Taming the AI Model Jungle
AI Model Explosion: Navigating the New Frontier
Last updated:

Edited By
Mackenzie Ferguson
AI Tools Researcher & Implementation Consultant
Discover the challenges behind evaluating the booming AI model landscape, where popular models like Gemini 2.5 Pro and GPT-4o are pushing boundaries. Explore the gap between benchmarks and real-world applications, and the ethical dilemmas shaping AI's future.
Introduction to the Rapid Growth of AI Models
The rapid evolution and proliferation of AI models are reshaping the landscape of technology, influencing everything from daily operations to strategic planning in organizations worldwide. Central to this growth are models like Gemini 2.5 Pro Experimental, GPT-4o, and Meta's Llama 3.3, each contributing uniquely to the field with diverse features and capabilities. As these models advance, they promise enhanced reasoning, creativity, and problem-solving skills, yet they also bring challenges in terms of evaluation, as traditional benchmarks often fail to capture their full spectrum of utility in real-world scenarios. As noted in a TechCrunch article, the gap between technical performance indicators and practical applications remains a significant hurdle, challenging developers to create models that truly reflect user needs.
The market for AI solutions is buoyed by an ongoing quest for models that not only excel in speed and efficiency but also demonstrate robust decision-making capabilities. However, as this technological wave swells, ethical concerns such as data privacy, potential misuse, and the infamous "hallucination" phenomenon are growing louder. These potential pitfalls necessitate comprehensive regulations and governance frameworks designed to harness AI's benefits while mitigating risks. The scrutiny over these models reflects society's broader struggle to align AI capabilities with ethical standards, as highlighted by the ARC-AGI-2 test, which addresses earlier benchmark flaws by emphasizing pattern interpretation over mere data processing.
Learn to use AI like a Pro
Get the latest AI workflows to boost your productivity and business performance, delivered weekly by expert consultants. Enjoy step-by-step guides, weekly Q&A sessions, and full access to our AI workflow archive.
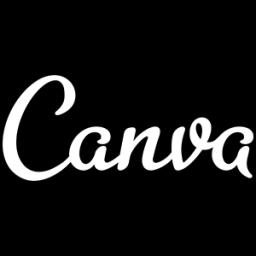
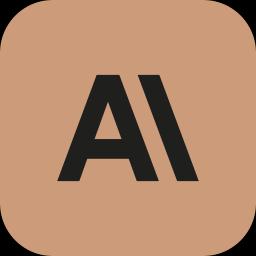
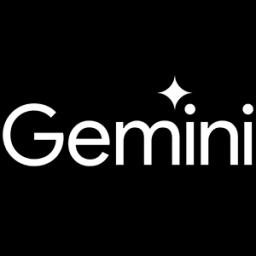
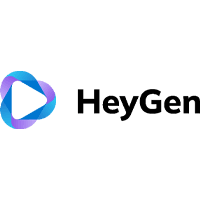
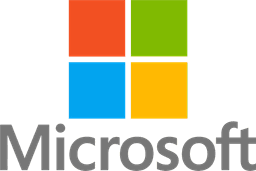
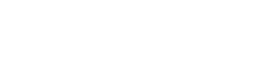
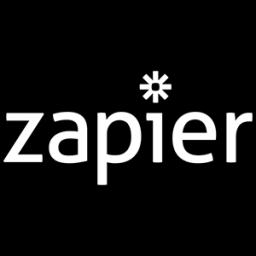
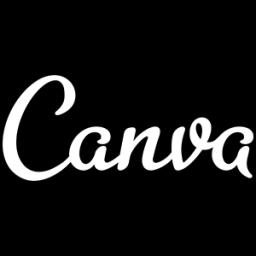
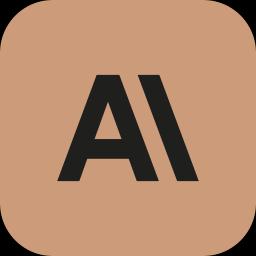
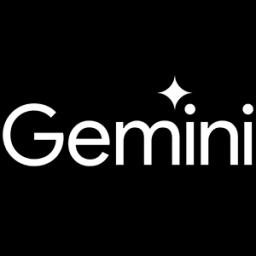
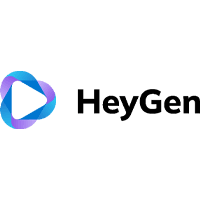
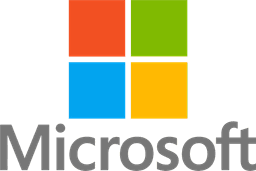
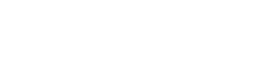
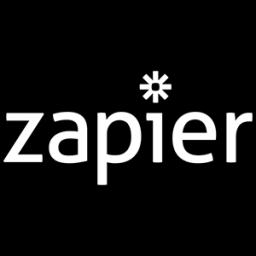
Amid the challenges, AI continues to capture the imagination and optimism of the tech industry and the public alike. Releases like Google's Gemini 2.5 Pro Experimental inject excitement into the sector, promising new levels of cognitive processing and potential applications across various domains. This excitement is tempered by a necessary caution, as experts emphasize the complexity involved in evaluating and deploying these models. According to Anthropic, the subjectivity and potential biases in human evaluations and third-party frameworks must be carefully managed to ensure fairness and transparency. As AI models become increasingly central to global innovation, their development underscores the importance of responsible and strategic application.
Evaluation Challenges: Benchmarks vs. Real-World Applications
Evaluating AI models poses significant challenges due to the gap between technical benchmarks and real-world applications. Benchmarks are intended to provide a standardized way of assessing the performance of AI models. However, they often fail to capture the nuanced needs and unpredictable nature of real-world tasks. This misalignment means that a model excelling in benchmark tests, like Google's Gemini 2.5 Pro or OpenAI's GPT-4o, might underperform when faced with complex, real-world problems [1](https://www.bundle.app/en/technology/cad4fc73-5f9c-4cc0-9e6b-c4defc58defc?l=1).
This disparity presents a critical challenge: determining the most suitable model for specific real-world applications becomes complicated. As highlighted by Epoch AI, benchmarks tend to focus on a model's improvement on predefined tasks rather than its ability to navigate real-world economic complexities [2](https://techcrunch.com/2025/03/24/a-new-challenging-agi-test-stumps-most-ai-models/). This issue is compounded by the fact that many existing benchmarks test models' abilities to memorize information rather than to apply reasoning in varied, unpredictable contexts, as noted by NowNextLater.AI [3](https://www.nownextlater.ai/Insights/post/ai-benchmarks-misleading-measures-of-progress-towards-general-intelligence).
To address these challenges, innovations like the ARC-AGI-2 benchmark test have been developed to better evaluate AI systems' efficiency and pattern recognition capabilities in more complex environments. By focusing on how models interpret and apply information dynamically, rather than rote memorization, these tests aim to bridge the gap between benchmark assessments and practical performance [2](https://techcrunch.com/2025/03/24/a-new-challenging-agi-test-stumps-most-ai-models/). Such developments are crucial in improving the relevance and reliability of AI evaluations in real-world contexts.
Learn to use AI like a Pro
Get the latest AI workflows to boost your productivity and business performance, delivered weekly by expert consultants. Enjoy step-by-step guides, weekly Q&A sessions, and full access to our AI workflow archive.
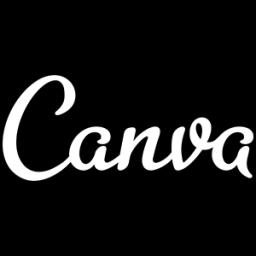
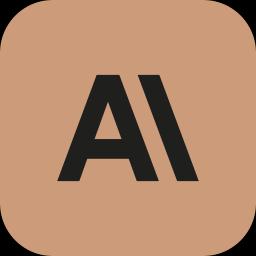
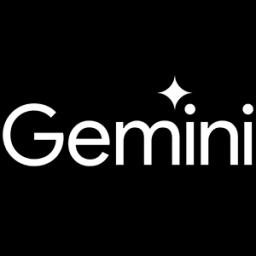
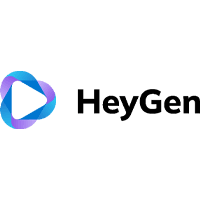
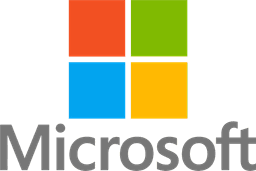
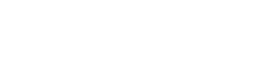
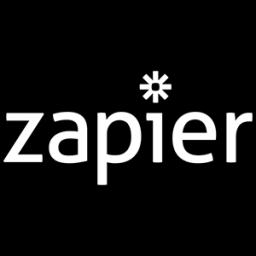
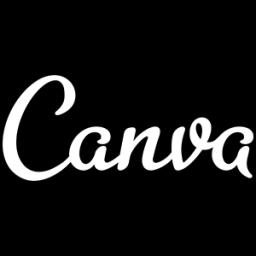
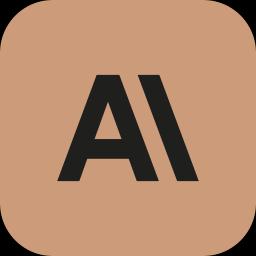
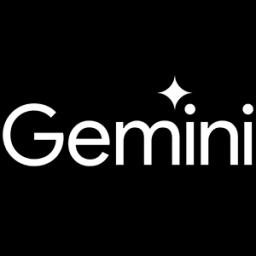
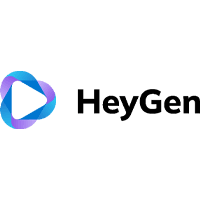
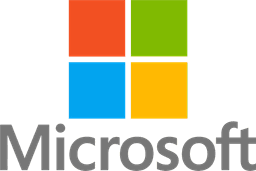
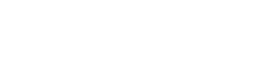
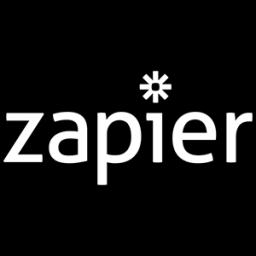
The ethical and technical implications of this evaluation gap are significant. As AI continues to integrate into key areas like healthcare and finance, the ability to accurately gauge AI performance beyond standardized tests becomes critical. Without reliable evaluation methods, there's a risk of deploying AI systems that could make incorrect decisions, potentially leading to negative social and economic impacts. Hence, there's a need for ongoing dialogue and collaboration among technologists, policymakers, and ethicists to develop more holistic evaluation frameworks [1](https://www.bundle.app/en/technology/cad4fc73-5f9c-4cc0-9e6b-c4defc58defc?l=1), [3](https://www.nownextlater.ai/Insights/post/ai-benchmarks-misleading-measures-of-progress-towards-general-intelligence).
Prominent AI Models: Features and Availability
Prominent AI models have become pivotal in shaping the technological landscape, with different models offering unique features tailored to specific needs. Among these, Google's Gemini 2.5 Pro Experimental stands out with its advanced reasoning and coding capabilities, reflecting a significant milestone in AI development. As detailed in a TechCrunch article, Gemini 2.5 not only improves on previous versions through enhanced performance metrics but also aims to bridge the gap between technical benchmarks and practical, real-world applications.
In contrast, OpenAI's GPT-4o brings a different set of strengths to the AI arena, emphasizing user-friendliness and accessibility. This model doesn't just cater to developers and tech-savvy users; it's available for general use, allowing more people to leverage its capabilities for diverse tasks. Its availability and performance, also highlighted in the TechCrunch article, make it a popular choice for educators, businesses, and hobbyists alike. However, despite its advanced features, challenges remain in evaluating its effectiveness outside controlled testing environments.
Llama 3.3 by Meta is another key player in the mix, notable for its open-source nature which distinctly contributes to the model's adaptability and growth. As discussed, its free availability helps lower the entry barrier for individuals and smaller organizations keen on experimenting with AI technology without substantial financial investment. Yet, this accessibility also underscores the ongoing debate around AI ethics, as open-source models can potentially be misused if not properly regulated.
The availability of these models for free or at minimal cost, at least for limited usage, democratizes AI technology, offering invaluable opportunities for learning and innovation. This is particularly crucial as users increasingly demand models that extend beyond simple data processing to include nuanced reasoning and decision-making capabilities. While technical benchmarks provide a framework for measurement, as noted in , they often fail to encompass the real-world challenges these models face, hence the ongoing push for better evaluative tools and methodologies.
Navigating Free and Subscription-based AI Models
Navigating the landscape between free and subscription-based AI models requires a nuanced understanding of their differing functionalities and constraints. While free AI models such as Cohere's Aya Vision and Meta's Llama 3.3 offer initial access without financial commitment, they often include limitations in terms of usage volume and advanced features. These constraints can restrict their applicability in more demanding environments where robust, continuous performance is crucial. On the other hand, subscription-based models typically provide enhanced capabilities, including higher processing power, reliability, and advanced features tailored for specific needs. For instance, models like Google's Gemini 2.5 Pro Experimental are valued for their enhanced reasoning and coding capabilities, as highlighted in their recent release announcement [TechCrunch](https://www.bundle.app/en/technology/cad4fc73-5f9c-4cc0-9e6b-c4defc58defc?l=1).
Learn to use AI like a Pro
Get the latest AI workflows to boost your productivity and business performance, delivered weekly by expert consultants. Enjoy step-by-step guides, weekly Q&A sessions, and full access to our AI workflow archive.
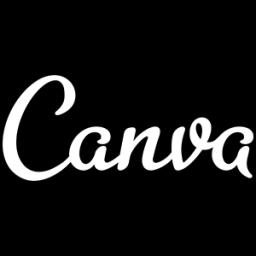
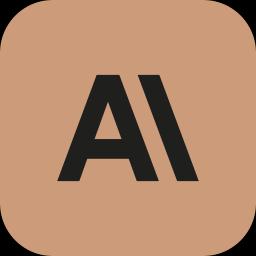
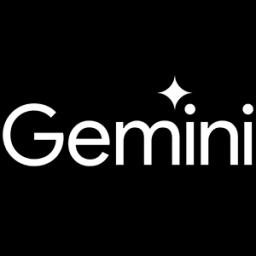
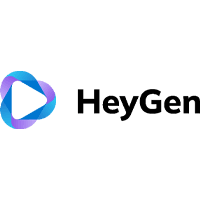
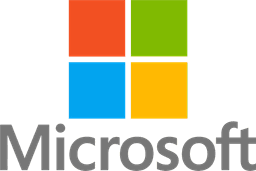
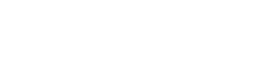
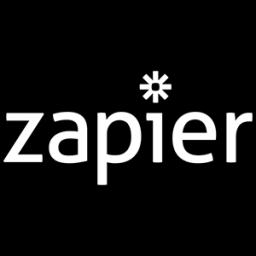
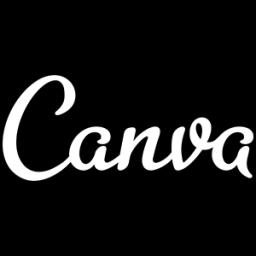
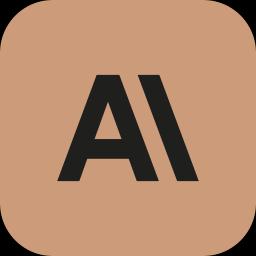
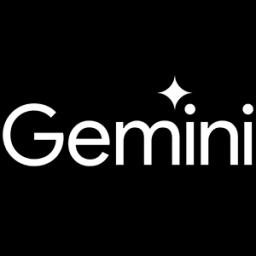
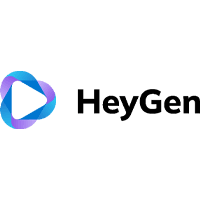
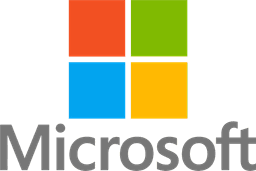
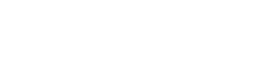
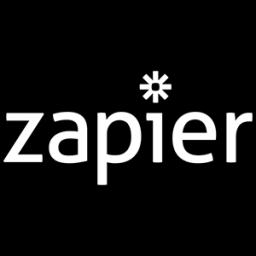
The choice between utilizing free or subscription-based AI models often hinges on the specific requirements and objectives of the user, whether an individual researcher or a large enterprise. Free versions might be sufficient for experimentation and learning, providing an opportunity to explore AI capabilities without upfront costs. However, as the complexity of tasks increases, these free models might fall short in delivering the needed performance and precision, driving the consideration of subscription models. For businesses, investing in a subscription-based model could translate into tangible benefits, such as improved decision-making processes, streamlined operations, or innovative product development. This decision-making process is further complicated by evolving benchmarks and the real-world applicability of AI models, as discussed in various expert opinions [Epoch AI](https://epoch.ai/gradient-updates/the-real-reason-ai-benchmarks-havent-reflected-economic-impacts).
Moreover, ethical considerations and potential misuse of AI must inform decisions around AI model adoption. Free AI models can be more accessible and potentially exploited for illicit purposes due to their availability. This raises concerns about data privacy and security, echoing broader public debates about the responsible use of technology. Subscription models, while offering more control and support, still face challenges around ethical AI usage and "hallucinations," or factual inaccuracies, an issue not entirely addressed by techniques like Retrieval-Augmented Generation (RAG). RAG attempts to enhance AI model accuracy by improving information retrieval, yet it does not completely mitigate the risks associated with AI-generated misinformation [TechCrunch](https://www.bundle.app/en/technology/cad4fc73-5f9c-4cc0-9e6b-c4defc58defc?l=1).
Understanding Reasoning Models in AI
Reasoning models in AI represent a pivotal advancement in artificial intelligence development, offering a shift from traditional rapid-response capabilities to more sophisticated, deliberate thinking processes. Unlike conventional AI models that may generate surface-level solutions, reasoning models strive to simulate human-like thought patterns, analyzing problems step-by-step. This methodical approach aims to tackle complex tasks requiring a deeper understanding of context and nuance. Notable examples of reasoning models include Google's Gemini 2.5 Pro Experimental and Anthropic's Claude Sonnet 3.7, celebrated for their ability to emulate a more comprehensive problem-solving strategy, thereby extending AI's applicability to more intricate scenarios. However, it's important to acknowledge that despite their promising capabilities, reasoning models still encounter limitations and challenges in practical applications, particularly concerning ethical considerations and "hallucinations," or factual inaccuracies .
The development of reasoning models within AI signifies a response to the demand for more reliable and insightful artificial cognitive processes. As industries increasingly rely on AI systems, the necessity for models that can reason and provide contextually rich insights has become paramount, especially for decision-making processes that involve complex variables and unpredictability. One of the main issues with existing AI models is their reliance on benchmarks that do not necessarily translate well into real-world scenarios. The mismatch between testing environments and actual application needs, as discussed in the TechCrunch article, highlights the need for AI systems like reasoning models to bridge this gap, enabling more pragmatic and impactful integration of AI technologies in business and societal contexts .
Reasoning models are ushering in a new era of AI by focusing on the quality and depth of generated outputs rather than sheer speed. This evolution is informed by growing recognition of the importance of artificial systems capable of foundational reasoning. The development and release of Google's Gemini 2.5 Pro Experimental, for instance, have set benchmarks for what AI reasoning can achieve, while also showcasing inherent challenges such as "hallucinations" and potential ethical dilemmas like data privacy and copyright concerns. These challenges emphasize the need for balanced, well-regulated AI policies that support the ethical growth and integration of advanced reasoning models across sectors . The continued development of such models, however, is critical for advancing AI's capacity to solve more profound societal issues that require nuanced understanding and thoughtful intervention.
Ethical Concerns and Limitations of AI Models
As artificial intelligence models become increasingly prevalent, ethical concerns and limitations consistently arise, highlighting the importance of responsible development and application. Central to these concerns is the issue of copyright infringement, where AI's capability to generate new content could unintentionally replicate or alter copyrighted materials. This presents a significant challenge, as legal frameworks are often slow to adapt to technological advancements, thus necessitating robust policies to protect creative rights. Moreover, the rapid release and development cycles of AI models like Google's Gemini 2.5 Pro Experimental and Meta's Llama 3.3 put pressure on regulators to keep up with both innovation and ethical practices on a global scale.
Learn to use AI like a Pro
Get the latest AI workflows to boost your productivity and business performance, delivered weekly by expert consultants. Enjoy step-by-step guides, weekly Q&A sessions, and full access to our AI workflow archive.
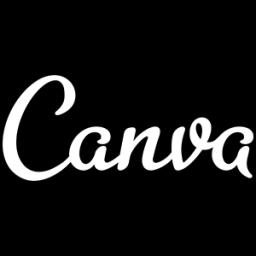
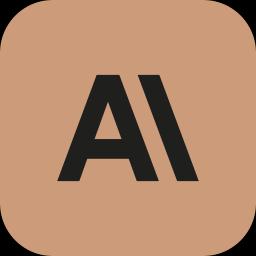
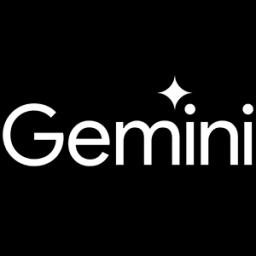
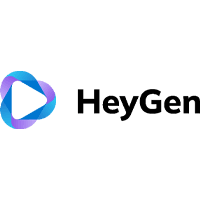
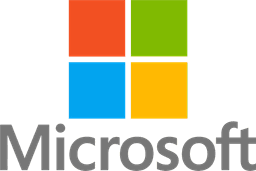
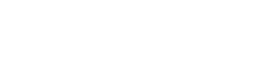
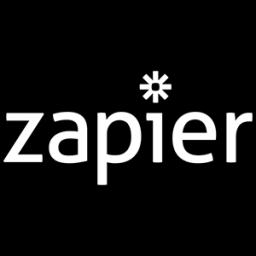
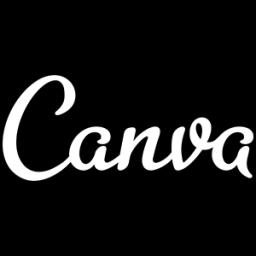
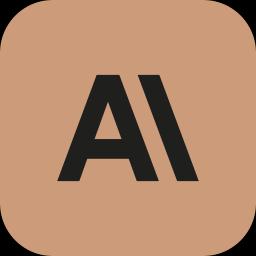
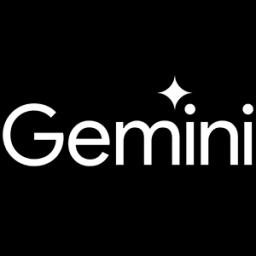
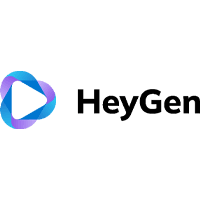
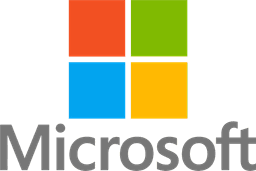
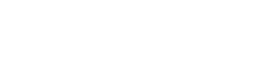
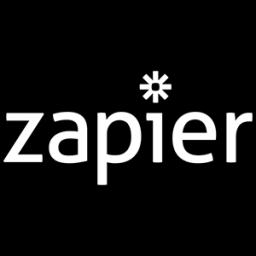
While AI models promise unprecedented advancements in efficiency and capability, they also pose significant risks to data privacy. As these systems require vast amounts of data to function and improve, the potential for misuse or unauthorized access increases. This is a growing concern among both developers and end-users, who are often wary of how their data may be used beyond its intended scope. In this context, regulatory frameworks must evolve to ensure that user data is handled with utmost transparency and security. Furthermore, the issues of censorship and AI 'hallucinations', which refer to factual inaccuracies produced by AI, are troubling for those relying on machine-generated content for decision-making. These limitations underscore the need for continuous improvement in training and developing models that can manage complex real-world scenarios more accurately.
Moreover, ethical considerations must address the impact of AI on job markets and societal structures. The rise of models equipped with reasoning capabilities, such as Anthropic's Claude Sonnet 3.7 and Google's Gemini 2.5 Pro Experimental, can feasibly substitute human roles in certain industries, leading to economic shifts. While AI can enhance productivity, it also has the potential to displace jobs, suggesting a need for proactive measures, such as retraining and upskilling programs, to mitigate adverse economic impacts. Accordingly, ethical AI deployment should inclusively consider societal well-being, ensuring that technologies advance without exacerbating inequalities or creating new socio-economic divides.
Failures in adequately evaluating AI models pose another ethical limitation. Traditional benchmarks frequently fail to capture the nuances of AI performance in real-world applications, leading to a discrepancy between expected and actual outcomes. As reported by Epoch AI, benchmarks are often not designed to reflect complex economic impacts, but are rather aimed at simpler task improvements, thus skewing perceived progress. To address this, there is a pressing need for novel evaluation methods that align more closely with practical applications rather than theoretical performance. Initiatives like ARC-AGI-2 seek to overcome these test limitations by focusing on efficiency and pattern interpretation, as outlined by the Arc Prize Foundation. This evolution in evaluation can help bridge the gap between AI capabilities and their societal utility, ensuring models are both effective and ethically sound.
Innovative Solutions: RAG and Addressing Hallucinations
In the rapidly evolving field of artificial intelligence, innovative approaches like Retrieval-Augmented Generation (RAG) are pushing the boundaries of what these technologies can achieve. RAG leverages external data sources to retrieve relevant information, enhancing the AI's capacity to generate more accurate and contextually appropriate responses. This is particularly useful in addressing the issue of 'hallucinations,' where AI models produce incorrect or fabricated information. Integrating RAG into AI systems not only mitigates these inaccuracies but also improves their ability to provide well-rounded and substantiated outputs. For instance, a RAG-enabled AI could cross-verify facts from diverse data repositories before presenting an answer, significantly reducing the likelihood of errors ().
Despite these advancements, RAG is not a panacea for all the challenges posed by AI hallucinations. While the technique considerably improves accuracy, it cannot entirely eliminate the problem. AI models must still contend with the intricacies of context and semantics, aspects that are not easily captured by retrieval processes. Furthermore, the reliance on available data poses its own risks; if the data itself is flawed, the output will inevitably reflect those imperfections. This highlights the importance of continuous improvements and updates in both AI models and their underlying datasets to ensure reliability and accuracy in real-world applications ().
The implementation of RAG in AI models marks a significant stride towards creating more reliable and user-friendly technologies. It is especially crucial as the industry moves from technical benchmarks towards real-world applicability, where the true test of a model's success lies. As models like Gemini 2.5 Pro Experimental, GPT-4o, and Llama 3.3 demonstrate, the integration of advanced methodologies such as RAG not only addresses current deficiencies but also sets the stage for the next wave of AI innovations that prioritize human-centric applications over abstract technical achievements ().
Learn to use AI like a Pro
Get the latest AI workflows to boost your productivity and business performance, delivered weekly by expert consultants. Enjoy step-by-step guides, weekly Q&A sessions, and full access to our AI workflow archive.
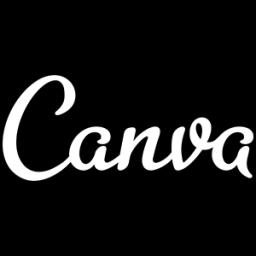
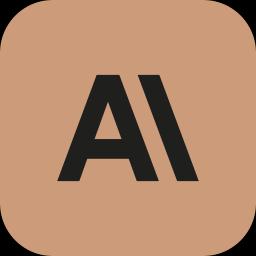
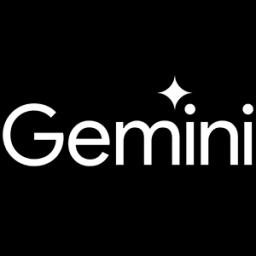
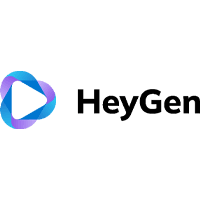
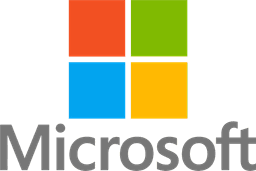
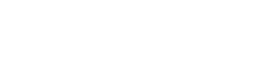
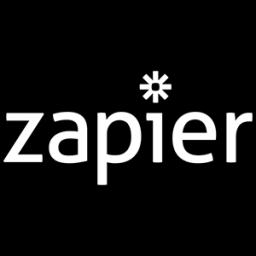
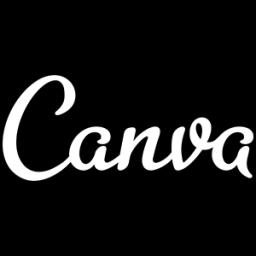
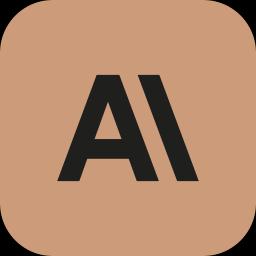
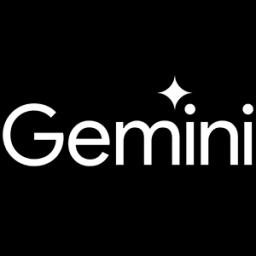
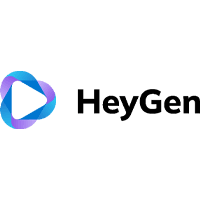
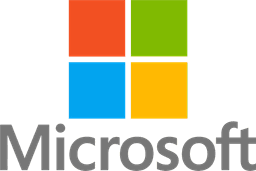
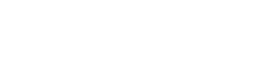
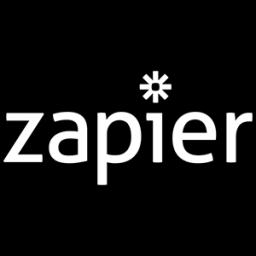
Key Developments: Google's Gemini 2.5 Pro Experimental
Google's release of the Gemini 2.5 Pro Experimental represents a significant milestone in the world of AI, demonstrating enhanced reasoning and coding abilities. This model is a part of Google's broader efforts to advance the capabilities of AI systems, particularly those that can reason through problems methodically rather than just generating quick responses. Such developments are vital as they align more closely with human-like problem-solving methods. The enhancements in Gemini 2.5 Pro also underscore Google's commitment to bridging the gap between technical advancement and practical usability, addressing one of the critical challenges highlighted in AI development. For more details, check out the full release on Google’s blog .
The launch of Gemini 2.5 Pro Experimental marks a leap forward from previous models in terms of both functionality and real-world application. This model is designed to tackle complex reasoning tasks, offering solutions that are not only technically sound but also applicable in everyday scenarios. The refinement in its processing capabilities allows it to better understand and interpret the nuances of human language and coding, making it a valuable asset for businesses and developers looking to integrate robust AI solutions into their operations. Such developments reflect the ongoing efforts to tailor AI models that meet the diverse needs of various industries, enhancing productivity and innovation in the process.
Gemini 2.5 Pro Experimental is also a testament to the rapid pace of innovation in AI technology. As highlighted in recent developments, there is a burgeoning need to evaluate these models not just based on technical benchmarks but how they perform under real-world conditions. The model's release comes in the wake of growing acknowledgment among tech companies that evaluating AI requires a more comprehensive approach. This includes considering factors like ethical implications, practical usability, and potential biases in decision-making processes. For further insights on the broader implications of this model, explore how it compares with other experimental models on TechCrunch .
The development of Gemini 2.5 Pro Experimental is aligned with the latest trends in AI, where there is a distinct focus on enhancing models to address complex and nuanced challenges in various fields. With its introduction, Google aims to push the boundaries of what AI can achieve, particularly in reasoning and adaptive learning. This model not only highlights the technological advances but also ignites discussions about the ethical aspects of AI deployment, especially considering its potential impact on industries. Such advancements need to be balanced with responsible practices and robust regulation to ensure that AI's growth benefits society as a whole.
Addressing Benchmark Flaws: ARC-AGI-2 Test
The ARC-AGI-2 test, developed by the Arc Prize Foundation, shines a spotlight on the persistent challenges facing benchmark evaluations of artificial intelligence. Unlike traditional benchmarks that often fall short in measuring real-world applicability, ARC-AGI-2 aims to assess AI models on their ability to efficiently interpret patterns—a task more aligned with actual scenarios these models encounter in everyday applications. Such benchmarks are crucial, as they strive to bridge the gap between an AI's technical prowess and its effectiveness in practical situations [2](https://techcrunch.com/2025/03/24/a-new-challenging-agi-test-stumps-most-ai-models/).
The reliance on benchmarks to evaluate AI models often obscures their real-world efficacy, leading to a disconnect that ARC-AGI-2 aims to address. Traditional benchmarks usually test for model improvements on straightforward tasks, lacking the complexity of scenarios encountered in the real world. By incorporating tests that require advanced pattern recognition and problem-solving skills, ARC-AGI-2 seeks to elevate the standards of AI evaluation, aligning it more closely with how these technologies are employed across industries [2](https://techcrunch.com/2025/03/24/a-new-challenging-agi-test-stumps-most-ai-models/).
Learn to use AI like a Pro
Get the latest AI workflows to boost your productivity and business performance, delivered weekly by expert consultants. Enjoy step-by-step guides, weekly Q&A sessions, and full access to our AI workflow archive.
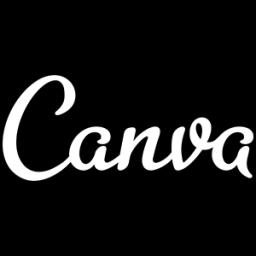
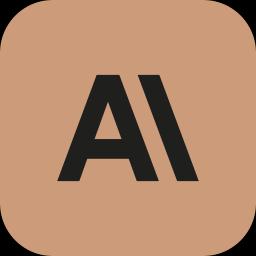
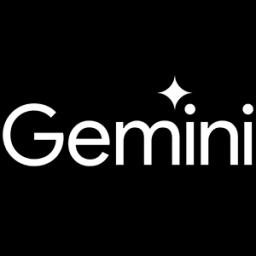
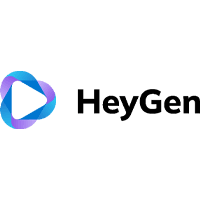
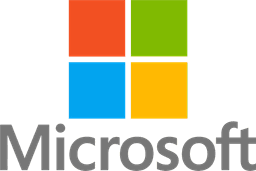
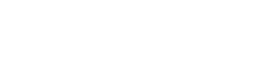
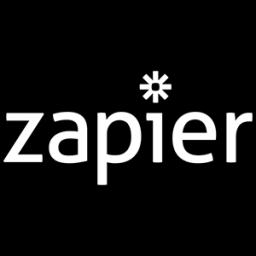
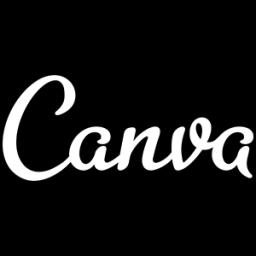
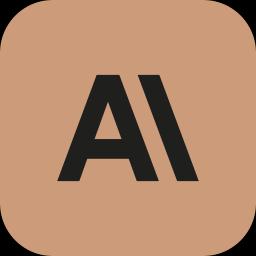
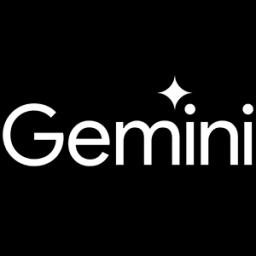
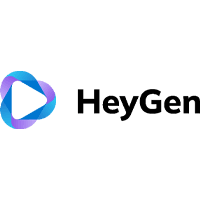
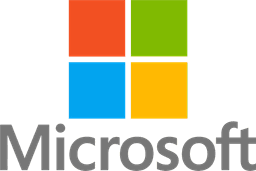
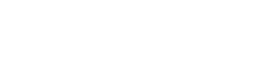
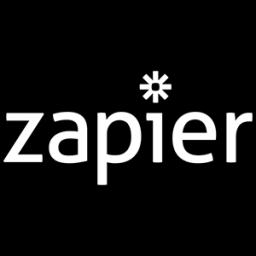
Furthermore, renowned experts like those from Epoch AI and NowNextLater.AI highlight that most benchmarks do not accurately translate to real-world economic impacts. They argue that benchmarks are tailored to facilitate a model's enhancement on basic tasks, rather than reflecting its capacity to handle comprehensive, complex operations seen in various industry applications [2](https://epoch.ai/gradient-updates/the-real-reason-ai-benchmarks-havent-reflected-economic-impacts). This underscores the necessity for more robust and reality-based testing scenarios such as those introduced by ARC-AGI-2.
An essential aspect of addressing benchmark flaws is understanding the limitations imposed by multiple-choice evaluations, which don't always capture the nuances needed for comprehensive assessment. Anthropic and other thought leaders in AI research point out that these types of evaluations, while useful, often fail to account for subjective elements and potential biases inherent in evaluation processes. Therefore, the ARC-AGI-2's focus on pattern recognition and efficiency can better mirror the complexities AI systems navigate in real-world settings [1](https://www.anthropic.com/research/evaluating-ai-systems).
The development and implementation of ARC-AGI-2 are pivotal steps toward refining how AI's capabilities are quantified and perceived. By challenging models to perform tasks that require nuanced understanding and interpretation, it not only sets a new standard for evaluation but also encourages the development of AI that truly resonates with business needs and public expectations. As scrutiny on AI technologies intensifies, tests like ARC-AGI-2 will likely play an increasingly critical role in shaping future AI innovations and ensuring their alignment with societal and ethical standards [2](https://techcrunch.com/2025/03/24/a-new-challenging-agi-test-stumps-most-ai-models/).
Views from Experts on AI Evaluation
In the ever-evolving field of artificial intelligence, expert opinions often highlight the complexities surrounding AI evaluation. The rapid proliferation of AI models like Google's Gemini 2.5 Pro Experimental or Meta's Llama 3.3 underscores the challenges inherent in accurately measuring their real-world applicability. As noted by experts from Anthropic, traditional evaluation methods such as multiple-choice tests or keyword association benchmarks are insufficient. These evaluations frequently fail to capture the full scope of a model's capabilities, leading to discrepancies between benchmark scores and meaningful economic impacts (Source: ). Epoch AI further explains that traditional benchmarks were initially designed to test model improvements on simpler tasks, not to emulate real-world complexity ().
Furthermore, the introduction of reasoning models, designed to tackle problems through step-by-step analysis rather than rapid response generation, exemplifies the innovative avenues AI development is exploring. Experts emphasize the importance of developing evaluation techniques that reflect such sophisticated capabilities. The ARC-AGI-2 benchmark test by the Arc Prize Foundation represents a leap towards such evaluation precision, addressing previous benchmark flaws by focusing on pattern interpretation and efficiency (). However, as AI models grow more sophisticated, developers must confront challenges such as data privacy violations and hallucinations, which continue to hinder their credibility and deployment in sensitive applications. NowNextLater.AI suggests that benchmarks should aim to assess a model's genuine reasoning skills rather than just its memorization capabilities ().
Public Concerns and Reactions to AI Advancements
With the rapid advancement in artificial intelligence over the past few years, public concerns have increasingly come to the forefront. One of the primary issues is the potential misuse of AI technologies for manipulative purposes, such as creating persuasive propaganda or manipulating discourse on social media platforms. This concern is evidenced by the AI's capability to mimic human speech convincingly, raising fears about authenticity in digital communications [source](https://www.rand.org/pubs/articles/2024/social-media-manipulation-in-the-era-of-ai.html). With the development of more sophisticated models like Google's Gemini 2.5 Pro, there is further anxiety about how AI might be employed outside ethical boundaries, necessitating stringent controls and oversight.
Learn to use AI like a Pro
Get the latest AI workflows to boost your productivity and business performance, delivered weekly by expert consultants. Enjoy step-by-step guides, weekly Q&A sessions, and full access to our AI workflow archive.
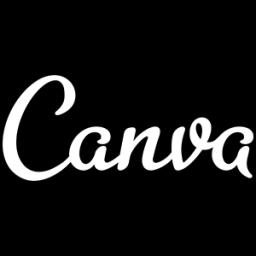
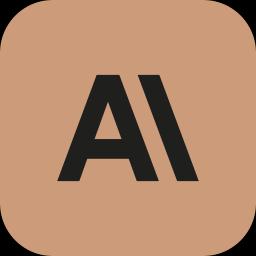
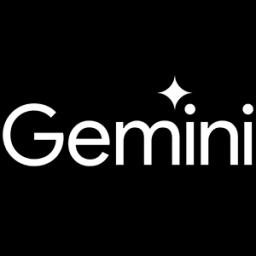
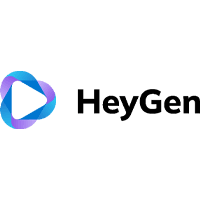
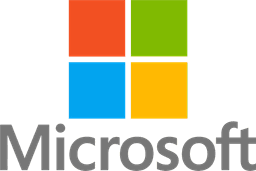
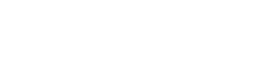
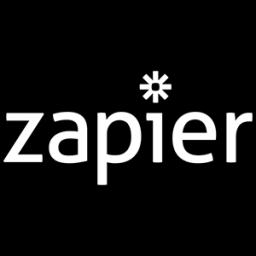
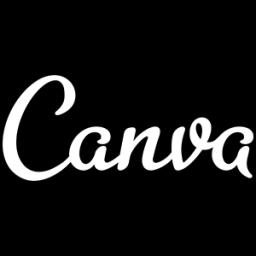
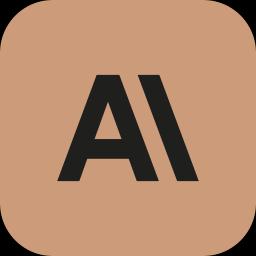
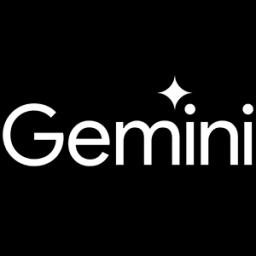
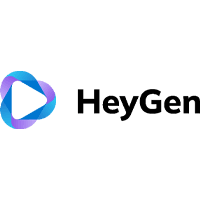
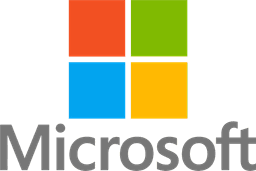
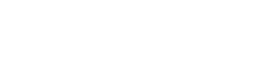
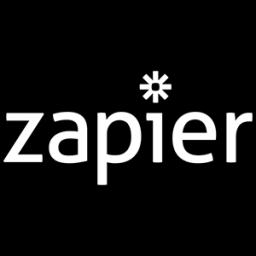
Moreover, the disconnect between technical benchmarks and real-world applications of AI models exacerbates public skepticism. While technical evaluations may indicate progress, they often fail to reflect a model's effectiveness in practical scenarios [source](https://epoch.ai/gradient-updates/the-real-reason-ai-benchmarks-havent-reflected-economic-impacts). This gap creates uncertainty regarding the true capabilities of these models. The public’s wariness is compounded by ongoing challenges in developing robust evaluation methods that match the complexities faced in real-world environments.
Ethical concerns also form a significant part of public discourse surrounding AI advancements. Issues such as data privacy violations, potential for copyright infringement, and the phenomenon of AI "hallucinations"—where models generate inaccurate or nonsensical information—are part of the broader conversation [source](https://techcrunch.com/2025/03/24/a-new-challenging-agi-test-stumps-most-ai-models/). These problems highlight the need for comprehensive ethical guidelines to govern AI development and deployment, to safeguard public interests.
Despite these concerns, there is a palpable interest in the tech community towards new AI model launches. When companies like Google release new iterations, such as the Gemini 2.5 Pro, tech enthusiasts and professionals closely monitor these developments through forums and technology blogs, eager to explore the capabilities these models offer [source](https://www.reddit.com/r/singularity/comments/1jl1eti/man_the_new_gemini_25_pro_0325_is_a_breakthrough/). This fascination with cutting-edge technology underscores the dual nature of public reaction, balancing apprehension with anticipation.
As AI models continue to proliferate, their implications on society and economics become points of serious discussion. The potential for economic shifts, such as job displacement, and productivity gains is evident, driving the call for proactive measures in workforce retraining [source](https://www.calmu.edu/news/future-of-artificial-intelligence). At the same time, the social implications, including the risk of exacerbating biases or threatening data privacy, underscore the urgent need for regulation and ethical consideration in AI deployment [source](https://www.calmu.edu/news/future-of-artificial-intelligence). Politically, these advancements may shape international relations and national security landscapes, further calling for cooperative global efforts.
Future Implications: Economic and Societal Shifts Due to AI
The rapid advancement of artificial intelligence (AI) models is poised to lead to significant economic and societal shifts in the near future. A major implication is the potential for widespread job displacement as AI systems become more capable of performing tasks traditionally completed by humans. Consequently, there may be a push for proactive retraining initiatives to ensure that the workforce can adapt to the new demands and opportunities presented by AI. The economic shifts brought on by AI are not just limited to job displacement; there is also the possibility of increased productivity and efficiency in various sectors, which could lead to economic growth and new job creation. However, this growth must be managed carefully to avoid exacerbating existing inequalities .
Socially, the increased use of AI models raises concerns about exacerbating existing biases and threatening data privacy. The inherent biases present in the datasets used to train AI models could result in systems that reinforce prejudices rather than alleviate them. Moreover, as AI systems become more integrated into daily life, the potential for data breaches and privacy violations increases, necessitating the implementation of stringent regulations and ethical guidelines to protect individual rights , .
Learn to use AI like a Pro
Get the latest AI workflows to boost your productivity and business performance, delivered weekly by expert consultants. Enjoy step-by-step guides, weekly Q&A sessions, and full access to our AI workflow archive.
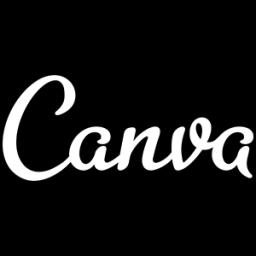
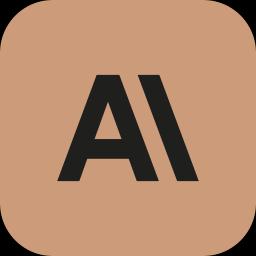
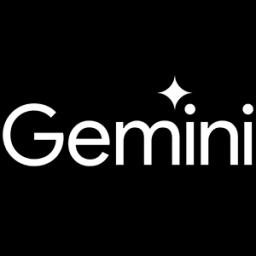
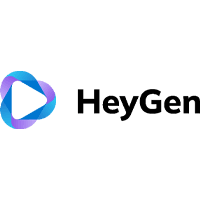
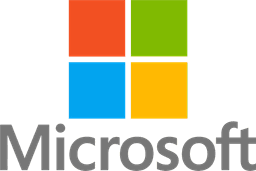
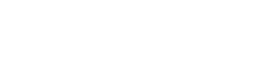
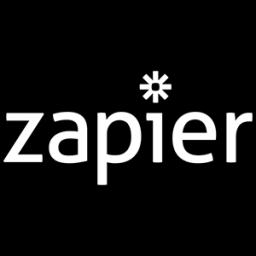
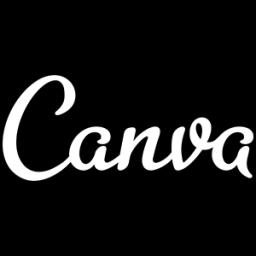
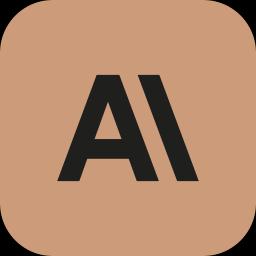
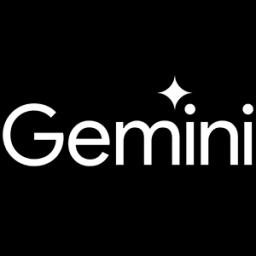
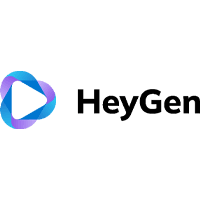
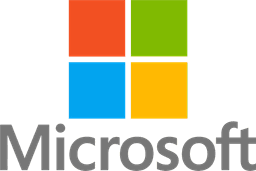
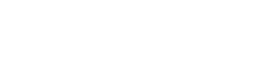
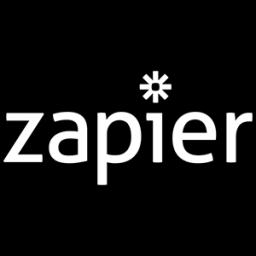
Politically, advancements in AI are likely to intensify geopolitical competition as nations race to establish dominance in AI capabilities. Such competition may raise national security concerns, as AI technologies become crucial components of both military and intelligence operations. There is an increasing need for international cooperation to manage these challenges, ensuring that AI advancements do not lead to instability or conflict but rather facilitate global progress and understanding .
The challenge of evaluating AI models accurately continues to pose additional issues. Current benchmarks, which often fail to reflect real-world applications, make it difficult to assess the true abilities of AI systems. This problem is further compounded by concerns over copyright infringement and "hallucinations," where AI generates incorrect or misleading content. To address these challenges, a collaborative approach involving both researchers and policymakers is necessary to ensure the responsible development and deployment of AI technologies. By working together, stakeholders can create evaluation metrics that accurately capture the capabilities and limitations of AI models, driving innovation while safeguarding ethical standards , .