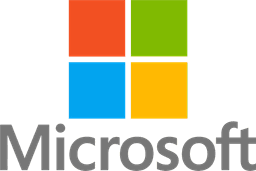
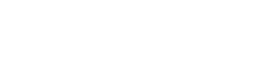
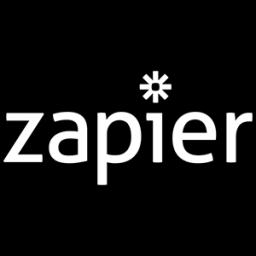
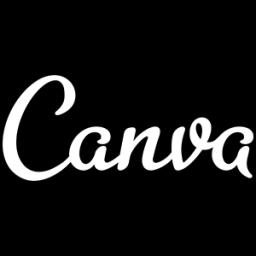
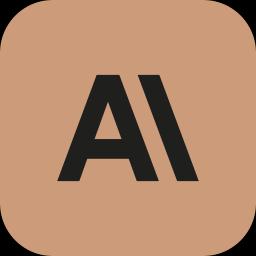
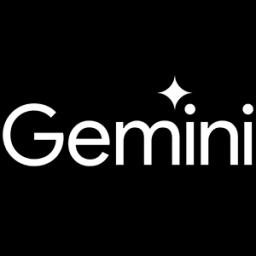
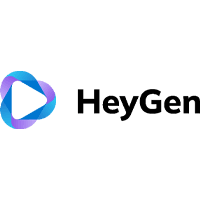
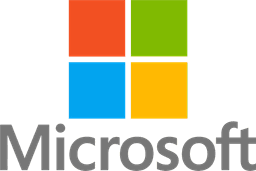
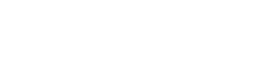
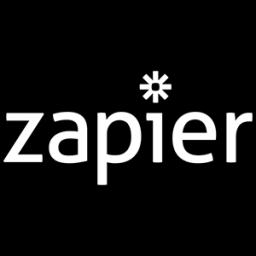
In the realm of software engineering, the transition from traditional ASTs to the more comprehensive LSTs marks a significant evolution in handling code data. LSTs augment the depth of code understanding by embedding metadata that includes type attribution and formatting details, which are crucial for accurate transformations. This enhancement not only facilitates better code analysis but also empowers AI systems to make informed decisions that align with the original intention behind the code's architecture. Consequently, it reduces the risk of introducing inaccuracies during the modernization process.
AI's ability to invoke specific deterministic tools through mechanisms like tool calling further underscores its potential in code modernization efforts. This capability allows AI models to harness the precision of pre-built tools, marrying them with AI's dynamic reasoning to execute sophisticated code transformations. As a result, organizations benefit from improved security, optimized dependency management, and reduced manual efforts. The culmination of these elements signifies a leap towards achieving seamless, efficient, and effective modernization processes. The comprehensive insights provided by expert opinions paint a vivid picture of the potential that lies in this synergy.
Limitations of Abstract Syntax Trees (ASTs)
Abstract Syntax Trees (ASTs) offer a structural representation of source code, presenting the syntax in a hierarchical tree format that illustrates the syntactic structure of the program. However, one major limitation of ASTs is their inability to capture the full semantics of a codebase. They often lack critical information such as formatting details and environmental context necessary for accurate code interpretation. This absence can lead to misinterpretations during code analysis, resulting in unexpected runtime errors or inefficient modernizations, especially when scaling across large codebases. The shortcomings of ASTs become evident as developers attempt to use them for advanced code transformations, where the lack of contextual metadata restricts the precision required for such complex tasks. Learn more about AST limitations.
Furthermore, ASTs do not inherently store type information or the dependencies between the components of the code, which can lead to significant challenges. As developers strive to upgrade codebases, particularly in a large-scale environment, these missing components can prevent the proper propagation of type information across interconnected modules. This omission can complicate dependency management and increase the risk of introducing compatibility issues when the system is updated, leading to potentially costly setbacks or system failures. In essence, without these vital pieces, ASTs cannot provide the holistic view necessary for seamless transformations across interconnected systems as detailed in recent advancements.
Learn to use AI like a Pro
Get the latest AI workflows to boost your productivity and business performance, delivered weekly by expert consultants. Enjoy step-by-step guides, weekly Q&A sessions, and full access to our AI workflow archive.
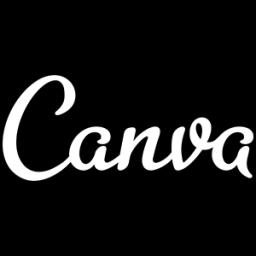
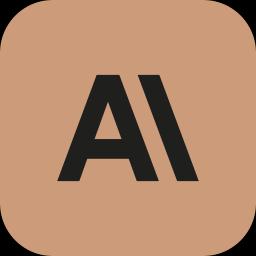
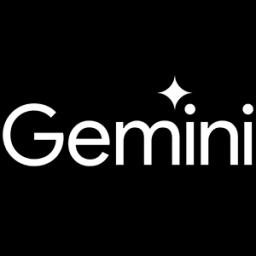
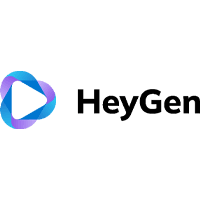
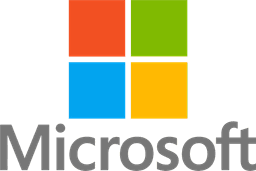
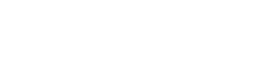
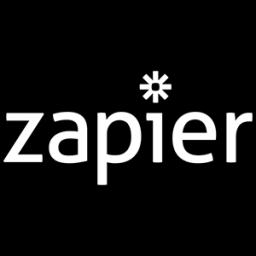
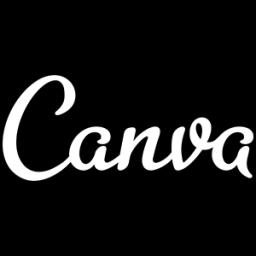
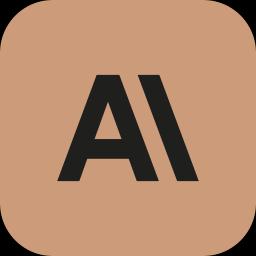
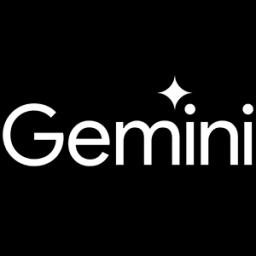
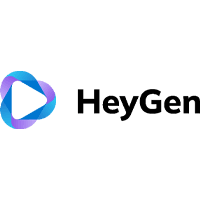
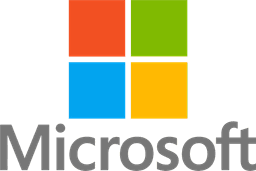
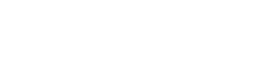
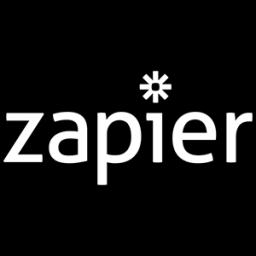
In addition to type and dependency issues, ASTs lack the capacity to represent the formatting intricacies of source code, such as whitespace and line breaks, which, while seemingly trivial, are crucial for maintaining certain structural and aesthetic properties of code. These elements can be vital for understanding the intent and functionality, especially in languages where indentation levels have semantic meaning, such as Python. This deficiency means that any refactoring based solely on ASTs may not retain the original formatting, potentially causing further complications or misinterpretations in code modernization efforts.
Moreover, ASTs are often challenged by the dynamic nature of modern software systems where behaviors are not solely defined by static code. For example, aspects such as runtime configuration or environment-specific dependencies, which are crucial for contextual understanding and accurate transformations, are often missing in AST representations. This limitation necessitates the introduction of more advanced models, such as Lossless Semantic Trees (LSTs), which aim to integrate semantic richness by encapsulating environment-specific information alongside the code's structural data, thereby overcoming many of the limitations posed by traditional ASTs. Explore this transition further.
Benefits of Lossless Semantic Trees (LSTs)
Lossless Semantic Trees (LSTs) offer significant advantages over traditional Abstract Syntax Trees (ASTs) by providing a more detailed and accurate representation of code. Unlike ASTs, which often miss key details such as type information, formatting, and the broader environmental context, LSTs include these critical components, delivering a comprehensive view of the codebase. This enriched representation is essential for AI-driven code modernization, allowing for more precise transformations. By incorporating all the necessary metadata, LSTs enable systems to perform accurate, context-aware code analyses and refactorings, minimizing the risks of errors and inconsistencies, particularly in complex, large-scale projects. This capability is highlighted in various innovative tools like Moderne's Moddy, a multi-repository AI code agent that uses the LST structure to enhance code analysis across numerous repositories [source].
The adoption of LSTs, along with AI's sophisticated reasoning capabilities and deterministic tools like OpenRewrite, radically transforms the landscape of code modernization. This synergy allows AI models to interact with deterministic tools, enabling precise modifications and adaptable code transformations that previously relied heavily on manual interventions. LSTs provide the rich, exhaustive data necessary for these processes, ensuring that every change considers the code's full context. Consequently, organizations can achieve greater accuracy and reliability in refactoring, significantly reducing the time and resources spent on code maintenance and enhancement. This efficient methodology not only accelerates modernization efforts but also enhances software quality and security by seamlessly integrating automated solutions to address vulnerabilities and code defects.
In practical terms, the integration of LSTs into the code modernization workflow can lead to notable improvements in both operational efficiency and strategic outcomes for organizations. By reducing reliance on error-prone and time-consuming manual interventions, LSTs facilitate a streamlined modernization process that frees up developer resources for innovation and strategic projects. Additionally, by maintaining a lossless, comprehensive record of the code’s structure and dependencies, LSTs guard against potential pitfalls during upgrades, such as dependency mismatches and runtime errors. This ensures that systems remain stable and reliable, a critical aspect for enterprises seeking to maintain competitive advantage through continual technological advancement. These improvements underscore the significant impact that LSTs can have when used in conjunction with advanced AI and tool-calling frameworks.
The Role of Tool Calling in AI-Driven Modernization
Tool calling represents a transformative approach within AI-driven modernization, enabling the seamless integration of deterministic tools with AI predictive analytics. By effectively calling these tools, AI systems can combine reasoning with the precision of existing code transformation recipes like OpenRewrite. This synergy ensures that AI can perform complex code analysis and refactoring tasks with accuracy and efficiency. Such integrations are crucial because they allow AI models to focus on strategic planning and decision-making, while deterministic tools ensure that the code transformations are executed accurately, minimizing human error and enhancing reliability .
Learn to use AI like a Pro
Get the latest AI workflows to boost your productivity and business performance, delivered weekly by expert consultants. Enjoy step-by-step guides, weekly Q&A sessions, and full access to our AI workflow archive.
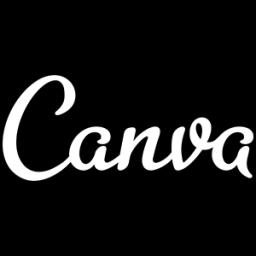
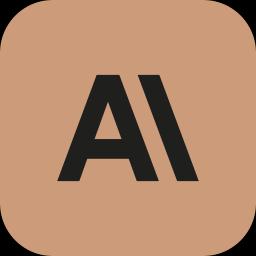
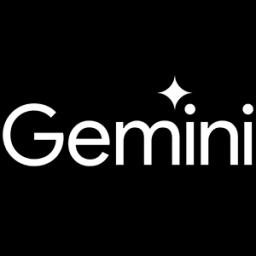
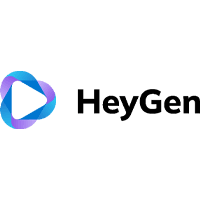
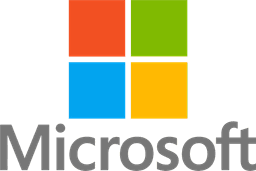
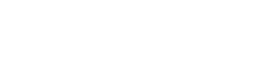
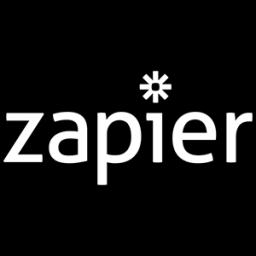
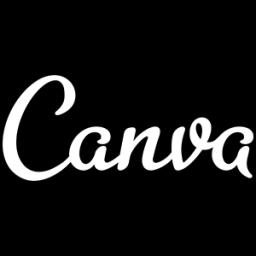
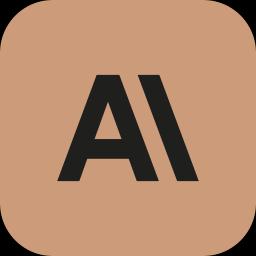
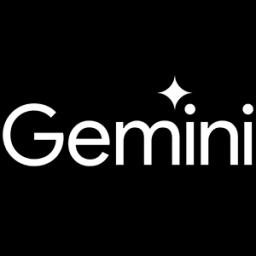
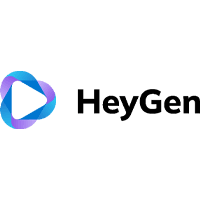
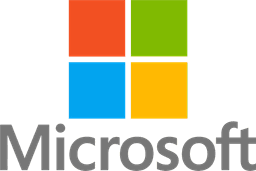
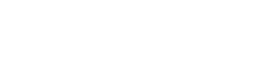
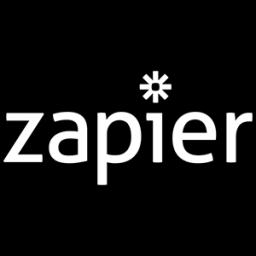
In AI-driven modernization, the capacity for tool calling is indispensable. It not only aids in executing precise code changes but also ensures that these changes are informed by data determinism. This means AI systems can rely on tools like OpenRewrite to undertake code modifications that are both context-aware and comprehensive. Moreover, this integration is vital for achieving scalability in code modernization projects. The collaborative force of AI's machine learning capabilities and deterministic tool functionality enables organizations to re-engineer vast codebases efficiently and accurately without sacrificing quality or introducing errors in the process .
Furthermore, the role of tool calling extends to enhancing autonomous AI-driven systems. By leveraging deterministic tools, AI systems gain the ability to perform self-directed refactoring tasks, aligning with the demands of large-scale code modernizations that require both precision and adaptability. This autonomous functioning not only reduces the need for manual intervention but also speeds up the modernization process, translating to significant cost savings for businesses. The incorporation of Lossless Semantic Trees and tool calling ensures that these modernizations are robust, addressing all facets of the code’s environment and dependencies effectively .
Tool calling also plays a pivotal role in enhancing the strategic value of AI in organizational alignments and migrations. It allows AI-driven systems to initiate and adapt to new methodologies by leveraging existing toolsets, ensuring transformations are not only technically sound but aligned with business objectives. By embedding precision tool calling into these processes, organizations can ensure that their AI systems enhance productivity, maintain high standards of code integrity, and support ongoing innovations .
The development and deployment of tool calling in AI present a forward-thinking model for managing complex code ecosystems. By integrating deterministic tools into AI frameworks, stakeholders can facilitate more dynamic, efficient interactions with codebases, greatly enhancing the modernization and maintenance processes. This ensures that modernization efforts are underpinned by both technological and strategic robustness. Tool calling, therefore, is an essential component in leveraging AI for comprehensive and scalable code modernization, ultimately transforming how organizations approach technological evolution and sustainability .
The Synergy of LSTs, Tool Calling, and AI
The growing interplay between Lossless Semantic Trees (LSTs), tool calling, and AI is spearheading a new era in software development, particularly in the domain of large-scale code modernization. LSTs provide an enriched representation of code by encapsulating not just the structural elements but also the metadata, such as type attribution and environmental context. This holistic approach greatly surpasses the traditional Abstract Syntax Trees (ASTs), which often lack these crucial details, leading to inaccuracies and potential errors during code refactoring. By leveraging LSTs, AI models are empowered to engage in precise and context-aware transformations, ultimately reducing the risk of errors and enhancing the efficiency of the code modernization process. This innovative approach is elaborated further in an insightful article on using AI and data determinism for code modernization, which you can access here.
Tool calling in AI-driven environments serves as a pivotal function that allows AI to utilize pre-existing deterministic tools, thus marrying the intuitive reasoning of AI with the established precision of these tools. This amalgamation ensures that complex tasks such as code analysis, refactoring, and adaptation are performed with heightened accuracy and efficiency. For instance, OpenRewrite recipes, when used in conjunction with LSTs, offer a formidable setup for exact code transformations. This blend of AI and deterministic tools not only accelerates the modernization process but also ensures traceability and predictability in the actions undertaken, aligning with concepts detailed in this article.
Learn to use AI like a Pro
Get the latest AI workflows to boost your productivity and business performance, delivered weekly by expert consultants. Enjoy step-by-step guides, weekly Q&A sessions, and full access to our AI workflow archive.
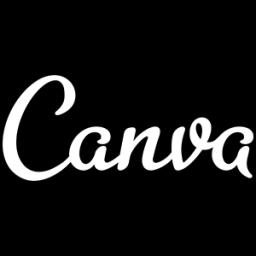
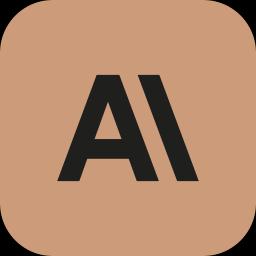
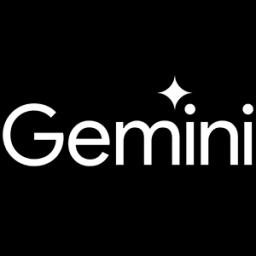
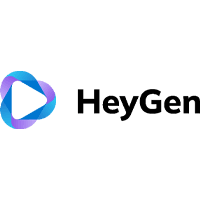
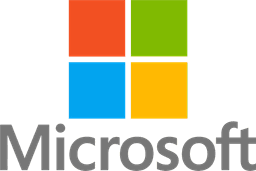
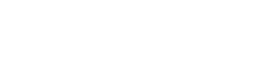
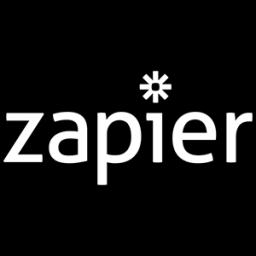
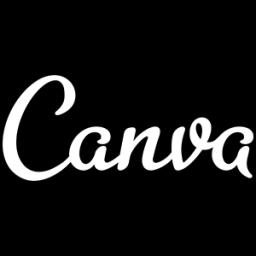
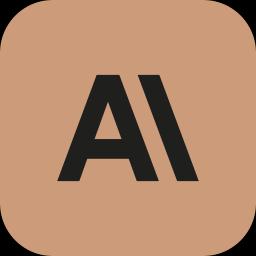
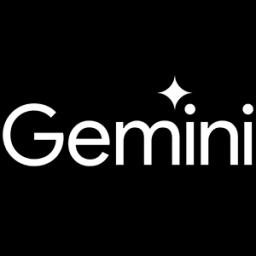
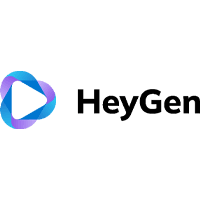
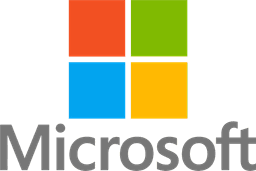
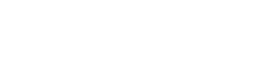
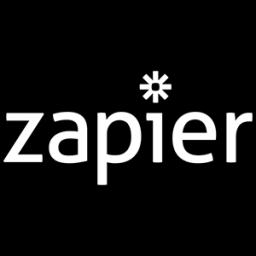
AI's role in this synthesis cannot be understated. By operationalizing its ability to reason and predict outcomes, AI provides the essential adaptability required in the ever-evolving terrain of software development. When integrated with LSTs and tool calling, AI’s orchestration takes on a new level of sophistication, overseeing scalable code modernization processes that are both autonomous and highly efficient. The importance of this synergy is pivotal as it allows organizations to not only maintain but enhance the security and functionality of vast codebases with minimal manual intervention, as discussed in the aforementioned article.
Collectively, the integration of LSTs, tool calling, and AI marks a significant leap forward in the field of code modernization. This multidisciplinary approach equips enterprises with the tools needed to not just keep up with, but to actively shape the technological tides. The financial implications for businesses embracing this strategy include reduced operational costs and an accelerated pathway to innovation, due to streamlined processes and enhanced capabilities in handling large-scale legacy systems. More information regarding the fusion of these technologies can be explored in the full article available here.
Practical Benefits for Organizations
Organizations today are increasingly recognizing the practical benefits that AI-driven code modernization brings, particularly when leveraging tools that integrate data determinism and advanced code representations like Lossless Semantic Trees (LSTs). Integrating these technologies not only enhances the accuracy and efficiency of code transformation projects but also significantly reduces manual effort and the risk of errors. By providing a more comprehensive and detail-rich understanding of codebases, LSTs enable organizations to tackle the complexities of large-scale modernization with greater ease and precision. This functionality proves especially beneficial in managing interdependent repositories and ensuring seamless transitions during upgrades, such as adopting new programming languages or frameworks.
One of the key practical benefits for organizations embracing AI-driven code modernization is the increased efficiency in managing extensive and complex codebases. By combining AI's reasoning capabilities with deterministic tools like OpenRewrite, companies can achieve autonomous code analysis, refactoring, and adaptation. This approach not only speeds up modernization processes but also enhances the robustness of the code by minimizing vulnerabilities and ensuring compliance with best practices. The automatic identification and rectification of legacy code issues, through technologies like AI and LSTs, allow organizations to maintain competitive advantage by continuously improving their software offerings without significant downtime or resource allocation.
Moreover, as organizations seek to optimize their digital transformation strategies, the adoption of AI-driven code modernization provides essential strategic advantages. Improved security through automated vulnerability scanning, effective dependency management, and reduced manual intervention are just a few highlights. These benefits translate to lower operational costs, as the time and resources traditionally required for manual code review and refactoring are drastically reduced. Consequently, organizations can reallocate resources to innovation and strategic initiatives, ultimately fostering a more agile and responsive business environment.
Moderne's Moddy AI Code Agent
Moderne's latest innovation, the Moddy AI Code Agent, represents a significant leap in the field of code modernization. Utilizing the OpenRewrite Lossless Semantic Tree (LST) representation, Moddy operates as a multi-repository AI agent capable of analyzing and evolving large codebases [source]. Unlike traditional methods that rely heavily on Abstract Syntax Trees (ASTs), which often fail to capture comprehensive details needed for precise code changes, LSTs provide a full-fidelity view that includes environmental context and metadata. This advancement allows Moddy to engage in deterministic code transformations with an unprecedented level of accuracy and efficiency, particularly vital for organizations managing vast and complex code repositories [source].
Learn to use AI like a Pro
Get the latest AI workflows to boost your productivity and business performance, delivered weekly by expert consultants. Enjoy step-by-step guides, weekly Q&A sessions, and full access to our AI workflow archive.
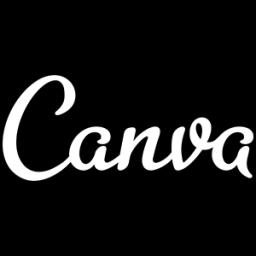
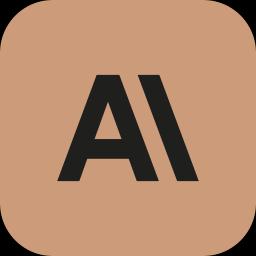
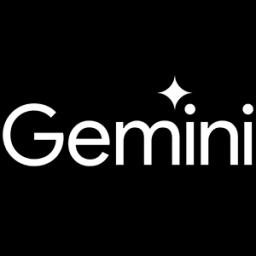
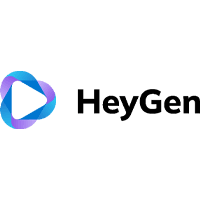
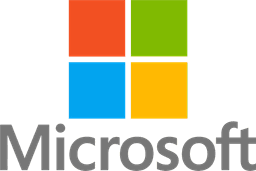
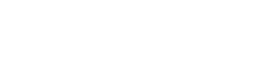
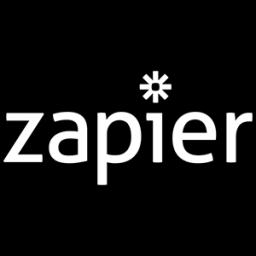
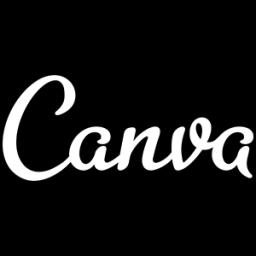
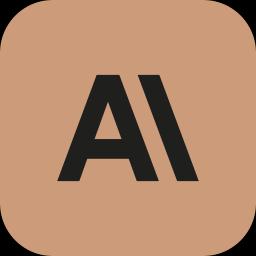
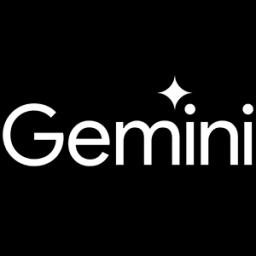
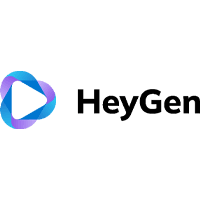
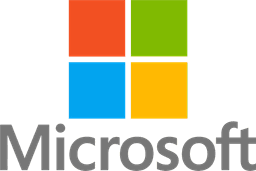
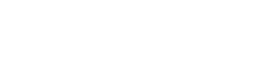
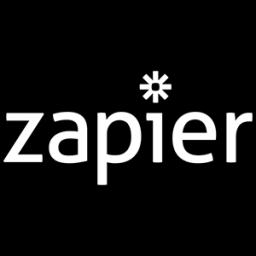
AI-driven code modernization is vastly improved through the integration of LSTs and deterministic tools like Moddy. This methodology offers a richer and more detailed representation of the code's architecture, significantly enhancing the agent's ability to conduct accurate transformations autonomously. With the backing of LSTs, Moddy can address limitations found in ASTs by preserving all necessary coding metadata, formatting, and dependencies, leading to a smoother refactoring process and minimized risk of errors or compatibility issues [source]. The introduction of tool calling within this framework further augments Moddy's capabilities, allowing it to utilize deterministic tools like OpenRewrite recipes seamlessly. This synergy of AI and deterministic tools, made possible through tool calling, ensures scalable and accurate code modernization [source].
Advantages of LSTs in Code Understanding
Lossless Semantic Trees (LSTs) have emerged as a transformative tool in enhancing code understanding, especially in the realm of large-scale modernization. Unlike traditional Abstract Syntax Trees (ASTs), LSTs preserve critical information about code, such as formatting, type attribution, and environmental context. This enriched data model provides a comprehensive view that is crucial for precise and effective code transformations. By retaining every nuanced detail, LSTs enable automated systems to perform more accurate code analysis and refactoring. This ensures that AI-driven modernization efforts are both efficient and reliable, reducing the risk of runtime errors due to misinterpretations inherent in using ASTs.
The integration of LSTs in code understanding paves the way for more scalable and autonomous code refactoring processes. With their ability to maintain a 'full-fidelity model' of the codebase, LSTs allow AI systems to operate with higher accuracy in code transformations. By providing rich metadata, LSTs facilitate a deeper understanding of the code's dependencies and the intricate connections within multi-repository projects. This capability is especially beneficial when dealing with complex systems requiring a thorough understanding of interdependent components and transitive dependencies, thereby minimizing potential errors during upgrades or adaptations.
AI's role in utilizing LSTs is amplified through the concept of tool calling, where AI-powered agents leverage deterministic tools alongside AI's reasoning capabilities. This synergy allows for precise execution of code changes and adaptations, harnessing the depth of information provided by LSTs. For instance, deterministic tools like OpenRewrite recipes can be invoked by AI to perform highly accurate modifications based on the LSTs' detailed insights. Thus, AI can plan, execute, and adapt code transformations with an unprecedented level of precision and predictability, ensuring that large-scale modernization efforts align closely with strategic business goals.
Organizations that harness the advantages of LSTs for code understanding realize multiple benefits that extend beyond mere technical improvements. By enabling more efficient code management and modernization, LSTs contribute to reducing the time and cost involved in these processes, which in turn allows organizations to allocate resources toward innovation and strategic initiatives. Moreover, the enhanced accuracy and reduced risk associated with LST-driven transformations help improve software quality and security, delivering robust and reliable systems that meet the evolving needs of users.
In the competitive landscape of technology, having an edge in code modernization is pivotal, and LSTs provide that advantage. They are not only tools for transformation but also for ensuring quality, compliance, and strategic alignment. As industries continue to evolve at a rapid pace, the integration of LSTs in AI-driven frameworks for code refactoring is poised to redefine how organizations approach code analysis, modernization, and execution, setting a new standard for efficiency and innovation in software development.
Learn to use AI like a Pro
Get the latest AI workflows to boost your productivity and business performance, delivered weekly by expert consultants. Enjoy step-by-step guides, weekly Q&A sessions, and full access to our AI workflow archive.
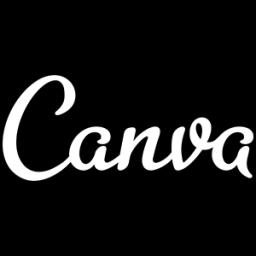
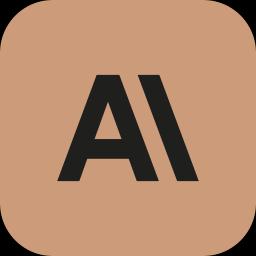
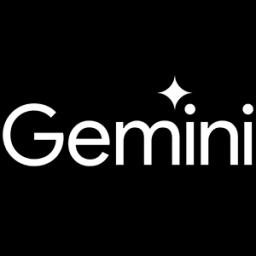
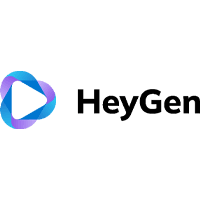
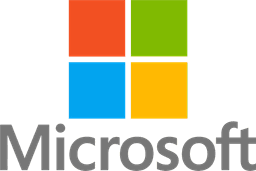
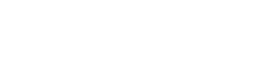
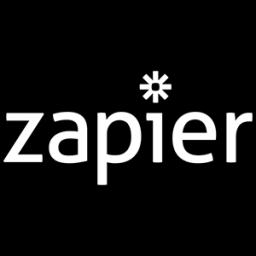
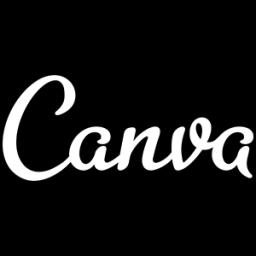
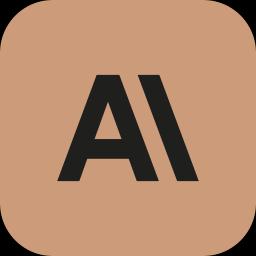
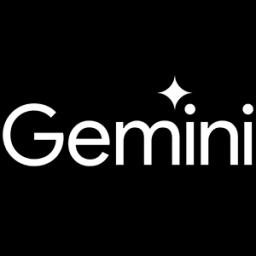
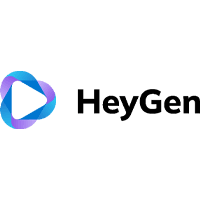
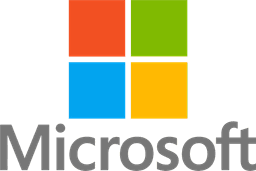
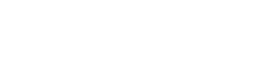
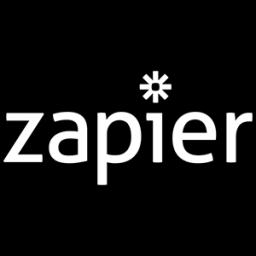
AI-Driven Code Refactoring and Automation
AI-driven code refactoring and automation represent a significant leap forward in the field of software engineering. As technology evolves, the demand for efficient, precise, and scalable code modernization techniques becomes a priority for many organizations. Leveraging AI capabilities in this context offers promising avenues for automating complex and repetitive refactoring tasks. AI tools, equipped with sophisticated algorithms, can actively scan through vast codebases, identify areas for improvement, and implement changes with minimal human intervention, thus reducing the potential for human error during manual code manipulation. According to [DevOps](https://devops.com/data-determinism-and-ai-in-mass-scale-code-modernization/?utm_source=rss&utm_medium=rss&utm_campaign=data-determinism-and-ai-in-mass-scale-code-modernization), this advancement in AI not only speeds up the code modernization process but also ensures a higher degree of accuracy in implementing code changes.
The automation of code refactoring through AI is primarily driven by enriched code modeling techniques such as Lossless Semantic Trees (LSTs). Unlike Abstract Syntax Trees (ASTs), LSTs offer a rich, detailed depiction of code, retaining critical environmental data necessary for accurate transformation. This enriched model allows AI systems to make informed decisions about refactoring without the risk of missing critical context found in traditional code representations. The [article](https://devops.com/data-determinism-and-ai-in-mass-scale-code-modernization/?utm_source=rss&utm_medium=rss&utm_campaign=data-determinism-and-ai-in-mass-scale-code-modernization) highlights how these superior data models, coupled with AI's reasoning power, are instrumental in driving scale and efficiency in code modernization projects.
Moreover, the concept of tool calling has revolutionized how AI interacts with deterministic tools within the realm of code modernization. By integrating deterministic tools like OpenRewrite with AI agents, organizations can achieve precise and predictable transformations across their codebases. According to [DevOps](https://devops.com/data-determinism-and-ai-in-mass-scale-code-modernization/), this technique capitalizes on AI's foresight and predictive capabilities, while relying on deterministic tools for executing code changes with surgical precision. This synergy vastly increases the reliability of AI-driven modernization efforts, ultimately minimizing risks associated with large-scale software changes.
Practical applications of these AI-driven techniques are already being observed in various sectors. For businesses dealing with vast, legacy codebases, the integration of AI-driven refactoring offers a pathway to modernize without the extensive cost and time investments traditionally required. As highlighted in the [DevOps article](https://devops.com/data-determinism-and-ai-in-mass-scale-code-modernization/), the ability to autonomously manage and update complex software systems means businesses can allocate more resources towards innovation while maintaining software security and efficiency. The automated identification and resolution of potential vulnerabilities and redundant code ensure that the software remains robust and up-to-date.
Looking ahead, the future of AI-driven code refactoring and automation holds the potential for even greater transformation across the industry. With continuous advancements in AI technology and data models, such as the ongoing development of LSTs, the scope for even more intricate and efficient automation techniques is vast. The disruptive potential of these technologies promises to radically alter software development practices, paving the way for a new era of streamlined and intelligent software engineering. According to [DevOps](https://devops.com/data-determinism-and-ai-in-mass-scale-code-modernization/), embracing these innovations not only ensures competitiveness but also positions businesses to better navigate the complex landscape of modern software demands.
Combining AI with Deterministic Tools for Scalability
At the heart of modern software development lies the challenge of balancing innovation with the scalability of processes. In recent years, the combination of Artificial Intelligence (AI) with deterministic tools has emerged as a revolutionary strategy to tackle this challenge, particularly in the context of code modernization efforts. AI technologies excel at mimicking human intelligence by analyzing patterns and learning over time, which endows them with the ability to make complex predictions and decisions. However, when AI is paired with deterministic tools—which are pre-configured to carry out specific, predictable tasks reliably—this synergy allows for enhanced scalability in software engineering projects. As highlighted by the recent advancements in using AI with data determinism for large-scale code modernization, it becomes clear that such a symbiotic relationship amplifies the strengths of both AI's flexibility and the precision of deterministic systems. More on this approach can be explored through the insights shared by industry experts [here](https://devops.com/data-determinism-and-ai-in-mass-scale-code-modernization/?utm_source=rss&utm_medium=rss&utm_campaign=data-determinism-and-ai-in-mass-scale-code-modernization).
Learn to use AI like a Pro
Get the latest AI workflows to boost your productivity and business performance, delivered weekly by expert consultants. Enjoy step-by-step guides, weekly Q&A sessions, and full access to our AI workflow archive.
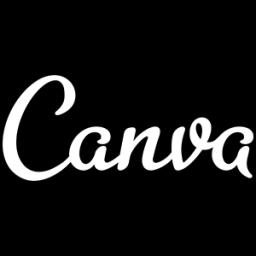
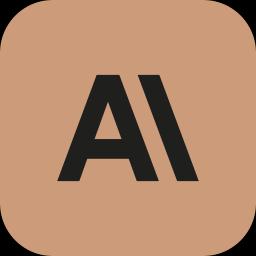
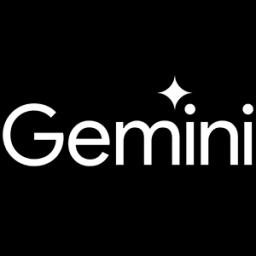
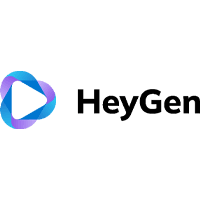
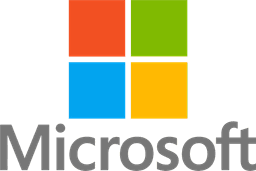
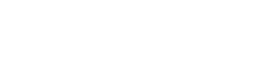
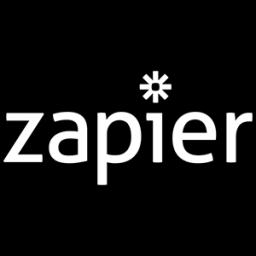
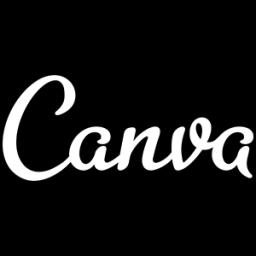
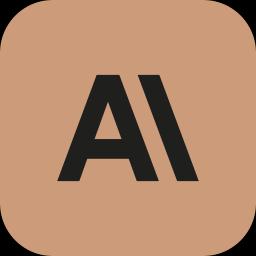
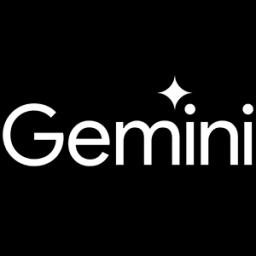
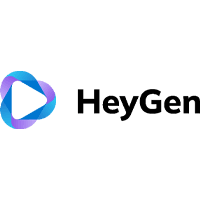
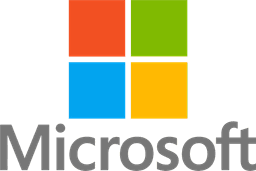
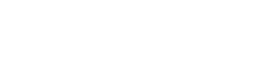
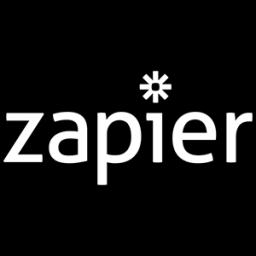
One of the cornerstones of combining AI with deterministic tools is the concept of using Lossless Semantic Trees (LSTs). Unlike traditional Abstract Syntax Trees (ASTs), LSTs provide a complete, enriched representation of code that includes essential metadata detailing the code’s environment. This comprehensive code representation allows AI systems to make more informed, accurate decisions during the modernization process. By synergizing AI's adaptable intelligence with the precise protocol execution of deterministic tools, teams are empowered to undertake complex refactoring tasks with confidence in the reliability of outcomes. The fusion of deterministic tools with AI doesn't just improve accuracy but also enhances the speed and scalability of code modernization efforts. To gain a deeper understanding of how LSTs outperform ASTs in this synergy, read more [here](https://www.moderne.ai/blog/lossless-semantic-tree-the-complete-code-data-model-for-automated-code-refactoring-and-analysis).
Scalability in AI-driven modernization is further supported by the concept of 'tool calling,' a method where AI agents dynamically leverage pre-existing deterministic tools, like OpenRewrite recipes, to perform specific code transformations. This integration fosters an environment where AI can independently assess and direct these tools to deliver optimal results in high-stakes application updates, such as those involving extensive codebases or critical system updates. As AI technology continues to evolve, its integration with deterministic tools helps nurture a unified platform capable of tackling the complexities inherent in maintaining and upgrading sprawling code infrastructures. More insights into the benefits and methodologies of 'tool calling' are available [here](https://devops.com/data-determinism-and-ai-in-mass-scale-code-modernization/?utm_source=rss&utm_medium=rss&utm_campaign=data-determinism-and-ai-in-mass-scale-code-modernization).
A remarkable advantage of this combination lies in the automation it brings to error-prone, labor-intensive tasks, freeing developers to focus on more strategic initiatives. With AI and deterministic tools working in concert, organizations benefit from streamlined processes that not only cut down time spent on routine checks and updates but also minimize the risk of human error. This level of autonomous oversight is particularly beneficial in large-scale enterprises where even minor errors can escalate into critical system failures. The continuous evolution of AI capabilities, aligned with deterministic methods, paves the way for autonomous systems that are both self-sufficient and self-correcting. For organizations looking to harness these advancements, understanding the full spectrum of potential that AI-deterministic tools offer is crucial, details of which can be found [here](https://devops.com/data-determinism-and-ai-in-mass-scale-code-modernization/?utm_source=rss&utm_medium=rss&utm_campaign=data-determinism-and-ai-in-mass-scale-code-modernization).
Ultimately, the integration of AI with deterministic tools represents a transformative approach to establishing a scalable framework for code modernization. Organizations adopting this strategy can anticipate not only improved efficiency but also increased accuracy in managing complex digital transformations. Given the growing need for agility and scalability in software solutions, the dynamism offered by AI combined with the robust dependability of deterministic tools is poised to redefine the landscape of code modernization, enabling businesses to innovate faster while effectively managing growth. This mindset shift toward leveraging AI's potential in concert with deterministic methodologies is available in detailed discussions by experts [here](https://devops.com/data-determinism-and-ai-in-mass-scale-code-modernization/?utm_source=rss&utm_medium=rss&utm_campaign=data-determinism-and-ai-in-mass-scale-code-modernization).
Expert Opinions on Code Modernization Challenges
Code modernization poses significant challenges, a fact underscored by expert opinions in the field. One major challenge lies in the limitations of traditional tools like Abstract Syntax Trees (ASTs). As noted by experts, ASTs often lack critical information such as code formatting and environmental context, which are essential for accurate code transformation at a large scale. This absence can lead to misinterpretations and subsequent runtime failures, especially when dealing large codebases. The adoption of Lossless Semantic Trees (LSTs) is highlighted as a key solution by experts. LSTs enrich code representation with metadata, providing a more holistic view that mitigates the traditional shortcomings of ASTs, thereby enhancing the accuracy and reliability of AI-driven transformations. More insights on this approach are available in an article discussing data determinism and AI in code modernization .
Another significant challenge identified by experts is ensuring precision in executing complex code transformations. This calls for a synergistic approach, combining AI's generative abilities with deterministic tools. Experts advocate for leveraging tools such as OpenRewrite recipes within AI workflows to bridge the gap between experimental AI outputs and the necessity for predictable, reliable results. This hybrid methodological approach is seen as pivotal in maintaining the balance between AI's potential and practical application needs at scale. The importance of such a strategy is detailed further .
Learn to use AI like a Pro
Get the latest AI workflows to boost your productivity and business performance, delivered weekly by expert consultants. Enjoy step-by-step guides, weekly Q&A sessions, and full access to our AI workflow archive.
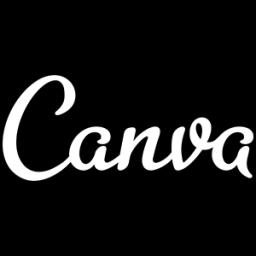
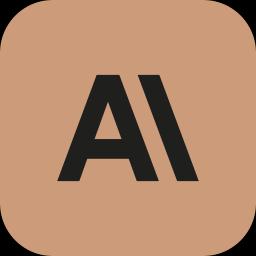
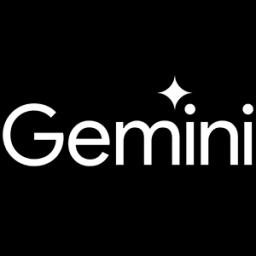
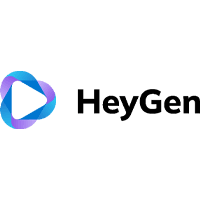
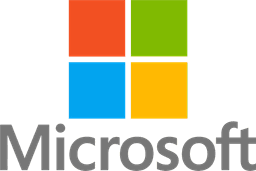
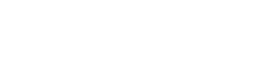
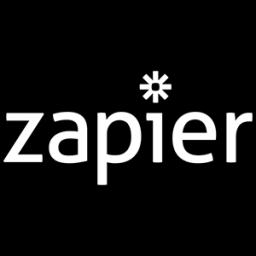
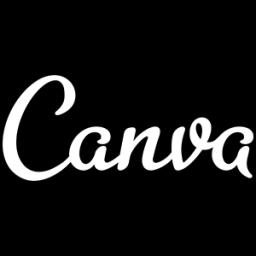
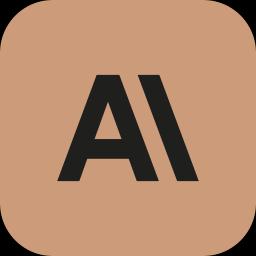
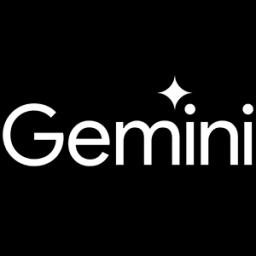
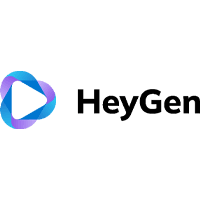
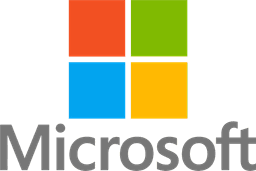
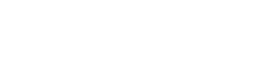
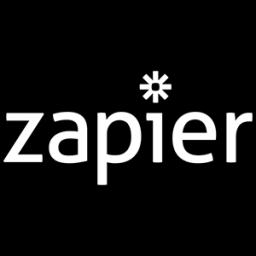
Moreover, experts highlight the adoption of "tool calling" as a crucial aspect of modern code transformation challenges. Tool calling enables AI agents to utilize established deterministic tools seamlessly. This capability ensures that even as AI models propose transformational changes, deterministic tools like OpenRewrite ensure these changes are feasible and correctly implemented. This approach not only enhances code quality but also builds a feedback loop that continuously improves both AI models and tool-sets. Insights into this application can be found in detailed discussions .
Future Implications: Economic, Social, and Political
The integration of AI and data determinism into code modernization practices has the potential to reshape economies on a grand scale. For businesses, adopting Lossless Semantic Trees (LSTs) and deterministic tools like OpenRewrite could slash the costs associated with extensive code updates and refactoring. According to various industry insights, such technological advancements could free up significant financial resources, enabling companies to redirect capital towards innovation and strategic pursuits. However, there remains a considerable upfront investment in AI infrastructure and the necessary expertise, which might prove challenging for smaller firms to overcome. Additionally, increasing reliance on AI-driven solutions may heighten the demand for AI specialists while traditional roles, such as software engineers, may face the necessity of retraining to stay relevant in an evolving job market.