The Power of Scaling in AI Evolution
AI Scaling: The Key to Future Breakthroughs, Says EconTalk
Last updated:

Edited By
Mackenzie Ferguson
AI Tools Researcher & Implementation Consultant
In a recent EconTalk episode, Dwarkesh Patel delves into the transformative potential of AI scaling. By focusing on increased computational resources and data, rather than solely on algorithmic advancements, Patel argues that this approach holds the key to future AI milestones, including the enigmatic pursuit of Artificial General Intelligence (AGI).
Introduction to the Scaling Era in AI
The introduction to the scaling era in AI marks a transformative period in the development of artificial intelligence. Emphasizing the vast leaps in capabilities achieved through increased computational power and extensive datasets, this era represents a shift from solely crafting new algorithms to optimizing existing ones on a larger scale. According to Dwarkesh Patel on the EconTalk podcast, this emphasis on scaling rather than innovation in algorithm design has rapidly advanced AI technologies. This approach has powered significant breakthroughs in machine learning models, such as the development of highly proficient large language models and advanced neural network architectures, which significantly outperform their predecessors.
In this scaling era, models like transformers have become crucial in processing vast amounts of data efficiently. Their ability to handle complex tasks through attention mechanisms enables them to excel in domains ranging from natural language processing to image recognition. The discussion highlights that while scaling has achieved remarkable accuracy in tasks like text generation and image processing, it has also unveiled challenges, such as the integration of common-sense reasoning and understanding of nuanced social interactions. These limitations underscore the ongoing need to balance scaling with fundamental improvements in AI reasoning capabilities.
Learn to use AI like a Pro
Get the latest AI workflows to boost your productivity and business performance, delivered weekly by expert consultants. Enjoy step-by-step guides, weekly Q&A sessions, and full access to our AI workflow archive.
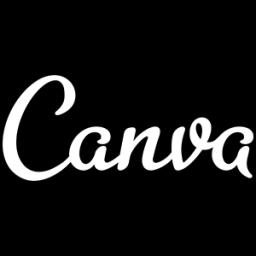
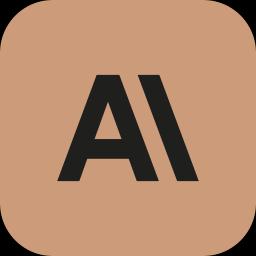
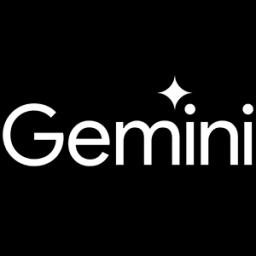
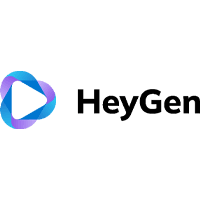
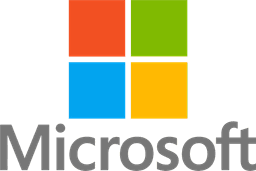
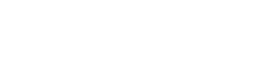
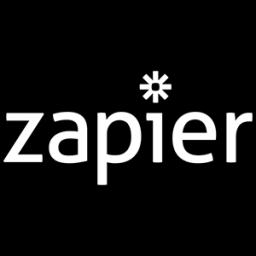
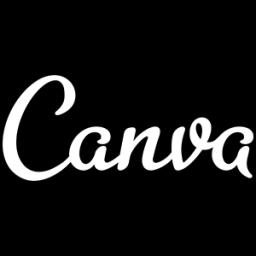
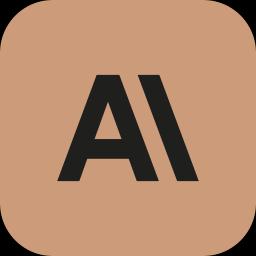
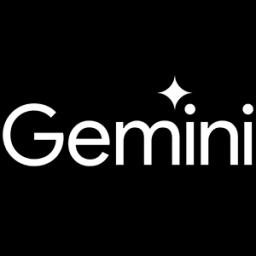
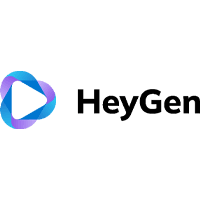
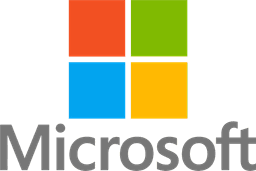
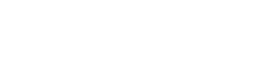
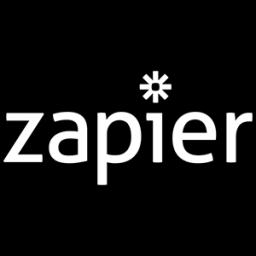
Significant attention in the scaling era is directed towards achieving Artificial General Intelligence (AGI). As Patel discusses, the potential of AGI hinges on models that can generalize learning across varied domains much like human cognitive processes. While the idea of reaching AGI solely through scaling is a hot topic of debate, it is evident that immense computational resources are needed to even approach this goal. As AI systems continue to grow in complexity, the necessity for trial and error methods to iteratively refine and improve algorithms becomes ever more prominent, showcasing the dynamic interplay between scaling and innovation.
Alongside scaling efforts, the development of hybrid AI models has become pivotal. These models combine the brute force of computation with sophisticated reasoning abilities, aiming to emulate aspects of human problem-solving. This evolution in AI reflects the insights shared in the podcast regarding the potential and pitfalls of scaling. While hybrid models promise enhanced decision-making capabilities, they also highlight the current AI models' struggle with generating secure code and accurately interpreting human emotions and intentions, reaffirming the need for ongoing research and innovation.
Understanding Transformers and Their Role
Transformers represent a significant milestone in the development of AI, fundamentally changing how linguistic tasks are approached and handled. The transformer model, introduced by Vaswani et al. in 2017, uses a mechanism known as 'self-attention'. This allows the model to weigh the significance of different words in a sentence contextually, enabling it to understand and generate human-like text more effectively than previous models. This architecture has been incredibly influential in the "scaling era" of AI, where success is driven by leveraging vast amounts of data and computational power rather than developing novel algorithms. As highlighted in the EconTalk podcast, the rise of transformers aligns with the trend of prioritizing scaling to enhance AI capabilities.
The role of transformers in the scaling era of AI can't be overstated. They operate by processing data in parallel rather than sequentially, which greatly increases efficiency and performance on large datasets. This is crucial in modern-day AI applications like language translation, text summarization, and sentiment analysis, where the demand for processing power and data utilization is high. Furthermore, the adaptation of transformers in models like GPT and BERT has catalyzed advancements in natural language processing tasks, paving the way for more sophisticated AI models. As discussed in the EconTalk episode with Dwarkesh Patel, the emphasis on scaling has driven major improvements in AI performance, especially in fields requiring nuanced understanding and generation of human language.
Learn to use AI like a Pro
Get the latest AI workflows to boost your productivity and business performance, delivered weekly by expert consultants. Enjoy step-by-step guides, weekly Q&A sessions, and full access to our AI workflow archive.
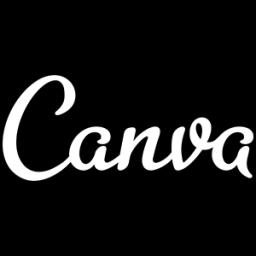
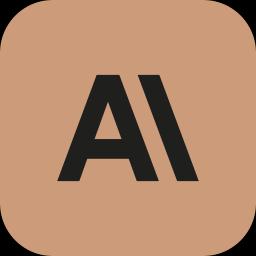
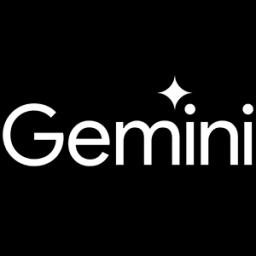
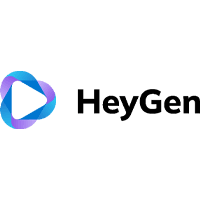
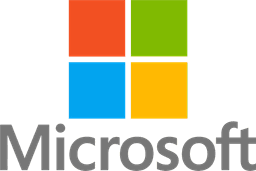
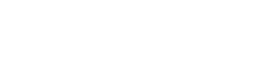
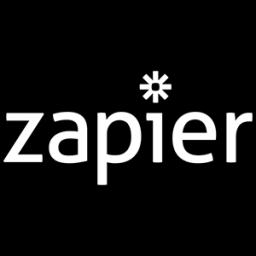
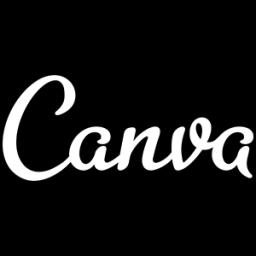
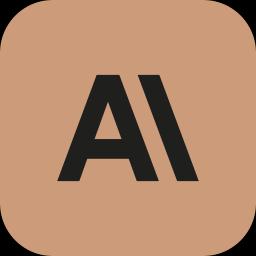
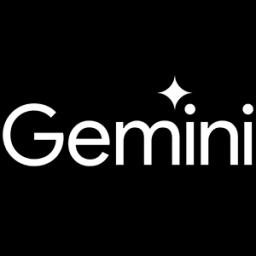
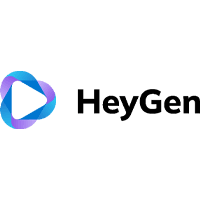
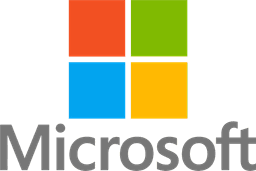
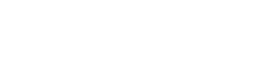
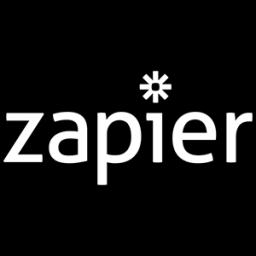
Despite their success, transformers and the AI models built around them face certain limitations. Current large language models, such as those utilizing transformer architecture, sometimes struggle with real-world tasks that require a deep understanding of context and common sense reasoning. The EconTalk podcast notes these challenges, emphasizing that while scaling has led to substantial improvements, it hasn't yet resolved these fundamental issues. Additionally, these models are prone to generating misleading or incorrect information, which underscores the importance of continuous oversight and refinement in AI development. The conversation in the podcast further explores the balance between scaling and the need for innovative algorithms to push the boundaries of AI closer to Artificial General Intelligence (AGI).
Challenges and Limitations of Current AI Models
The current landscape of AI models presents numerous challenges and limitations that are crucial to understanding the technology's future trajectory. One of the primary obstacles is the reliance on massive data sets and computational resources to achieve progress, underscoring the 'scaling era' of AI. This approach, while effective in boosting performance metrics, often falls short in addressing the nuances of human reasoning and decision-making processes. As highlighted in a recent discussion on EconTalk, AI still struggles with tasks that require contextual understanding and common sense, critical for achieving true artificial general intelligence (AGI) ().
The limitations inherent in large language models (LLMs) further complicate the development of advanced AI systems. These models, despite their sophistication, frequently generate incorrect information. This issue was notably explored in an EconTalk podcast episode, revealing the significant gap between AI capability and human-like reasoning (). Moreover, social understanding remains a critical weakness; AI systems are particularly challenged in interpreting and responding to human interactions, an essential component of real-world applications ().
The emergence of hybrid AI models represents a promising, albeit partial, solution to these challenges by integrating advanced reasoning capabilities with the scaling of data and computational power. This trend indicates a shift towards models that can think and adapt more like humans, although the question of achieving AGI through scaling remains a topic of intense debate. The insights shared in the EconTalk podcast elaborate on this balance between computation and cognitive capabilities necessary for sustainable AI development ().
Accuracy issues also plague AI-generated outputs, such as code, where security vulnerabilities are prevalent, emphasizing the need for human oversight. Approximately 40% of AI tool-generated code suggestions are prone to security flaws, highlighting the ongoing challenges in achieving reliability and trust in AI systems (). As AI continues to evolve, these limitations underscore the importance of developing robust oversight mechanisms alongside technological advancement.
Exploring the Concept of Artificial General Intelligence
Artificial General Intelligence (AGI) represents the next frontier in the pursuit of creating machines with the cognitive capabilities akin to human intelligence. Unlike narrow AI, which is designed to perform specific tasks, AGI aims to master a wide range of intellectual functions, mirroring human versatility in problem-solving and learning. The journey to AGI is fraught with challenges, as discussed by Dwarkesh Patel on the EconTalk podcast, where the emphasis on scaling computational resources rather than solely relying on algorithmic innovation was highlighted as a pivotal aspect of AI progress.
Learn to use AI like a Pro
Get the latest AI workflows to boost your productivity and business performance, delivered weekly by expert consultants. Enjoy step-by-step guides, weekly Q&A sessions, and full access to our AI workflow archive.
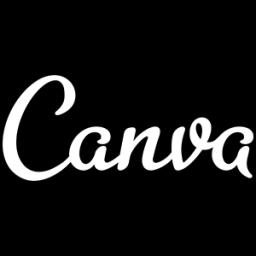
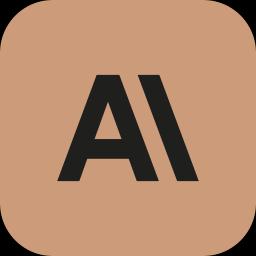
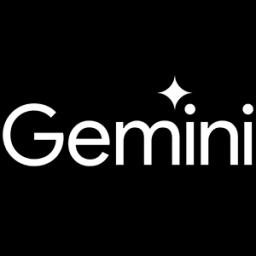
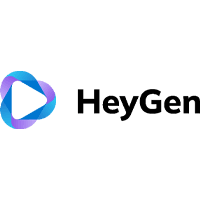
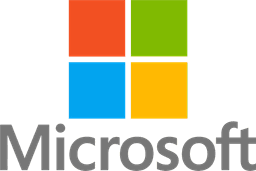
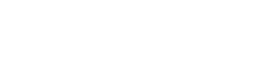
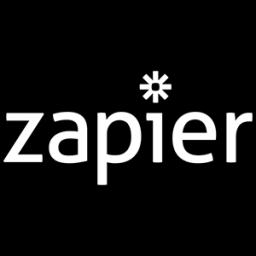
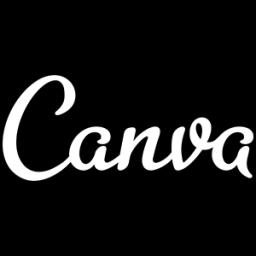
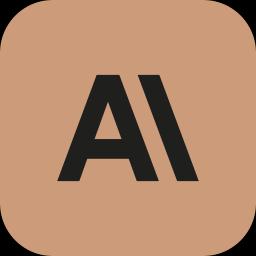
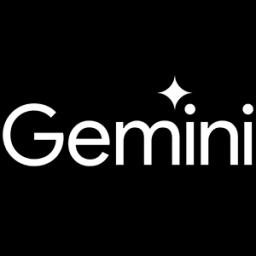
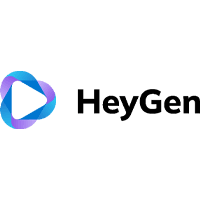
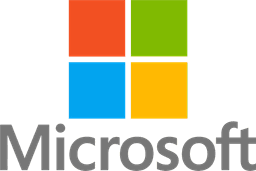
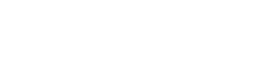
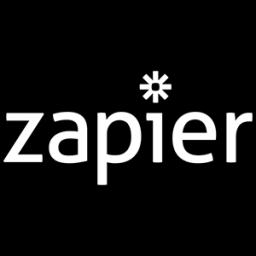
One of the intriguing elements of the AGI debate is whether mere scaling of current AI architectures can lead to general intelligence, a concept that researchers continue to explore vigorously. Some propose that increasing the scale of models and the data they are trained on could eventually yield AGI, circumventing the need for novel algorithms. However, this perspective is not without its critics, as others argue that overcoming the limitations of current AI models—such as their struggles with tasks requiring common sense reasoning and the propensity to produce incorrect information—demands new breakthroughs beyond mere scaling, a theme also touched upon in Patel's discussion on EconTalk.
The potential of AGI carries profound implications not only for technology but also for economic, social, and political spheres. Economically, AGI could drastically boost productivity and efficiency, though not without the risk of job displacement and the exacerbation of income inequality, as noted in the EconTalk podcast. Beyond economics, socially and politically, AGI presents both opportunities and challenges. On one hand, it could enhance decision-making processes and innovation. On the other, it might exacerbate misinformation and manipulation risks, further complicating the landscape of trust and governance.
Exploring the broader impacts of AGI involves understanding its role in revolutionizing different sectors. From automating complex tasks to potentially designing new drugs or creating art, the versatility of AGI could redefine traditional industries. However, the ethical and regulatory frameworks for such a transformative technology are still nascent, echoing the cautionary insights shared by Dwarkesh Patel on EconTalk. The pace at which AGI develops could outstrip our ability to govern it responsibly, highlighting the urgent need for comprehensive policy-making that anticipates rather than reacts to technological evolution.
As the quest for AGI continues, the conversation often circles back to the fundamental question of what it means for a machine to 'understand'—a philosophical inquiry as much as a technical one. Unlocking AGI would not only transform technology but force a reevaluation of our understanding of intelligence itself. The ongoing dialogue, as seen in platforms like the EconTalk podcast, underscores the need for interdisciplinary approaches, drawing from cognitive science, ethics, and artificial intelligence research to chart a path forward.
The Importance of Trial and Error in AI Development
Trial and error is a cornerstone in the evolution of artificial intelligence (AI), serving as a pivotal learning mechanism. This approach allows AI developers to iteratively improve their models, often by observing what works and what doesn't through practical experimentation. Such a methodology is crucial in an era where AI is rapidly advancing, driven by large-scale computational capabilities. As discussed in the EconTalk podcast with Dwarkesh Patel, scaling through enhanced compute and data resources defines much of the current progress in AI, rather than solely relying on revolutionary algorithmic techniques (source).
The importance of trial and error in AI development lies in its ability to uncover the limitations and potentials of AI models that theoretical approaches might overlook. By engaging in this process, developers can fine-tune models to better perform real-world tasks, including those requiring nuanced reasoning and social understanding—areas where AI traditionally struggles. The podcast episode highlights these challenges and discusses the potential for Artificial General Intelligence (AGI), underscoring the significance of continual model iteration through trial and error to achieve breakthroughs (source).
Learn to use AI like a Pro
Get the latest AI workflows to boost your productivity and business performance, delivered weekly by expert consultants. Enjoy step-by-step guides, weekly Q&A sessions, and full access to our AI workflow archive.
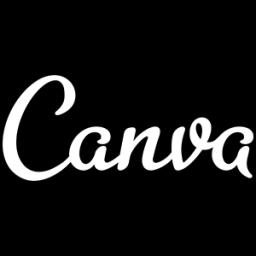
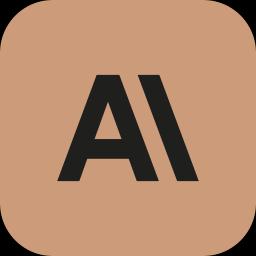
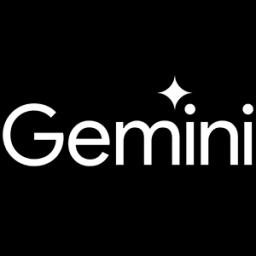
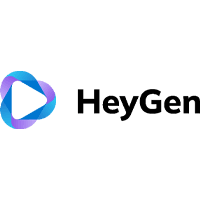
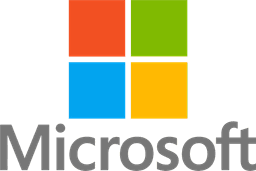
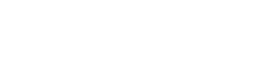
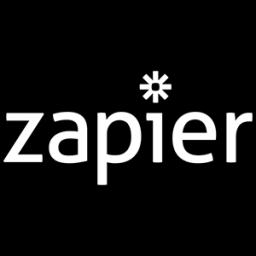
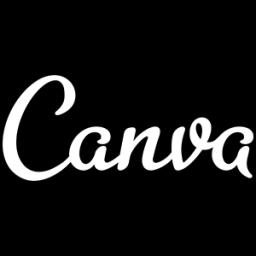
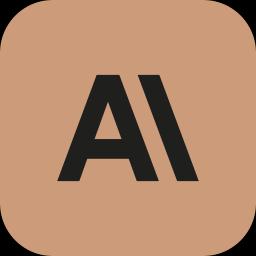
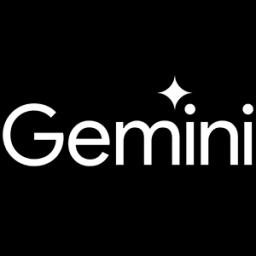
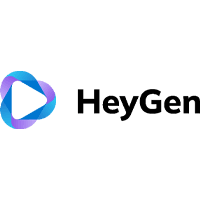
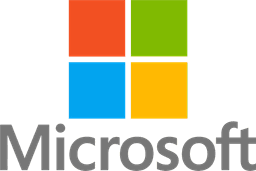
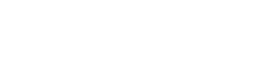
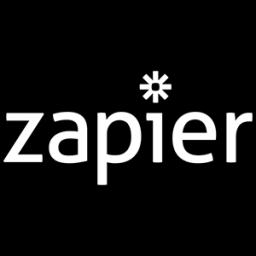
AI systems, notably those involved in coding and language tasks, benefit tremendously from trial and error during their development phases. This process has led to substantial improvements over generations of AI models, as seen with OpenAI's release of GPT-4.1, which showcased notable performance enhancements. These iterations, backed by extensive trial and error, align with Patel's insights into the necessity of scaling resources to achieve better results in AI development, a concept well articulated in the podcast (source).
Recent Advances: OpenAI's GPT-4.1 and Hybrid AI Models
OpenAI's recent introduction of GPT-4.1 marks a significant leap in the evolution of AI models, reinforcing the concept of the 'scaling era' in artificial intelligence. This latest iteration demonstrates a notable 21% improvement in performance compared to its predecessor, GPT-4.0, and showcases exceptional gains, particularly in coding scenarios, outperforming GPT-4.5 by 27%. Such advancements align with discussions highlighted in the EconTalk podcast featuring Dwarkesh Patel, where the emphasis was placed on the power of scaling—leveraging massive computational resources and vast datasets to push the boundaries of AI capabilities—rather than relying solely on algorithmic innovations [1](https://www.econtalk.org/the-past-and-future-of-ai-with-dwarkesh-patel/).
Moreover, GPT-4.1's success underscores the ongoing trend toward developing hybrid AI models that merge the scalability of traditional AI frameworks with reasoning and decision-making capabilities. This transformation is pivotal, as it addresses the inherent limitations of large language models, which often falter in tasks requiring nuanced understanding and common-sense reasoning. The fusion of scaling power and cognitive flexibility in these hybrid models reflects a broader quest within the AI research community to balance computational might with intelligent problem-solving, as explored in media like the podcast [1](https://www.econtalk.org/the-past-and-future-of-ai-with-dwarkesh-patel/).
In parallel with the technical strides seen in OpenAI's advancements, there's a growing recognition of the challenges that lie ahead, particularly regarding the social and ethical dimensions of AI. Current models still grapple with interpreting complex social cues and interactions, a limitation discussed by AI experts striving towards achieving Artificial General Intelligence (AGI) [1](https://www.econtalk.org/the-past-and-future-of-ai-with-dwarkesh-patel/). Addressing these challenges requires not just improved technology but also thoughtful incorporation of ethical frameworks to guide AI development responsibly.
Furthermore, the heightened focus on hybrid AI models is not just a technological refinement but a necessary evolution to tackle real-world applications with higher efficacy and safety. This shift aims to mitigate some of the existing issues, such as the high rate of errors in AI-generated code, which reportedly holds security vulnerabilities at a concerning 40% [7](https://www.rdworldonline.com/6-ai-megatrends-to-keep-an-eye-on-in-2025-from-hybrid-reasoning-to-superhuman-coding/). By enhancing the innate reasoning capabilities of models through hybridization, researchers hope to not only improve accuracy but also ensure that AI systems can autonomously handle complex, dynamic tasks in diverse environments.
Social Understanding: Limitations in Current AI Models
Current AI models often face significant challenges in comprehending social nuances and subtle human interactions, a limitation prominently highlighted in the EconTalk episode featuring Dwarkesh Patel. These models are typically trained on vast datasets predominantly composed of text, which might not fully capture the complexities of social situations or culturally specific interactions. As a result, AI can often misinterpret or fail to identify the context of conversations, leading to responses that may be perceived as inappropriate or irrelevant .
Learn to use AI like a Pro
Get the latest AI workflows to boost your productivity and business performance, delivered weekly by expert consultants. Enjoy step-by-step guides, weekly Q&A sessions, and full access to our AI workflow archive.
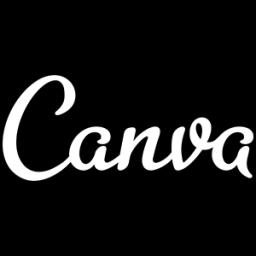
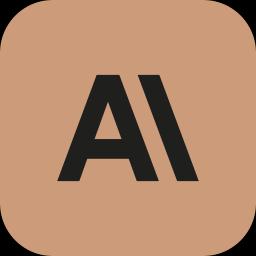
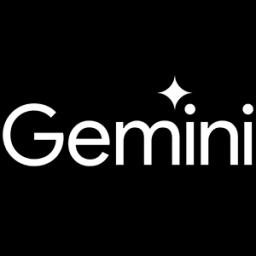
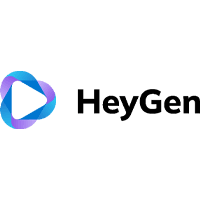
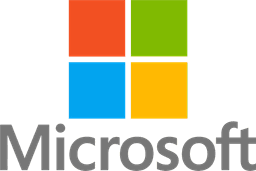
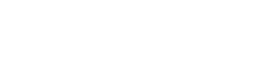
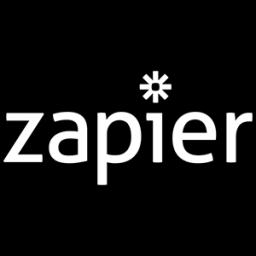
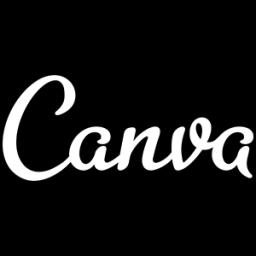
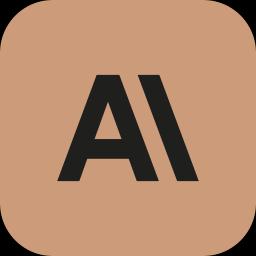
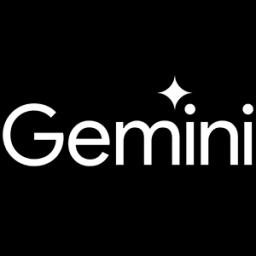
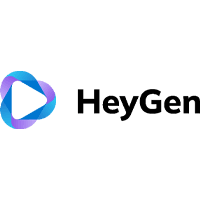
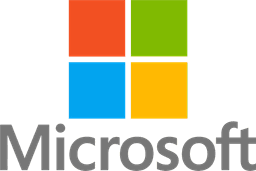
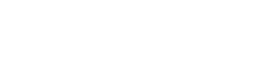
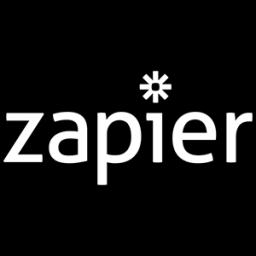
The fundamental issue with social understanding in AI is rooted in the lack of common sense reasoning capabilities, which are natural to humans but difficult for machines to grasp. This shortcoming is due to AI's dependency on statistical correlations rather than an understanding of context or intent behind human communication. Even though large language models have shown remarkable capabilities in language generation, they still struggle with tasks requiring empathy, emotion recognition, and social judgment .
Moreover, the integration of multimodal data, such as visual and auditory signals, into AI processing remains an ongoing research challenge. While current models excel in processing linguistic data, they often lack the sophistication needed to integrate other forms of data that humans naturally use to navigate social interactions. Therefore, to enhance AI's social understanding, there needs to be a concerted effort in developing models that can better interpret and respond to such nuanced social cues, as discussed in various AI-focused forums and podcasts .
Security Concerns with AI-Generated Code
The rise of AI-generated code has brought significant advancements in software development, but it has also introduced new security challenges. A major concern is the tendency of AI-generated code to contain vulnerabilities that could be exploited by malicious actors. It is estimated that about 40% of code suggestions from AI tools have security vulnerabilities, making human oversight crucial to mitigate potential risks. This highlights the critical need for developers to carefully review and verify AI-generated outputs to ensure robust security measures are implemented.
In the rapidly evolving landscape of AI, the concerns surrounding the security of AI-generated code resonate with the broader discussions about the limitations of current AI models. During a EconTalk podcast episode featuring Dwarkesh Patel, it was emphasized that while AI models have grown in capacity, they still struggle with real-world tasks that require common sense reasoning. This limitation extends to the generation of secure, reliable code, underscoring the importance of balancing AI capabilities with the necessity for human intervention.
The reliance on AI to assist in coding practices has introduced efficiency and innovation, but it is not without its caveats. As discussed in the EconTalk podcast, the future of AI lies in its ability to scale, but this does not automatically translate to enhanced security in code generation. Hybrid models that combine scaling with reasoning might offer a pathway to more securely generated AI code, addressing some of the inherent flaws currently seen in AI outputs.
As AI tools become more integrated into the software development lifecycle, the scrutiny over AI-generated code continues to grow. The ability of these tools to suggest and even autonomously produce code at scale poses new challenges for cybersecurity. The discussion on EconTalk suggests that while AI can streamline certain coding tasks, the inherent inaccuracies in such code require developers to remain vigilant about the potential security threats AI might inadvertently introduce.
Learn to use AI like a Pro
Get the latest AI workflows to boost your productivity and business performance, delivered weekly by expert consultants. Enjoy step-by-step guides, weekly Q&A sessions, and full access to our AI workflow archive.
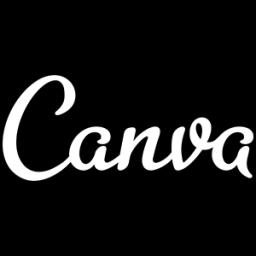
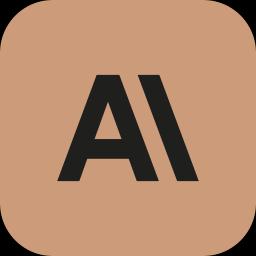
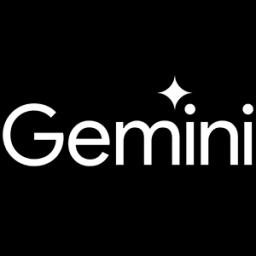
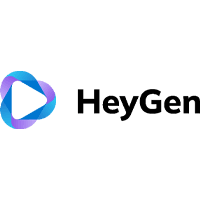
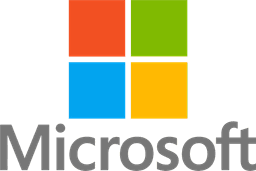
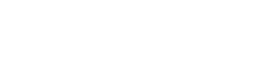
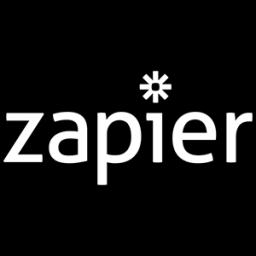
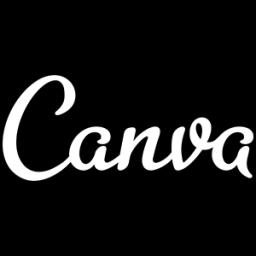
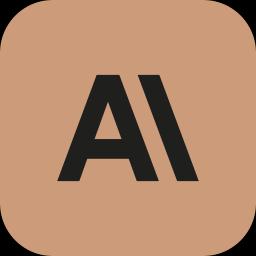
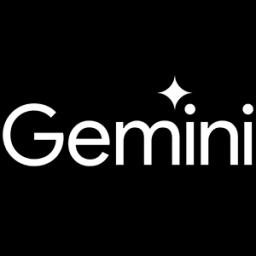
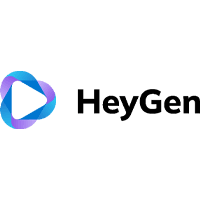
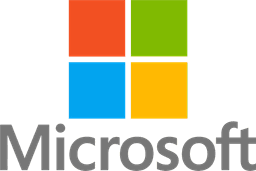
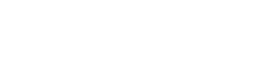
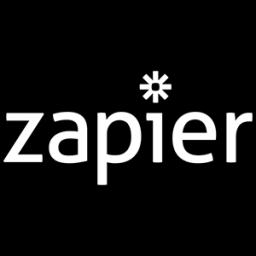
Expert Opinions on AI Developments
Looking ahead, the future of AI holds promising potential but is fraught with challenges, as described by experts discussing its implications on EconTalk. Economically, AI stands to revolutionize industries, driving productivity and reshaping job landscapes, while culturally, it poses questions about trust and information integrity. Politically, the dual-edged nature of AI reflects both the power to enhance communication and the potential to disrupt democratic processes through misinformation. These discussions reveal a landscape where AI's trajectory could lead to unprecedented growth and ethical dilemmas, warranting a careful and informed approach to harnessing AI's capabilities.
Public Reactions to AI Advancements
In the public sphere, reactions to advancements in artificial intelligence (AI) are as diverse as the technology's applications. Tech enthusiasts and industry leaders express profound excitement about the potential of AI to revolutionize industries, streamline operations, and solve complex problems. The emphasis on scaling, as discussed by Dwarkesh Patel in the EconTalk podcast, highlights an era where AI's capabilities grow exponentially through increased computational power and data availability, fostering positive anticipations about future innovations.
Yet, amidst optimism, there is significant public apprehension regarding the ethical and societal implications of these rapid advancements. Concerns about job displacement and income inequality, exacerbated by AI-driven automation, fuel debates across political and economic forums. In particular, the fear that AI could exacerbate existing social divides is echoed by various experts who caution about the unchecked proliferation of AI technologies. These concerns are not unfounded, as highlighted by issues such as AI's inaccuracies in coding and continued struggles with social interactions .
Moreover, the potential of achieving AGI (Artificial General Intelligence), as opposed to its speculative nature, elicits discussions rife with excitement and trepidation alike. The limitations of current models in handling tasks requiring common sense or understanding social nuances, as discussed by Patel, present significant barriers that foster public skepticism about AI's near-term potential to emulate human-like cognition. Public discourse often reflects a dichotomy where advancements are hailed for their promise while their implications are critically scrutinized.
Furthermore, the dramatic improvements seen with the release of models like OpenAI's GPT-4.1, demonstrating enhanced capabilities and performance, incite varied public reactions. While some view these developments as stepping stones towards AGI, others remain cautious, viewing AI's rapid pace as potentially disruptive if left unchecked. Discussions across social media and public forums often pivot around balancing innovation with ethical considerations, as consumers advocate for responsible AI deployment to mitigate risks such as misinformation and deepfakes that threaten democratic processes.
In conclusion, public reactions to AI advancements encapsulate a spectrum of perspectives. While technological progress offers opportunities for economic growth and innovation, it also necessitates robust discussions on ethics, equity, and societal impact. These conversations are crucial in shaping policies that ensure AI tools are utilized ethically and responsibly, aligning with shared values and societal goals.
Learn to use AI like a Pro
Get the latest AI workflows to boost your productivity and business performance, delivered weekly by expert consultants. Enjoy step-by-step guides, weekly Q&A sessions, and full access to our AI workflow archive.
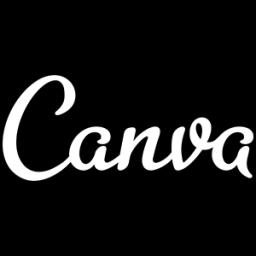
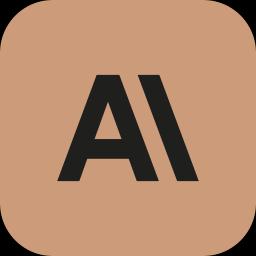
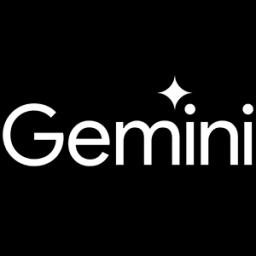
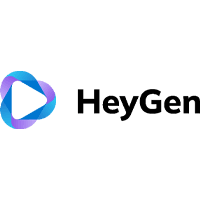
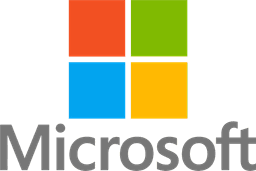
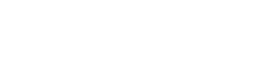
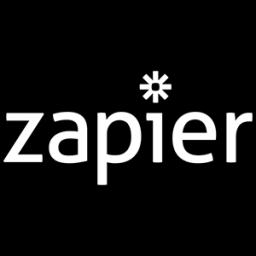
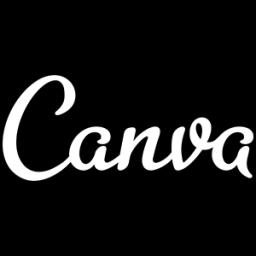
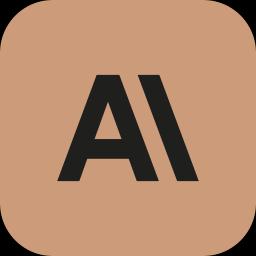
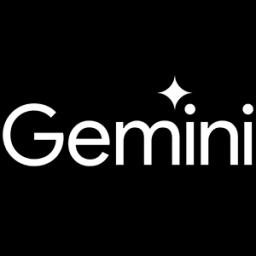
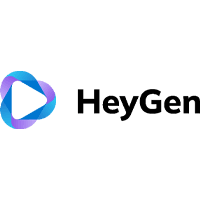
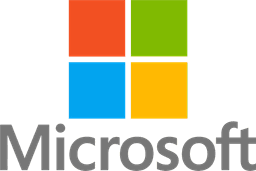
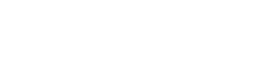
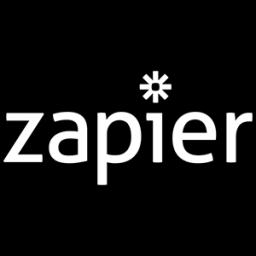
Future Implications of AI Advancements
The future implications of artificial intelligence (AI) advancements are profound and multifaceted, impacting various aspects of society. As highlighted in the EconTalk podcast with Dwarkesh Patel, one of the primary drivers of AI progress is the concept of scaling, which focuses on increasing computational resources and data availability rather than solely relying on new algorithmic developments. This approach has the potential to revolutionize industries by significantly enhancing productivity, yet it also raises concerns about job displacement and widening income inequality. The economic ramifications of such advancements are subject to ongoing debate, as illustrated by discussions on platforms like Vox, which examine the delicate balance between innovation and its socio-economic impact.
In the social sphere, AI advancements present both opportunities and challenges. AI's ability to generate vast amounts of content efficiently can bolster creativity and innovation but also poses risks related to misinformation and the erosion of trust. The use of AI in creating deepfakes and spreading targeted disinformation, as discussed in works from Wilson Center, underscores the potential for AI to manipulate public opinion and undermine democratic processes. This highlights the necessity for robust regulatory frameworks and ethical guidelines to mitigate these risks and ensure that AI technologies are used responsibly and transparently.
On the political front, the implications of AI advancements extend to national security and governance. The ability of AI-driven tools to influence and manipulate voter perceptions through sophisticated algorithms could potentially destabilize democratic institutions. The growing influence of AI in politics necessitates an urgent discourse on developing policies that prevent misuse while fostering innovation. This issue is particularly poignant in discussions about the role of AI in shaping future political landscapes, as highlighted in research from the Wilson Center.
The concept of Artificial General Intelligence (AGI) remains a focal point of interest and debate within the AI community. AGI's potential to perform any intellectual task that a human can presents both exciting possibilities and ethical quandaries. Current discourse suggests a dual approach involving scaling and algorithmic breakthroughs as necessary to achieve true AGI, a point of contention that echoes sentiments expressed in various expert discussions, such as those documented in the EconTalk podcast. As we continue to advance towards this ambitious goal, ongoing collaboration and dialogue among technologists, ethicists, and policymakers will be crucial in navigating the complex landscape of future AI developments.
Conclusion and Insights from the EconTalk Podcast
In this episode of the EconTalk podcast, host Russ Roberts engages with Dwarkesh Patel to explore the burgeoning landscape of artificial intelligence (AI) and its trajectory. Patel underscores the transformative power of scaling in AI's evolution, moving beyond mere algorithmic advances and focusing on the substantial benefits of increased compute and data availability. The discussion paints a vivid picture of how scaling is not only a hallmark of current AI progress but a cornerstone for future developments, posing both opportunities and limitations in the quest for Artificial General Intelligence (AGI).
One of the critical insights drawn from the conversation is the dual-edged nature of AI advancement. While scaling and hybrid models promise significant leaps forward, they also highlight the persistent challenges, such as the limitations of current language models in processing nuanced social interactions and contexts. This aspect of AI, as discussed by Patel, stresses the need for continued innovation in algorithm design alongside scaling efforts. The episode effectively captures the essence of modern AI debates, posing thought-provoking questions about the future of human-like AI intelligence and its societal impact.
Learn to use AI like a Pro
Get the latest AI workflows to boost your productivity and business performance, delivered weekly by expert consultants. Enjoy step-by-step guides, weekly Q&A sessions, and full access to our AI workflow archive.
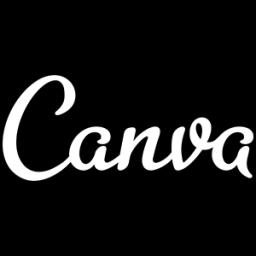
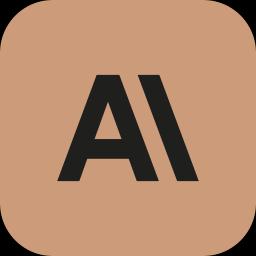
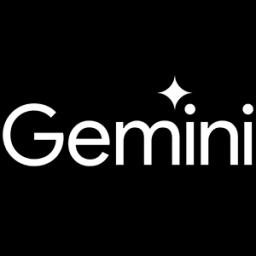
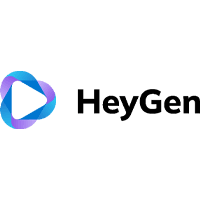
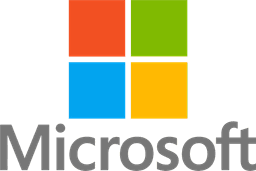
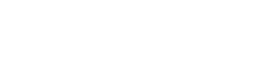
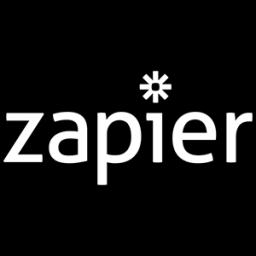
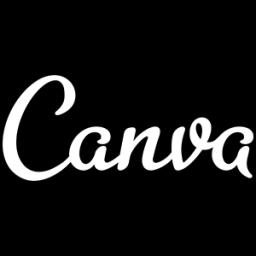
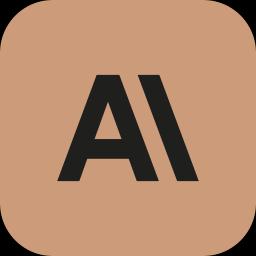
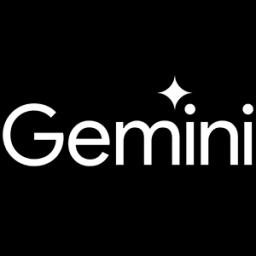
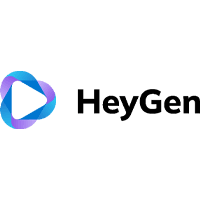
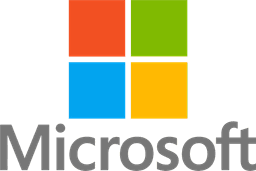
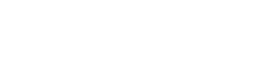
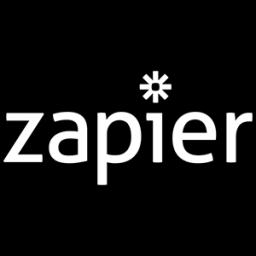
The podcast also delves into the economic and social ramifications of AI capabilities expanding at an unprecedented rate. The implications of AI scaling extend not only to technological innovation but also to broader economic shifts, such as job displacement and shifts in economic structures. Patel and Roberts discuss these themes candidly, acknowledging the role of AI in potentially exacerbating economic inequalities while also offering avenues for significant productivity gains through automation. These insights align with ongoing discussions and concerns about the ethical deployment of AI technologies in various sectors.
In light of recent advancements, the episode contrasts traditional algorithmic achievements with the promises held by new scaling approaches. Such advancements, as mentioned in this discussion, are mirrored in the release of OpenAI's GPT-4.1, which demonstrates the practical improvements achievable through scaling. The conversation navigates the fine line between optimism and cautious realism, acknowledging the spectrum of AI's potential to revolutionize industries, public spaces, and even individual lifestyles.
Ultimately, the EconTalk episode with Dwarkesh Patel presents a rich tapestry of ideas and concerns, weaving through the technical and ethical considerations of AI's future. Listeners are left with a deeper understanding of how scaling is reshaping AI's capabilities and potential pathways towards AGI, while also contemplating the profound implications these changes herald for society at large. The thoughtful analysis offered by Patel and Roberts underscores the importance of balancing technological enthusiasm with critical reflections on the socio-economic landscapes being altered by these innovations.