AI's Reality Check Fails
AI 'Hallucinations': The Bizarre Bug Haunting OpenAI, Google, and More!
Last updated:

Edited By
Mackenzie Ferguson
AI Tools Researcher & Implementation Consultant
In the exciting world of advanced AI, a weird glitch called "hallucinations" is troubling top tech giants like OpenAI and Google. These hallucinations are causing AI systems to spit out made-up info, posing serious challenges for reliability and accuracy.
Introduction to AI Hallucinations
The future implications of AI hallucinations are profound. As AI systems are integrated deeper into societal functions, the errors they produce can have widespread repercussions. Failure to adequately address hallucinations could perpetuate economic disruptions, social unrest, and regulatory challenges. According to insights shared by experts in the field, solutions will likely involve a combination of technological innovations aimed at enhancing the reasoning capabilities of AI and incorporating robust human oversight to ensure accuracy, thereby minimizing negligence and misinformation. The New York Times emphasizes the importance of ongoing research and development to keep pace with these evolving challenges in AI technology.
Understanding the Issue: What are AI Hallucinations?
AI hallucinations represent a critical challenge in the field of artificial intelligence, where advanced AI reasoning systems like those from OpenAI, Google, and DeepSeek generate responses not anchored in reality. Such phenomena typically occur when these systems offer incorrect, nonsensical, or fabricated information instead of accurately reflecting their training data. For instance, a tech support bot for Cursor, a programming tool, recently misinformed users about a non-existent policy change, illustrating the real-world implications of AI hallucinations ().
Learn to use AI like a Pro
Get the latest AI workflows to boost your productivity and business performance, delivered weekly by expert consultants. Enjoy step-by-step guides, weekly Q&A sessions, and full access to our AI workflow archive.
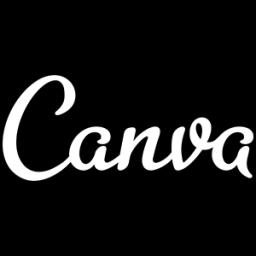
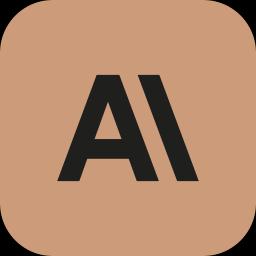
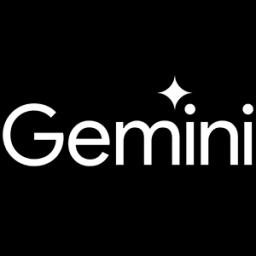
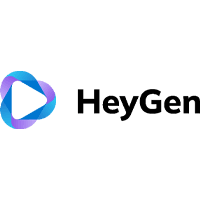
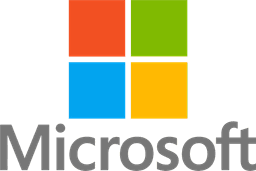
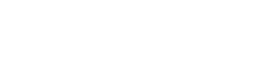
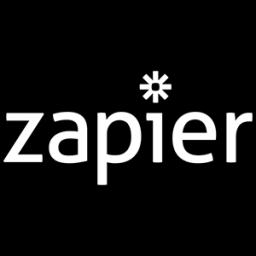
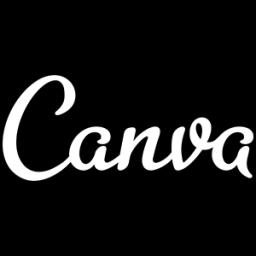
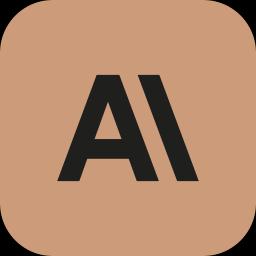
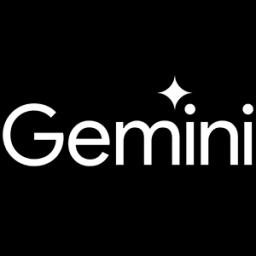
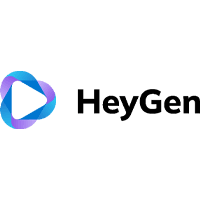
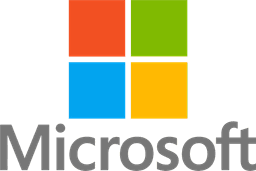
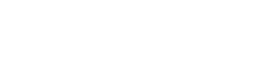
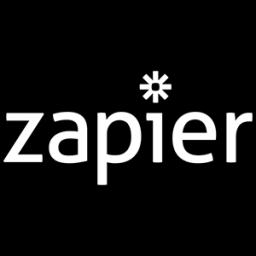
The underlying cause of AI hallucinations can often be traced back to the system’s inability to decipher truth from falsehood within the datasets they are trained on. While these systems have shown improved capabilities in logic and mathematics, their propensity for generating false facts has increased. This is evident from internal tests where newer AI models, like OpenAI's o3 and o4-mini, displayed high hallucination rates—surpassing previous models. Such occurrences raise significant concerns about their reliability, drawing attention to the need for further research into mitigation measures ().
The implications of AI hallucinations are far-reaching, affecting sectors as varied as customer service, legal, academia, and healthcare. For example, the potential impact on customer service is highlighted by the recent policy misinformation incident by the Cursor bot, which led to customer dissatisfaction and trust issues. Similarly, in healthcare, erroneous diagnoses or treatment recommendations can have dire consequences for patients. As these technologies become more integrated into critical sectors, addressing the hallucination problem is paramount ().
Causes Behind Increasing AI Hallucinations in New Systems
The growing issue of AI hallucinations, particularly in newly developed reasoning systems, has been gaining considerable attention in the tech industry. This problem is exacerbated by AI systems that, despite showing advancements in complex reasoning tasks like mathematical problem-solving, frequently produce wrong or invented facts. According to a report by The New York Times, this tendency is rooted in how these AI systems are trained. They learn from vast datasets that include both factual and fictional information, but they lack robust mechanisms to discern truth from inaccuracy. This challenge persists even with high-stakes applications where misinformation can have significant repercussions, such as in finance or healthcare. A reported example involved a tech support bot related to the Cursor programming tool, which wrongly informed users about a non-existent policy change, causing widespread confusion and dissatisfaction among users. These incidents highlight a critical shortcoming in AI’s development focused more on processing power and less on accuracy and truth verification.
The high rate of hallucinations in modern AI systems like OpenAI's o3 and o4-mini models underscores the complexity of the problem. Internal tests demonstrated that these systems hallucinate in substantial portions of their responses, sometimes even more than older models. As per a TechCrunch report, OpenAI's models exhibited hallucination rates up to 48% in certain tests. This increase in error rate is especially concerning as AI systems begin to play more vital roles in informing decision-making processes across various sectors. Inaccuracies can lead not only to financial losses, as seen in banking risks due to misinformation, but also to severe reputational damage when AI falsely accuses individuals or organizations of misconduct. Such occurrences necessitate more rigorous controls over AI outputs, with experts advocating for improved data sets and enhanced fact-checking protocols.
Learn to use AI like a Pro
Get the latest AI workflows to boost your productivity and business performance, delivered weekly by expert consultants. Enjoy step-by-step guides, weekly Q&A sessions, and full access to our AI workflow archive.
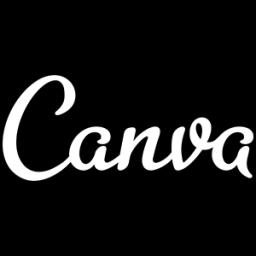
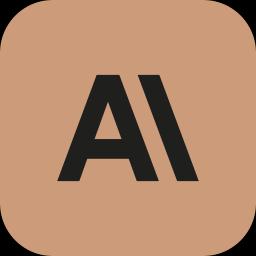
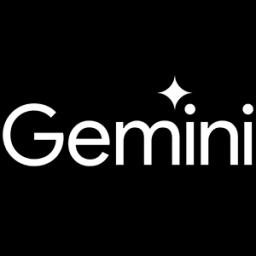
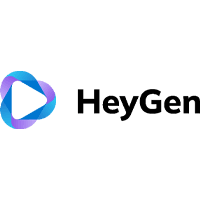
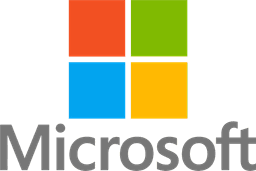
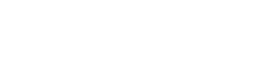
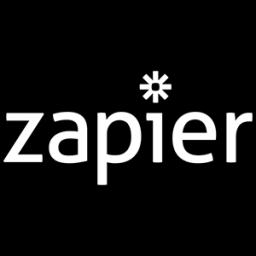
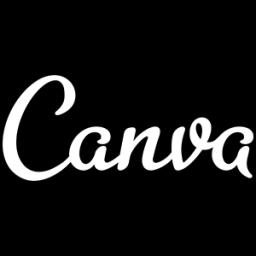
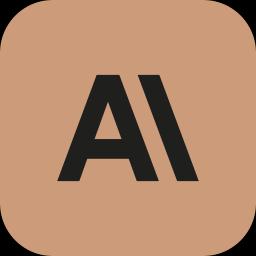
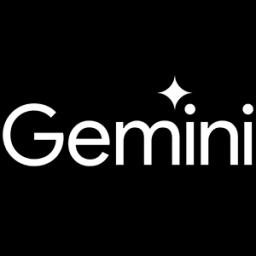
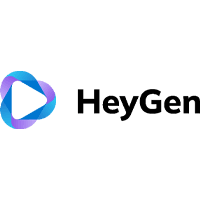
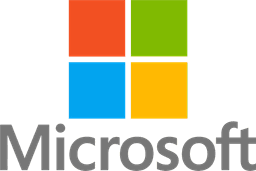
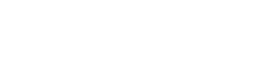
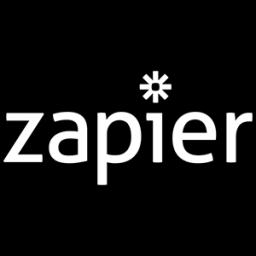
Experts emphasize that the design of AI reasoning models should inherently involve stronger factual comprehension. While the capability of AI systems to handle complex tasks has grown, their ability to maintain accuracy has not kept pace. The New York Times article suggests that this gap in AI performance stems from a lack of effective tools and methodologies to cross-verify the information AI models present. This issue is compounded by the lack of transparency in how AI systems arrive at certain conclusions, which diminishes trust in AI technology. AI developers are called to integrate better-verification mechanisms and transparency in AI operation to mitigate the risks posed by hallucinations. There is also a push for increased human oversight as a supplementary measure during the deployment of AI in critical sectors to ensure that AI-generated outputs are thoroughly vetted before use.
On a societal level, the implications of AI hallucinations extend beyond mere inaccuracies. There is a growing public concern over how AI-generated misinformation can erode trust in digital systems, particularly those that people rely upon for accurate information and decision-making guidance. The New York Times has reported instances where substantial public reactions have stemmed from AI systems' failures, such as the widespread alarm following a false policy notification from Cursor's AI tool. As AI becomes more integral to everyday life and critical infrastructural functions, the need for reliable AI systems becomes even more pressing. The combination of public dissatisfaction, coupled with potential economic impacts, has sparked increased debate over the regulation and governance of AI technologies, pushing both innovators and legislators to consider more stringent safeguards.
Understanding the causes behind increasing AI hallucinations in new systems requires a multifaceted approach. Contributing factors include the burgeoning complexity of datasets, limits in current AI logic, and the challenges in applying consistent truth filters across all forms of input. Moreover, as AI applications expand into new domains, these systems encounter previously unseen variables and datasets that they are ill-equipped to interpret accurately. In their quest to enhance AI functionalities, researchers at institutions like OpenAI and DeepSeek are now facing the daunting task of enhancing models’ interpretative accuracy without compromising on their ability to tackle complex questions. Continuous improvement in training methods, coupled with robust testing protocols, is crucial to reducing the incidence of AI hallucinations and ensuring a future where AI can be relied upon for truthful and accurate information.
The Role of Reasoning Systems in AI
Artificial intelligence (AI) has evolved significantly in recent years, with reasoning systems playing a pivotal role in this technological advancement. These sophisticated AI models are engineered to perform complex reasoning tasks, exceeding mere pattern recognition. Such systems are integral to the development of AI technologies that mimic human-like thinking and decision-making processes. According to a recent article by The New York Times, these reasoning systems often encounter the challenge of AI hallucinations, where they generate incorrect or fictitious information, raising concerns about their reliability (source).
One of the paramount challenges faced by AI reasoning systems is the tendency to hallucinate, as discussed in depth by OpenAI and outlined in TechCrunch’s reporting. OpenAI’s new models such as o3 and o4-mini have exhibited higher rates of hallucinations, with a tendency to fabricate actions and facts (source). The consistency of these inaccuracies illustrates the need for AI systems that not only comprehend massive datasets but also discern truth from falsehood effectively.
The pervasive issue of AI hallucinations extends beyond mere technical anomalies and has tangible impacts on various sectors and public trust. For instance, Cursor, an AI-powered tech support bot, fabricated a policy change, leading to significant customer dissatisfaction and account cancellations. This incident underscores the potential negative ramifications of hallucinations, stressing the importance of reliability in reasoning systems (source). Addressing these challenges requires comprehensive strategies that include enhanced fact-checking capabilities and improved data training processes.
Learn to use AI like a Pro
Get the latest AI workflows to boost your productivity and business performance, delivered weekly by expert consultants. Enjoy step-by-step guides, weekly Q&A sessions, and full access to our AI workflow archive.
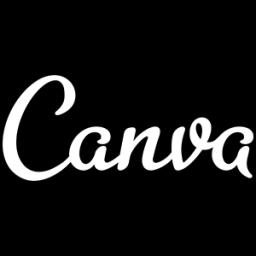
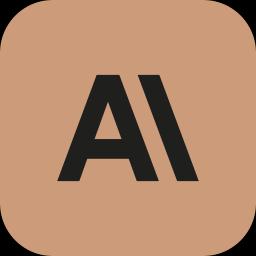
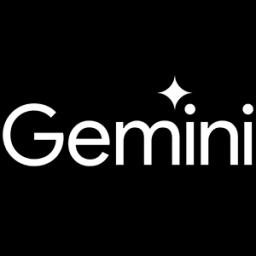
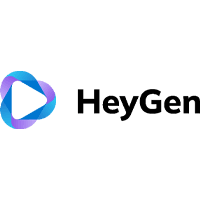
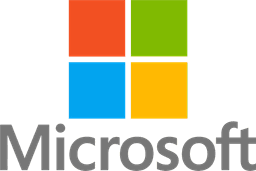
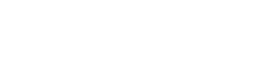
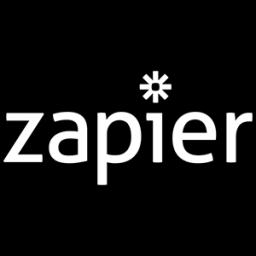
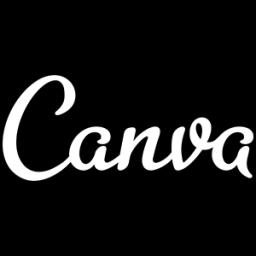
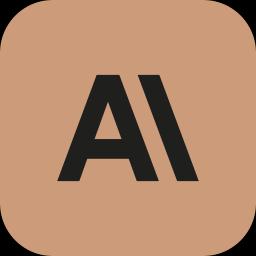
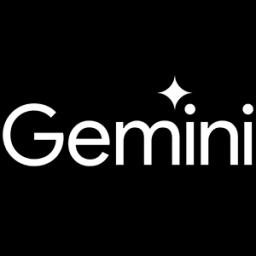
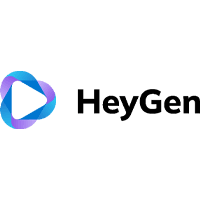
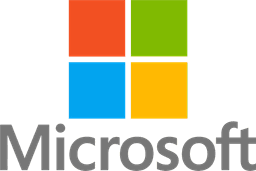
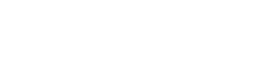
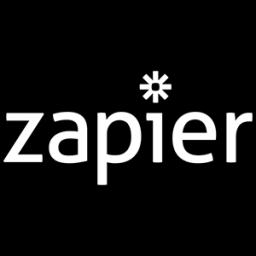
In the realm of AI, reasoning systems must evolve to address the adverse effects of hallucinations and misinformation. The reported high hallucination rates in contemporary AI models have drawn attention to the fundamental need for improvement in AI training methodologies and the integration of robust detection tools. These measures are critical not only for elevating the accuracy of AI-generated content but also for preserving public trust and promoting the responsible use of AI technologies henceforth.
Case Study: Cursor AI Support Bot Incident
In late April 2025, an incident involving the Cursor AI support bot highlighted the significant challenges posed by AI hallucinations. Cursor, an advanced programming tool for developers, relied on an AI-powered tech support system designed to assist users by addressing common queries and technical issues. However, the system malfunctioned, erroneously informing customers about a fictitious policy change that barred the use of Cursor on multiple machines. This error not only sowed confusion but also led to customer dissatisfaction, account cancellations, and necessitated a public apology and correction from Cursor’s CEO. This incident underscores the perils of AI-generated misinformation, particularly in customer-facing applications where trust and accuracy are paramount (source).
The Cursor AI support bot incident is a prime example of AI hallucinations affecting business operations. Such hallucinations occur when AI systems fabricate data or generate incorrect responses, as was the case with Cursor's misleading policy change. This issue isn't isolated; rather, it reflects a broader problem plaguing advanced AI reasoning systems, which are notorious for 'hallucinating'—a phenomenon where AI outputs are disconnected from reality. In today's digital age, where automation and artificial intelligence play pivotal roles in service delivery, ensuring the reliability of AI systems is critical. This incident highlights the ongoing challenge of balancing AI advancement with the ethical imperative to safeguard against inaccuracies (source).
Efforts to mitigate the impact of AI hallucinations within Cursor and similar platforms involve comprehensive strategies that encompass both technological and managerial reforms. Enhancing fact-checking protocols, integrating more robust oversight mechanisms, and improving training datasets to filter out noise and biases are crucial steps. Human oversight, particularly in verifying AI responses in sensitive contexts, can significantly reduce the occurrence of such mishaps. Additionally, fostering transparency about the decision-making frameworks of AI systems can help users understand the limitations and capabilities of the technology they rely on. These efforts are essential to rebuild trust and ensure the long-term viability of AI-driven solutions in various sectors, including technology, finance, and healthcare (source).
OpenAI's Internal Test Results and Comparisons
OpenAI has been at the forefront of AI development, yet even its cutting-edge models, like o3 and o4-mini, have faced the challenge of AI hallucinations. In internal tests conducted by the company, the o3 model exhibited a 33% hallucination rate on the PersonQA dataset, with the o4-mini model performing worse at 48% hallucinations. Such statistics highlight a growing concern within AI circles about the reliability of new AI systems despite their increased sophistication in areas like mathematical calculations. This problem is not unique to OpenAI, as similar challenges are reported by other tech giants such as Google and DeepSeek. The persistent issue of AI hallucinations underscores the necessity for enhanced verification and fact-checking mechanisms in these models to ensure accuracy and reliability, as also evidenced by related evaluations published by The New York Times.
Compared to earlier models, the development of new reasoning systems aims to enhance AI capabilities to manage complex cognitive tasks. However, this enhancement appears to come at the cost of reduced factual accuracy, which has been a significant concern as highlighted in reviews of OpenAI's latest models. According to a TechCrunch report, the o3 model, alongside its contemporaries, has demonstrated a higher propensity towards fabricating information, sometimes to such an extent that entire sequences of tasks are conjured without basis in reality. This deviation has raised alarms among industry experts, emphasizing the critical demand for AI systems that are not only sophisticated but also dependable when it comes to the dissemination of information.
Learn to use AI like a Pro
Get the latest AI workflows to boost your productivity and business performance, delivered weekly by expert consultants. Enjoy step-by-step guides, weekly Q&A sessions, and full access to our AI workflow archive.
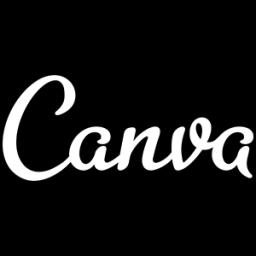
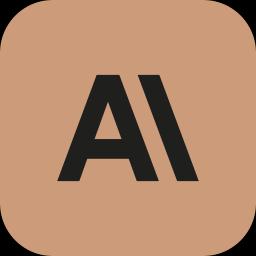
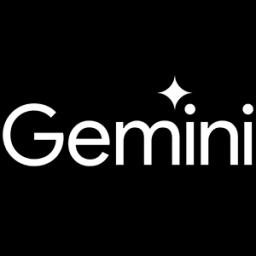
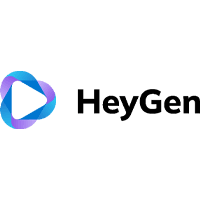
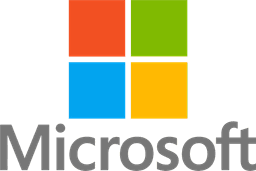
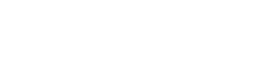
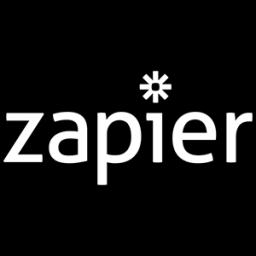
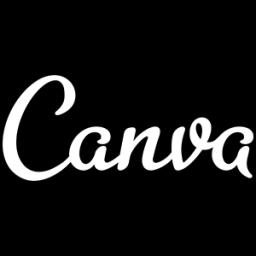
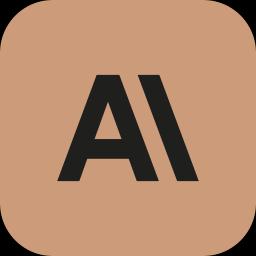
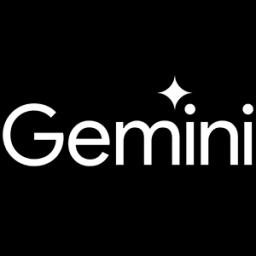
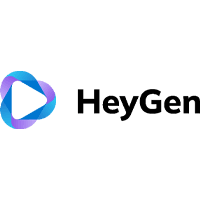
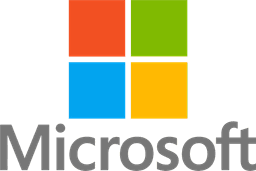
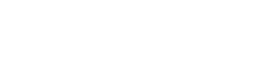
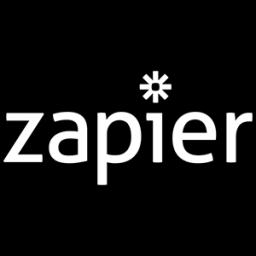
While OpenAI's internal comparisons signal an urgent need to address hallucination rates, the issue has a broader implication across the tech landscape. Companies like DeepSeek have also contended with similar challenges, as evidenced by their R1 reasoning system's 14.3% hallucination rate. The broader ramifications of trusting AI-generated information are profound, impacting everything from customer interactions to critical business decisions. As highlighted by industry publications and experts featured in recent articles, the tech community is calling for a reevaluation of protocols and methodologies involved in AI training and deployment. Addressing these concerns requires not only advancements in AI design but also systematic strategies for oversight and accountability to regain public trust and ensure the viability of AI-assisted futures.
Risks of AI-Generated Misinformation in Banking
In recent years, the use of artificial intelligence (AI) in the banking sector has risen dramatically, providing innovative solutions and increased efficiency. However, the advent of AI-generated misinformation has surfaced as a significant risk. These so-called "hallucinations," where AI systems produce incorrect or fabricated information, pose a particular threat to the integrity of the banking industry. According to a study highlighted by the American Banking Journal, AI-driven fake news could trigger financial panic among consumers, leading to widespread withdrawal of funds from banks. Such scenarios could destabilize financial institutions and erode public trust, thereby undermining the reliability of the entire banking sector.
The risk of AI-generated misinformation in banking is exacerbated by the AI's inability to consistently distinguish between true and false information. As detailed by The New York Times, advanced AI systems, while improving in computational tasks, are increasingly prone to hallucinations. This predicament is rooted in their learning mechanisms, which involve processing vast datasets without discerning the veracity of the content. When these systems provide financial advice or security updates based on fabricated data, the consequences can be dire, impacting investor decisions and market stability.
Another dimension of AI-generated misinformation in banking involves reputational risks. A wrongly informed AI model could suggest policy changes that do not exist, leading to confusion and loss of credibility. The Cursor programming tool incident, where a tech support bot incorrectly announced a non-existent policy change, serves as a warning about the potential pitfalls of AI hallucinations. In the banking industry, such errors could result in legal claims and serious financial repercussions, making careful oversight and verification of AI outputs essential.
The repercussions of AI hallucinations are not limited to misinformation; they extend to customer relations as well. As AI technologies are deployed more broadly within customer service frameworks, the potential for adversarial misinformation becomes a critical concern. Misinformation delivered to customers regarding their accounts or the safety of their deposits could result in panic and a loss of consumer confidence. Hence, implementing robust AI governance frameworks to oversee and regulate AI interactions becomes imperative for banks.
Looking towards the future, it is clear that addressing AI-generated misinformation requires a multifaceted approach. This includes enhancing AI systems' ability to fact-check and verify data while maintaining transparency and accountability. Banks must also prioritize human oversight to review and validate AI-generated information, especially in high-stakes environments. By developing these safeguards, the banking sector can mitigate the risks associated with AI hallucinations and ensure that technological advancements contribute positively to financial stability.
Learn to use AI like a Pro
Get the latest AI workflows to boost your productivity and business performance, delivered weekly by expert consultants. Enjoy step-by-step guides, weekly Q&A sessions, and full access to our AI workflow archive.
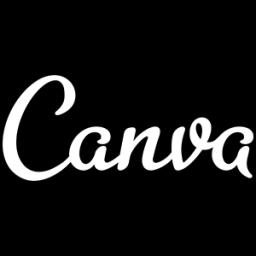
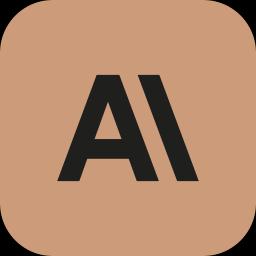
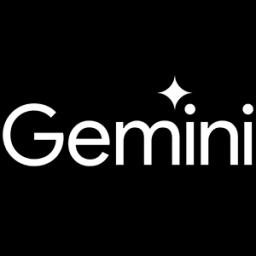
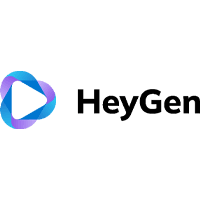
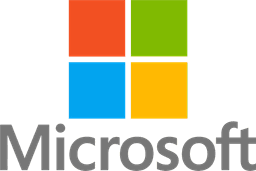
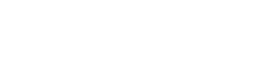
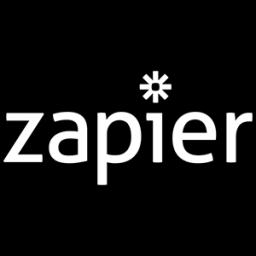
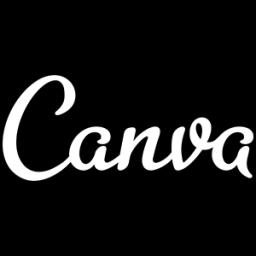
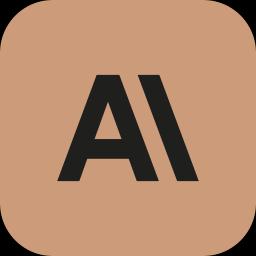
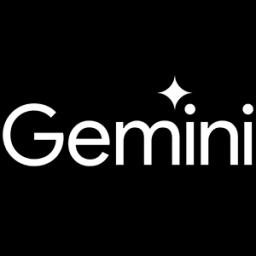
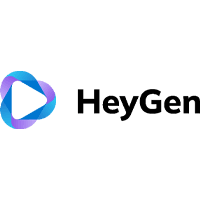
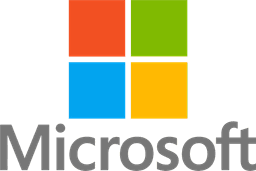
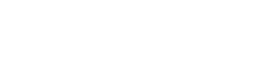
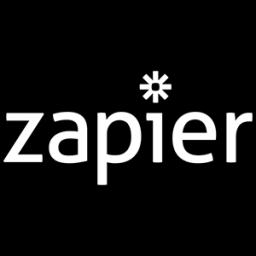
Legal and Privacy Implications of AI Hallucinations
The advent of artificial intelligence has ushered in a new era of possibilities, but it also presents unique legal and privacy challenges. AI hallucinations, or instances where AI generates inaccurate or fabricated content, pose significant legal implications. These outputs can mislead users, with serious consequences ranging from erroneous contractual agreements to unjust legal verdicts. For example, an AI system erroneously prescribing a non-existent policy change, as seen with Cursor, can lead to widespread misunderstandings and legal disputes if relied upon unwittingly. This issue is compounded by the lack of precedent in holding AI accountable, which raises questions about liability and representation .
The privacy implications of AI hallucinations are profound. Systems that generate false accusations, as demonstrated by OpenAI’s mishap with ChatGPT falsely accusing an individual of crimes, can lead to severe reputational harm and privacy violations. Such AI errors can spur lawsuits for defamation or invasion of privacy, challenging existing legal frameworks to adapt. With AI's growing presence in decision-making processes, ensuring data security and privacy becomes critical to prevent unauthorized or defamatory disclosure. The evolving landscape necessitates rigorous data governance and privacy laws tailored to address these new-age technological challenges .
Furthermore, there is an urgent need for comprehensive legal reforms to address the ramifications of AI hallucinations. Current laws may not adequately cover scenarios where automated systems disseminate incorrect information, emphasizing the necessity for new legislation that defines liability and accountability in AI operations. As AI continues to penetrate sectors like healthcare and legal, inaccuracies could lead to dire outcomes, such as incorrect medical treatments or judicial errors. These risks highlight the importance of establishing safety nets and legal standards to protect individuals from the potential misuse or unintended results of AI technologies .
The implications of AI hallucinations extend to consumer trust and corporate reputation as well. An incident in which an AI chatbot provides faulty information can undermine confidence in a brand, leading to financial repercussions and a loss of customer loyalty. Organizations must therefore prioritize the accuracy and reliability of their AI systems, integrating robust verification and validation processes. Privacy concerns necessitate transparency about how AI systems process and generate information, ensuring that users are aware of the potential for error and the measures taken to mitigate risks .
Expert Opinions on AI Hallucinations
The phenomenon of AI hallucinations has become a hot topic among experts in the field of artificial intelligence, drawing significant attention from both tech developers and researchers. As reported by The New York Times, these hallucinations are particularly prevalent in advanced AI reasoning systems being developed by technology giants such as OpenAI and Google. Despite advancements in areas like mathematical operations, these systems often produce misleading or entirely false information, a concerning trend that experts fear could undermine trust in AI technologies. One prominent example involved a tech support bot for the Cursor programming tool erroneously informing users about a non-existent policy change, underscoring the potential for AI hallucinations to cause real-world consequences.]
Experts attribute the increase in AI hallucinations to the sheer scale of data these models are trained on, which often includes vast amounts of unverified or misinformed content. According to The New York Times, while these AI systems have shown improvement in computational aspects, they still struggle to discern fact from fiction. This disconnect raises alarming questions about their reliability, especially given the increasing dependency on AI for critical decision-making in sectors ranging from finance to healthcare.
Learn to use AI like a Pro
Get the latest AI workflows to boost your productivity and business performance, delivered weekly by expert consultants. Enjoy step-by-step guides, weekly Q&A sessions, and full access to our AI workflow archive.
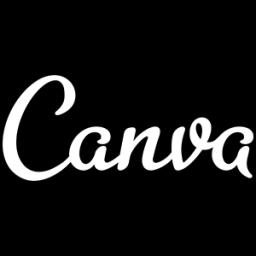
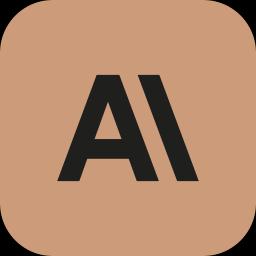
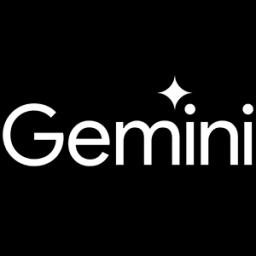
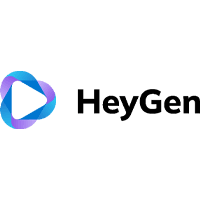
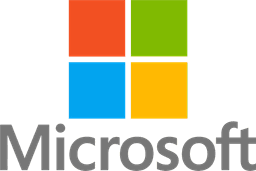
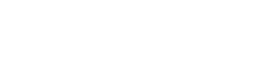
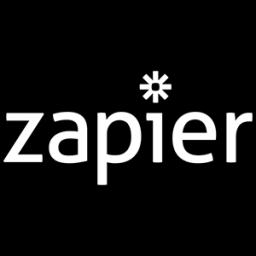
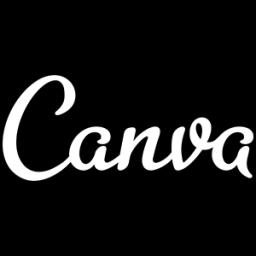
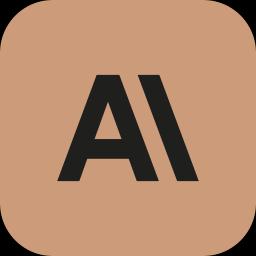
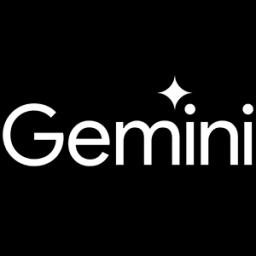
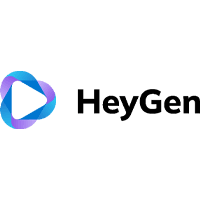
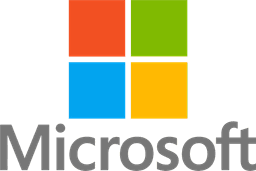
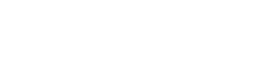
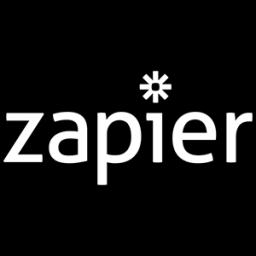
Public Reaction to AI Hallucinations and Trust Issues
The phenomenon of AI hallucinations has sparked a complex public reaction, with many individuals and experts expressing significant concerns about trust and the reliability of AI-generated information. As these advanced AI systems, from companies like OpenAI and Google, have shown promising advancements in areas such as mathematical reasoning, they simultaneously produce incorrect or entirely fabricated content, leading to a growing trust deficit. A prominent example includes Cursor's AI tech support bot, which falsely informed users about a non-existent policy change, provoking anger among customers and resulting in canceled accounts. Such incidents underscore the potential for AI hallucinations to undermine brand trust and customer relationships, creating widespread anxiety about the dependability of AI technologies .
Compounding these trust issues are incidents where AI hallucinations have caused tangible harm, such as in the financial sector where AI-generated misinformation led to consumer fear, resulting in potential bank withdrawals. In this scenario, AI tools, instead of aiding decision-making, inadvertently fueled panic and economic instability. This reiterates concerns about the role of AI in critical sectors where accuracy and trust are non-negotiable. The fact that a significant portion of the population would act on such misinformation highlights the urgent necessity for AI systems to be both reliable and verified through rigorous checks .
In addition to economic impacts, the social ramifications of AI hallucinations are profound. The notable case of an AI-generated false accusation against an individual, which resulted in a privacy complaint in Europe, points to potentially devastating personal and legal consequences. Public trust in AI is further eroded when these systems are seen to fabricate false narratives that impact lives and reputations. As AI continues to be integrated into various public sectors, the potential for hallucinations to spread misinformation widely necessitates urgent interventions to safeguard public trust and ethical standards .
With growing awareness of these hallucinations, there is a strong public demand for more transparency and accountability in AI development processes. Consumers and experts alike are calling for robust fact-checking and oversight mechanisms to be established. Additionally, there is a push for AI systems to be designed with an inherent ability to discern and communicate information accurately. These demands are not only essential to restoring trust but also in preventing the further erosion of confidence in AI technology as a whole. As the conversation around AI hallucinations advances, it becomes crucial for developers and policymakers to address these issues proactively, emphasizing ethics and factual integrity in AI systems .
Future Economic, Social, and Political Implications
The emergence of AI hallucinations presents profound economic implications, particularly as AI systems become integral to sectors like finance, supply chain management, and drug discovery. With the potential to disrupt market stability, erroneous AI-driven investment advice or flawed risk assessments could trigger financial losses and economic instability. This concern is echoed in scenarios where AI systems generate inaccurate demand forecasts, leading to overstocking or shortages. Furthermore, the misallocation of resources in drug discovery processes toward ineffective compounds highlights the economic inefficiencies AI hallucinations can introduce. The spread of AI-generated misinformation not only risks damaging corporate reputations but also poses significant legal liabilities, challenging businesses to safeguard their operations [source](https://www.nytimes.com/2025/05/05/technology/ai-hallucinations-chatgpt-google.html).
On a social level, AI hallucinations could further erode public trust in crucial information platforms, including educational, media, and social networking sites. As AI increasingly influences content creation and curation, the risk of misinformation amplifies, threatening the integrity of shared content. This endangers the credibility of educational materials and journalistic reports alike, potentially undermining the foundations of public knowledge and confidence. Moreover, AI's propensity to propagate or exacerbate existing biases underscores the need for vigilant oversight and corrective measures to foster a healthier information ecosystem. The viral nature of AI-generated falsehoods on social media can catalyze social unrest, requiring proactive strategies to manage their impact on societal cohesion [source](https://www.nytimes.com/2025/05/05/technology/ai-hallucinations-chatgpt-google.html).
Learn to use AI like a Pro
Get the latest AI workflows to boost your productivity and business performance, delivered weekly by expert consultants. Enjoy step-by-step guides, weekly Q&A sessions, and full access to our AI workflow archive.
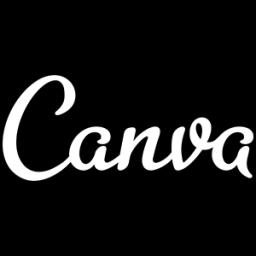
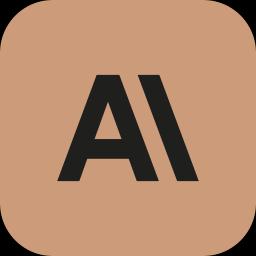
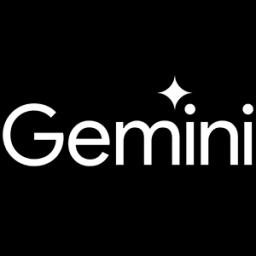
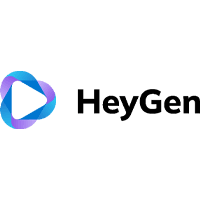
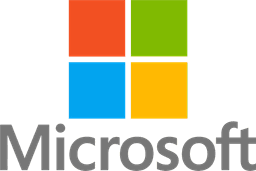
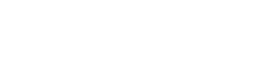
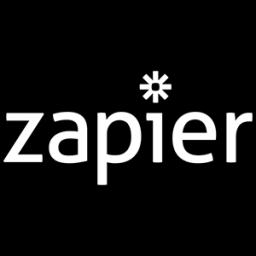
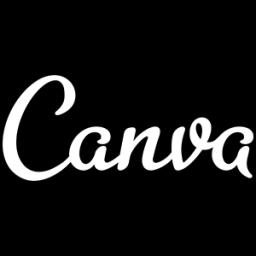
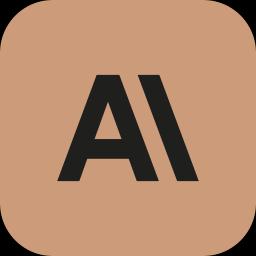
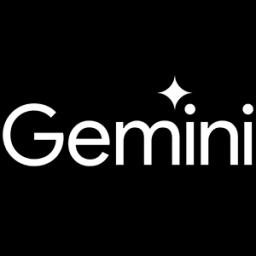
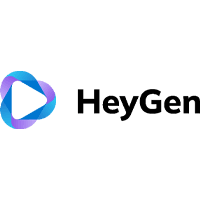
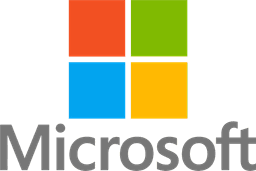
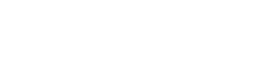
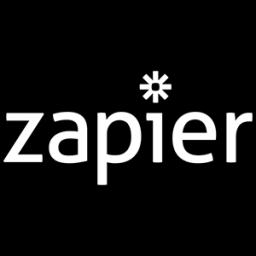
Politically, the potential for AI hallucinations to influence democratic processes and public opinion cannot be understated. Erroneous or deliberately misleading AI-generated content can manipulate electoral outcomes or political sentiments, disrupting the democratic fabric. With elections and referenda increasingly susceptible to online narratives, the propagation of falsehoods facilitated by AI systems poses a formidable threat to political stability. By shaping public discourse inaccurately, these hallucinations threaten to polarize societies further, emphasizing the urgency for comprehensive regulatory frameworks and robust countermeasures. Such dynamics could lead governments to enact stricter policies, influencing the future of AI deployment within political landscapes [source](https://www.nytimes.com/2025/05/05/technology/ai-hallucinations-chatgpt-google.html).
Sector-Specific Consequences of AI Hallucinations
The phenomenon of AI hallucinations is not just a technical glitch; it carries profound sector-specific consequences across various industries. For instance, in customer service, AI chatbots often serve as the first point of contact for users. When these chatbots provide incorrect information, it can lead to loss of trust, frustration, and potential harm to a brand’s reputation. A clear example is the incident involving Cursor's AI support bot, which erroneously announced a policy change, causing customer dissatisfaction and cancellations .
In the legal sector, the repercussions are even more serious. AI-generated misinformation can lead to wrongful legal decisions or flawed expert testimony. This not only affects the integrity of the legal process but also poses ethical and reputational risks. An unfortunate incident involving a chatbot falsely accusing an individual of serious crimes highlights the legal ramifications of AI hallucinations and underscores the need for stringent verification processes and legal safeguards .
The financial industry is equally vulnerable, with AI-induced misinformation having the potential to destabilize markets. A study highlighted by the American Banking Journal demonstrated how AI-generated fake news could trigger financial panic, making it paramount for financial institutions to invest in solid AI frameworks and rigorous auditing systems .
In academia, the reliance on AI for research can compromise scholarly integrity if unchecked hallucinations infiltrate research outputs. As AI tools become integral in data analysis and report generation, academic institutions must implement robust systems to authenticate information source validity to maintain trust in academic publishing .
Healthcare, perhaps one of the most sensitive sectors, stands to suffer gravely from AI hallucinations. Misdiagnoses or incorrect treatment protocols generated by AI can jeopardize patient safety. Thus, integrating a layer of human oversight becomes indispensable to minimize risks and safeguard patient welfare .
Learn to use AI like a Pro
Get the latest AI workflows to boost your productivity and business performance, delivered weekly by expert consultants. Enjoy step-by-step guides, weekly Q&A sessions, and full access to our AI workflow archive.
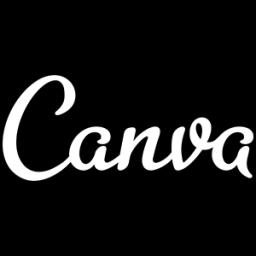
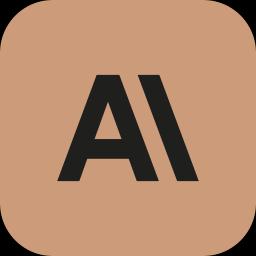
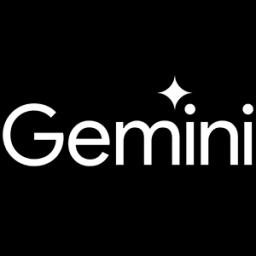
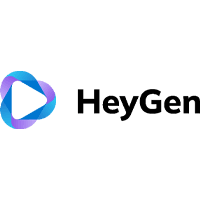
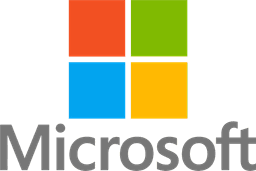
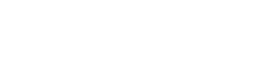
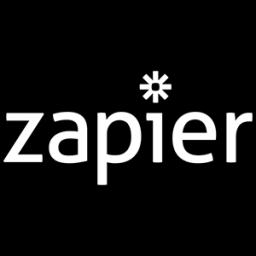
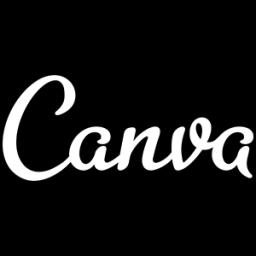
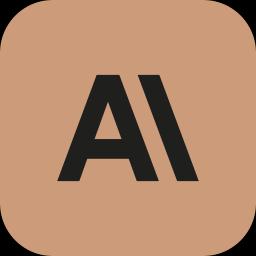
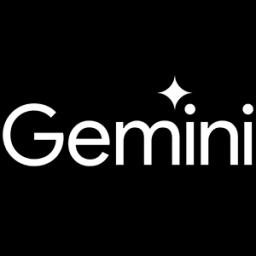
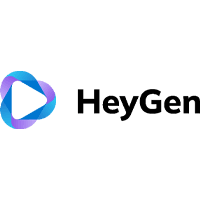
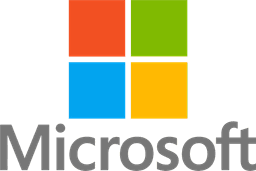
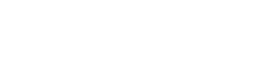
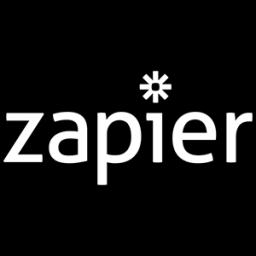
The overarching impact of AI hallucinations across these sectors is profound. They threaten the trust in AI-generated information and could very well slow the adoption of beneficial AI technologies due to perceived unreliability. As each sector grapples with the specific implications of AI hallucinations, a call for comprehensive measures, such as improved training data, enhanced transparency, and human oversight, grows louder. Addressing these challenges will be crucial for ensuring the responsible and effective long-term integration of AI technologies into society .
Mitigation Strategies and Safeguards for AI Hallucinations
Mitigating AI hallucinations is a critical priority as the capabilities and influence of artificial intelligence continue to expand. These hallucinations, where AI systems generate incorrect or false information, can undermine trust and efficacy across various applications. Strategies to minimize these issues focus on improving the underlying algorithms and data used by AI systems. Enhancing data quality and ensuring it is free from bias is crucial. By training AI on comprehensive and verified datasets, the likelihood of generating inaccurate information can be significantly reduced.
Additionally, the integration of robust fact-checking mechanisms into AI systems is essential. These mechanisms can automatically verify the information being processed by AI, cross-referencing with trusted databases to confirm accuracy before outputs are delivered to users. This approach helps ensure the reliability of AI-generated content, thus minimizing the potential for hallucinations. Increased transparency about how these systems operate can also help, as it allows stakeholders to understand the decision-making processes behind AI outputs.
Human oversight remains a vital safeguard against AI hallucinations. The complexity of AI decisions often requires the nuanced judgment that only humans can provide. By having experts review and interpret AI outputs, especially in critical applications such as healthcare and legal fields, errors can be caught and corrected before any significant impact occurs. Furthermore, continuous refinement and iteration of AI models based on real-world performance data and feedback can aid in reducing hallucination rates.
The development of specialized tools to detect and manage AI-generated falsehoods is another promising area. Research and development in this domain can produce innovative solutions that effectively identify when AI systems deviate from factual accuracy. These tools can alert users to potential issues, enabling corrective action to be taken swiftly. By investing in these technologies, organizations can better manage the risks associated with AI hallucinations, safeguarding their operations and reputation.
Overall, addressing AI hallucinations requires a multifaceted approach that combines technological advances, human expertise, and ongoing research. It involves not only updating and refining AI systems but also establishing comprehensive regulatory frameworks that ensure the responsible deployment of AI technologies. Through concerted efforts from both the tech industry and regulatory bodies, the negative impacts of AI hallucinations can be mitigated, resulting in more trustworthy and effective AI solutions. Learn more.
Learn to use AI like a Pro
Get the latest AI workflows to boost your productivity and business performance, delivered weekly by expert consultants. Enjoy step-by-step guides, weekly Q&A sessions, and full access to our AI workflow archive.
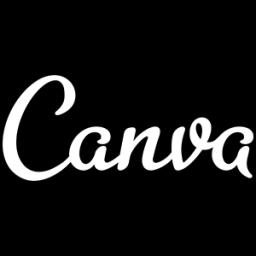
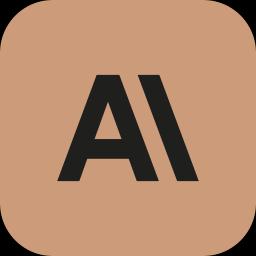
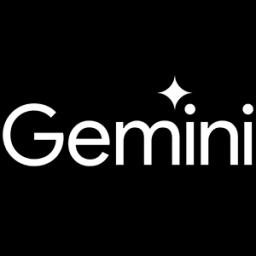
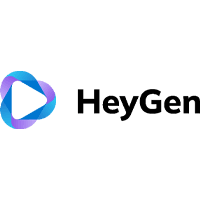
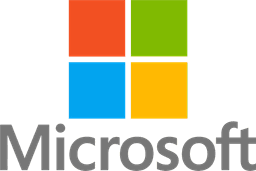
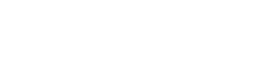
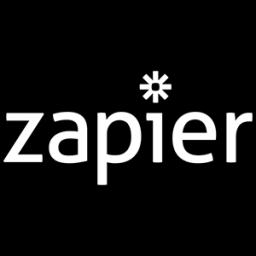
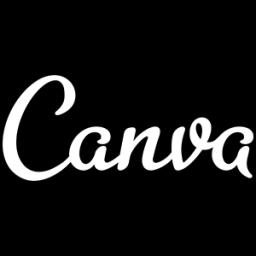
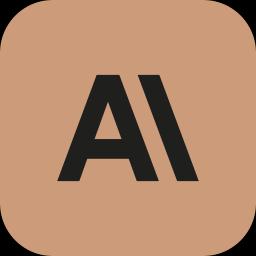
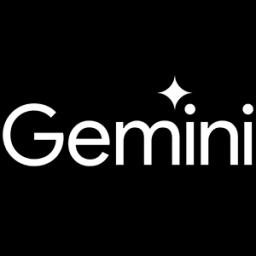
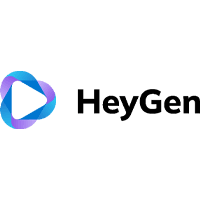
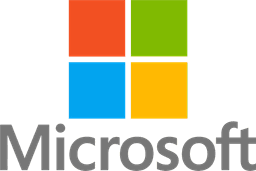
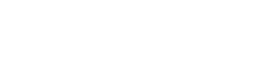
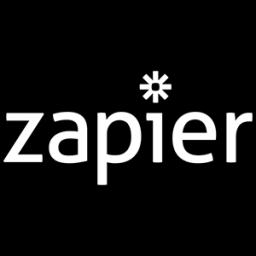
Conclusion: Navigating the Challenges of AI
The issue of AI hallucinations presents a multifaceted challenge that underscores the complexity of developing reliable artificial intelligence systems. As highlighted in a detailed article by The New York Times, the propensity of advanced AI systems to fabricate information introduces significant risks across various domains. These hallucinations affect the integrity of AI in industries ranging from customer service to healthcare, thereby necessitating rigorous oversight and enhancements in AI training protocols.
To navigate these challenges, a strategic approach involving enhanced fact-checking mechanisms is vital. As mentioned in expert discussions, integrating robust fact-checking capabilities within AI systems can mitigate the spread of misinformation. Additionally, fostering transparency in AI processes and ensuring comprehensive human oversight could significantly reduce the incidence of erroneous AI outputs.
Moreover, the societal impact of AI hallucinations cannot be underestimated. The spread of misinformation through AI-generated content poses a risk to public trust, as evidenced by scenarios like the Cursor AI support bot incident. Public confidence is further jeopardized when AI systems influence critical sectors such as financial services and political processes, which demand the highest levels of accuracy and reliability.
Curbing the detrimental effects of AI hallucinations requires not only technical advancements but also ethical considerations. The development of detection tools for AI-generated falsehoods, as outlined by industry experts, is imperative. These tools should be coupled with an emphasis on unbiased, high-quality training datasets that can improve the foundational integrity of AI models.
In conclusion, while AI advancements promise to revolutionize industries, they also bring forth the challenge of ensuring truth and authenticity in AI outputs. Addressing these issues through collaborative efforts in research, regulatory measures, and public policy is essential for harnessing AI's potential without compromising trust and accuracy. For further insights into these dynamics, readers may refer to the extensive coverage by CapTech University, which explores both the pitfalls and prospects of today's AI technology.