Cutting-edge AI Breaths New Life into Protein Research
AI Revolutionizes Protein Sequencing: Discovering New Frontiers in Biology
Last updated:

Edited By
Mackenzie Ferguson
AI Tools Researcher & Implementation Consultant
Explore how groundbreaking AI tools like Casanovo and InstaNova are transforming protein sequencing beyond traditional methods. By accurately predicting peptide weights and allowing for the discovery of novel proteins, AI is paving the way for advancements in fields ranging from medical diagnostics to archaeology.
Introduction to AI in Protein Sequencing
Artificial intelligence (AI) is making significant inroads into the field of protein sequencing, offering novel solutions to some of the limitations posed by traditional methods. Traditionally, protein sequencing has relied heavily on matching peptide fragments to existing databases, a process that can often miss novel proteins not already cataloged. AI disrupts this model by predicting peptide masses, which include potential chemical modifications, and assembling these into full-length proteins, even if they are absent from current databases. This capability marks a substantial leap forward, enabling researchers to delve into previously inaccessible areas of protein science. According to a recent article on Science.org the AI revolution is profoundly impacting protein sequencing capabilities.
AI-powered tools like Casanovo and InstaNova exemplify this technological leap. Casanovo employs deep neural networks to refine its protein predictions, while InstaNova uses diffusion strategies for higher accuracy in identifying novel proteins within complex samples. These tools represent a paradigm shift, allowing scientists to venture beyond the constraints of conventional sequencing. The development of such tools has spurred interest across various fields, including medical diagnostics, environmental studies, and archaeology, where the ability to identify unknown proteins holds tremendous promise. More details can be referenced in the comprehensive overview available on Science.org.
Learn to use AI like a Pro
Get the latest AI workflows to boost your productivity and business performance, delivered weekly by expert consultants. Enjoy step-by-step guides, weekly Q&A sessions, and full access to our AI workflow archive.
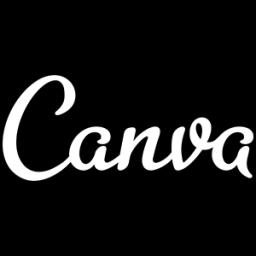
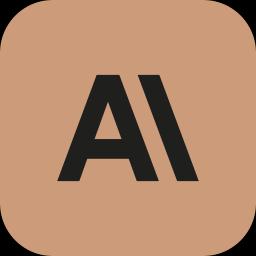
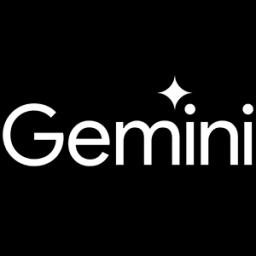
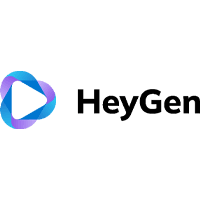
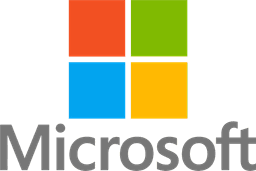
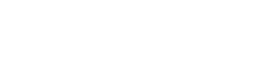
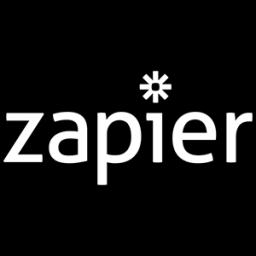
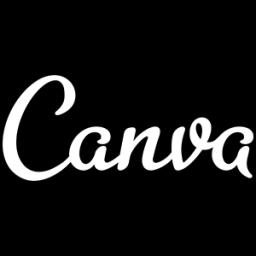
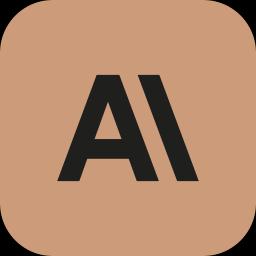
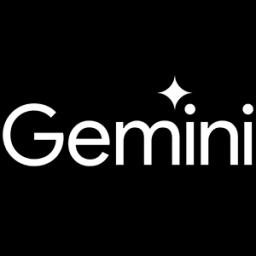
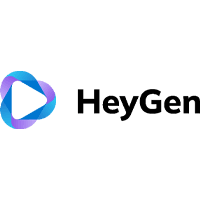
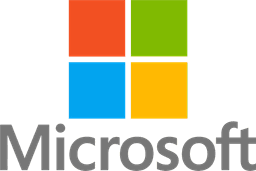
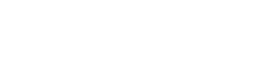
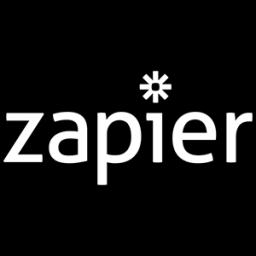
One of the key advantages offered by AI in the realm of protein sequencing is speed. AI algorithms process and analyze data at a rate significantly faster than traditional methods, enabling quicker identification and verification of proteins. Additionally, this technology facilitates the discovery of proteins that are omitted from existing datasets, which could prove invaluable in areas such as therapeutic drug development and disease diagnostics. As highlighted by researchers in the field, these capabilities not only broaden the scope of scientific inquiry but also enhance the potential applications of proteomics, particularly when tackling complex biological samples. For additional insights, you can visit Science.org.
Traditional vs. AI Protein Sequencing Methods
The realm of protein sequencing has seen transformative changes with the advent of AI-enhanced methods. Traditionally, protein sequencing relied heavily on database-dependent techniques. These methods involved breaking down the protein into peptides and matching them with existing databases to reconstruct the protein sequence. This approach inherently constrained the identification of novel proteins, which aren't captured in existing databases. However, AI methods have fundamentally shifted this paradigm. With tools like Casanovo and InstaNova, leveraging deep neural networks and diffusion strategies, researchers can now predict peptide weights, inclusive of possible chemical modifications, and accurately assemble them into full-length proteins even when these sequences are absent from current databases .
AI-powered protein sequencing stands out in several ways compared to traditional techniques. One significant advantage is speed; AI methods can process and analyze data much faster than conventional ones. Furthermore, they have the remarkable ability to identify proteins that do not exist in current databases, which is a substantial limitation of traditional methods. This capability opens new frontiers in various research fields, including medical diagnostics, environmental science, and archaeology, enabling scientists to decipher proteins that were previously unrecognizable. Such advancements underscore the potential of AI to overcome the confines of traditional methodologies, facilitating a deeper understanding of complex biological systems .
Key AI Tools for Protein Sequencing
Artificial intelligence (AI) is transforming the landscape of protein sequencing, offering innovative tools that significantly enhance the precision and capabilities of modern research. Traditional protein sequencing methods often rely on the identification of peptide fragments by matching them against extensive databases. However, these approaches struggle to identify novel peptides that do not match existing database entries. AI-driven techniques overcome these limitations by using deep learning algorithms to predict the weights of peptides, including potential chemical modifications, and assemble them into full-length proteins. This ability significantly expands the potential for discovery in fields requiring the identification of unknown proteins, such as medical diagnostics, environmental studies, and archaeology (Science.org).
Learn to use AI like a Pro
Get the latest AI workflows to boost your productivity and business performance, delivered weekly by expert consultants. Enjoy step-by-step guides, weekly Q&A sessions, and full access to our AI workflow archive.
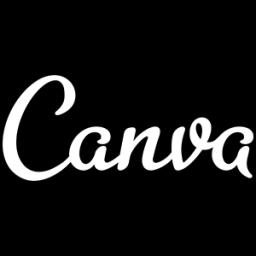
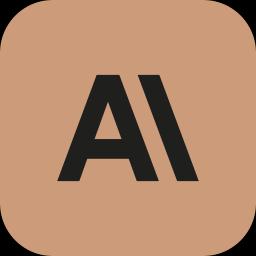
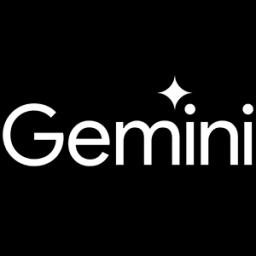
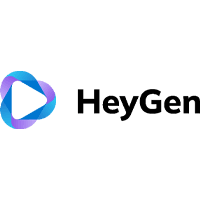
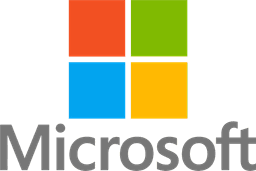
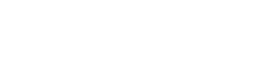
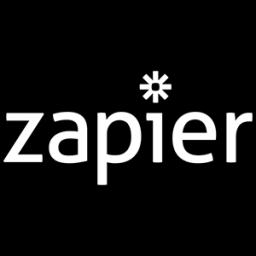
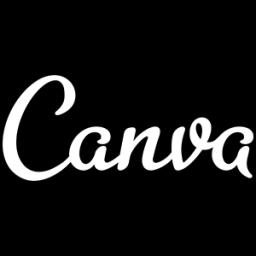
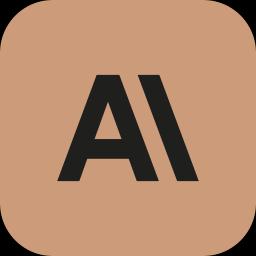
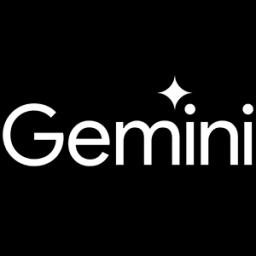
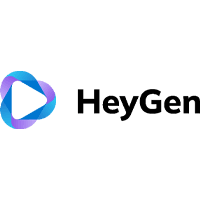
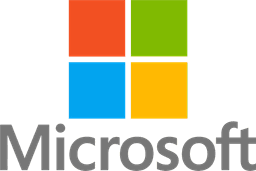
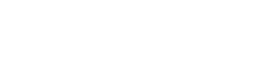
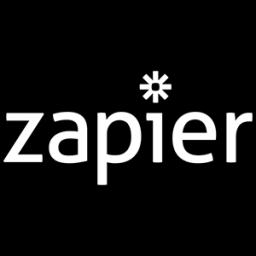
Among the pioneering AI tools revolutionizing protein sequencing are Casanovo and InstaNova. These tools leverage advanced deep learning and diffusion-based strategies to achieve high accuracy in identifying proteins from complex samples. Casanovo utilizes a deep neural network framework, enabling it to predict peptide sequences with remarkable precision. InstaNova, on the other hand, employs diffusion models that further refine the process, reducing false discovery rates and enhancing prediction accuracy. These tools are not only exemplary of the advancements in AI but are also crucial in facilitating the discovery of novel proteins potentially involved in disease mechanisms and environmental processes (Science.org).
The application of AI in protein sequencing extends far beyond simple identification—it allows for unprecedented insights into the complexities of proteomics. In practical scenarios, researchers employ these AI tools to identify pathogenic proteins in infected tissues, explore unknown proteins in marine environments, and study ancient proteins preserved in archaeological artifacts. Such applications underscore the technology's versatility and potential to redefine what is possible in both research and applied sciences, providing a more comprehensive understanding of biological processes (Science.org).
In addition to enhancing identification processes, AI tools for protein sequencing are accelerating advancements in related technologies and methods. For example, novel next-generation protein sequencing (NGPS) techniques are integrating traditional methods like Edman degradation with AI-enhanced fluorescence labeling and microscopy, vastly increasing throughput and efficiency. This synergy of AI with established techniques highlights the innovative trajectories that protein science is embarking on, promising to accelerate discovery within not just medical fields, but across a wide range of scientific disciplines that study biological molecules (Frontline Genomics).
Applications of AI in Various Fields
Artificial Intelligence (AI) has made substantial inroads into numerous fields, with its applications in healthcare standing out prominently. One of the significant advancements is in protein sequencing, where AI's ability to predict peptide weights and assemble full-length proteins without the need for extensive databases is revolutionizing medical diagnostics. This method is proving especially beneficial in rapidly identifying pathogenic proteins, which aids in timely and accurate diagnoses. For instance, AI-driven tools such as Casanovo and InstaNova enhance the detection of novel proteins in complex biological samples, which is pivotal in advancing personalized medicine and immunotherapy. Furthermore, AI technology empowers researchers to explore previously inaccessible data, contributing to more comprehensive insights in biochemical and cellular processes for better health outcomes. source.
Beyond healthcare, AI's influence expands into environmental studies, where it assists in the identification and analysis of proteins within various ecological settings. These applications contribute to biodiversity assessments and environmental monitoring by detecting unknown peptides in seawater and other natural samples. Such advancements are crucial in understanding ecosystems' health and resilience, enabling better conservation strategies. The use of AI in these contexts illustrates its capability to handle vast and complex data sets, drawing meaningful conclusions that inform policy and preservation efforts. As AI applications continue to evolve, its role in addressing global environmental challenges gains prominence, providing tools for sustainable management and ecological protection. source.
In the field of archaeology, AI opens new avenues for exploring historical and prehistorical contexts. By applying protein sequencing technologies, archaeologists can examine ancient proteins embedded in artifacts, which offers insights into the dietary habits, environments, and technologies of past civilizations. This approach has already yielded discoveries, such as the analysis of protein residues in Neanderthal remains and ancient pottery, shedding light on early human innovation and adaptation. Furthermore, AI helps mitigate the destructive analysis traditionally associated with archaeological studies, preserving invaluable artifacts for future generations. The integration of AI in archaeology exemplifies its transformative potential in enhancing our understanding of history and heritage, setting the stage for groundbreaking discoveries in the years to come. source.
Learn to use AI like a Pro
Get the latest AI workflows to boost your productivity and business performance, delivered weekly by expert consultants. Enjoy step-by-step guides, weekly Q&A sessions, and full access to our AI workflow archive.
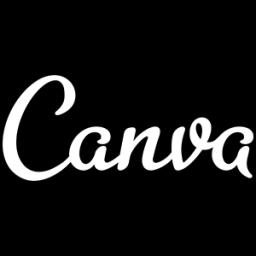
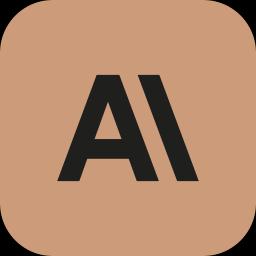
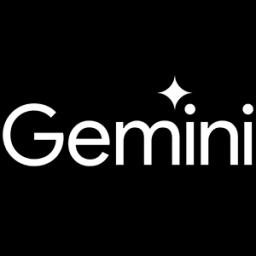
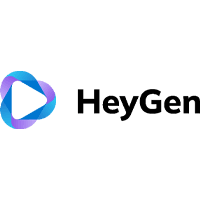
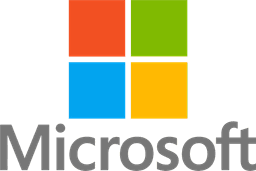
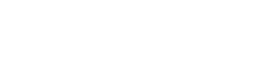
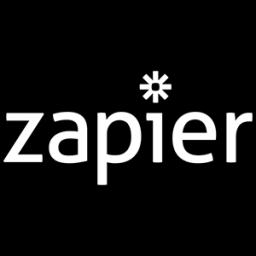
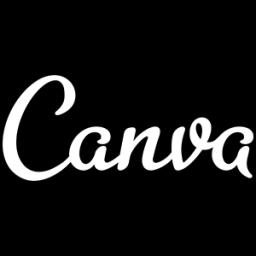
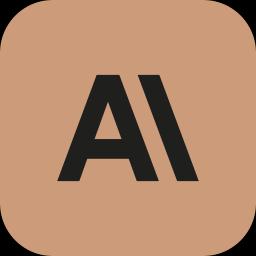
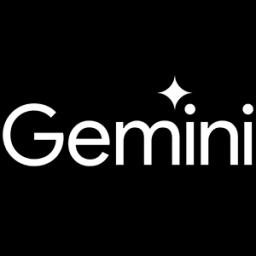
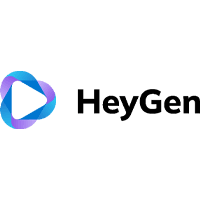
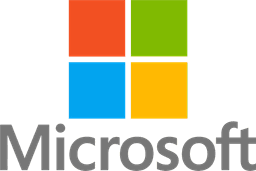
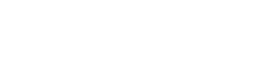
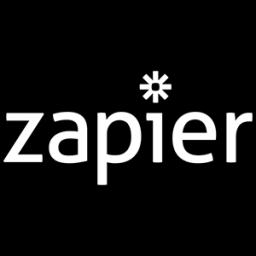
Training AI for Protein Sequencing
Training AI for protein sequencing marks a significant leap forward in the field of biotechnology. Traditional methods relied heavily on databases of known protein sequences, limiting their ability to identify novel proteins. AI-based methods, however, shift away from these constraints by predicting peptide weights and assembling them into full-length proteins using sophisticated algorithms. This shift allows researchers to explore new possibilities, including the discovery of proteins not found in existing databases. AI-powered tools like Casanovo and InstaNova utilize deep learning and diffusion models to achieve higher accuracy and efficiency, facilitating the identification of novel proteins in complex biological samples. This has major implications for various fields, including healthcare, environmental science, and archaeology, where identifying unknown proteins can lead to groundbreaking discoveries. For more insights, see this article on AI revolution in protein sequencing.
AI's role in protein sequencing goes beyond simple improvements in speed and efficiency; it opens the door to new research opportunities and applications. Tools like InstaNovo and InstaNovo+ from teams at the Technical University of Denmark and Delft University exemplify the cutting-edge of AI applications in protein science. These models employ a transformer-based architecture and a diffusion model, respectively, to enhance prediction accuracy, reducing false discovery rates and enabling researchers to unlock previously unexplored genomic territories. The ability to identify and sequence unknown proteins holds promise for advancing medical diagnostics, where speed and accuracy can save lives, and in environmental and archaeological studies, where new discoveries await. Further details can be found in the comprehensive article by Phys.org.
The advantages of AI-driven protein sequencing are particularly notable in the realm of novel peptide discovery. These peptides play crucial roles in immune recognition and can lead to advancements in immunotherapy and personalized healthcare solutions. This technological leap is changing the landscape of medical diagnostics, allowing professionals to address complex diseases with more targeted therapeutic strategies. AI models are also improving proteomics searches, broadening our understanding across various biological fields, from plant science to industrial biotechnology. This shift towards more dynamic and insightful research methods underscores the transformative power of AI in biology. Explore more on these advancements via Chemistry World.
Despite these advancements, AI-driven protein sequencing must address certain challenges to fully realize its potential. Concerns about the ethical implications, such as data privacy, misuse of genetic information, and the potential displacement of jobs due to automation, need consideration. Strategies to integrate these tools into existing workflows require careful planning and coordination. Additionally, the long-term impacts on the economy and society need ongoing evaluation. Ethical governance and international cooperation will be essential in shaping the future of this revolutionary technology. The issues surrounding data ownership and intellectual property rights also demand attention to ensure fair and beneficial use. Further insights into these challenges can be found in a detailed paper from Science News.
Real-world Implications and Case Studies
The real-world implications of AI-driven protein sequencing are far-reaching, providing transformative insights and advancements across various fields. In medical diagnostics, these AI systems are pivotal in identifying pathogenic proteins, offering a significant leap forward for rapid and precise diagnoses. This approach revolutionizes how medical conditions are detected and treated, potentially leading to early intervention and improved patient outcomes. Environmental studies also benefit greatly, as AI tools can now analyze complex protein compositions in diverse ecosystems, such as identifying unknown proteins in seawater. This capability not only aids in understanding biodiversity but also in monitoring environmental health and detecting changes in marine ecosystems.
Case studies showcasing AI-driven sequencing tools highlight significant breakthroughs in several areas. In the field of archaeology, for instance, protein analysis of ancient artifacts through AI sequencing techniques provides unprecedented insights into historical diets and human evolution. Researchers have successfully applied these methods to study ancient protein residues on tools and pottery, revealing interactions between past humans and their environments. Such applications underscore the versatility and transformative potential of AI in unraveling historical mysteries and enhancing our understanding of ancient civilizations.
Learn to use AI like a Pro
Get the latest AI workflows to boost your productivity and business performance, delivered weekly by expert consultants. Enjoy step-by-step guides, weekly Q&A sessions, and full access to our AI workflow archive.
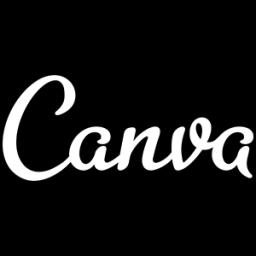
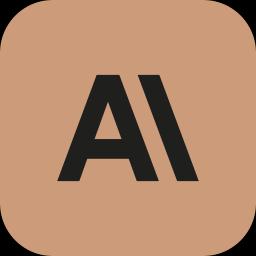
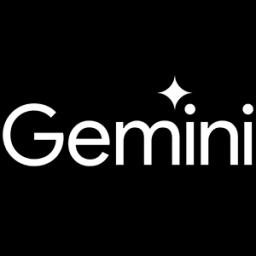
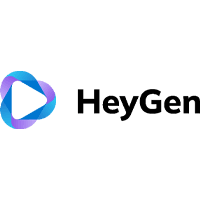
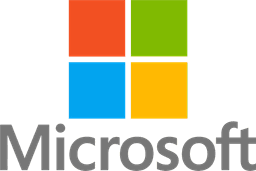
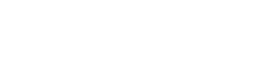
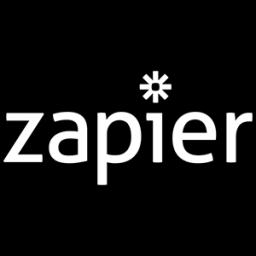
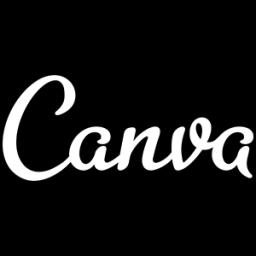
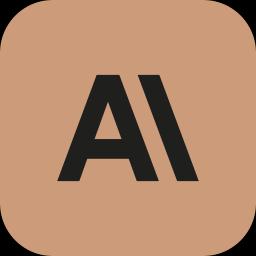
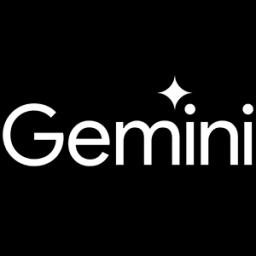
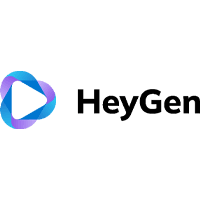
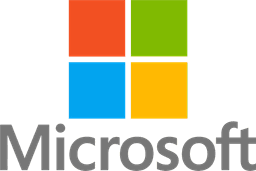
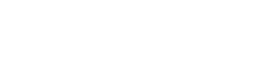
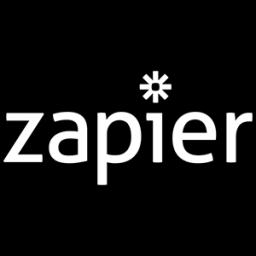
In industry, the deployment of AI for protein sequencing is reshaping biotechnological processes. AI models are improving the efficiency of proteomics searches across various sectors, including biotechnology, energy, and food industries. With enhanced accuracy in identifying novel peptides, AI is paving the way for the development of innovative solutions, such as bioengineered enzymes and sustainable biofuels. This optimization not only leads to resource savings and increased productivity but also supports sustainable industrial practices, aligning with global efforts toward environmental sustainability.
Moreover, the discovery of novel peptides facilitated by AI-driven sequencing is opening new avenues in drug development and healthcare. By pinpointing unique protein structures involved in immune responses, researchers are identifying new targets for immunotherapy and personalized cancer treatments. This advancement accelerates the development of therapies that are tailored to individual patients, potentially transforming cancer treatment protocols and improving survival rates. The integration of AI in protein sequencing represents not just a technological advancement but a paradigm shift in both scientific research and clinical practice.
Recent Advancements in Sequencing Algorithms
AI-driven tools like Casanovo and InstaNova have been game-changers in the world of protein sequencing. Both employ cutting-edge techniques to enhance the sequencing process. For instance, Casanovo utilizes deep neural networks to substantially improve the accuracy of peptide identification, while InstaNova incorporates diffusion strategies to refine sequence predictions further, as detailed in Science. Such tools are proving invaluable across diverse scientific domains, from medical diagnostics, where unknown pathogenic proteins must be identified rapidly, to archaeology, which benefits from the ability to analyze ancient proteins from various artefacts.
Expert Opinions on AI's Transformative Potential
AI's transformative potential is undeniable, particularly through its impact on fields like protein sequencing. Experts in the domain highlight that traditional methods struggle with limitations in database dependency. In contrast, AI-driven models bring a seismic shift by predicting protein structures and their weights, facilitating a more nuanced understanding of biological sequences. Such advancements are epitomized by tools like Casanovo and InstaNova, which use cutting-edge deep learning technologies to reconstruct proteins in ways previously deemed impossible.
The revolutionary nature of AI extends beyond technological prowess; it has significant implications for scientific research and practical applications. Experts from renowned institutions such as the Technical University of Denmark and Delft University have been pivotal in developing InstaNovo and InstaNovo+. These innovations, characterized by transformer-based architectures and diffusion strategies, are not only increasing accuracy but also minimizing false discovery rates. This paradigm shift in protein sequencing echoes profound potentials that experts foresee in various scientific and healthcare domains.
Furthermore, analysts such as Benjamin Neely from the National Institute of Standards and Technology view AI models like InstaNovo as cornerstones of a new era in protein science. The capability to decode previously unstudied proteins opens unexplored avenues in genetic research. Similarly, Amanda Smythers from the Dana-Farber Cancer Institute sees potential in tackling complex disease-related questions using these tools. The expertise consolidates the view that AI is set to redefine the landscape of protein analysis and its application in therapeutics and diagnostics.
Learn to use AI like a Pro
Get the latest AI workflows to boost your productivity and business performance, delivered weekly by expert consultants. Enjoy step-by-step guides, weekly Q&A sessions, and full access to our AI workflow archive.
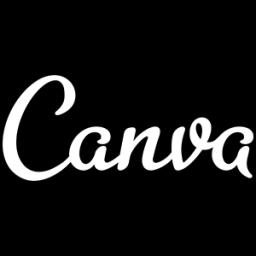
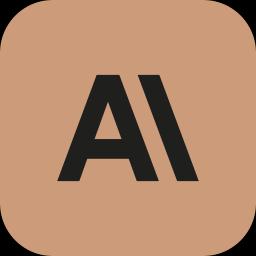
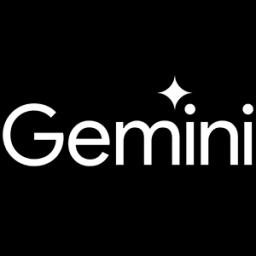
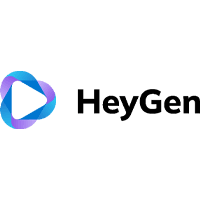
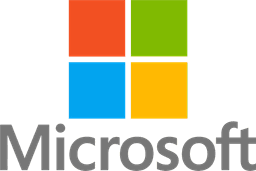
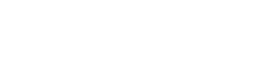
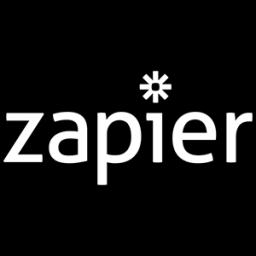
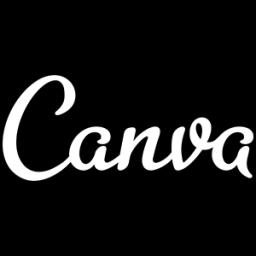
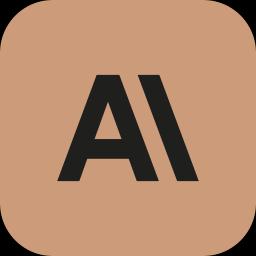
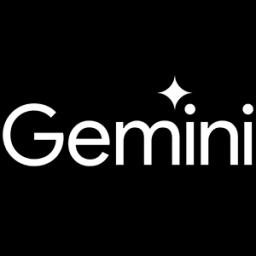
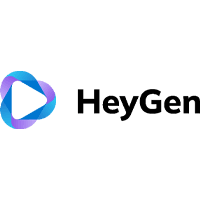
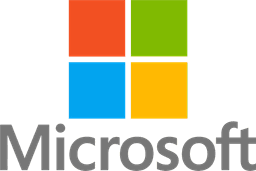
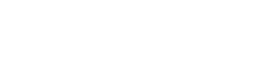
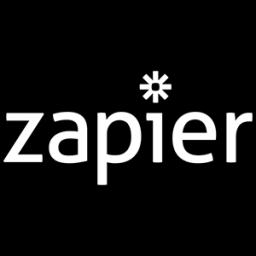
The broader implications of AI in protein sequencing are profound. Economically, these technologies promise to reduce the cost and time of drug discovery, heralding a revolution in healthcare by providing affordable medicines. Social benefits are also anticipated, such as improved public health outcomes and increased life expectancy due to rapid diagnostic advancements. Nonetheless, experts caution about ethical considerations, emphasizing the need for careful management of genetic data and awareness of job displacement risks due to automation.
Looking towards the future, experts predict that AI's application in protein sequencing could guide national or international scientific agendas and trigger shifts in research funding. Potential collaborations and competition in AI-driven biotechnologies may shape geopolitical dynamics around innovation. Experts argue that to fully harvest the benefits while mitigating risks, sustained investment in AI research and collaborative efforts among scientists worldwide will be pivotal. The insights provided by the experts are invaluable in navigating the ethical and social implications tied to these transformative technologies.
Public Reactions and Sentiments
The advent of AI-driven protein sequencing has sparked diverse reactions across the scientific and public spheres. On the one hand, experts within the scientific community hail these advancements as groundbreaking, paving the way for unprecedented discoveries in proteomics and molecular biology. This enthusiasm is echoed by researchers who are eager to explore new realms in medical diagnostics, environmental science, and archaeological research using tools like Casanovo and InstaNova, which leverage deep learning to decipher complex protein structures [1](https://www.science.org/content/article/ai-revolution-comes-protein-sequencing).
However, the public sentiment towards AI in protein sequencing is mixed, reflecting broader concerns about AI technologies. Some individuals express excitement about the potential for personalized medicine and more effective treatments, eager for the promised advancements in healthcare and the possible extension of human life expectancy. Nonetheless, there are also apprehensions about ethical implications, particularly regarding data privacy, the environmental impact of enhanced biotechnology, and the potential misuse of AI in sensitive areas like genetic information management [3](https://www.sciencenews.org/article/ai-decode-indecipherable-proteins).
Social media platforms teem with discussions where some users commend the acceleration of scientific research and improved health diagnostics thanks to AI technologies. Others, however, voice skepticism about the accuracy and reliability of these AI systems, pointing out past incidents of AI errors and emphasizing the need for rigorous testing and validation. As AI-driven tools are increasingly integrated into healthcare and research, the tension between innovation and caution becomes a central theme in the public dialogue [3](https://www.sciencenews.org/article/ai-decode-indecipherable-proteins).
Ultimately, while public reactions vary, there's a shared recognition of the transformative potential AI holds for reshaping various sectors. The consensus is that while the benefits are immense, they must be weighed carefully against the ethical challenges and technical uncertainties that accompany these cutting-edge technologies. Engaging transparently with the public and integrating ethical considerations into technological advancements will be key to aligning progress with societal values and expectations [3](https://www.sciencenews.org/article/ai-decode-indecipherable-proteins).
Learn to use AI like a Pro
Get the latest AI workflows to boost your productivity and business performance, delivered weekly by expert consultants. Enjoy step-by-step guides, weekly Q&A sessions, and full access to our AI workflow archive.
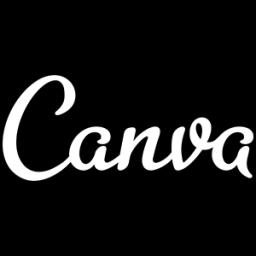
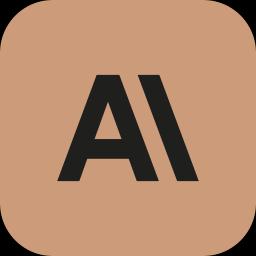
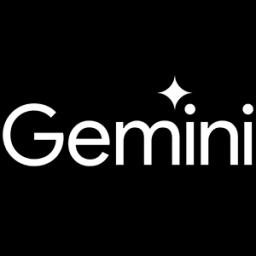
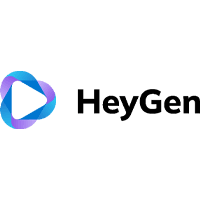
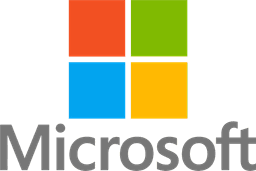
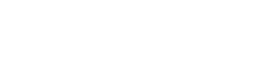
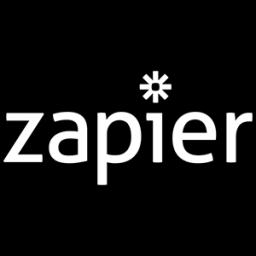
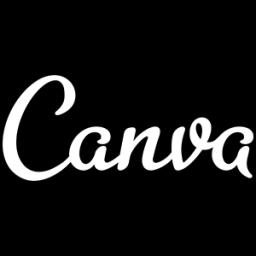
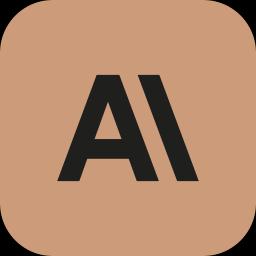
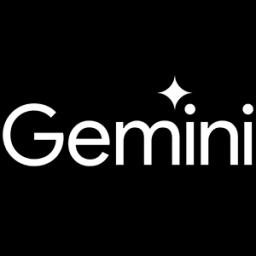
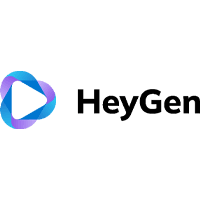
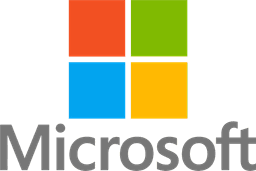
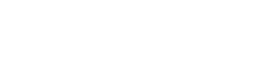
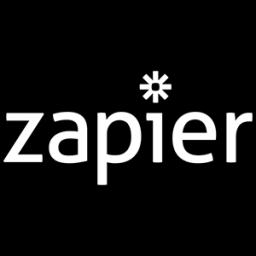
Future Implications of AI in Protein Sequencing
The integration of artificial intelligence in protein sequencing promises to revolutionize fields far beyond the current scientific landscape. AI-driven models provide a pathway to overcoming the deficiencies of traditional methods that often fall short when encountering novel proteins. By predicting peptide weights and assembling them into complete proteins, AI systems alleviate the limitations of database-dependent sequencing methodologies.
In medical diagnostics, the potential of AI in protein sequencing is profound. Enhanced by innovative tools such as Casanovo and InstaNova, clinicians can identify proteins that may serve as early indicators of disease, even those not yet cataloged in medical databases. This application could fundamentally enhance diagnostic precision and speed, leading to improved treatment strategies and patient outcomes. Additionally, AI tools contribute to groundbreaking research in genetic diseases by offering insights into previously uncharacterized proteins.
On an economic level, AI-driven protein sequencing holds the potential to significantly reduce the costs associated with drug discovery. The rapid identification and characterization of proteins can accelerate the development of new therapeutics, making the process more cost-effective. This could, in turn, lead to cheaper medicines and widespread healthcare savings. Furthermore, the creation of innovative bio-based products through AI sequencing efforts could bolster industries from agriculture to pharmaceuticals, sparking new market opportunities.
Socially, the advancements in AI protein sequencing could lead to improved healthcare access and increased life expectancy by accelerating the development of targeted treatments and diagnostics. By reducing the time and cost of healthcare innovations, these tools could ensure more equitable distribution of healthcare technologies. Additionally, the sustainability of new materials developed through protein sequencing could further enhance public health outcomes by minimizing environmental impacts. Nevertheless, the rise of AI in protein sequencing also necessitates a careful examination of ethics, particularly concerning genetic information privacy and the potential for automation to displace jobs.
Politically, the implications of AI in protein sequencing may drive countries to rethink their research and funding priorities, potentially leading to new global alliances or conflicts over technology access. As nations advance their capabilities in this burgeoning field, international regulations will be needed to oversee ethical use, data ownership, and intellectual property rights. Governments may need to bolster regulations to safeguard against misuse, ensuring that the burgeoning AI capabilities serve global interests rather than narrow, nationalistic agendas.
Challenges and Uncertainties Ahead
The rise of artificial intelligence-driven protein sequencing marks a significant milestone in biotechnology, yet it is accompanied by various challenges and uncertainties. While AI tools like Casanovo and InstaNova have enhanced the accuracy of protein identification, their integration into existing laboratory and clinical workflows remains complex. This shadow of uncertainty stems from the need to adapt regulatory frameworks that were primarily designed for traditional methods to accommodate these advanced technologies. Additionally, as these AI systems become more prevalent, ethical concerns such as data privacy and the potential misuse of genetic information become more pressing. Addressing these concerns will require collaborative efforts from technologists, ethicists, and policymakers [3](https://www.sciencenews.org/article/ai-decode-indecipherable-proteins).
Learn to use AI like a Pro
Get the latest AI workflows to boost your productivity and business performance, delivered weekly by expert consultants. Enjoy step-by-step guides, weekly Q&A sessions, and full access to our AI workflow archive.
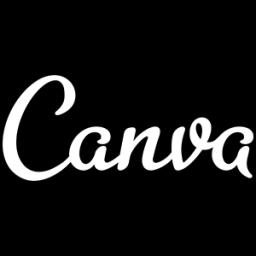
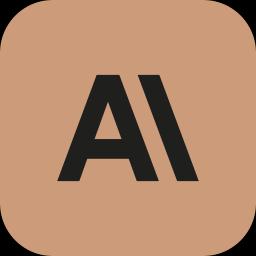
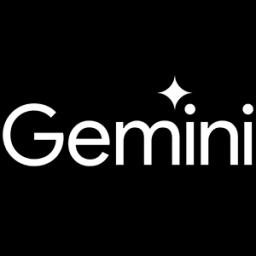
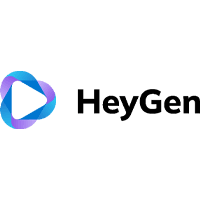
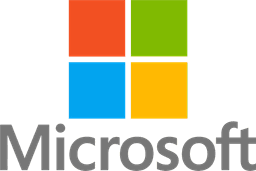
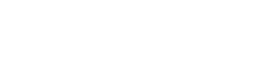
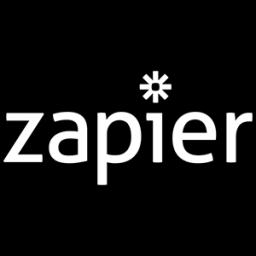
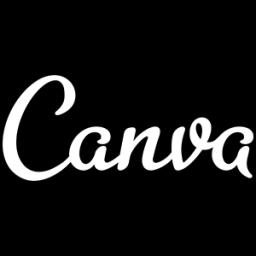
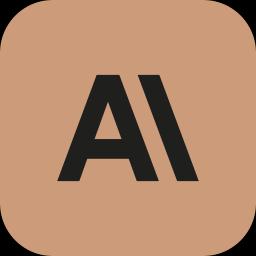
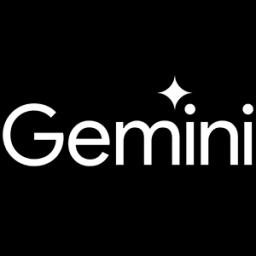
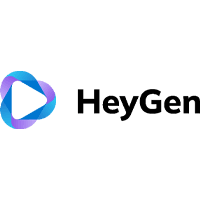
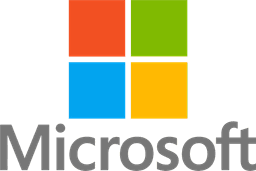
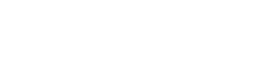
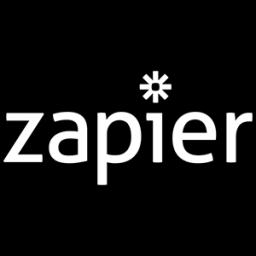
Economically, AI-driven protein sequencing offers exciting opportunities but also brings uncertainties that require attention. The technologies have the potential to revolutionize pharmaceutical development, thereby impacting everything from drug pricing to healthcare dynamics. Conversely, the rapid pace of AI advancement may lead to an inequitable distribution of these benefits, particularly in regions lacking robust technological infrastructure. This could exacerbate existing disparities in healthcare access and outcomes. Furthermore, the shift towards automated systems might result in job displacement in certain sectors, challenging the current economic landscape [3](https://www.sciencenews.org/article/ai-decode-indecipherable-proteins).
Socially, the implications of AI in protein sequencing are profound yet fraught with uncertainties. On one hand, these innovations could lead to improved patient outcomes through faster and more accurate diagnostics, particularly benefiting underserved communities. On the other hand, the rapid implementation of such technology imposes ethical challenges, including the fair distribution of resources and the need to address the digital divide. Achieving a balance between technological advancement and social equity will be crucial to harness the full potential of these scientific breakthroughs [3](https://www.sciencenews.org/article/ai-decode-indecipherable-proteins).
Politically, the global landscape could be reshaped by the burgeoning field of AI-driven protein sequencing. Governments might need to reallocate research priorities and funding to support the growth of biotechnology sectors, which in turn might influence international relations. The race to master and implement these technologies could either foster international collaborations or fuel competitive tensions. Furthermore, these advancements necessitate the development of new policies concerning data ownership and intellectual property, which could become points of contention in international forums [3](https://www.sciencenews.org/article/ai-decode-indecipherable-proteins).
The potential of AI in protein sequencing is as vast as it is uncertain. Despite the breakthroughs in methodologies, the accuracy and robustness of AI predictions in protein science remain areas of active investigation. Ensuring these tools are reliable enough for widespread application requires rigorous testing and validation. Additionally, as the models evolve, continuous research will be vital to understand their unforeseen impacts on the economy and society. Collaboration across disciplines will play a pivotal role in advancing these technologies responsibly and ethically [3](https://www.sciencenews.org/article/ai-decode-indecipherable-proteins).