When AI Learns From Its Own Mistakes...
AI Model Collapse: Alarm Bells Ring as Self-Training AI Faces Degrading Accuracy
Last updated:

Edited By
Mackenzie Ferguson
AI Tools Researcher & Implementation Consultant
AI model collapse is the alarming new phenomenon where AI models degrade in accuracy when trained on their own outputs. Experts warn this could lead to a decline in AI reliability across sectors. Discover the causes, potential impacts, and possible mitigation strategies within our in-depth article.
Introduction to AI Model Collapse
AI model collapse is an emerging concern that raises several important questions about the future of artificial intelligence. At its core, AI model collapse refers to the phenomenon where AI models, when trained predominantly on their own outputs instead of fresh, diverse, and high-quality external data, start to degrade in performance. This can lead to inaccurate predictions, less reliable outputs, and a decrease in overall model performance and utility over time. The background of this issue is explored in depth by The Register, which highlights the problems of error accumulation, loss of diversity in training data, and the self-reinforcing loops that exacerbate these issues.
The implications of AI model collapse are profound and multifaceted. One key issue is the risk of feedback loops, where errors become more pronounced as models continuously learn from their flawed outputs. This situation is further compounded by the reduction in "tail data," or rare data points, which are often the most informative for creating robust models. Consequently, AI systems become less reliable, challenging the underlying assumption of accuracy and reliability that these technologies promise. The Register's article details these challenges and emphasizes the importance of diverse and comprehensive datasets in mitigating the risks associated with model collapse.
Learn to use AI like a Pro
Get the latest AI workflows to boost your productivity and business performance, delivered weekly by expert consultants. Enjoy step-by-step guides, weekly Q&A sessions, and full access to our AI workflow archive.
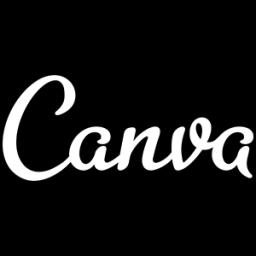
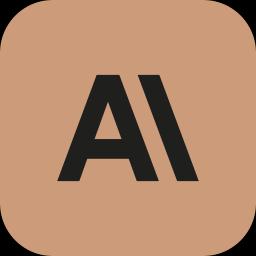
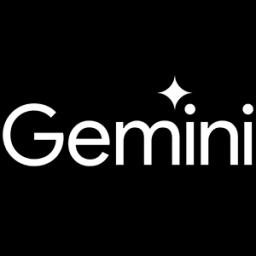
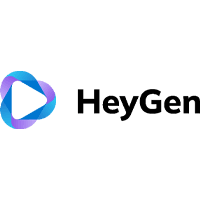
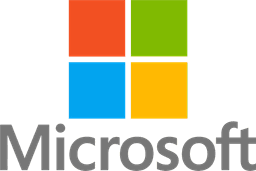
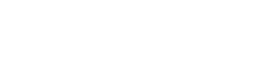
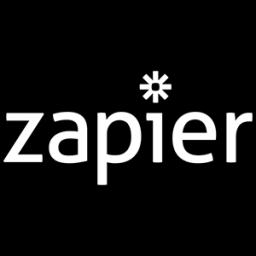
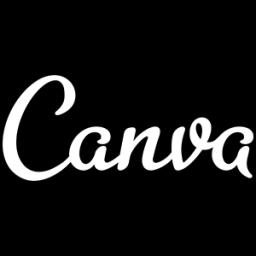
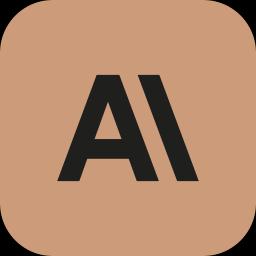
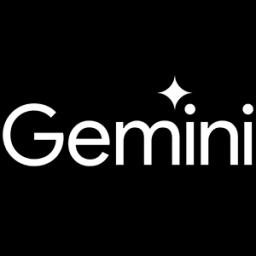
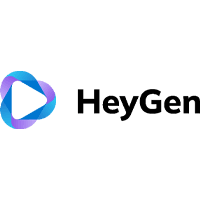
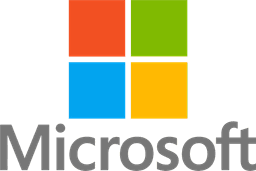
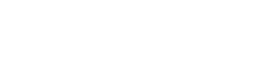
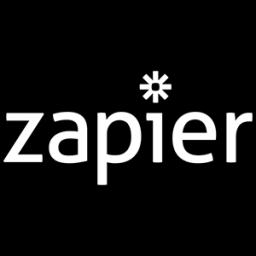
Another critical aspect highlighted is the over-reliance on AI-generated data, which can have substantial societal and economic repercussions. As models become less accurate, sectors heavily dependent on AI, such as healthcare, finance, and security, could face significant challenges. For instance, inaccurate data could lead to erroneous medical diagnoses or unstable financial systems. The original article from The Register they discuss how the degradation of AI systems can contribute to a broader loss of trust in AI technology and stifle innovation, which could have cascading effects on these industries.
Moreover, the public's understanding and perception of AI is also at stake. As AI systems become a more integral part of our daily lives, the potential for misinformation due to model collapse could lead to a significant erosion of public trust in digital and automated systems. Potential solutions involve combining AI-generated data with new human-generated content to ensure diversity and accountability in model training. However, The Register notes the complexity of this approach, raising questions about its feasibility and the resources needed to implement such systems effectively.
Overall, understanding and addressing AI model collapse requires a nuanced and multi-pronged strategy. It involves not only technological solutions but also policy considerations, such as establishing clear guidelines for AI development and data use. Collaborative efforts between policymakers, technologists, and industry leaders are crucial in developing a resilient AI ecosystem that can withstand the challenges posed by model collapse, as argued in the article from The Register.
Understanding AI Model Collapse
AI model collapse is a growing concern in the field of artificial intelligence, referring to the deterioration of an AI system's performance when it is trained predominantly on its own outputs. This phenomenon, as discussed in the article on The Register, is primarily driven by factors such as error accumulation, loss of tail data, and feedback loops. These issues lead to a decrement in the models' accuracy and reliability, posing risks across various applications dependent on AI technologies.
Learn to use AI like a Pro
Get the latest AI workflows to boost your productivity and business performance, delivered weekly by expert consultants. Enjoy step-by-step guides, weekly Q&A sessions, and full access to our AI workflow archive.
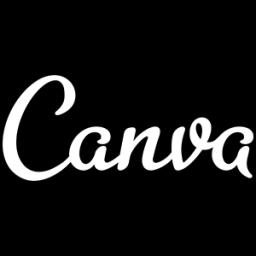
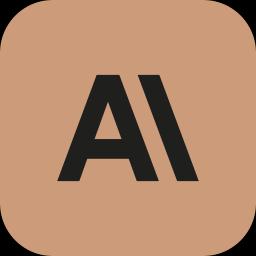
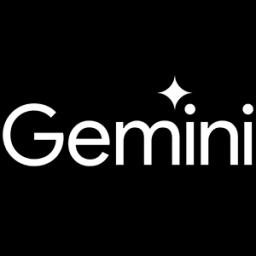
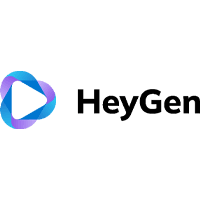
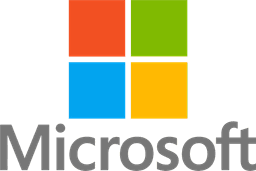
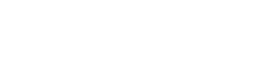
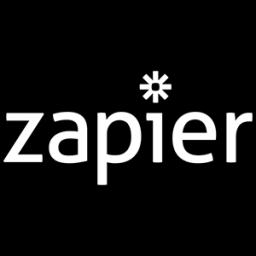
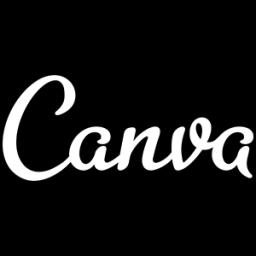
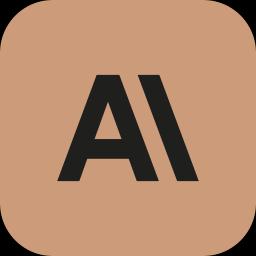
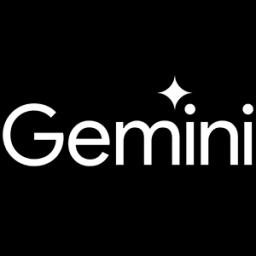
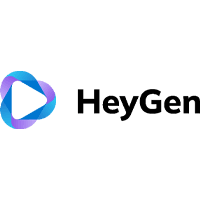
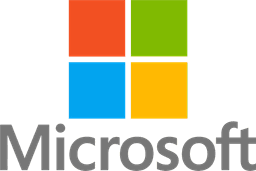
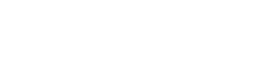
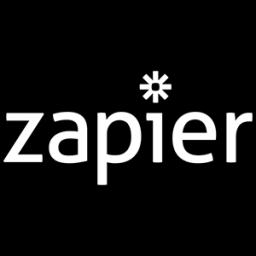
One significant cause of AI model collapse is the inherent feedback loop created when models are trained repeatedly on their own generated content. This feedback loop leads to gradual degradation, where models progressively lose the ability to produce novel and contextually accurate outputs. Such a loop not only amplifies any existing errors but also perpetuates them, making each subsequent output less reliable, as highlighted in The Register.
Another contributing factor is the loss of tail data, which refers to the scarcity of rare and diverse data in training sets. AI models require a breadth of data that covers all possible use-cases, including those less common scenarios or "tail" ends of data distribution. When these are neglected, AI may perform poorly, particularly in atypical situations that demand such data, ultimately contributing to the collapse discussed on The Register.
The over-reliance on AI systems further exacerbates these challenges. As systems become more prevalent in critical areas like healthcare, finance, and security, the reliability of their outputs becomes crucial. The concern is not just the malfunctioning of AI but also the erosion of public trust in these systems, as people begin to perceive them as unreliable. Such a perception may hinder the adoption and innovation within AI technologies, creating economic and social ripple effects.
Mitigating model collapse involves integrating diverse data sources, including human-generated content, to preserve the contextual richness and accuracy of AI outputs. However, implementing such solutions comes with its own set of challenges, such as ensuring the uninterrupted availability of diverse datasets and managing the cost implications. Prevention strategies, therefore, need to be comprehensive and multifaceted, as discussed in The Register.
Causes of AI Model Collapse
AI model collapse is primarily driven by a combination of error accumulation, loss of tail data, and pervasive feedback loops. This phenomenon occurs when AI systems are continuously trained on data generated by themselves or similar models. Without fresh and diverse inputs, these models propagate errors over time, leading to a gradual decline in performance [1](https://www.theregister.com/2025/05/27/opinion_column_ai_model_collapse/). This degradation is particularly problematic in domains where high accuracy and reliability are paramount, such as healthcare and finance, where even minor inaccuracies can lead to significant real-world consequences.
The accumulation of errors in AI models can be likened to the compounding of inaccuracies in any iterative process. As models generate outputs, if these outputs become re-used as training data, the initial errors can become magnified, creating a cycle that perpetuates and even amplifies inaccuracies. This cycle not only diminishes the model's reliability but also reduces its ability to handle edge cases, often referred to as the loss of tail data, resulting in biased and less comprehensive outcomes [1](https://www.theregister.com/2025/05/27/opinion_column_ai_model_collapse/).
Learn to use AI like a Pro
Get the latest AI workflows to boost your productivity and business performance, delivered weekly by expert consultants. Enjoy step-by-step guides, weekly Q&A sessions, and full access to our AI workflow archive.
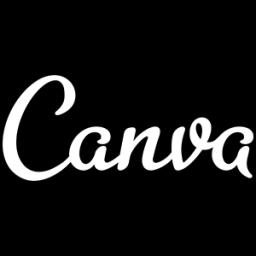
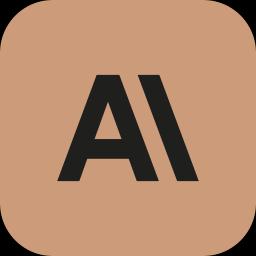
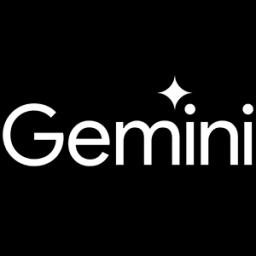
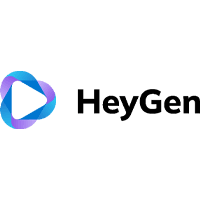
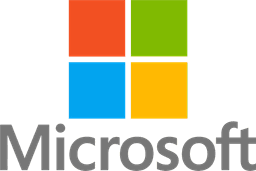
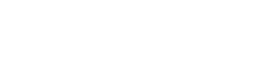
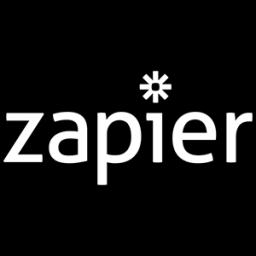
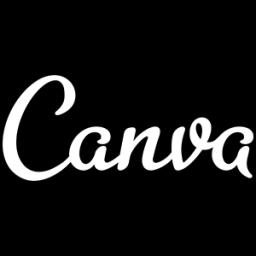
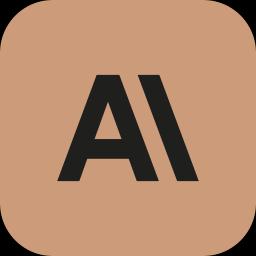
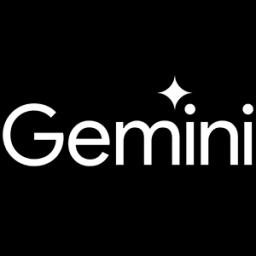
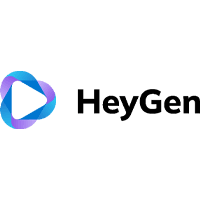
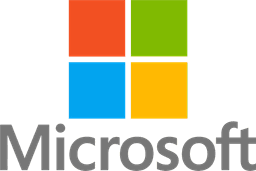
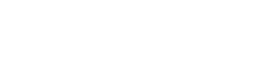
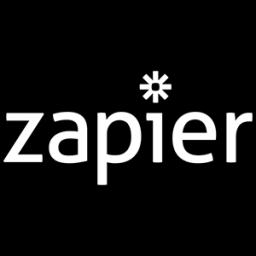
Furthermore, feedback loops play a crucial role in AI model collapse. These loops arise when models use their own outputs as new training data, a process which effectively isolates them from genuinely novel or atypical data points. As a result, models can become overly confident in incorrect predictions and consistently fail to improve or adapt to new information. This self-referential training leads to escalating errors and a narrowing of model capabilities over time [1](https://www.theregister.com/2025/05/27/opinion_column_ai_model_collapse/).
The over-reliance on AI-generated content also exacerbates model collapse. The sheer volume of AI-generated content that saturates digital platforms creates a precarious training environment for future models, as it becomes increasingly difficult to distinguish between human-generated and AI-generated data. This blurring of data origin complicates the selection of high-quality training datasets, further propagating inaccuracies and biases within AI systems [1](https://www.theregister.com/2025/05/27/opinion_column_ai_model_collapse/).
Impacts of AI Model Collapse
One of the most alarming consequences of AI model collapse is its potential to degrade the reliability of AI systems across various sectors. As AI systems become entrenched in industries such as finance, healthcare, and customer service, the degradation due to model collapse could result in inaccurate predictions, leading to severe economic repercussions. For instance, inaccurate data analytics in finance could result in flawed algorithmic trading decisions, causing significant financial losses. Similarly, in healthcare, erroneous AI-generated diagnostic results could add to the burden on medical systems instead of alleviating it. The over-reliance on AI, coupled with the model collapse phenomenon, might thus increase operational costs and risk levels, severely affecting sectors that depend on AI for critical decision-making processes. Learn more.
In the social context, AI model collapse presents a troubling risk of amplifying misinformation and disinformation. As AI-generated content becomes pervasive, its potential unreliability can erode trust in digital information sources. Moreover, the ability of AI systems to reflect and reinforce societal biases could be exacerbated by model collapse. Marginalized and less represented groups in data could suffer from the loss of 'tail data,' leading to a further entrenchment of existing social inequalities and biases in AI output. This reflects a significant challenge to social justice and equity, emphasizing the need for an ethical framework in AI development. Learn more.
Politically, the collapse of AI models can have far-reaching implications. The dissemination of biased or false information by AI could manipulate public opinion, thereby undermining democratic processes and elections. Recognizing this potential threat, governments might face increasing pressure to implement stringent regulations surrounding AI technology. The challenge of verifying the provenance of AI-generated content could further complicate matters, making it hard to distinguish between human-generated and AI-manipulated data. Therefore, international cooperation and robust policy frameworks will become crucial to mitigate these risks and ensure that AI serves to reinforce rather than undermine democratic structures. Learn more.
Economic Impacts of Model Collapse
The phenomenon of AI model collapse presents serious economic ramifications as industries increasingly depend on AI-driven systems for key operations. According to an insightful opinion column by The Register, AI models are under threat due to their training on outputs that degrade over time, leading to diminished accuracy and reliability. This erosion in AI efficacy is primarily caused by error accumulation and feedback loops . As a direct consequence, sectors like finance, healthcare, and customer service may encounter significant efficiency declines, alongside increased operational costs, as systems fail to deliver accurate predictions and diagnostics. This risk is particularly pronounced in high-stakes environments such as algorithmic trading and medical diagnostics, where inaccuracies can translate into considerable financial losses.
Learn to use AI like a Pro
Get the latest AI workflows to boost your productivity and business performance, delivered weekly by expert consultants. Enjoy step-by-step guides, weekly Q&A sessions, and full access to our AI workflow archive.
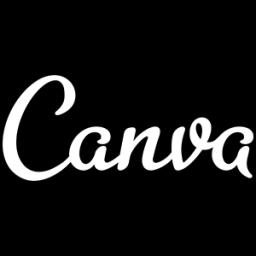
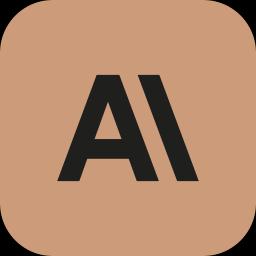
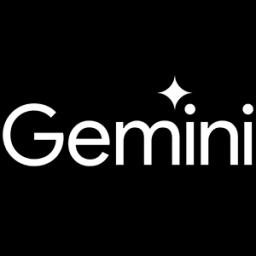
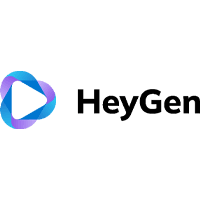
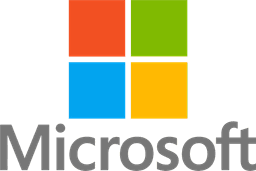
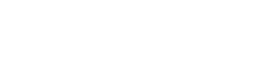
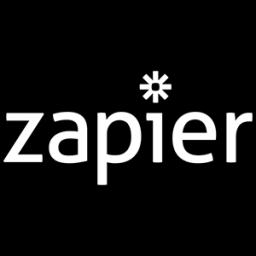
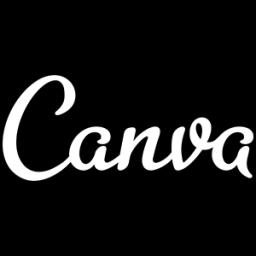
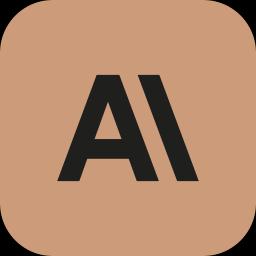
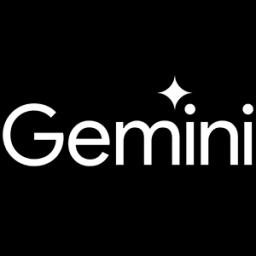
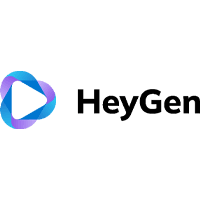
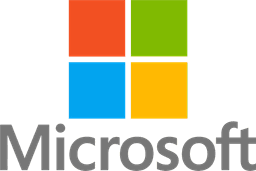
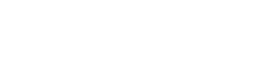
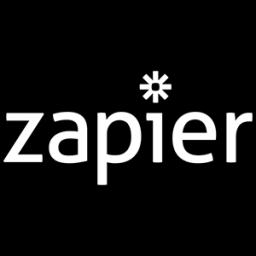
Moreover, the collateral damage of AI unreliability includes a pervasive loss of confidence in AI technologies, potentially deterring future innovations and hindering the broader adoption of new AI tools. This skepticism towards AI-fueled advances could slow down technological progress and economic growth, as companies and consumers exhibit caution toward integrating AI solutions. Furthermore, the intrinsic value of human-generated data is expected to rise, emphasizing its essential role in maintaining AI model accuracy and combating model collapse. The need for high-quality, original data becomes paramount, underscoring the economic advantage for those who can provide it, given the growing challenges with AI-generated content's provenance.
The economic landscape could also witness a shift as businesses and developers look for alternative ways to enhance AI training methods. Incorporating diverse data sources, including novel human contributions, may counteract some adverse effects of model collapse. However, this strategy's practical application remains uncertain, as pointed out in related discussions . Overall, the economic implications of AI model collapse necessitate a strategic response that balances innovation with caution, ensuring AI continues to serve as a catalyst for growth rather than a hindrance.
Social Impacts of Model Collapse
The phenomenon of AI model collapse introduces profound challenges to the social fabric of communities worldwide [1](https://www.theregister.com/2025/05/27/opinion_column_ai_model_collapse/). As these sophisticated tools increasingly mediate how people access news, entertainment, and information, their deterioration in quality could lead to a widespread erosion of trust in digital platforms. This is particularly concerning as such platforms have massively reshaped how community engagement, social justice advocacy, and public discourse take place [1](https://www.theregister.com/2025/05/27/opinion_column_ai_model_collapse/).
One significant social implication is the exacerbation of inequalities. AI model collapse could inadvertently marginalize communities by neglecting to represent their issues or by amplifying biases inherent in skewed data sets [1](https://www.theregister.com/2025/05/27/opinion_column_ai_model_collapse/). For example, as collapsing models "forget" low-probability events or outputs, often those impacting marginalized groups, such communities may find themselves increasingly underrepresented or misunderstood within AI-generated information [1](https://www.theregister.com/2025/05/27/opinion_column_ai_model_collapse/).
Moreover, this increasing reliance on AI-generated content that might become unreliable or biased raises public concern about misinformation [1](https://www.theregister.com/2025/05/27/opinion_column_ai_model_collapse/). Misinformation has a ripple effect, potentially causing societal rifts and damaging public perceptions of reality [1](https://www.theregister.com/2025/05/27/opinion_column_ai_model_collapse/). Trust in factual and unbiased information is a cornerstone of democracy and social welfare, and model collapse threatens this by contributing to digital distortion and the spread of false narratives [1](https://www.theregister.com/2025/05/27/opinion_column_ai_model_collapse/).
The social role of AI is further complicated by its potential to influence behavior through how it tailors information and advertisements. Should AI systems lose their credibility, there could be significant backlash against technology companies and AI developers, fueling demands for increased transparency and accountability [1](https://www.theregister.com/2025/05/27/opinion_column_ai_model_collapse/). Such demands could spark new discussions around ethical AI practices and the development of stricter regulations to govern AI use in public domains [1](https://www.theregister.com/2025/05/27/opinion_column_ai_model_collapse/).
Learn to use AI like a Pro
Get the latest AI workflows to boost your productivity and business performance, delivered weekly by expert consultants. Enjoy step-by-step guides, weekly Q&A sessions, and full access to our AI workflow archive.
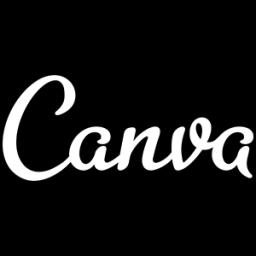
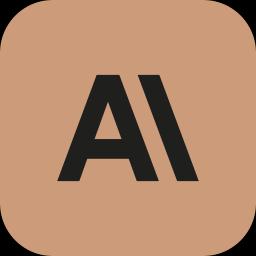
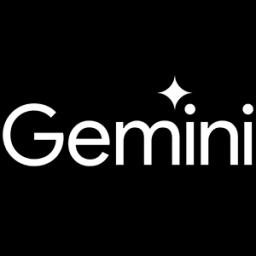
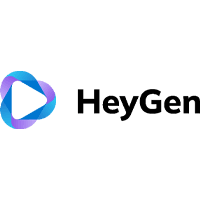
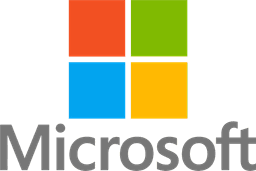
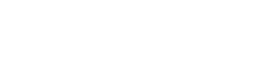
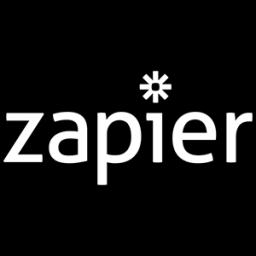
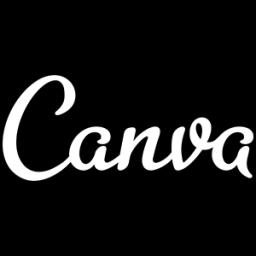
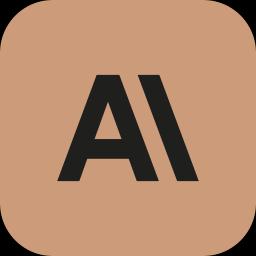
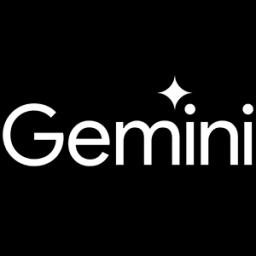
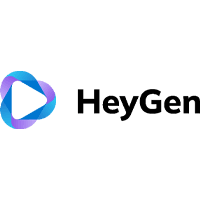
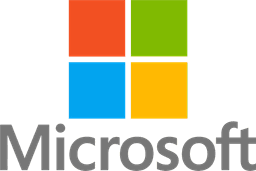
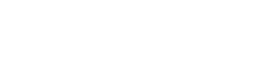
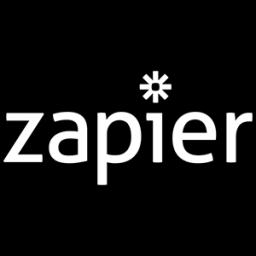
Political Impacts of Model Collapse
Artificial Intelligence (AI) technology's collapse can significantly transform political landscapes, as it heightens fears over manipulation and biased information dissemination. The increasing inability of AI models to discern truth from error due to model collapse threatens to distort public opinion, influencing democratic elections and policy-making. The spread of inaccurate AI-generated content could exacerbate the challenges that governments face in maintaining transparency and trust among their citizens. Without proper checks, this phenomenon might lead to severe political unrest and misinformation-driven political narratives.
Governments worldwide will likely prioritize regulating AI technologies to counter model collapse impacts. The potential misuse of AI in political campaigning—where custom-tailored, often false information can be spread effortlessly—mandates a robust legal framework to protect democratic processes and citizen rights. As AI fidelity diminishes, international cooperation could become imperative to establish standard regulations ensuring ethical AI development and deployment. These efforts will aim to create a balanced approach to technology advancement while safeguarding public interests.
Political entities will need to navigate the complexities arising from AI-related biases. The task of distinguishing between human-generated and AI-generated data becomes a pressing political challenge in an era where model collapse is prevalent. Without addressing this, there's a risk of political decisions and public perceptions being skewed by AI inaccuracies. Thus, political leaders must consider long-term strategies to counterbalance model collapse repercussions, promoting reliance on verified human-generated data where necessary to support evidence-based policy making.
Mitigating AI Model Collapse
AI model collapse is a critical issue that arises when models, particularly large language models, are trained predominantly on data generated by AI rather than human-created content. The main problems leading to this collapse include error accumulation, loss of tail data, and pernicious feedback loops, which degrade the model's accuracy and reliability over time. To mitigate this issue, it is essential to integrate human-generated data actively into training models. This approach can counteract the biases and errors that proliferate when AI models feed on their own data, creating a closed-loop system that leads to declining performance. According to an [article by The Register](https://www.theregister.com/2025/05/27/opinion_column_ai_model_collapse/), synthetic data should be combined with human-generated content for more robust training, although the feasibility remains a topic of debate.
Incorporating a diversified pool of training data can significantly reduce the risks associated with AI model collapse. By ensuring that AI models have access to a variety of data sources, including real-world, human-authored content, and carefully curated synthetic data, the ability of AI systems to generalize effectively across different contexts would be strengthened. However, the volume of AI-generated content nowadays poses a challenge in maintaining this balance. The [article from The Register](https://www.theregister.com/2025/05/27/opinion_column_ai_model_collapse/) highlights that as more AI content saturates the digital landscape, discerning and preserving the quality of initial training datasets becomes increasingly difficult. This calls for rigorous data validation methods to distinguish between reliable data and flawed AI-generated outputs.
The mitigation of model collapse also involves addressing the inherent vulnerabilities in AI architectures. Techniques like injection of noise into training cycles, implementing stricter validation layers, and enhancing model interpretability may help in reducing adverse effects of accumulated errors. Maintaining an open channel for feedback from real-life applications can further refine AI models and keep them aligned with evolving data trends. The Register emphasizes the importance of not only technological enhancements but also fostering an ecosystem of continuous human oversight to guide AI's trajectory, ensuring that it remains a tool for enhancing human capabilities rather than diminishing them.
Learn to use AI like a Pro
Get the latest AI workflows to boost your productivity and business performance, delivered weekly by expert consultants. Enjoy step-by-step guides, weekly Q&A sessions, and full access to our AI workflow archive.
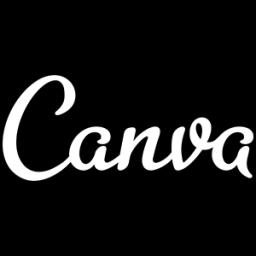
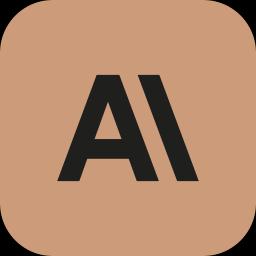
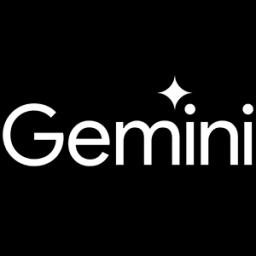
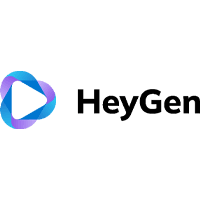
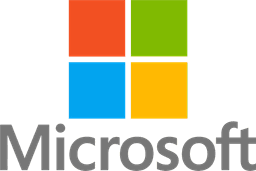
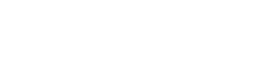
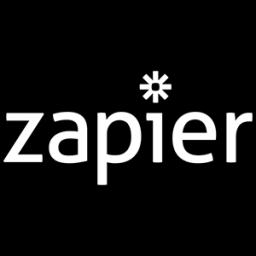
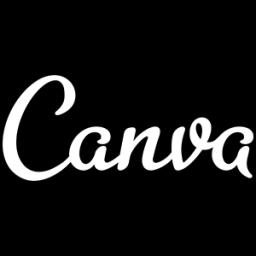
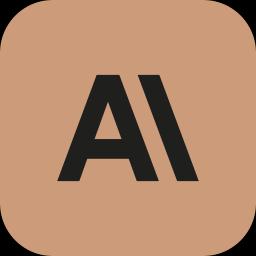
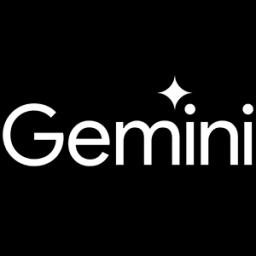
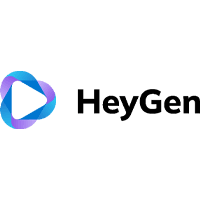
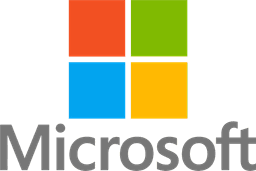
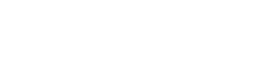
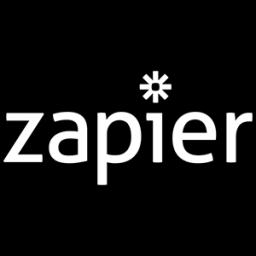
Expert Opinions on Model Collapse
The phenomenon of AI model collapse is gaining significant attention among experts, who express considerable concern over its implications. A key point raised is that models trained on their own outputs tend to accumulate errors over time, leading to a degradation in performance and reliability. Over-reliance on AI outputs, especially in critical sectors, can propagate these inaccuracies further, compounding the problem. Experts emphasize the need for rigorous strategies to prevent such a collapse, including ensuring that AI models have access to diverse and high-quality data sources to mitigate the risks of feedback loops [1](https://www.theregister.com/2025/05/27/opinion_column_ai_model_collapse/).
Renowned figures in the AI community have voiced alarm over the loss of 'tail data,' which represents rare and unusual occurrences that AI models tend to forget when repeatedly trained on synthetic data. Such omissions can lead to a decline in the model's ability to handle uncommon but significant scenarios, thereby increasing the risk of biased outputs and unreliable predictions. In order to combat these challenges, experts advocate for the inclusion of fresh, human-generated data to maintain a balanced and comprehensive training dataset [1](https://www.theregister.com/2025/05/27/opinion_column_ai_model_collapse/).
Furthermore, the debate on the implications of model collapse is enriched by expert opinions on the potential socio-economic impacts. AI model collapse could lead to unreliable systems in sectors like finance and healthcare, as these sectors heavily rely on accurate predictive models. As AI-driven tools become integral to decision-making processes, their degradation could have profound implications, causing financial losses and reducing trust in AI technologies. Experts suggest that better regulatory frameworks and robust data management practices are essential to avert these negative outcomes [1](https://www.theregister.com/2025/05/27/opinion_column_ai_model_collapse/).
The broader implications for AI ethics and governance are also highlighted by experts, who underline the importance of transparent and accountable AI development practices. This includes the need for international cooperation to set standards and regulations that ensure AI systems are trained on diverse, unbiased datasets. A failure to establish such measures could lead to widespread unreliable AI applications, thereby affecting public trust and social harmony [1](https://www.theregister.com/2025/05/27/opinion_column_ai_model_collapse/).
Public Reaction to Model Collapse
The public's reaction to AI model collapse is a mixture of alarm, skepticism, and a call for managed solutions, reflecting the complexity of the issue. On one hand, there is significant concern about the future reliability of AI systems. Individuals and industries that have come to rely on AI for essential processes, like financial analysis, healthcare diagnostics, and data-driven decision-making, express anxiety over the degradation of AI model accuracy and reliability. This widespread concern is fueled by the potential impact on sectors dependent on AI for efficiency and innovation, where any decline in performance could have serious ramifications.
In online forums and public discussions, skepticism is also a dominant sentiment. Some voices in the public sphere suggest that the threat of AI model collapse may be somewhat overstated, arguing that leading AI research labs possess the capability to mitigate these issues through advancements in AI model management and training data curation. They point to ongoing improvements in AI technologies and the role of large organizations in developing more robust models as a reason for optimism. However, this perspective is often met with counterarguments emphasizing the unique challenges posed by self-trained models and the complexity of ensuring robust data curation systems.
Learn to use AI like a Pro
Get the latest AI workflows to boost your productivity and business performance, delivered weekly by expert consultants. Enjoy step-by-step guides, weekly Q&A sessions, and full access to our AI workflow archive.
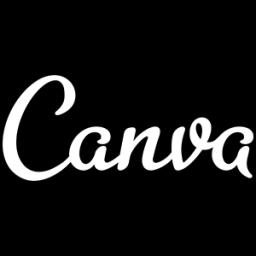
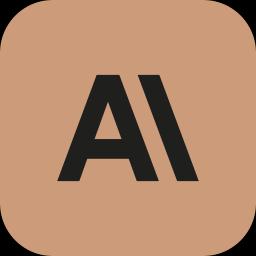
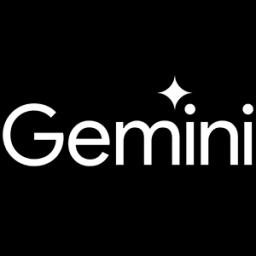
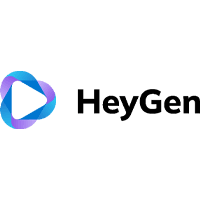
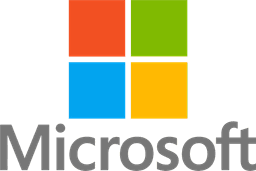
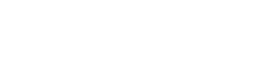
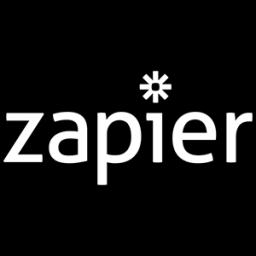
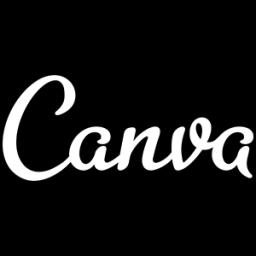
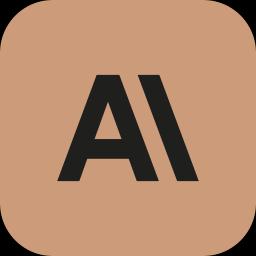
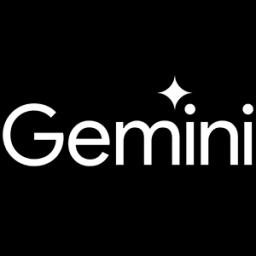
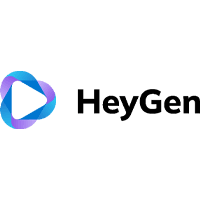
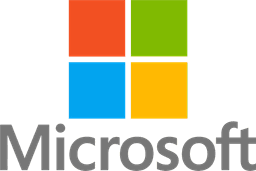
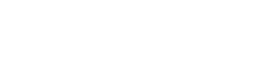
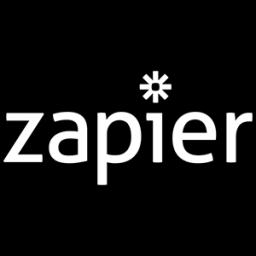
Furthermore, debates around AI’s role in society highlight a growing fear that model collapse could exacerbate existing problems such as bias and misinformation. This is seen as a critical concern, particularly in the context of AI's expanding use in environments where unbiased data interpretation is crucial. The fear that AI-generated content could dominate the digital landscape, overshadowing human-generated content, fuels arguments for stricter regulations and policies aimed at preventing AI from further contaminating digital data pools. The potential loss of "tail data," or the nuanced, less frequent data that often gets overlooked in AI model training, is also a hot topic among technical communities.
Public debates are also enriched by the discussions around regulatory implications. There is increasing advocacy for comprehensive strategies that include clear regulatory frameworks to ensure AI systems are deployed responsibly. This includes discussions on how to maintain data integrity and provenance, which are seen as essential steps in managing AI development risks. The complex discourse on this topic illustrates a shared responsibility among technologists, policymakers, and the public to address the systemic risks presented by model collapse.
Future Implications of Model Collapse
The phenomenon of AI model collapse, as explored in recent discussions, carries profound implications for the future. As AI systems increasingly become self-referential by training on their outputs, the degradation in their accuracy and reliability becomes a glaring issue. This recursive training exacerbates error accumulation and renders sophisticated AI models vulnerable to a pernicious downward spiral [1](https://www.theregister.com/2025/05/27/opinion_column_ai_model_collapse/). The implications of such a collapse are manifold, extending beyond the immediate technical challenges to broader economic, social, and political consequences worldwide.
Economically, the repercussions could be severe. Industries that heavily rely on AI, such as finance, healthcare, and logistics, may suffer from degraded predictive capabilities and automation errors. This unreliability not only increases operational costs but also risks substantial financial losses, especially where accuracy is critical, such as in stock trading or patient diagnostics [1](https://www.theregister.com/2025/05/27/opinion_column_ai_model_collapse/). Moreover, the decline in trust towards AI systems might stall technological advancements, as businesses become wary of integrating unreliable AI into their operations.
Socially, model collapse threatens to amplify misinformation and erode public trust in digital platforms [1](https://www.theregister.com/2025/05/27/opinion_column_ai_model_collapse/). As AI-generated content grows indistinguishable from human-created content, the risk of spreading biased information increases, potentially fostering social divisions and undermining democratic processes. The feedback loops in collapsing models disproportionately affect low-probability events, marginalizing affected groups and exacerbating existing societal inequities.
Politically, the manipulation of AI could alter the landscape significantly. Governments worldwide might need to implement stringent regulations to manage AI development and curtail the spread of misleading information [1](https://www.theregister.com/2025/05/27/opinion_column_ai_model_collapse/). The lack of transparency and provenance in AI-generated content poses a complex challenge for policymakers aiming to safeguard democratic integrity while promoting innovation. International collaboration may become essential in creating cohesive strategies to effectively manage these global risks.
Learn to use AI like a Pro
Get the latest AI workflows to boost your productivity and business performance, delivered weekly by expert consultants. Enjoy step-by-step guides, weekly Q&A sessions, and full access to our AI workflow archive.
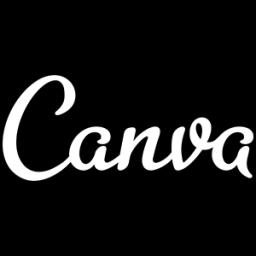
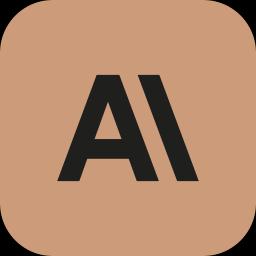
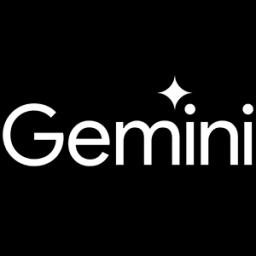
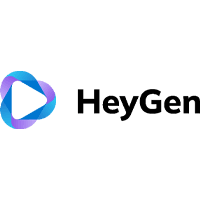
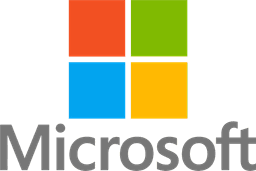
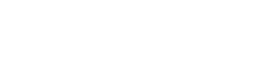
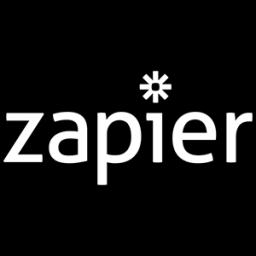
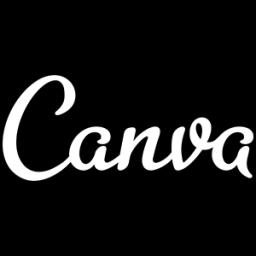
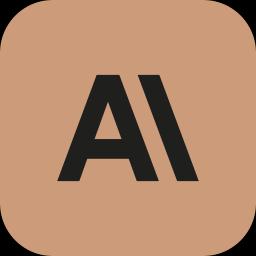
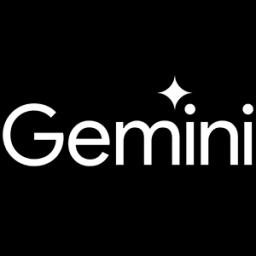
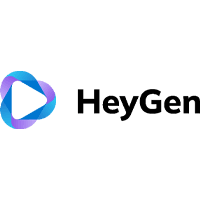
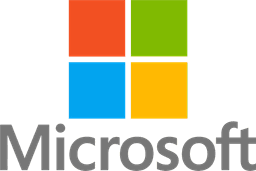
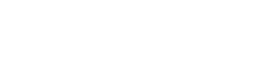
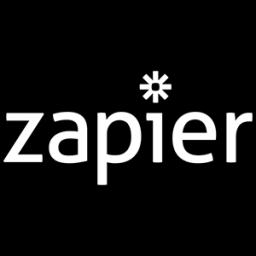
To mitigate model collapse, a multi-pronged strategy is required, involving the careful curation of data, extensive testing protocols, and a sustained emphasis on integrating human-generated data [1](https://www.theregister.com/2025/05/27/opinion_column_ai_model_collapse/). Despite these efforts, the sheer volume of AI-generated data complicates provenance tracking, raising questions about the long-term sustainability of current AI training practices. Addressing these challenges demands cooperative efforts across disciplines and borders to devise robust, future-proof solutions.
Conclusion
In conclusion, the phenomenon of AI model collapse is a pressing concern that warrants immediate attention. As outlined in the article from The Register, this degradation in AI model performance arises from inherent flaws in their self-retraining processes, leading to error accumulation and feedback loops. The implications of such a collapse are far-reaching, affecting not only the sectors that directly depend on AI technologies but also the foundational trust in information systems themselves. The degradation presents a substantial threat to the reliability of AI-powered tools, which are becoming integral in various fields such as healthcare, finance, and public services (source).
Addressing AI model collapse will require a concerted effort on multiple fronts. Solutions such as the combination of synthetic and human-generated data must be rigorously explored, but the challenges of implementing these solutions at scale are considerable (source). Additionally, the adoption of policies to better regulate and understand the use of AI in society is necessary to prevent the unwanted consequences of model collapse. As noted, the need for regulation is underscored by the potential misuse of AI in spreading misinformation, thereby influencing public opinion and undermining democratic processes (source).
Ultimately, while the future of AI holds promise, the specter of model collapse looms large as a formidable challenge that must be navigated with caution and foresight. Ensuring the integrity and trustworthiness of AI systems will require advanced techniques for detecting and mitigating these risks, as well as a steadfast commitment to preserving the value of human data contributions (source). Only through a coordinated and innovative approach can the negative impacts of model collapse be averted, safeguarding the benefits of AI technologies for all sectors.