New Wave of Inference Chips Poses a Challenge to Nvidia's GPU Dominance
AI Chip Wars: Nvidia Faces Fierce Competition from Inference Innovators
Last updated:

Edited By
Mackenzie Ferguson
AI Tools Researcher & Implementation Consultant
Nvidia's rivals like Cerebras, Groq, AMD, and Intel are challenging its position by developing specialized AI inference chips. These chips, aimed at executing AI models rather than training them, promise higher efficiency and lower costs, potentially revolutionizing AI adoption.
Introduction to AI Chips
Artificial Intelligence (AI) chips are specifically designed to accelerate machine learning tasks in AI applications. Unlike traditional general-purpose chips, AI chips are optimized for handling large datasets and performing complex calculations quickly and efficiently. The recent developments in AI chips have sparked significant interest among technological firms aiming to boost the performance and capabilities of AI systems while reducing latency and power consumption.
Nvidia's position in the AI chip market has predominantly focused on Graphics Processing Units (GPUs), which are well-suited for training AI models due to their ability to process multiple tasks simultaneously. However, emerging AI inference chips are carved out to handle tasks post-training, leading to an operational edge in efficiency and cost-effectiveness.
Learn to use AI like a Pro
Get the latest AI workflows to boost your productivity and business performance, delivered weekly by expert consultants. Enjoy step-by-step guides, weekly Q&A sessions, and full access to our AI workflow archive.
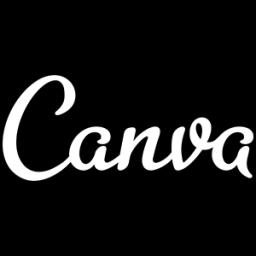
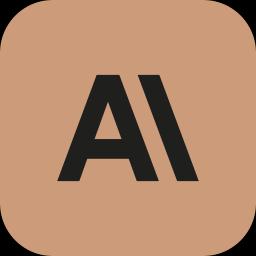
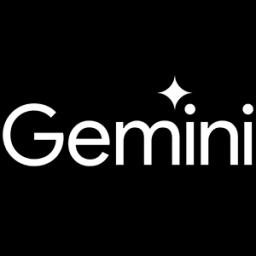
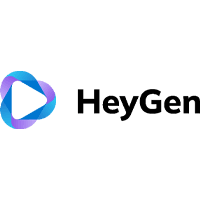
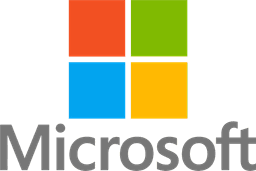
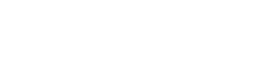
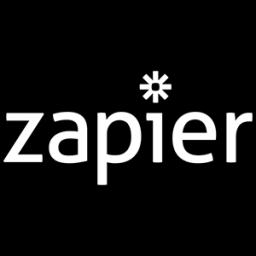
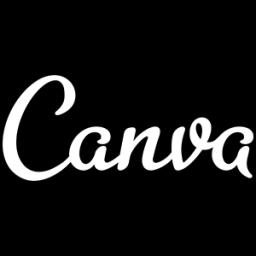
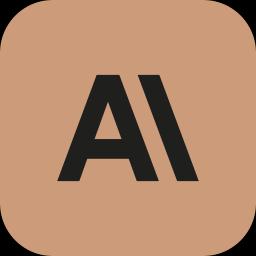
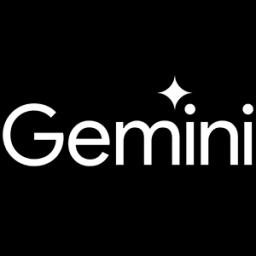
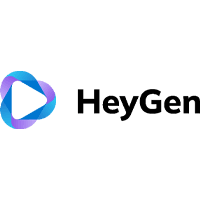
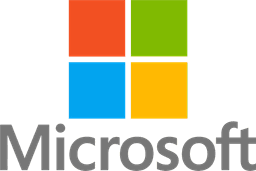
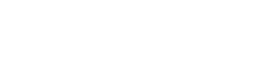
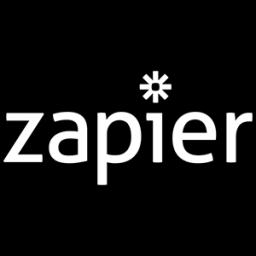
Companies like Cerebras and Groq are stepping up with dedicated AI inference chips, challenging Nvidia's stronghold. These companies are designing chips tailored for executing AI models, which consume less power and provide quicker outputs. This evolution is fueled by the growing demand for AI-driven innovations and applications across sectors ranging from industry to consumer electronics.
The AI chip landscape is rapidly evolving, with significant financial and technological investment driving research and product development. These specialized chips offer enhanced speed, efficiency, and reduced costs, making AI more accessible and practical for diverse applications. As AI becomes integral to more industries, the development of efficient AI chips marks a pivotal shift towards more sustainable and scalable AI solutions.
Understanding AI Training vs. Inference
Artificial Intelligence (AI) Training and AI Inference, while part of the same AI development cycle, serve distinct purposes. Training involves the use of machine learning algorithms to create AI models. This process requires massive amounts of data and computational power to adjust the model's parameters until it can perform specific tasks accurately. GPUs have historically been the go-to hardware for training due to their ability to perform large-scale parallel processing, which is essential for handling the extensive computations involved in training complex AI models.
In contrast, AI Inference is the stage where these trained models are deployed to make predictions on new data. This process requires less computational power compared to training, as it involves applying the learned model weights to input data to generate outputs. Inference is critical in real-world applications where AI models interact with users or external systems, providing real-time insights and decisions. As such, efficiency in inference processing is crucial to ensure rapid responses and lower operational costs.
Learn to use AI like a Pro
Get the latest AI workflows to boost your productivity and business performance, delivered weekly by expert consultants. Enjoy step-by-step guides, weekly Q&A sessions, and full access to our AI workflow archive.
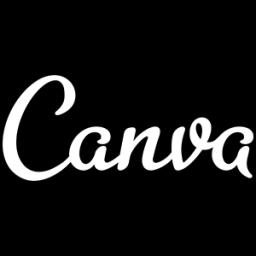
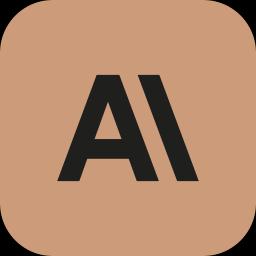
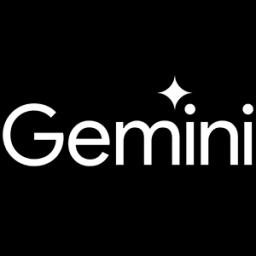
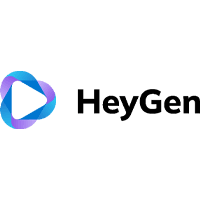
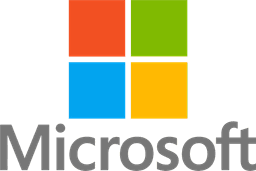
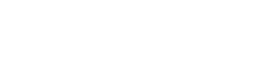
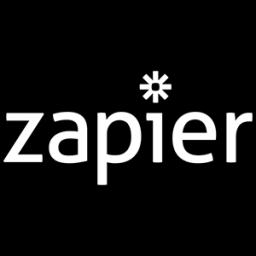
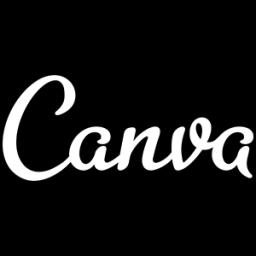
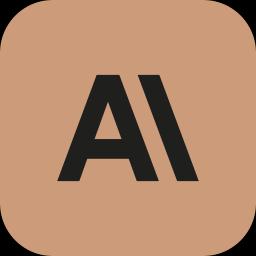
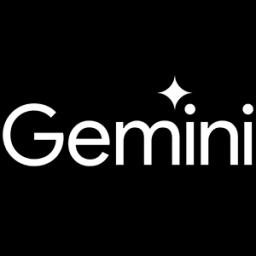
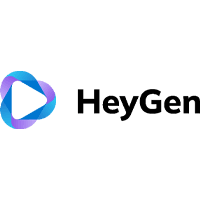
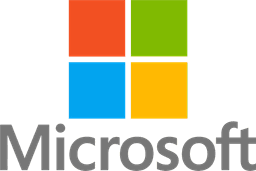
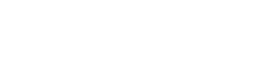
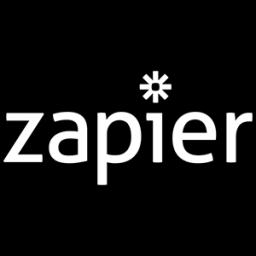
The inefficiency of GPUs in inference tasks arises mainly due to their design, which is optimized for high-throughput data processing rather than handling the lightweight, flexible workloads typical in inference scenarios. This has paved the way for specialized AI inference chips that are engineered to handle such tasks more effectively. These chips can process data with lower latency and reduced energy consumption than conventional GPUs, making them better suited for applications requiring real-time data processing, such as in autonomous vehicles, edge computing, and IoT devices.
Specialized AI inference chips, such as those developed by companies like Cerebras and Groq, offer various benefits. They significantly reduce the operational costs associated with running AI models by optimizing power usage and computational efficiency. Moreover, they support the sustainable growth of AI technologies by lowering energy consumption and minimizing the carbon footprint of AI deployments—critical in an era where climate change is a pressing concern. As a result, these chips enable businesses, particularly those without extensive AI infrastructures, to adopt AI solutions without incurring significant costs.
While the emergence of AI inference chips holds promise for advancing AI technologies economically and sustainably, it also raises various considerations. On the environmental front, the energy savings during AI model execution address key sustainability challenges, but there is also a need to consider the environmental impacts associated with chip manufacturing. Furthermore, as specialized inference chips make AI technologies more accessible across industries, policymakers must address potential job displacement and ethical considerations, such as algorithmic bias, that come with increased automation.
Nvidia's Role in AI Training
Nvidia has long been at the forefront of AI training technologies, heavily relying on its powerful GPUs that have revolutionized large-scale data processing tasks. These GPUs have been instrumental in the training phase of AI model development, which involves processing extensive datasets to enable models to learn and predict accurately. However, as AI technology expands, the tasks following this training, known as inference, are becoming crucial, creating an emerging market for chips designed specifically for this purpose.
The AI landscape is witnessing a shift as companies focus on inference, which involves using trained AI models to make predictions or decisions without the intensive computational load required during training. While Nvidia's GPUs excel in the training process due to their high processing power and parallelism capabilities, they are not optimized for the lighter computational tasks required during inference. This opens opportunities for other players in the industry, such as Cerebras and Groq, which are developing specialized AI inference chips to handle these specific tasks more efficiently.
AI inference chips offer distinctive advantages over traditional GPUs, primarily in terms of energy efficiency and cost-effectiveness. These chips are designed to deliver high performance for inference operations while consuming significantly less power, which not only reduces operational costs but also helps mitigate environmental impact. The promise of substantial cost savings and reduced energy consumption is attracting a wide range of businesses eager to leverage AI technologies without making massive infrastructure investments.
Learn to use AI like a Pro
Get the latest AI workflows to boost your productivity and business performance, delivered weekly by expert consultants. Enjoy step-by-step guides, weekly Q&A sessions, and full access to our AI workflow archive.
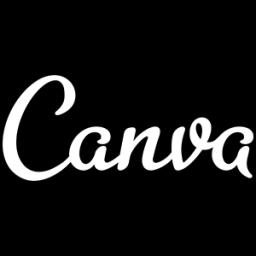
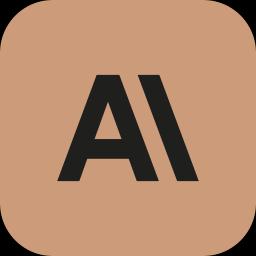
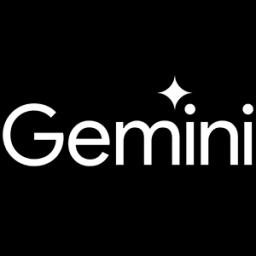
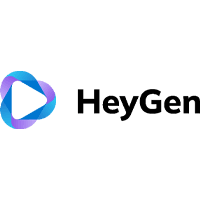
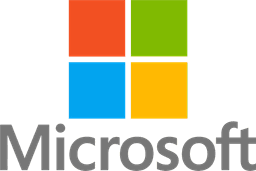
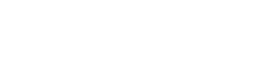
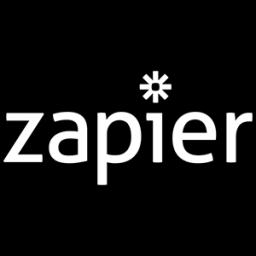
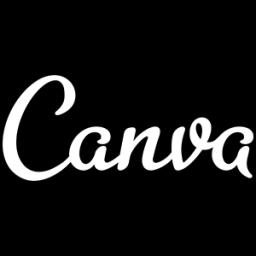
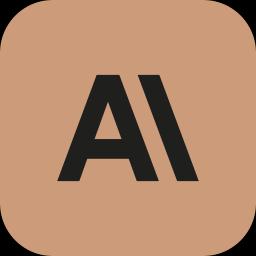
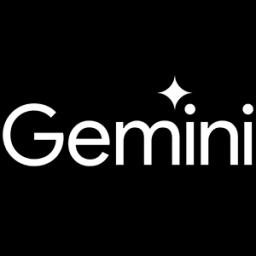
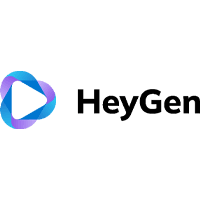
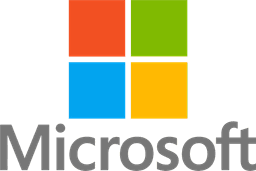
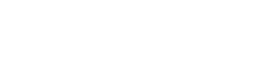
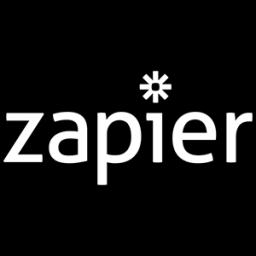
Industries likely to benefit significantly from the advancements in AI inference chips include those deploying AI at scale without an extensive backend setup. Large corporations, particularly those in sectors like technology, finance, healthcare, and consumer electronics, are poised to integrate these chips into their operations to enhance their AI capabilities. Smaller, agile startups might also take advantage of these cost-effective solutions to compete in a tech-driven marketplace.
Environmental considerations play a critical role in the adoption of AI inference chips. As these chips consume less energy compared to traditional GPUs, they contribute to lowering the carbon footprint of data centers and devices that rely on AI for processing. This trend towards more environmentally conscious technology adoption is not only beneficial from a sustainability perspective but also aligns with corporate responsibility goals, as companies seek innovative ways to reduce their environmental impact while enhancing their technological capabilities.
The Rise of AI Inference Chips
AI inference chips are transforming the landscape of artificial intelligence, offering specialized solutions that better suit the needs of businesses aiming to implement AI models within existing infrastructures. Unlike traditional GPUs that excel in training due to their ability to manage massive parallel processing tasks, inference chips are designed to efficiently handle the lighter workloads associated with AI application execution after training. This makes them ideal for real-time AI operations where both speed and energy efficiency are paramount.
Companies such as Cerebras and Groq, alongside tech giants like AMD and Intel, are at the forefront of this innovation, leveraging novel architectures to deliver substantial improvements in speed and energy efficiency over traditional GPU models. These advancements are not only setting new performance benchmarks but are also making AI more accessible to a wider range of applications and industries. By reducing both power consumption and operational costs, AI inference chips are poised to play a critical role in the sustainable expansion of AI technologies.
The societal impact of AI inference chips extends beyond the technology sector, potentially reshaping various industries. As AI models become more economically viable, their integration across different sectors—such as healthcare, where real-time data can significantly enhance patient outcomes, or education, where personalized learning paths could improve student engagement—becomes increasingly feasible. Additionally, this affordability could accelerate AI adoption in consumer electronics, improving device functionality while minimizing environmental impact.
Nevertheless, the development and deployment of these chips face hurdles, including high initial development costs and the maturation of software ecosystems required to support their full potential. The efficiency gains from inference chips come with the challenge of ensuring robust development environments, akin to those provided by Nvidia's CUDA for GPUs, to maximize their adoption. Addressing these challenges is crucial for the sustained growth of AI inference technologies, ensuring they complement their performance benefits with practical utility.
Learn to use AI like a Pro
Get the latest AI workflows to boost your productivity and business performance, delivered weekly by expert consultants. Enjoy step-by-step guides, weekly Q&A sessions, and full access to our AI workflow archive.
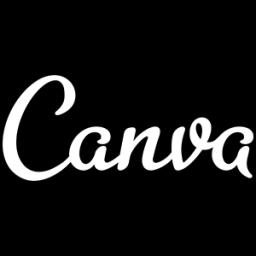
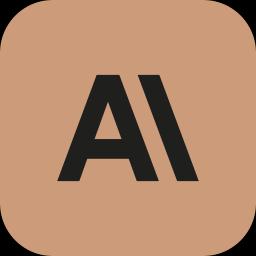
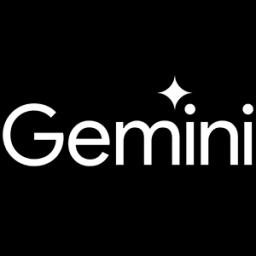
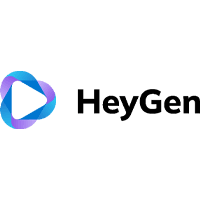
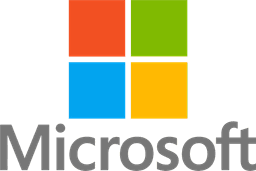
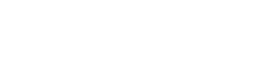
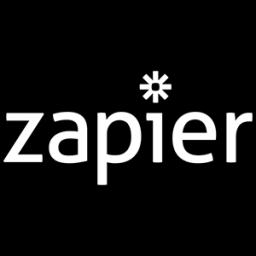
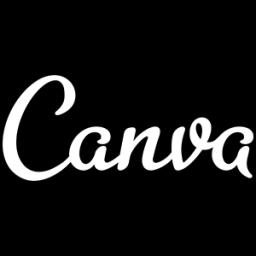
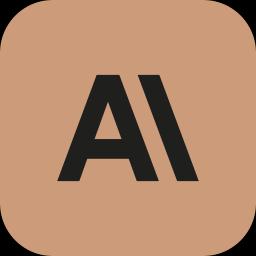
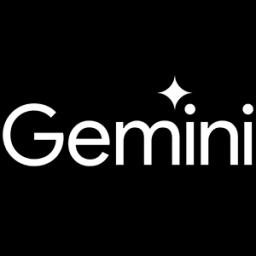
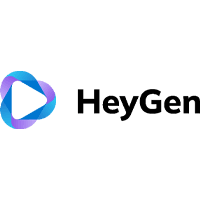
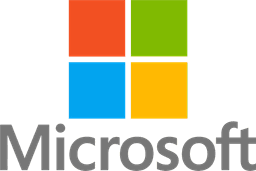
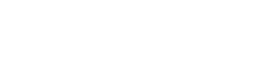
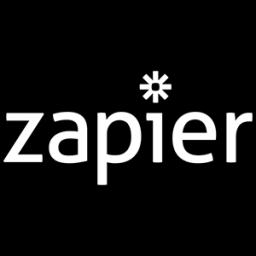
Looking forward, AI inference chips are likely to influence global economic and political landscapes significantly. Economically, they present opportunities for cost savings and increased efficiency that can redefine competitive paradigms in AI-intensive industries. Politically, the emergence of new competitors in AI hardware may influence global alliances and strategic technology partnerships, as countries seek to balance technological prowess with intellectual property rights in the evolving AI ecosystem.
Benefits of AI Inference Chips
In recent years, the development of AI inference chips has become a pivotal advancement in the field of artificial intelligence. These chips are specialized hardware designed to optimize the process of AI inference, which is the execution phase where AI models make predictions or decisions based on new data. Unlike AI training, which requires heavy computational resources to build models, inference involves applying these pre-trained models to perform tasks. Various companies are shifting their focus towards creating AI inference chips that promise substantial benefits over traditional GPUs, primarily used for AI training.
One of the core advantages of AI inference chips is their ability to deliver improved efficiency and cost-effectiveness over GPUs in performing inference tasks. As AI models become more prevalent in different sectors, the need for rapid, energy-efficient, and scalable inference capabilities has increased. These chips are tailored to handle such tasks with greater precision, consuming less power, and consequently lowering operational costs. For businesses, this translates into more feasible AI deployments, especially for those lacking expansive AI infrastructure, offering them a significant edge in maintaining competitiveness.
Companies such as Cerebras and Groq are at the forefront of this innovative trend, developing cutting-edge technologies like the Wafer-Scale Engine chip and the Tensor Streaming Processor. These products highlight the strides being made to enhance speeds and efficiency, with claims of up to 20 times performance improvements in certain applications compared to traditional GPU approaches. Such advancements not only increase performance but also address critical environmental concerns associated with power consumption and carbon emissions of widely used GPUs.
The environmental impact of AI is another compelling reason for the adoption of AI inference chips. As the demand for AI grows, so does the scrutiny over its energy footprint and carbon emissions. Inference chips provide a promising solution by minimizing energy use, thus aligning technological advancement with sustainability goals. They present an opportunity to mitigate the environmental impact of AI in sectors like technology and manufacturing, where large-scale implementations and continuous operation are common.
Looking ahead, the market for AI inference chips is expected to outpace that of training chips, driven by the high demand for efficient inference solutions. With projections estimating market growth to reach over $90 billion by 2030, it's clear that technological, economic, and environmental incentives for AI inference chip adoption are substantial. As investments pour in, these chips promise to unlock new potentials, making AI more accessible and integrated into everyday applications, from consumer electronics to autonomous technologies.
Learn to use AI like a Pro
Get the latest AI workflows to boost your productivity and business performance, delivered weekly by expert consultants. Enjoy step-by-step guides, weekly Q&A sessions, and full access to our AI workflow archive.
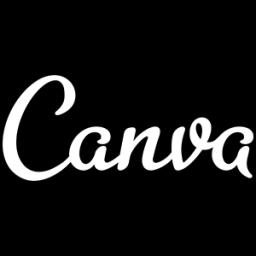
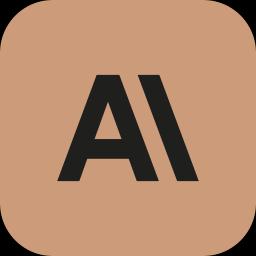
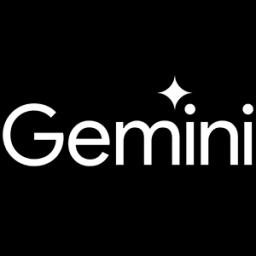
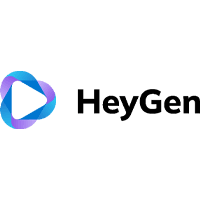
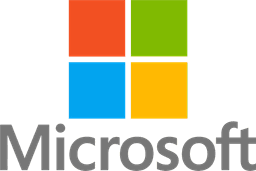
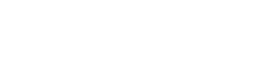
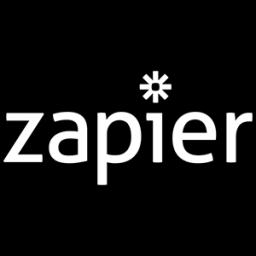
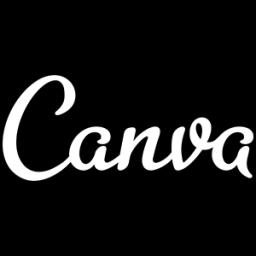
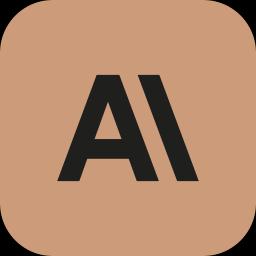
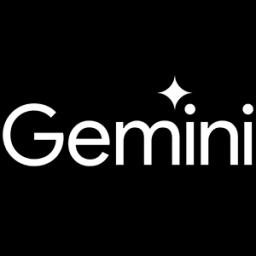
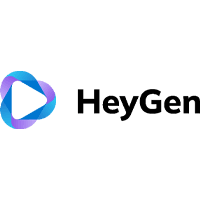
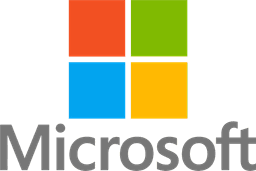
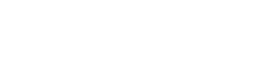
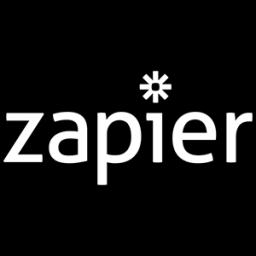
Leading Players in the AI Inference Market
In the rapidly evolving AI inference market, several leading players are emerging as challengers to Nvidia's dominance. These companies are developing distinct and innovative chip technologies that promise to enhance AI model execution post-training. Among the frontrunners are Cerebras and Groq, along with industry stalwarts like AMD and Intel. Their focus is on creating specialized AI inference chips that optimize tasks with greater efficiency than traditional GPUs, which are primarily designed for AI training.
Cerebras has gained particular attention with its groundbreaking Wafer-Scale Engine (WSE) chip technology, which offers exceptional speed and energy efficiency. Groq, another key player, has developed the Tensor Streaming Processor, known for its ability to provide significant performance improvements, reportedly up to 20 times faster in certain scenarios than Nvidia's offerings. These advancements underscore the growing competition within the AI inference chip space, as these companies strive to deliver solutions that not only meet but exceed Nvidia's capabilities in specific applications.
The strategic move by these companies to focus on AI inference reflects a broader opportunity in the market: the potential for inference chips to surpass training chips in terms of demand and importance. The inference aspect of AI, crucial for deploying AI models in real-time applications, demands chips that are cost-effective, energy-efficient, and capable of delivering low-latency performance. This shift could drive substantial growth in the market, with projections estimating its value to reach $90.6 billion by 2030.
This burgeoning market also sees significant investments fueling innovation in AI chip technologies. For instance, Groq's successful securing of $640 million highlights the financial backing these emerging players are receiving. Such investments not only bolster rapid development in AI hardware but also enhance prospects for increased accessibility and cost reductions, allowing a wider range of industries to adopt AI more feasibly.
In addition to cost benefits, these specialized inference chips promise substantial environmental advantages. By lowering energy consumption and reducing the carbon footprint of AI operations, they address growing concerns over the environmental impact associated with AI's expanding footprint. This ecological appeal adds a compelling dimension to their adoption, especially among businesses prioritizing sustainability in their operations.
Technological Advancements in AI Chips
Artificial Intelligence (AI) is transforming industries globally, offering unprecedented capabilities to businesses and consumers alike. Central to this transformation is the rapid advancement in AI chips, especially those designed for AI inference tasks. While AI model training has been heavily reliant on Graphic Processing Units (GPUs), like those developed by Nvidia, the industry is witnessing a shift towards alternative chip solutions that promise greater efficiency and cost-effectiveness for AI inference.
Learn to use AI like a Pro
Get the latest AI workflows to boost your productivity and business performance, delivered weekly by expert consultants. Enjoy step-by-step guides, weekly Q&A sessions, and full access to our AI workflow archive.
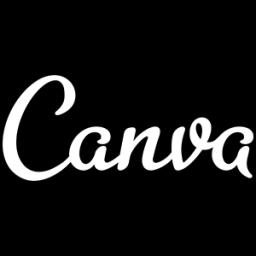
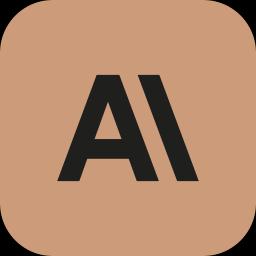
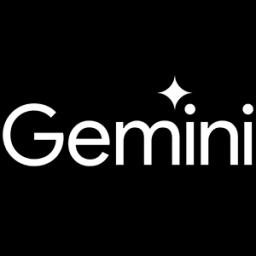
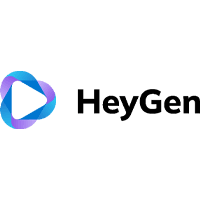
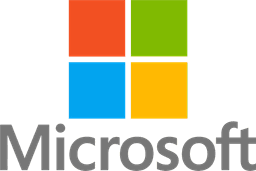
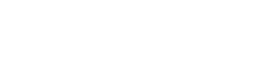
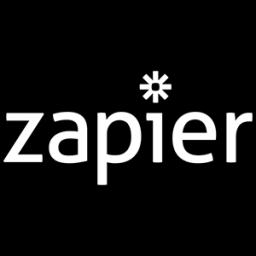
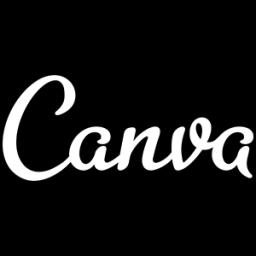
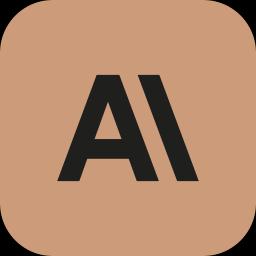
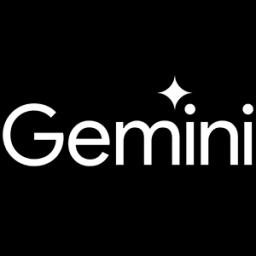
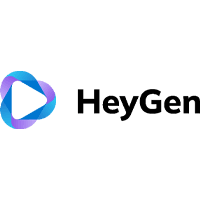
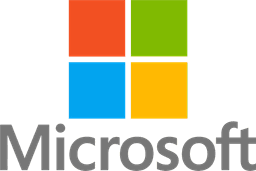
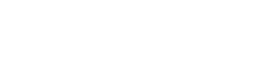
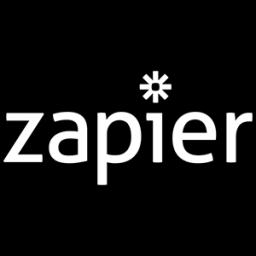
AI inference refers to using trained models to make predictions or decisions based on new data inputs. This aspect of AI applications has seen growing demand as businesses seek to incorporate AI into real-time decision-making processes without the need for extensive computational infrastructure. In response, tech companies such as Cerebras, Groq, and traditional chip manufacturers like AMD and Intel are pioneering the development of specialized AI inference chips. These chips are engineered to optimize AI operations post-training, offering improvements in speed, power efficiency, and reduced operational costs.
At the forefront of this technological evolution is the push to overcome limitations associated with GPUs in AI inference applications. Although GPUs excel at handling the massive parallel computations required during model training, their efficiency wanes in inference scenarios that demand lower latency and power consumption. Specialized AI chips, however, are tailored to excel in these areas, making them an attractive alternative for businesses aiming to deploy AI solutions more sustainably and cost-effectively.
The implications of advancing AI inference chip technology go beyond just computational efficiency. As companies embrace these new chips, the environmental impact associated with AI deployments is also expected to decrease. By lowering energy consumption and carbon emissions, AI inference chips are not only revolutionizing business models but also contributing positively towards sustainability goals. This aspect is particularly crucial at a time when enterprises are under increasing pressure to reduce their environmental footprint amidst rising concerns about climate change.
As the AI chip market continues to evolve, public and industry reactions have been varied, reflecting a mix of enthusiasm and caution. On one hand, the potential for reduced costs and improved energy efficiency offered by these chips is warmly welcomed across sectors. On the other hand, issues such as the maturity of the software ecosystem supporting these chips and broader societal implications like job displacement and ethical concerns remain areas of active discussion. Balancing these aspects will be essential as the market for AI inference chips matures and expands globally.
Market Growth and Investment in AI Technology
The rapid development and investment in Artificial Intelligence (AI) technology, particularly in the area of inference chips, is reshaping the technological landscape. The market is witnessing acceleration as companies like Nvidia, alongside its rising competitors such as Cerebras, Groq, AMD, and Intel, race to develop chips that address the specific demands of AI inference. AI inference, differentiated from AI training, involves deploying trained models to make predictions, and necessitates chips that are efficient, cost-effective, and energy-saving. This shift is fueling substantial market growth, with projections estimating the AI inference market to reach $90.6 billion by 2030.
At the core of this evolution lies the need for specialized chips that optimize AI inference tasks, moving beyond the capabilities of traditional GPUs that, while powerful, are not ideally suited for these operations. Companies like Cerebras are making significant strides with their Wafer-Scale Engine chips, which promise up to 20x performance improvements and greater energy efficiency. The focus is on reducing operational costs and energy consumption, aiming for a sustainable approach to AI technology implementation, which aligns with growing environmental concerns.
Learn to use AI like a Pro
Get the latest AI workflows to boost your productivity and business performance, delivered weekly by expert consultants. Enjoy step-by-step guides, weekly Q&A sessions, and full access to our AI workflow archive.
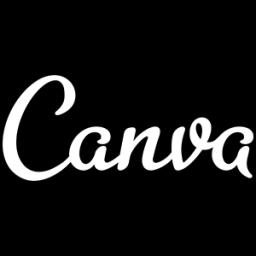
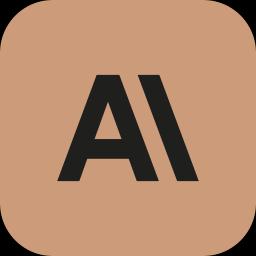
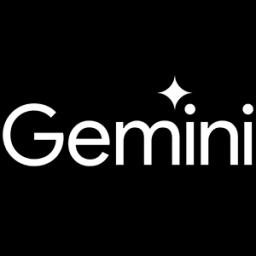
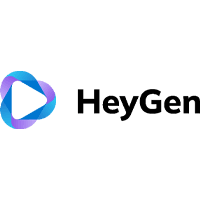
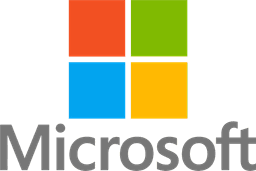
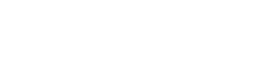
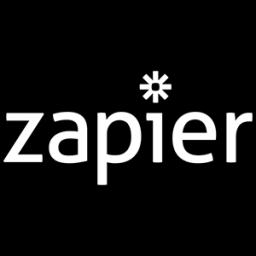
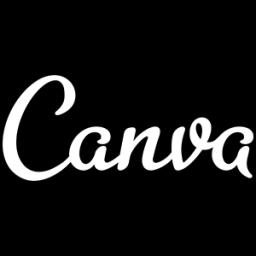
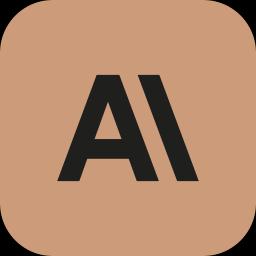
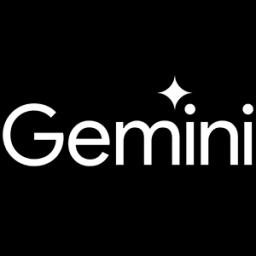
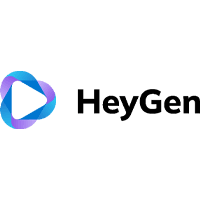
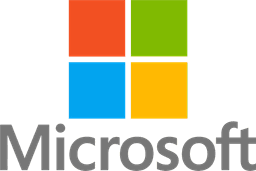
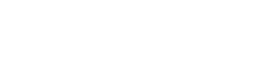
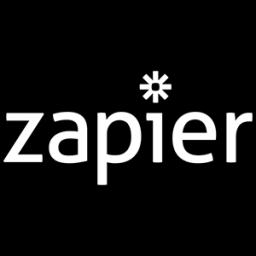
The investment landscape mirrors this technological evolution, with substantial funding directed towards AI chip development. Groq's successful $640 million funding round exemplifies the market's readiness to support innovations that can offer better performance at reduced costs. These developments are not just about maintaining competitive edges but are also about democratizing AI usage, making advanced AI tools accessible to smaller businesses without substantial infrastructure or resource investments.
Experts in technology and energy highlight the dual potential of these chips, not only to surpass the AI training market in growth but also to alleviate environmental issues associated with high-power GPU usage in AI inference tasks. The reduction in energy consumption offered by these new chips presents an opportunity to tackle the carbon footprint of AI technologies, aligning technological progression with sustainability goals.
Despite the optimistic projections, there are challenges, particularly regarding the software ecosystems that support these chips. The established dominance of Nvidia's CUDA platform provides a comprehensive set of tools and community support, which newer chipmakers must strive to match. Public reactions in social media have also shown a mix of enthusiasm and caution, with discussions often centering on the readiness of these chips for commercial application.
Looking to the future, the production of AI-specific inference chips could diversify the AI technology market, leading to more competitive pricing and innovation. Social implications include increased access to AI technology across various sectors which might enhance service delivery in healthcare, education, and more. However, this would also necessitate addressing concerns such as job displacement and ensuring ethical AI deployment. Politically, the market diversification could redefine global technology leadership dynamics, with implications for international cooperation and regulatory frameworks.
Cost Implications and Accessibility of AI Chips
The advancement in AI technology has led to the increased focus on developing inference chips, driven by the cost implications and accessibility they offer. As Nvidia's dominance in AI training chips confronts new challenges, competitors are focusing on building more energy-efficient and cost-effective inference chips. Companies like Cerebras, Groq, AMD, and Intel are spearheading this movement, aiming to optimize AI models' execution, which could significantly lower computing expenses and make AI adoption more feasible for a broad array of businesses.
Inference chips represent a turning point in making AI more accessible. By reducing operational costs through enhanced computing efficiency, these chips open up opportunities for businesses that may not have been able to afford high-powered AI solutions in the past. The shift from training to inference chips could lead to considerable energy savings, crucial for firms looking to implement AI solutions sustainably.
Learn to use AI like a Pro
Get the latest AI workflows to boost your productivity and business performance, delivered weekly by expert consultants. Enjoy step-by-step guides, weekly Q&A sessions, and full access to our AI workflow archive.
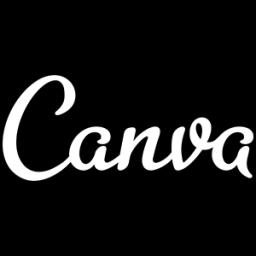
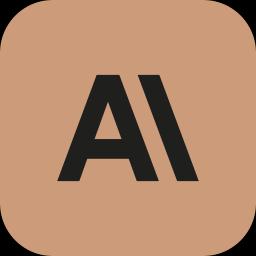
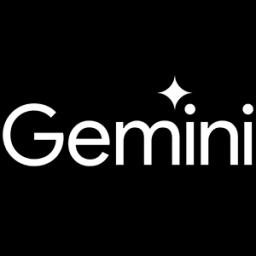
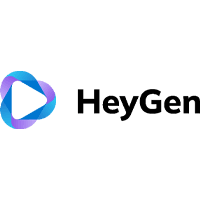
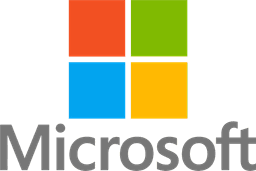
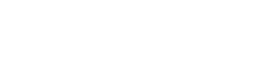
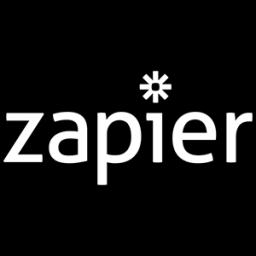
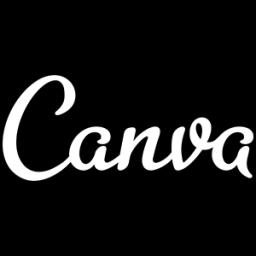
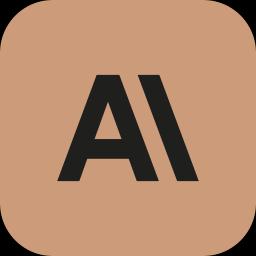
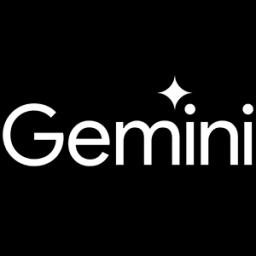
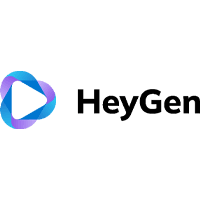
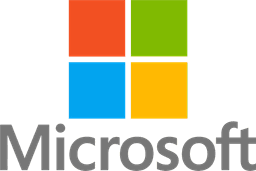
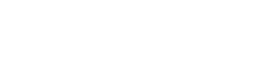
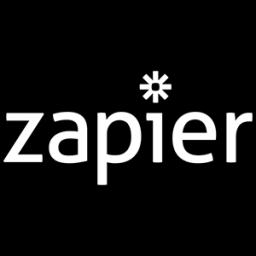
Significant market growth is anticipated in the AI inference sector, potentially overtaking the training chip market. Companies like Groq and Cerebras are not only innovating technologically but also attracting substantial investments crucial for their growth and expansion. As of 2030 projections, the AI inference chip market size is expected to reach $90.6 billion, driven by the demand for more efficient AI processing methods that promise improved speed and reduced costs.
Experts acknowledge that as AI technology evolves, the market for inference chips will likely outpace the training chip market. This shift is prompted by the need for more efficient, low-power-consumption solutions tailored for real-time processing. Such advancements could translate to lower expenses for companies while maintaining high-performance metrics, making them an attractive option for widespread corporate adoption. Moreover, as these chips consume less energy, they address environmental concerns that come with the extensive use of AI technologies.
The public is largely optimistic about the introduction of AI inference chips, recognizing their potential to make AI technologies more affordable and sustainable. However, this optimism is tempered by concerns about the nascent software ecosystems needed to support these chips, as well as broader societal issues like employment implications and algorithmic bias. These redundant factors indicate a cautious but hopeful perspective on the future of AI hardware diversification.
Looking ahead, the rise of specialized inference chips suggests a diverse future for AI, marked by increased economic implications and technological advancements. Economically, these chips could drive down the cost of AI deployment, fostering competitive markets and encouraging technological innovations. Socially, it could widen the adoption of AI across sectors such as healthcare and education, although it might raise concerns about job displacements and necessitate policy considerations to handle such transitions effectively. Politically, new players in the market might alter alliances regarding technological advancements, possibly encouraging more regional partnerships in AI development. As risks like algorithmic bias and data privacy persist, regulatory frameworks will need to adapt, ensuring equitable and secure deployment of AI technologies worldwide.
Environmental Impact of AI Inference Chips
Artificial intelligence (AI) inference chips represent a significant advancement in computing technology designed to execute AI models, commonly referred to as inference. Distinct from AI training, which involves teaching a model using large datasets, inference applies these trained models to new data for prediction purposes. This distinction is crucial as it underscores why different hardware solutions are needed for AI training and inference. While Graphics Processing Units (GPUs), particularly those developed by Nvidia, excel in handling the complex computations required during the training phase, they are not as cost-effective or efficient for inference tasks, leading to the emergence of specialized AI inference chips.
The rise of AI inference chips has the potential to dramatically impact the environmental landscape by reducing the energy consumption associated with AI tasks. By nature, these chips are engineered for efficiency, providing the same levels of computational power with far less energy usage than traditional GPUs. This efficiency not only holds the promise of decreased operational costs for companies deploying AI technologies but also stands to significantly cut down on the carbon emissions associated with high-density computational tasks. Companies developing these chips, such as Cerebras and Groq, are keenly focused on producing hardware that can sustain AI advancements while aligning with growing environmental sustainability goals.
Learn to use AI like a Pro
Get the latest AI workflows to boost your productivity and business performance, delivered weekly by expert consultants. Enjoy step-by-step guides, weekly Q&A sessions, and full access to our AI workflow archive.
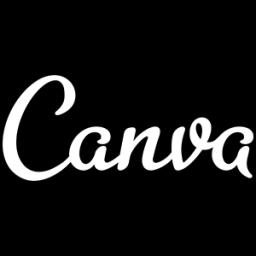
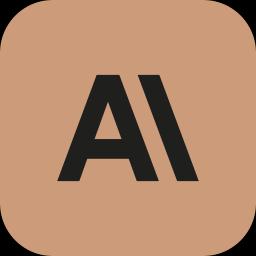
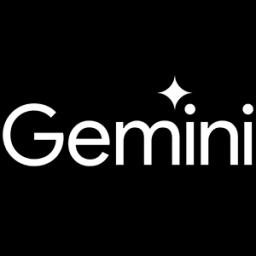
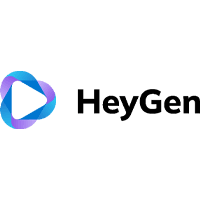
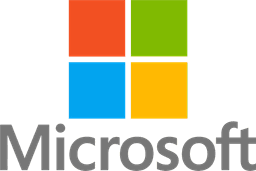
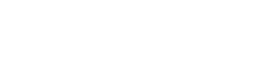
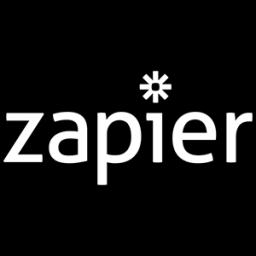
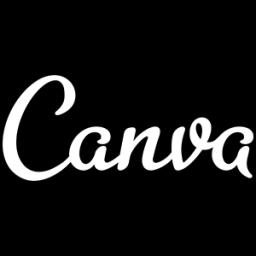
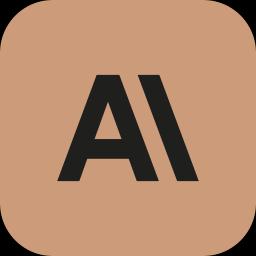
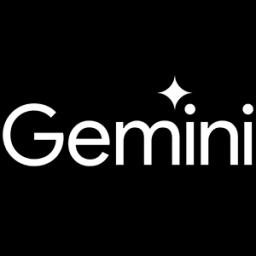
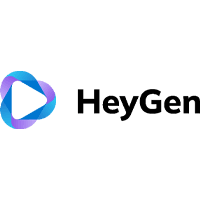
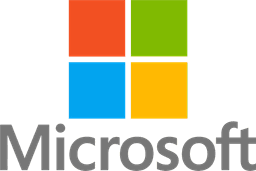
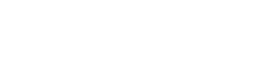
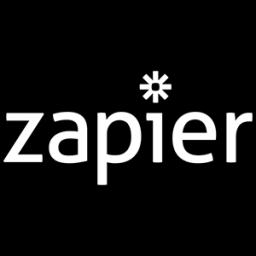
Market analysts and experts are closely watching the AI inference chip space, predicting it could eventually surpass the market for AI training chips due to increasing demand. This transition is fueled by the continual growth of AI applications and the financial and ecological benefits of using power-efficient hardware for inference. The push towards these advanced chips is supported by significant venture capital investments, with companies like Groq and Cerebras spearheading innovation in this area. As the technology evolves, these chips promise not only heightened performance but also to make AI technologies more accessible and affordable for a wider array of businesses, enhancing their appeal across industries.
The environmental implications of AI inference chips extend beyond their operational efficiency. The manufacturing processes for these chips are being scrutinized to ensure they align with sustainable practices, as the high energy costs and potential waste products from chip production could offset some ecological benefits. Experts advise that the development of these chips should carefully balance innovation with environmental responsibility. This notion is reinforced by energy experts who advocate for the inclusion of sustainability metrics in the design and production phases of these chips. As AI continues to integrate into more facets of daily life, there is a pressing need to mitigate any potential negative environmental impacts from associated hardware advancements.
Expert Insights on AI Chip Development
Nvidia's dominance in the AI hardware space is being challenged by new competitors focusing on AI inference chip technology. While Nvidia GPUs are highly efficient for training AI models, allowing them to handle complex calculations and vast data sets, they are not optimized for inference tasks. Inference involves deploying trained AI models to make predictions or decisions based on new data. This task can often require less computational power but demands high efficiency and speed; a space where Nvidia’s rivals are stepping in.
Companies like Cerebras, Groq, and traditional chipmakers such as AMD and Intel are developing specialized AI inference chips that provide better performance for inference by being more energy-efficient and cost-effective. These new chips can potentially reduce the operational costs of running AI models, making it feasible for more businesses to incorporate AI technologies. In particular, AI inference chips offer significant benefits such as reduced energy consumption and improved processing speed, thereby supporting environmental sustainability and economic accessibility.
One of the main driving forces behind the development of AI inference chips is the projected growth of the inference chip market. It is expected to surpass the training chip market, reaching an estimated value of $90.6 billion by 2030. This growth is fueled by a rising demand for AI applications across various industries and substantial investments in technological advancements. Emerging markets and improvements in chip technologies indicate a robust expansion trajectory for this segment.
Despite Nvidia's current leadership in the AI hardware industry, the focus has shifted to deliver more targeted, efficient solutions specifically for inference tasks. Startups like Cerebras emphasize creating cutting-edge technology, such as the Wafer-Scale Engine chip, designed to excel in speed, efficiency, and performance. Groq's Tensor Streaming Processor offers up to 20x performance improvements over traditional GPU setups for certain tasks, showcasing the potential of these new chips to revolutionize the landscape.
Learn to use AI like a Pro
Get the latest AI workflows to boost your productivity and business performance, delivered weekly by expert consultants. Enjoy step-by-step guides, weekly Q&A sessions, and full access to our AI workflow archive.
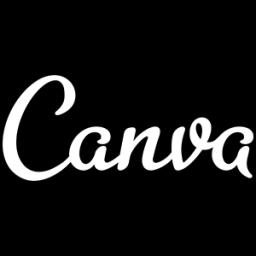
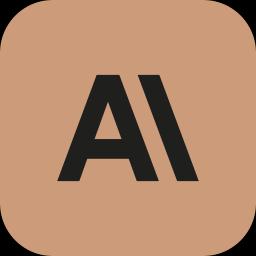
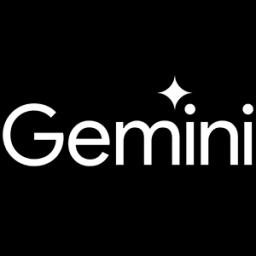
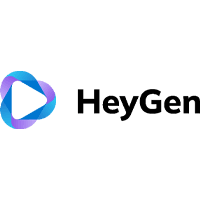
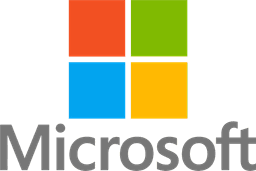
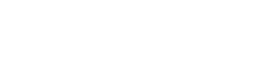
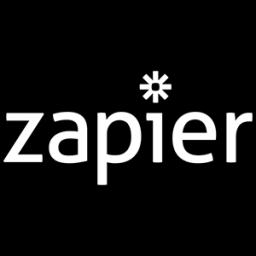
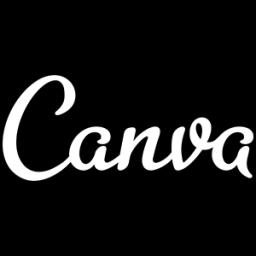
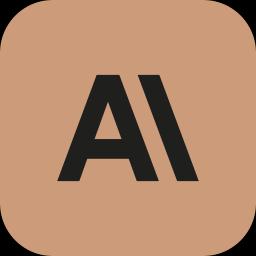
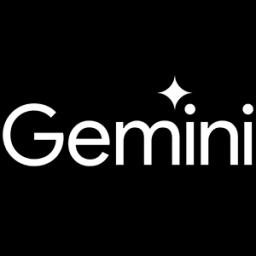
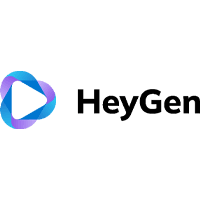
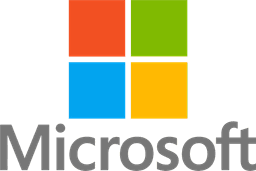
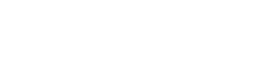
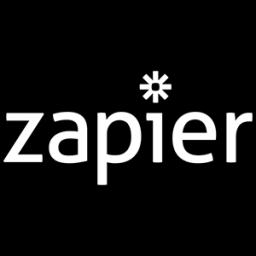
However, the adoption of alternative AI inference chips faces several challenges. While they offer ecological benefits by lowering energy consumption, the high initial development costs can pose significant financial barriers. Additionally, the relative novelty of these chips means software ecosystems and development tools are less mature compared to those built for Nvidia's CUDA platform, potentially slowing down widespread integration and adoption in the market.
Public Reactions to AI Inference Chips
Nvidia's dominance in AI training chips is being challenged by emerging competitors focusing on AI inference chips, as highlighted in a recent article from Star Tribune. These new players, including Cerebras and Groq, along with industry giants AMD and Intel, are developing chips specifically designed for AI inference processes. This shift is due to the realization that while Nvidia GPUs excel in training AI models through extensive data processing, they are less efficient when it comes to inference tasks. The new AI inference chips being developed promise to address this inefficiency by offering solutions that lower computing costs and reduce energy consumption, thereby making AI more accessible and sustainable for a wide array of businesses.
The distinction between AI training and AI inference is critical in understanding the market dynamics. Training involves exposing an AI model to large volumes of data to refine its parameters, making it computationally intense and well-suited to Nvidia's high-performance GPUs. In contrast, inference involves applying the trained model to new, unseen data, which requires lower computational power and benefits more from the tailored capabilities of inference chips. These chips are crafted to enhance efficiency and minimize costs, catering especially to businesses that require robust AI capabilities without investing heavily in computing infrastructure.
As the inference chip market evolves, it presents a significant economic and environmental opportunity. Expert opinions suggest that this market may eventually outgrow the training chip sector due to a higher demand for energy-efficient and low-cost AI inference solutions. The development trajectory spearheaded by Cerebras, Groq, and others includes breakthroughs like the WSE-3 chip and its photonic interconnects, which represent a paradigm shift in chip technology. This innovation drives the performance and efficiency of inference tasks, signifying a vital leap forward as businesses seek greener and more cost-effective AI solutions.
Public reactions to AI inference chips are mixed. While there is optimism about the lowered operational costs and energy savings these chips offer compared to traditional GPUs, there is skepticism about their maturity and readiness for widespread adoption. The software ecosystem for these new chips is still developing, which may slow down their integration compared to established Nvidia platforms. Additionally, societal implications, such as employment shifts and ethical considerations due to increased automation, are also sparking debates as these technologies advance.
The emergence of AI inference chips not only reshapes technological landscapes but also carries broader implications. Economically, this could democratize AI usage across varied sectors, from healthcare to consumer electronics, by lowering barriers to entry. Politically, it can redefine international alliances as countries seek to bolster their AI capabilities through strategic investments in chip technology and manufacturing. However, the rapid pace of innovation calls for robust regulatory frameworks to manage potential issues concerning data privacy and algorithmic accountability.
Learn to use AI like a Pro
Get the latest AI workflows to boost your productivity and business performance, delivered weekly by expert consultants. Enjoy step-by-step guides, weekly Q&A sessions, and full access to our AI workflow archive.
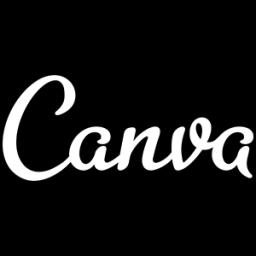
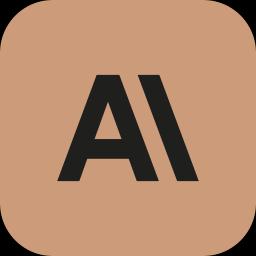
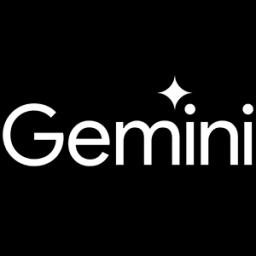
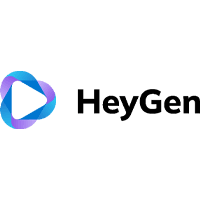
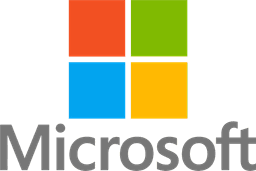
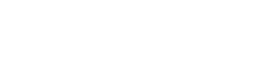
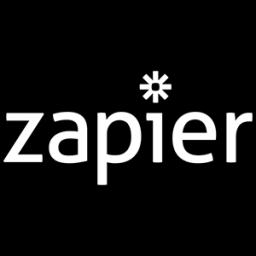
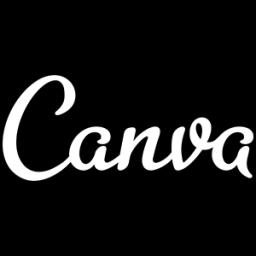
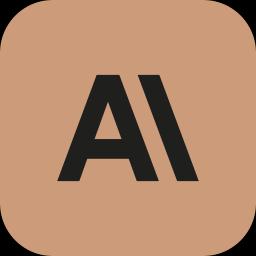
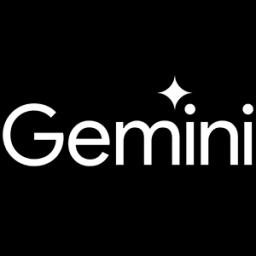
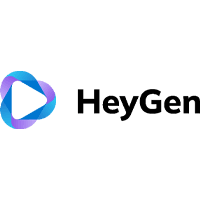
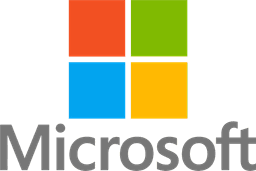
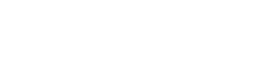
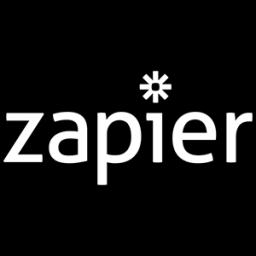
Future Implications of Specialized AI Chips
The advent of specialized AI inference chips marks a transformative shift in the realm of artificial intelligence and computing. These chips, designed specifically for the inference stage—the process of executing AI models—promise not only enhanced performance but also economic and environmental benefits. As the demand for AI-driven solutions grows, the efficiency and scalability of computing resources become critical. Traditional GPUs, like those manufactured by Nvidia, excel at the computationally intensive training phase but lag in inference efficiency, creating opportunities for specialized processors.
Companies like Cerebras, AMD, and Intel, along with emerging players like Groq, are at the forefront of this innovation. They are crafting chips optimized for inference tasks, which promise reduced energy consumption and operational costs. This shift is particularly meaningful as AI applications permeate various sectors, necessitating affordable and sustainable computing solutions. By lowering the barriers to AI implementation, these inference chips can facilitate broader adoption across industries, from healthcare and education to consumer electronics and edge devices.
Environmentally, the introduction of these inference chips could significantly curb the carbon footprint associated with AI operations. By enhancing energy efficiency, these chips not only lower operational costs but also align with global sustainability goals. This is increasingly important as organizations and governments worldwide prioritize eco-friendly initiatives. The potential environmental benefits extend beyond mere energy savings, potentially influencing corporate practices and policy-making to favor greener technologies.
Economically, the diversification of the AI chip market heralds a competitive landscape that could disrupt Nvidia's current dominance. As companies race to innovate, the resulting competition is likely to reduce costs and spur further technological advancements. This competitive dynamic could democratize AI technology, making it more accessible to small and medium-sized enterprises that lacked the resources to leverage AI effectively. The economic ripple effects are profound, potentially altering market dynamics and business strategies across multiple sectors.
Socially, the widespread availability of AI inference chips could accelerate the integration of AI into daily life, from smart home devices to personalized healthcare solutions. This ubiquity, however, raises questions about privacy, data protection, and the ethical use of AI technologies. As these chips empower more applications, their impact on employment and society at large could be significant, prompting calls for thoughtful policy interventions to mitigate potential disruptions.
Politically, the rise of AI chip alternatives to Nvidia could have geopolitical ramifications. Nations with burgeoning tech industries might shift focus toward chip manufacturing, influencing global alliances and trade policies. These developments could shape strategic collaborations and negotiations across the technology spectrum, influencing both economic policies and security considerations. The need for robust international frameworks to manage these shifts becomes apparent as AI continues to redefine local and global landscapes.
Learn to use AI like a Pro
Get the latest AI workflows to boost your productivity and business performance, delivered weekly by expert consultants. Enjoy step-by-step guides, weekly Q&A sessions, and full access to our AI workflow archive.
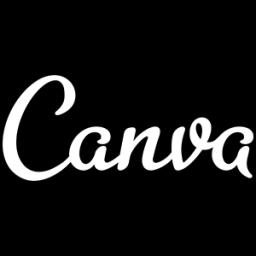
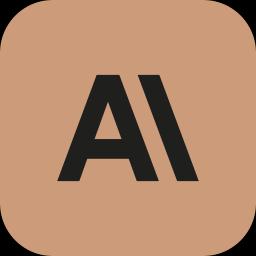
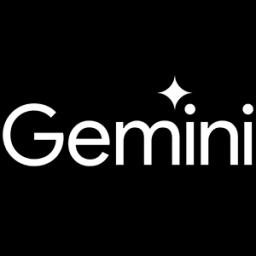
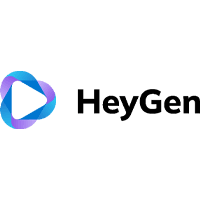
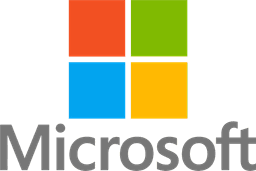
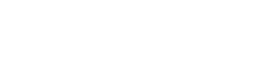
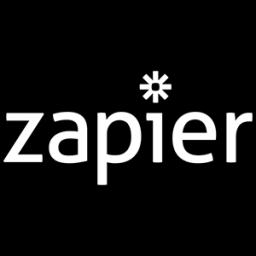
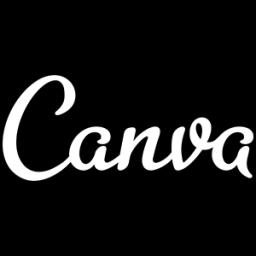
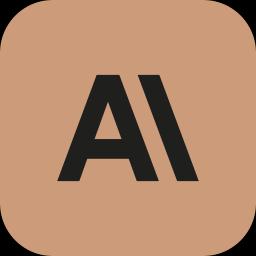
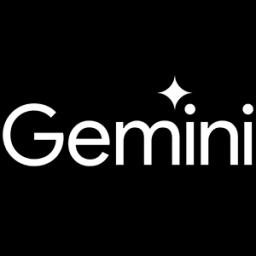
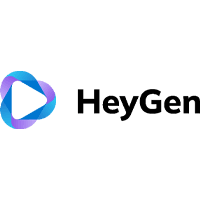
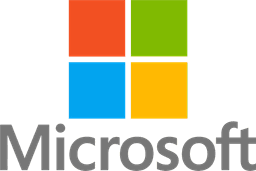
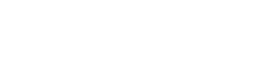
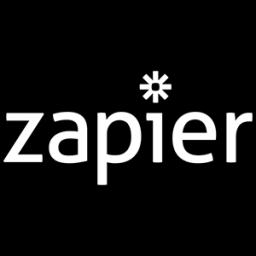