AI and Weather Prediction
AI to Revolutionize Weather Forecasting in India: A New Age of Predicting Extremes
Last updated:

Edited By
Mackenzie Ferguson
AI Tools Researcher & Implementation Consultant
Explore how Artificial Intelligence is set to transform weather forecasting in India, with the Indian government's "Mission Mausam" leading the way in utilizing AI to predict extreme weather events like heat waves, droughts, and floods. Despite challenges like data quality and the 'black box' nature of AI models, experts remain hopeful for more accurate and timely forecasting methods.
AI and Weather Forecasting: An Overview
In recent years, Artificial Intelligence (AI) has emerged as a promising tool in revolutionizing weather forecasting. Traditional forecasting relies heavily on numerical weather prediction (NWP) models, which are grounded in physics equations that simulate atmospheric behavior. These models, while effective, are sometimes limited in their ability to capture the complexities of weather systems, especially under the stress of climate change. This is where AI steps in, offering fresh perspectives by learning patterns directly from vast datasets without the constraints of pre-defined equations. AI's capability to unlock hidden relationships and patterns within the data signals a transformation in how extreme weather events like heat waves, floods, and droughts might be predicted more accurately [^1^](https://www.thehindu.com/sci-tech/science/artificial-intelligence-india-forecasting-extreme-weather/article69452413.ece).
India has recognized the potential of AI in weather forecasting through its ambitious "Mission Mausam" initiative. Launched with a substantial budget, this initiative seeks to enhance India's weather and climate observation capabilities by integrating AI-driven methodologies. The program aims to refine traditional earth system models and incorporate AI to address complex meteorological challenges. By adopting innovative AI techniques, "Mission Mausam" represents a significant step in leveraging technology to improve accuracy and timeliness in weather predictions, thus mitigating the impacts of extreme weather on its populace [^1^](https://www.thehindu.com/sci-tech/science/artificial-intelligence-india-forecasting-extreme-weather/article69452413.ece).
Learn to use AI like a Pro
Get the latest AI workflows to boost your productivity and business performance, delivered weekly by expert consultants. Enjoy step-by-step guides, weekly Q&A sessions, and full access to our AI workflow archive.
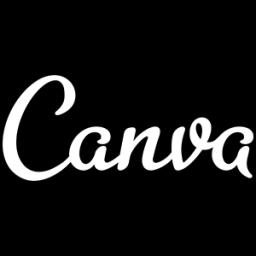
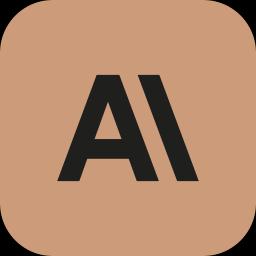
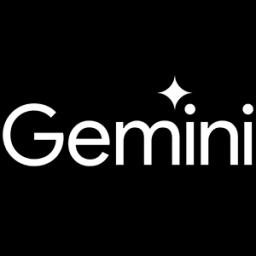
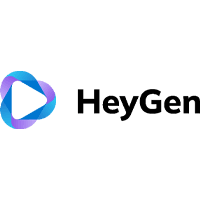
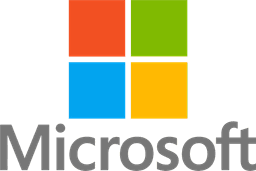
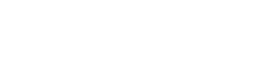
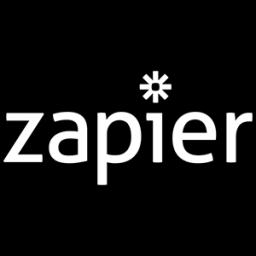
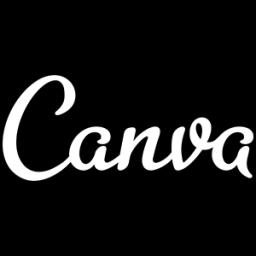
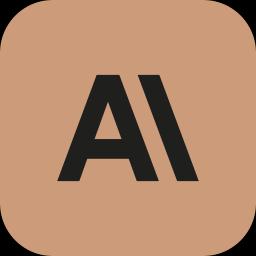
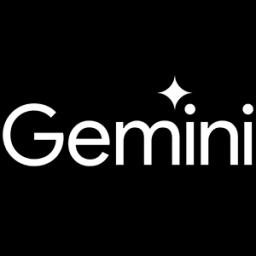
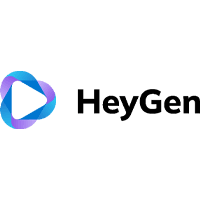
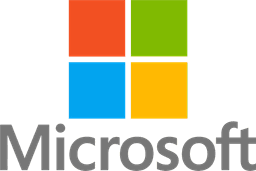
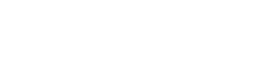
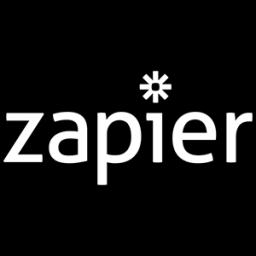
However, the integration of AI in weather forecasting is not without hurdles. One of the primary challenges involves the quality and quantity of data needed for AI models. Accurate forecasting demands large volumes of high-quality data to train AI systems effectively. Additionally, there's the "black box" problem, where the decision-making process of AI models is not always transparent, raising issues of trust and understanding. The complexity and chaotic nature of weather systems further compound these challenges, alongside the need for experts who are skilled in both meteorology and AI [^1^](https://www.thehindu.com/sci-tech/science/artificial-intelligence-india-forecasting-extreme-weather/article69452413.ece).
Despite these challenges, researchers remain optimistic about the potential of AI to enhance the accuracy of extreme weather predictions. AI's ability to handle complex data and recognize patterns can prove invaluable in predicting events that defy traditional forecasting methods. Such capability could lead to better preparedness and response strategies, ultimately saving lives and resources. The exploration of hybrid models that blend AI with physics-based approaches is a promising avenue, potentially combining the best of both worlds to improve weather predictions and resilience against climate impacts [^1^](https://www.thehindu.com/sci-tech/science/artificial-intelligence-india-forecasting-extreme-weather/article69452413.ece).
Mission Mausam: India's Initiative for Improved Weather Prediction
"Mission Mausam" stands as a significant milestone in India's endeavor to transform its weather forecasting capabilities by leveraging the power of Artificial Intelligence (AI). This initiative, which began in 2024 with a substantial budget allocation, seeks to modernize India's approach to predicting weather phenomena, particularly extreme weather events, through the integration of AI-driven methods and enhanced earth system models. As traditional forecasting relies heavily on numerical models that use physics-based equations, AI introduces a new frontier by learning from vast datasets, identifying patterns that these models may overlook. This approach promises not only improved accuracy but also the ability to provide timely warnings for events like heatwaves and floods, which are increasingly common in the context of climate change .
The potential of AI in weather prediction is significant, yet it comes with its set of challenges. The "black box" nature of AI models, where the decision-making process is not always transparent, raises concerns about their accountability and trustworthiness. Moreover, the success of AI applications in meteorology is contingent upon the availability and quality of data, which remains a considerable hurdle in many parts of India. The shortage of skilled professionals who are adept in both meteorology and AI also poses a challenge, as does the infrastructural readiness to support such advanced technology . Despite these challenges, researchers and policymakers remain optimistic about AI's role in refining prediction models and improving climate resilience strategies.
Learn to use AI like a Pro
Get the latest AI workflows to boost your productivity and business performance, delivered weekly by expert consultants. Enjoy step-by-step guides, weekly Q&A sessions, and full access to our AI workflow archive.
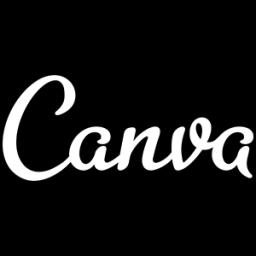
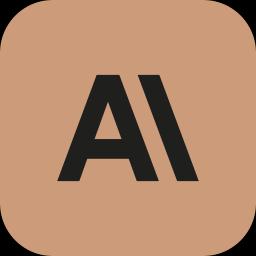
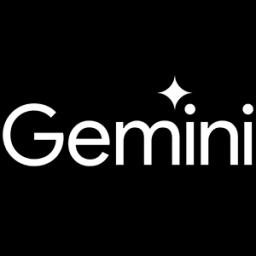
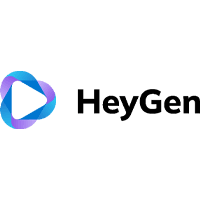
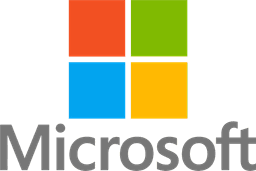
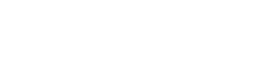
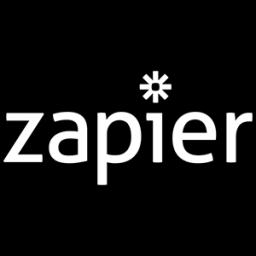
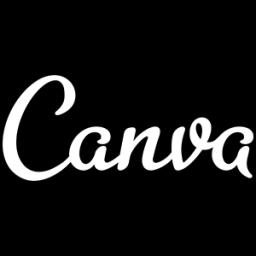
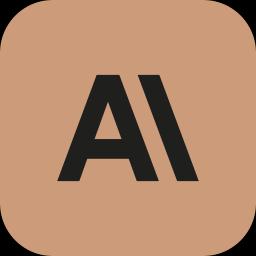
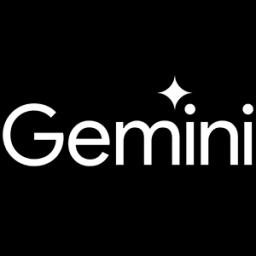
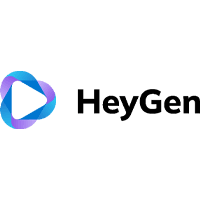
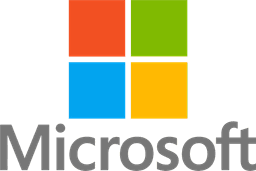
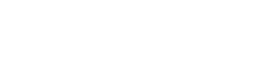
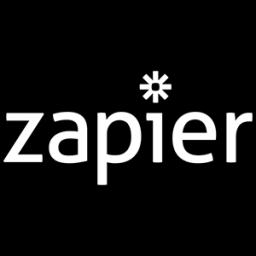
One of the innovative aspects of "Mission Mausam" is the exploration of hybrid climate models. These models aim to integrate the strengths of traditional physics-based approaches with cutting-edge AI and machine learning techniques. By doing so, they could potentially overcome the limitations inherent in using either model independently. This hybridization is crucial as it could enhance forecast reliability and accuracy, thereby supporting India's broader objectives of disaster preparedness and management. The success of these models, however, is heavily dependent on continuous improvements in data collection technologies and the development of specialized AI models that are tailored to the unique weather patterns of the region .
The global implications of India's "Mission Mausam" extend beyond national borders, as the initiative underscores the necessity for international cooperation in the battle against the escalating impacts of climate change. By enhancing their forecasting capabilities, India can contribute to a broader global understanding of weather patterns and climate dynamics. This initiative also highlights the need for robust frameworks surrounding data ownership, privacy, and policy, as AI technologies become increasingly embedded in national security and international collaborations. It is clear that while the challenges are manifold, the integration of AI and hybrid models in weather forecasting presents an unprecedented opportunity to safeguard infrastructure, enhance agricultural sustainability, and ultimately, save lives through better-prepared disaster response strategies .
Challenges in AI-Based Weather Prediction
Artificial Intelligence (AI) is bringing a transformative dimension to weather prediction, especially in predicting extreme weather events, yet it faces a slew of challenges. AI-driven models differ from traditional numerical weather prediction (NWP) techniques which rely heavily on physics to simulate atmospheric conditions. Although AI models promise significant advancements by analyzing vast datasets to identify predictive patterns, they struggle with several inherent challenges [1](https://www.thehindu.com/sci-tech/science/artificial-intelligence-india-forecasting-extreme-weather/article69452413.ece).
One major challenge is data quality and quantity. AI models require extensive and high-quality datasets to function accurately, which can be a significant bottleneck. In regions like India, disparities in data availability—especially in remote areas—pose a substantial challenge to the development and deployment of effective AI-based weather forecasting models [2](https://www.preventionweb.net/news/data-drought-challenge-ai-weather-forecasting-india). Additionally, the quality of the data from various sources must align to ensure consistent predictions. This requirement strains existing infrastructure and highlights the need for robust data management systems.
Moreover, AI models are often described as 'black boxes,' as they can offer results without clearly explaining their internal workings. This opacity can lead to trust issues among users and stakeholders, who may find it challenging to rely on predictions they cannot fully understand or validate. This "black box" challenge extends to the need for experts well-versed in both meteorology and AI, making interdisciplinary expertise critical yet scarce [4](https://www.insightsonindia.com/2025/04/23/ai-in-weather-forecasting/).
The complexity of weather systems themselves adds another layer of difficulty. Weather is inherently chaotic, and climate change exacerbates this unpredictability, making it difficult for AI models to reliably predict extreme weather events. This unpredictability demands sophisticated models that can handle nonlinear and chaotic systems, often beyond current capabilities [7](https://www.thehindu.com/sci-tech/science/artificial-intelligence-india-forecasting-extreme-weather/article69452413.ece).
Learn to use AI like a Pro
Get the latest AI workflows to boost your productivity and business performance, delivered weekly by expert consultants. Enjoy step-by-step guides, weekly Q&A sessions, and full access to our AI workflow archive.
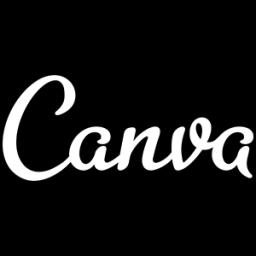
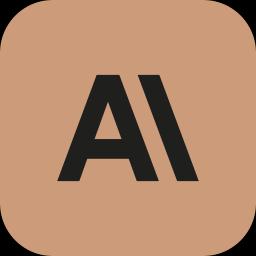
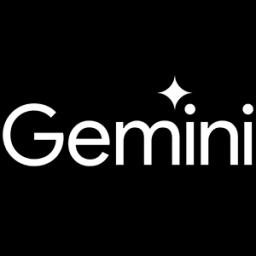
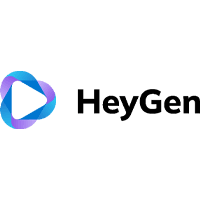
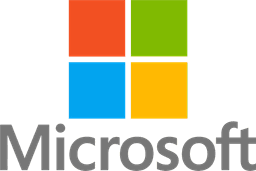
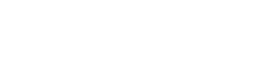
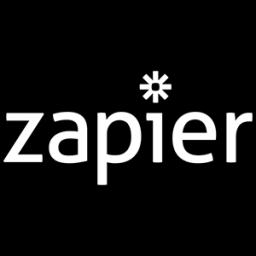
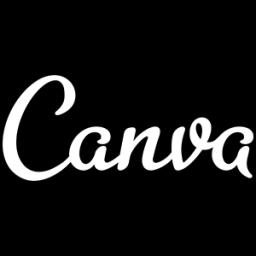
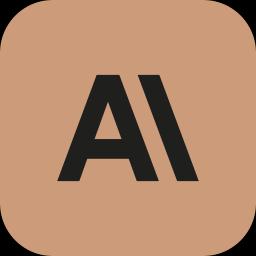
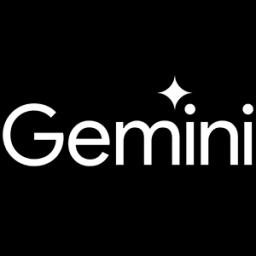
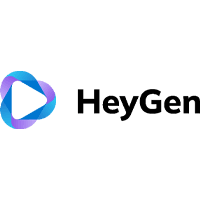
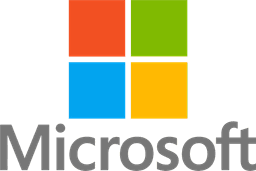
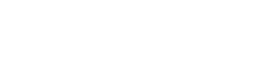
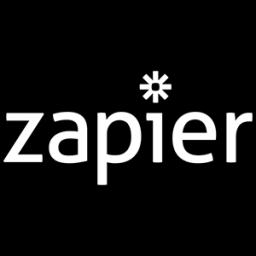
Hybrid models, which combine traditional physics-based methods with AI, present a promising way forward. These models aim to integrate the scientific rigor of physics with the data-driven insights of AI, increasing the accuracy and trustworthiness of forecasts. However, significant work remains in refining these hybrid models and ensuring they effectively leverage their combined strengths [3](https://hess.copernicus.org/articles/27/1865/2023/). Initiatives like India’s 'Mission Mausam' are exploring these avenues, aiming to overcome the limitations currently faced and optimize AI's potential in weather prediction [1](https://www.thehindu.com/sci-tech/science/artificial-intelligence-india-forecasting-extreme-weather/article69452413.ece).
The Role of AI in Predicting Extreme Weather Events
Artificial Intelligence (AI) is increasingly becoming a pivotal tool in predicting extreme weather events, a critical area of concern globally. This innovative approach marks a significant shift from traditional weather forecasting methods, which often rely solely on numerical weather prediction (NWP) models. These traditional models simulate atmospheric behavior based on physics equations, offering a structured yet sometimes limited perspective on weather patterns. In contrast, AI models harness the power of machine learning to analyze enormous datasets, identifying intricate patterns and relationships unperceived by conventional methods .
India's ambitious "Mission Mausam" exemplifies the nation's dedication to integrating AI into weather forecasting. Launched with a substantial budget, this initiative aims to refine weather predictions, improve climate observations, and enhance modeling techniques across the country. However, the implementation of AI in such complex systems is not without challenges. These include the need for high-quality data and overcoming the 'black box' nature of AI systems, which makes it challenging to understand the reasoning behind AI-driven predictions. Moreover, there is a pressing requirement for professionals adept in both meteorology and AI/ML technologies .
One of the promising aspects of AI in weather forecasting is its potential to accurately predict extreme weather events like heat waves, droughts, and floods. Through sophisticated data analysis, AI can highlight subtle climatic shifts that traditional methods might overlook, thus enhancing early warning systems and potentially saving lives and property. Despite its promise, the statistical definition of "extreme" weather events and the trustworthiness of AI models continue to pose significant challenges .
Hybrid models, which combine AI/ML methodologies with traditional physics-based climate models, are gaining traction as a balanced approach to weather predictions. These models leverage the strengths of both scientific paradigms, providing more reliable and accurate forecasts. Such hybrid models are not only aimed at enhancing prediction accuracy but also at addressing the skepticism surrounding the transparency and interpretability of purely AI-driven models. By integrating the comprehensive data analysis capabilities of AI with the proven reliability of physics-based models, these hybrid systems hold the promise of revolutionizing weather forecasting processes .
Hybrid Climate Models: Combining Traditional and AI Approaches
Hybrid climate models represent an innovative approach that seeks to integrate the strengths of both traditional and artificial intelligence (AI) methodologies in climate forecasting. Traditional models rely on well-established physical laws and equations to simulate atmospheric conditions, allowing for robust predictions of weather patterns. However, these models can be limited by their computational intensity and the inherent complexity of atmospheric systems, which might not fully capture the chaotic nature of weather, especially during extreme events.
Learn to use AI like a Pro
Get the latest AI workflows to boost your productivity and business performance, delivered weekly by expert consultants. Enjoy step-by-step guides, weekly Q&A sessions, and full access to our AI workflow archive.
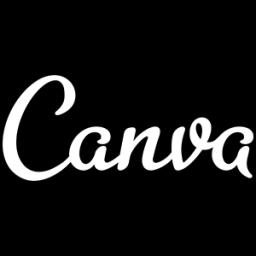
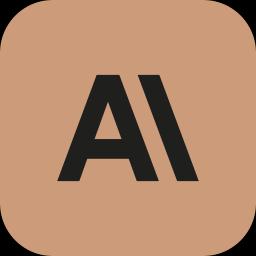
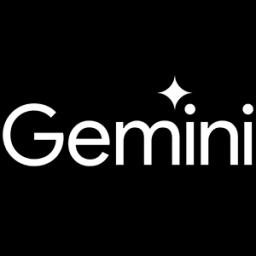
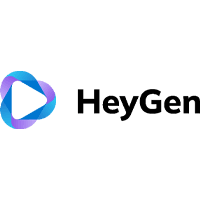
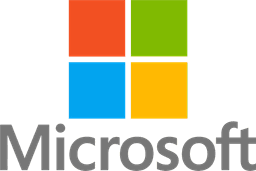
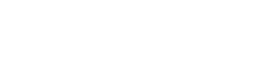
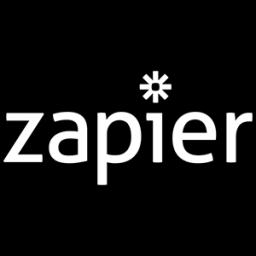
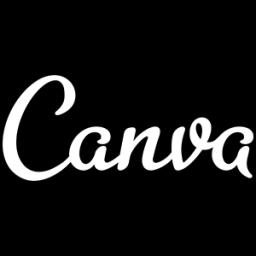
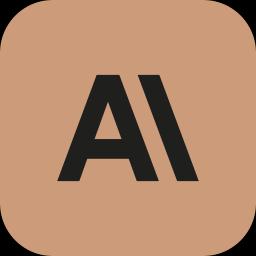
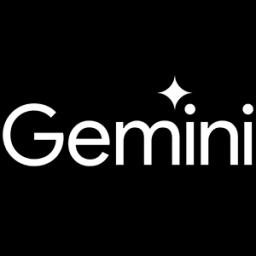
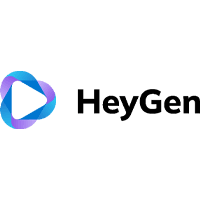
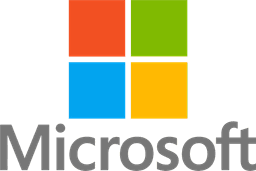
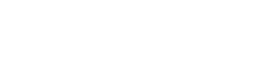
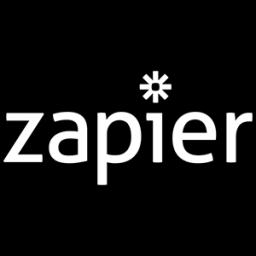
On the other hand, AI models have introduced a transformative potential by leveraging vast amounts of data to discern complex patterns and relationships that may be elusive to traditional methods. AI's ability to process and analyze large datasets enables the identification of subtle trends and interactions within climate systems, leading to possibly more nuanced predictions. However, AI models often face challenges such as the "black box" phenomenon, where the internal decision-making processes are not easily interpretable by human operators, and the need for high-quality data to ensure accuracy.
Hybrid climate models aim to bridge the advantages of these two methodologies, creating a synergistic relationship that enhances overall forecasting capabilities. By assimilating AI's pattern recognition prowess with the structured, physics-based insights of traditional models, researchers can develop more robust models that potentially predict climate behaviors with greater precision. This fusion not only boosts the accuracy of forecasts but also improves computational efficiency, as AI can optimize the processes handled by physics-based approaches.
In practical applications, hybrid models can be particularly beneficial for forecasting extreme weather events, where precise predictions are critical for timely disaster preparedness and risk reduction. India's "Mission Mausam" initiative exemplifies efforts to incorporate AI into traditional meteorological practices to enhance weather prediction accuracy. Despite challenges such as data quality and the demand for interdisciplinary expertise, hybrid models hold the promise of improved forecasting systems worldwide, as they can reduce operational costs and heighten the precision of both short-term and long-term weather predictions.
Furthermore, successful implementation of hybrid models requires addressing the trustworthiness and interpretability of AI components, while ensuring adequate data availability and infrastructure. Continuous collaboration between meteorologists, AI specialists, and policymakers is essential to navigate the challenges and unlock the full potential of these advanced systems. By integrating cutting-edge AI technology into the foundational framework of traditional climate models, the hybrid approach stands as a forward-thinking strategy poised to revolutionize the field of weather forecasting.
Global Advancements in AI Weather Forecasting
The global landscape of weather forecasting is undergoing a remarkable transformation with the integration of Artificial Intelligence (AI) technologies. In particular, AI's potential to enhance the precision and reliability of weather forecasts is becoming increasingly significant. Traditional weather forecasting methods, largely grounded in physics-based numerical weather prediction models, are now being complemented by AI and machine learning. These advanced technologies analyze vast amounts of historical and real-time data, identifying complex patterns and relationships that might elude conventional models. This paradigm shift is poised to improve the accuracy of forecasts, particularly for extreme weather events, which are notoriously difficult to predict accurately due to their complex and chaotic nature.
Countries around the world are making significant strides in leveraging AI for weather forecasting. For instance, the European Centre for Medium-Range Weather Forecasts (ECMWF) recently unveiled the world's first fully operational AI-driven weather forecasting system. This innovation not only improves forecast accuracy but also reduces computational energy consumption, signaling a more sustainable approach to meteorological advancements. Similarly, Google DeepMind's AI ensemble known as GenCast has outperformed traditional physics-based systems in accuracy, heralding a new era of AI dominance in meteorology. These advancements underscore the potential for AI to reshape traditional weather forecasting models and set new standards for precision and speed.
Learn to use AI like a Pro
Get the latest AI workflows to boost your productivity and business performance, delivered weekly by expert consultants. Enjoy step-by-step guides, weekly Q&A sessions, and full access to our AI workflow archive.
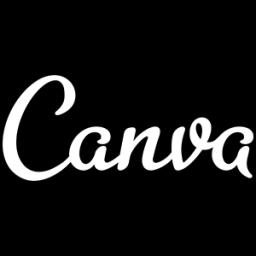
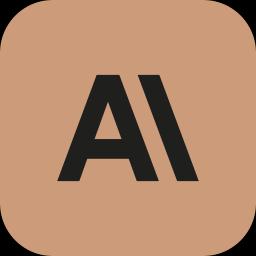
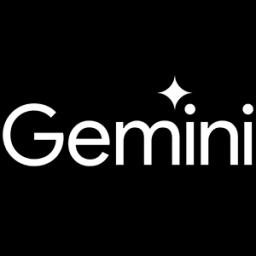
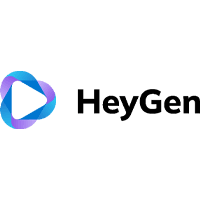
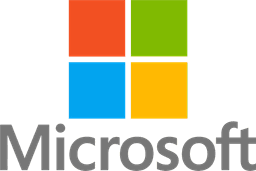
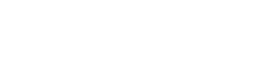
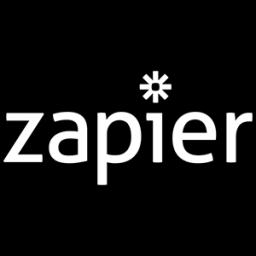
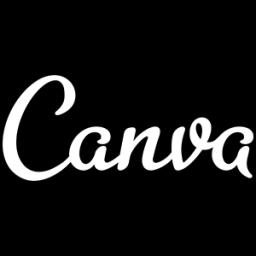
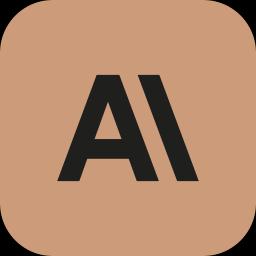
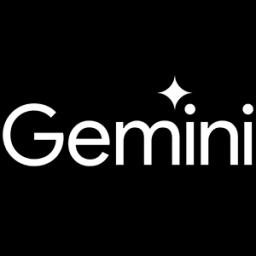
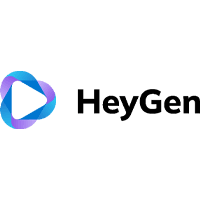
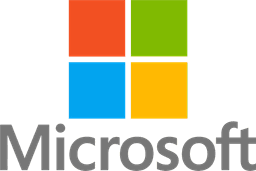
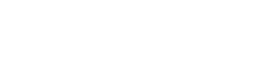
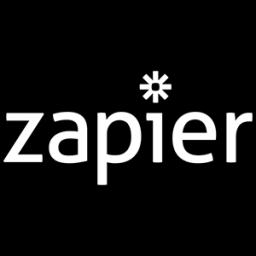
In India, the "Mission Mausam" initiative exemplifies the country's commitment to harnessing AI for enhanced weather prediction. Launched by the Indian government, this ambitious program aims to improve the country's preparedness for extreme weather events by developing sophisticated AI-driven models. Despite the challenges of data quality, the complexity of weather systems, and the need for skilled professionals, there is a palpable optimism about the potential of AI to address these issues. The "Mission Mausam" initiative highlights the strategic importance of AI in mitigating the adverse impacts of climate change by facilitating more accurate and timely weather forecasts.
Hybrid approaches combining AI and physics-based methods are gaining traction worldwide. By integrating the strengths of both methodologies, these hybrid models aim to enhance the reliability of weather forecasts. Traditional models benefit from the strong theoretical foundation and real-time data assimilation, while AI models contribute their ability to recognize patterns and make rapid predictions. This synthesis of AI and traditional methodologies is particularly promising for improving forecasts of extreme weather events such as floods and droughts. As researchers continue to fine-tune these hybrid systems, the global capacity to respond to and prepare for severe weather conditions will undoubtedly be strengthened.
Despite the promise of AI, challenges remain in its widespread adoption for weather forecasting. The "black box" nature of AI, which makes it difficult to interpret the decision-making processes of these models, poses a significant barrier. Moreover, the demand for high-quality datasets and interdisciplinary expertise in both meteorology and AI/ML is crucial yet challenging to fulfill. Addressing these challenges requires concerted efforts in data management, education, and international collaboration. However, the potential benefits of integrating AI into weather forecasting, such as improved accuracy, resource management, and disaster preparedness, provide compelling reasons to overcome these obstacles.
Expert Opinions on AI in Weather Prediction
The integration of AI in weather forecasting has been a topic of great interest, as experts weigh in on its potential benefits and challenges. A significant viewpoint is that AI can serve as a revolutionary tool for enhancing the accuracy and efficiency of weather predictions. Unlike traditional methods that rely heavily on numerical weather prediction models rooted in physics equations, AI leverages vast datasets to discern patterns and correlations, offering insights that may not be evident through conventional means. For instance, India's "Mission Mausam" reflects a strategic move to adopt AI for better forecasting outcomes. Experts highlight that this initiative could markedly improve predictions, especially for extreme weather events like heat waves, droughts, and floods ().
However, despite the optimism surrounding AI's potential, experts also express caution. One of the primary concerns is the requisite for high-quality datasets, without which AI models might falter in reliability. The issue is compounded by the "black box" nature of AI, which makes it challenging to interpret how decisions or predictions are made within these models. Furthermore, a shortage of professionals proficient in both AI technologies and meteorology could hinder effective implementation. The complexity of weather systems, especially in the context of climate change, adds another layer of difficulty to harnessing AI for accurate and trustworthy forecasts ().
A promising solution explored by experts is the development of hybrid climate models. These models combine the strengths of traditional physics-based climate systems with the capabilities of AI and machine learning. Such integration is expected to improve forecast accuracy and reliability by capturing the nonlinear relationships in atmospheric data while maintaining a reduced computational load. Success, however, depends on addressing existing data gaps and enhancing the interdisciplinary expertise required to effectively manage these advanced modeling systems ().
Learn to use AI like a Pro
Get the latest AI workflows to boost your productivity and business performance, delivered weekly by expert consultants. Enjoy step-by-step guides, weekly Q&A sessions, and full access to our AI workflow archive.
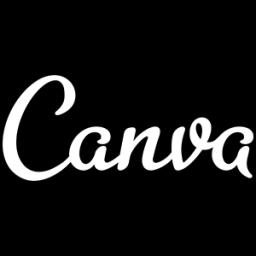
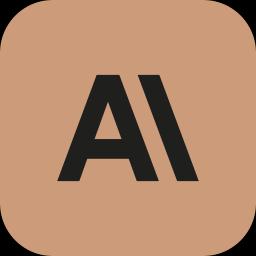
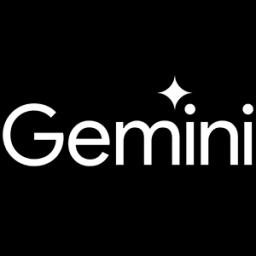
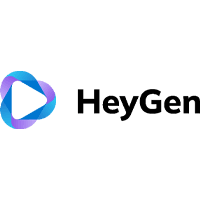
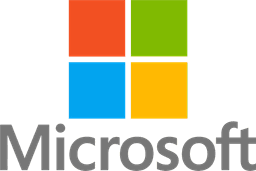
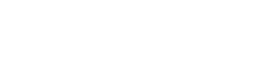
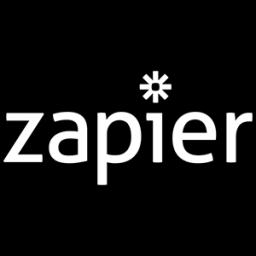
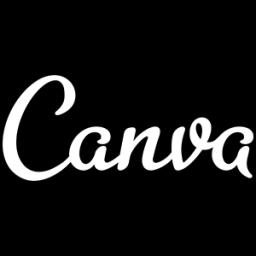
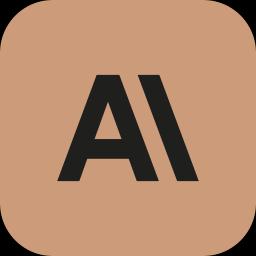
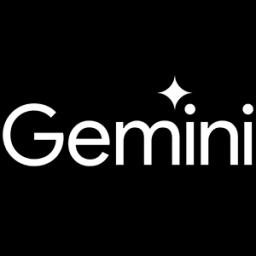
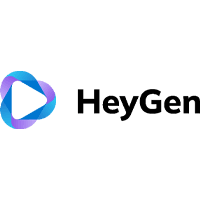
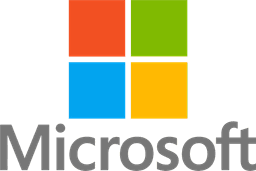
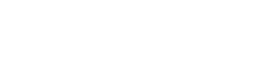
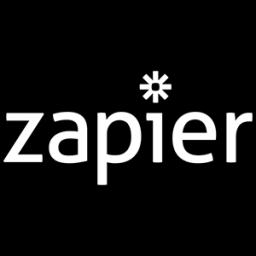
Future Implications of AI-Driven Weather Forecasting
As we stand on the brink of a technological revolution, AI-driven weather forecasting promises to reshape our understanding and prediction of weather patterns, especially in regions frequently beset by extreme weather events. The potential of AI to enhance the accuracy and timeliness of weather forecasts is particularly relevant to countries like India, where initiatives such as "Mission Mausam" are underway to harness the power of AI for improved forecasting [source]. By leveraging vast datasets, AI algorithms can detect patterns invisible to traditional methods, thereby delivering insights that could revolutionize how we prepare for and respond to climatic threats.
AI-driven models bring unprecedented possibilities to weather forecasting, enabling meteorologists to predict extreme events like heat waves, floods, and droughts with a degree of precision unattainable by conventional physics-based methods. This leap forward is facilitated by AI's ability to process and analyze immense amounts of data swiftly, identifying correlations and trends that are often overlooked in traditional models. AI's integration into climate science is not without its challenges, however. Issues related to data integrity, the opaqueness of AI models, and the necessity of integrating human expertise remain substantial hurdles [source].
The future implications of integrating AI into weather forecasting extend beyond technical forecasts to affect economic stability, social welfare, and political strategies. Economically, accurate weather predictions can mitigate disaster impacts, optimize agricultural output, and refine risk assessment models for insurance industries, thereby contributing to overall economic resilience. Socially, enhanced forecasts lead to increased public safety and better disaster preparedness, underscoring the societal value of timely and reliable weather predictions. Politically, the deployment of AI in weather forecasting necessitates robust international cooperation, the formulation of informed climate policies, and discussions around data privacy and technological dependencies [source].
In the quest to harness AI's full potential in weather forecasting, hybrid models that combine traditional physics-based approaches with cutting-edge AI techniques are gaining traction. These models aim to capitalize on the strengths of each method, offering a promising path towards more reliable and accurate forecasts. While AI excels in speed and scalability, especially for short-term predictions, the probabilistic aspect of physics-based models helps mitigate some of the uncertainties inherent in AI predictions. The synergy between these approaches is expected to catalyze advancements in early warning systems and climate science as a whole, offering new tools to combat the challenges posed by climate change [source].