Negation issues put medical AI's reliability under the spotlight
AI's Blind Spot: The Trouble with Understanding 'No' in Medicine
Last updated:

Edited By
Mackenzie Ferguson
AI Tools Researcher & Implementation Consultant
AI's struggle with negation poses potential risks in medical contexts, where misunderstandings of negative statements can lead to misdiagnosis and patient safety concerns. The article explores why AI finds negation challenging, its implications for healthcare, and emerging solutions to improve AI's handling of 'no' in critical applications.
Introduction to AI's Negation Challenge in Healthcare
The introduction of artificial intelligence into healthcare has marked a significant turning point in the way medical diagnoses and patient care are approached. However, AI's struggle with understanding negation presents a formidable challenge in this domain. As discussed in the article from New Scientist, AI's difficulty with processing negative statements can lead to grave misinterpretations, particularly in medical imaging and diagnostics. This challenge is primarily because AI models depend on statistical patterns rather than a deep understanding of logical constructs, such as negation [New Scientist](https://www.newscientist.com/article/2480579-ai-doesnt-know-no-and-thats-a-huge-problem-for-medical-bots/). As these technologies become more integrated into medical settings, the ramifications of this fundamental limitation become increasingly critical to address.
Understanding AI's Difficulty with Negation
Artificial Intelligence (AI) has become an integral part of various industries, but its challenges with processing language nuances—especially negation—pose a significant problem, particularly in sensitive sectors like healthcare. Understanding negation requires more than identifying statistical correlations in language data; it demands a logical comprehension of how words interact to convey a denial or contradiction of a premise [1](https://www.newscientist.com/article/2480579-ai-doesnt-know-no-and-thats-a-huge-problem-for-medical-bots/). Unlike humans, AI models primarily learn from patterns observed in data, which often lack explicit negation examples, thus leading to frequent misinterpretations of sentences containing 'no' or 'not.'
Learn to use AI like a Pro
Get the latest AI workflows to boost your productivity and business performance, delivered weekly by expert consultants. Enjoy step-by-step guides, weekly Q&A sessions, and full access to our AI workflow archive.
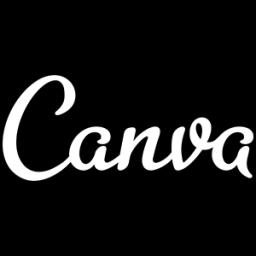
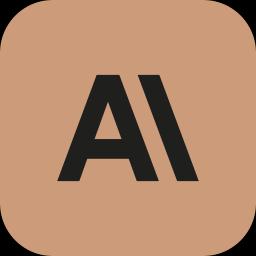
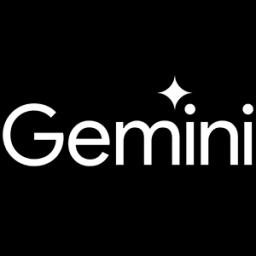
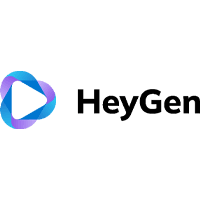
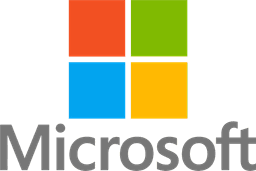
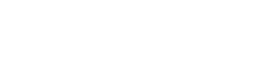
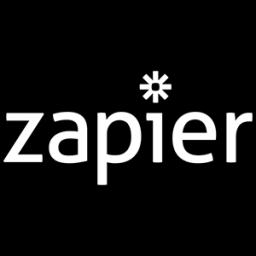
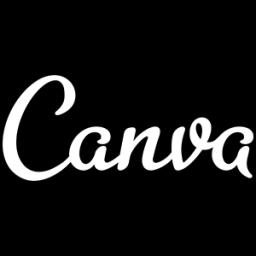
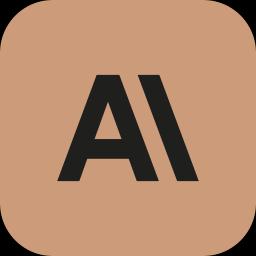
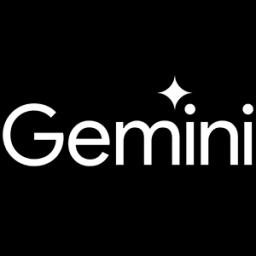
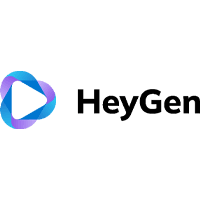
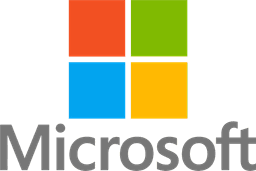
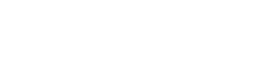
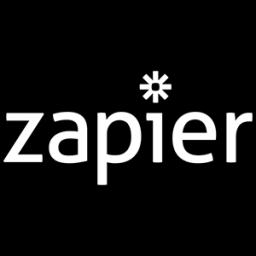
In medical contexts, AI's inability to accurately understand negation can have dire consequences. For instance, failing to accurately process a phrase like 'no signs of disease' as opposed to 'signs of disease' can result in significant clinical errors. These errors might include misdiagnosis, inappropriate treatments, and, consequently, detrimental health outcomes. This issue is exacerbated by the high dependency on AI for diagnostic imaging and patient information analysis, where precision is paramount [1](https://www.newscientist.com/article/2480579-ai-doesnt-know-no-and-thats-a-huge-problem-for-medical-bots/).
Efforts to mitigate these challenges are ongoing, with researchers focusing on enhancing AI models through enriched training datasets and developing algorithms specifically designed to better handle negation. Data augmentation techniques and the development of specialized negation-focused datasets represent some of the ways researchers are attempting to address this 'blind spot' in machine understanding [9](https://www.forwardpathway.us/ais-blind-spot-in-understanding-negation-potential-risks-and-responses-in-high-risk-applications). Despite these efforts, ensuring AI systems can safely and effectively handle negation remains a critical area of research necessary for the future of AI implementation in medicine and other high-risk fields.
Consequences of Negation Misunderstanding in Medical AI
The misunderstanding of negation in AI systems presents a profound challenge, especially in the medical field, where the stakes for accuracy are incredibly high. Medical AI applications often struggle with negation, which means that a negative statement such as 'no signs of pneumonia' could be misinterpreted as 'signs of pneumonia.' This misunderstanding can lead to severe misdiagnoses, inappropriate treatments, or even delayed intervention, all of which compromise patient safety and healthcare efficacy [source].
Current Research to Address Negation Issues
In recent years, researchers have made significant strides in addressing the challenges AI faces with negation, especially within medical contexts. Leveraging more sophisticated algorithms and improved natural language processing techniques, efforts are being directed towards reducing AI's misinterpretations of negative statements. These innovations seek to train AI models to better understand the linguistic nuances that are often overlooked in standard training data. By integrating databases specifically designed to include a wide array of negation examples, researchers aim to bolster AI's ability to discern positive and negative cues accurately. This not only enhances the reliability of medical bots but also improves their overall diagnostic efficiency, leading to safer outcomes for patients. For a deeper understanding of these challenges and solutions, refer to this article.
Learn to use AI like a Pro
Get the latest AI workflows to boost your productivity and business performance, delivered weekly by expert consultants. Enjoy step-by-step guides, weekly Q&A sessions, and full access to our AI workflow archive.
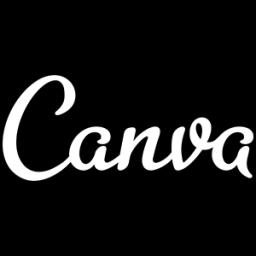
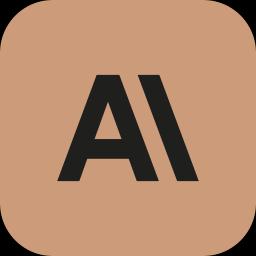
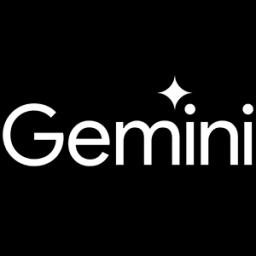
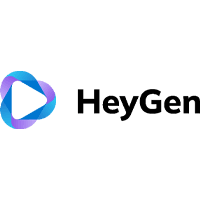
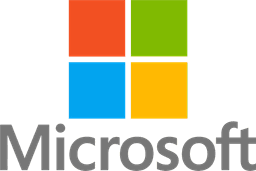
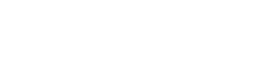
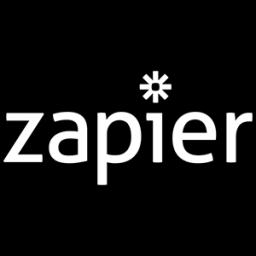
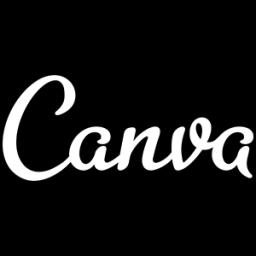
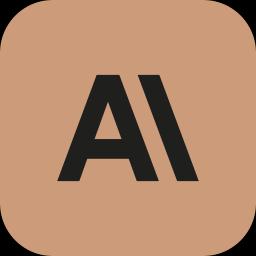
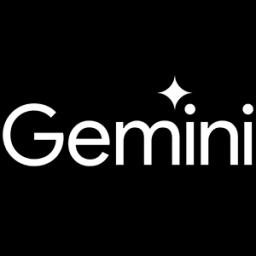
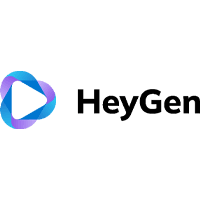
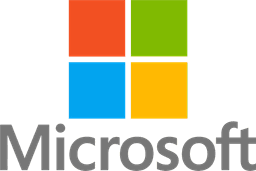
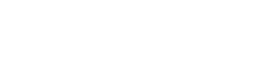
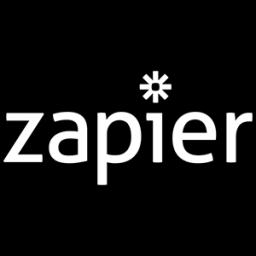
Furthermore, interdisciplinary collaboration is becoming increasingly important as the complexity of understanding negation deepens. Researchers across fields such as linguistics, computer science, and medicine are working together to develop training protocols that bring AI closer to human-like comprehensibility. This cooperative approach extends to creating more advanced datasets that reflect real-world language use, including the subtleties of negation. The aim is to not only enhance algorithms but also to bridge the gap between human communication and AI processing capabilities. Initiatives like these are crucial for reducing errors in high-stakes environments such as healthcare, where understanding nuanced language can significantly impact patient outcomes. Read more about these ongoing efforts at New Scientist.
Innovations in AI training techniques, such as data augmentation and recaptioning, are now being explored as potential solutions to the negation issue. By diversifying the types of data that AI systems process, researchers hope to create models that are more resilient to language complexities and can better navigate negational semantics. This approach, combined with developing verification steps that validate AI's interpretations, represents a comprehensive strategy to mitigate the negation blind spot in medical applications. Such initiatives not only illustrate the commitment to improving AI reliability but also highlight the importance of maintaining patient safety in all AI-driven clinical interventions. Detailed insights into these advancements can be found in the New Scientist article.
Implications for AI Governance and Regulation
AI's struggle to understand negation is not just a technical hurdle but poses significant implications for AI governance and regulation. As AI systems are increasingly integrated into sensitive fields like healthcare, the inability to process negations accurately could have dire consequences. Misdiagnosis due to AI misinterpreting negative medical information could result in inappropriate treatments and severe patient outcomes. This highlights the urgent need for robust regulatory frameworks that enforce stringent testing and validation of AI systems before their deployment in clinical settings. Approaches must include comprehensive evaluation criteria that account for language processing challenges, ensuring AI's reliability and safety.
The governance of AI in healthcare must address not only technical challenges but also ethical considerations. The difficulty AI systems face with negation can amplify biases present in training datasets, potentially affecting marginalized communities disproportionately. Regulation must therefore ensure that these systems are developed and deployed with fairness and equity in mind, incorporating diverse data samples and continuous monitoring for bias and performance issues. Strong governance policies are vital for fostering public trust and ensuring AI technologies do not inadvertently widen existing health disparities (as discussed [here](https://pmc.ncbi.nlm.nih.gov/articles/PMC10764412/)).
Stringent and adaptive regulation is crucial for mitigating the risks associated with AI's challenges in processing negation. Policymakers and industry leaders must work collaboratively to develop standards and protocols that address these limitations without stifling innovation. Through ongoing dialogue and collaboration with experts from different fields including AI, healthcare, and ethics, a balanced approach can be achieved. This holistic regulatory strategy must be agile enough to accommodate new insights and technologies as they emerge, adapting to address the dynamic landscape of AI applications in medicine and beyond.
AI governance must also take into account the broader societal implications of AI technologies struggling with negation. This includes potential impacts on other industries such as automotive and manufacturing, where misunderstanding negated commands could lead to significant safety risks or costly errors. Regulatory frameworks must therefore be interdisciplinary, recognizing the interconnected nature of AI applications across sectors. By adopting comprehensive risk assessment and management strategies, regulations can more effectively oversee AI's safe integration into various critical domains.
Learn to use AI like a Pro
Get the latest AI workflows to boost your productivity and business performance, delivered weekly by expert consultants. Enjoy step-by-step guides, weekly Q&A sessions, and full access to our AI workflow archive.
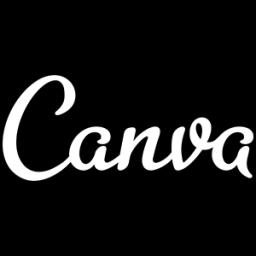
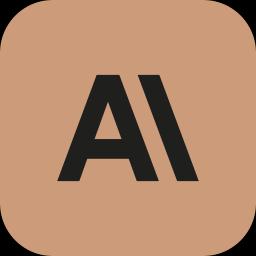
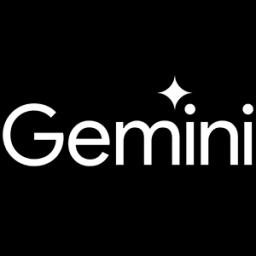
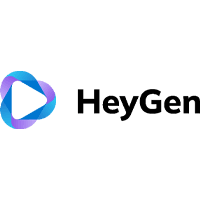
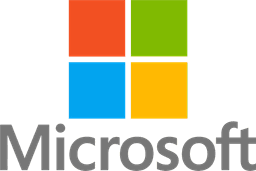
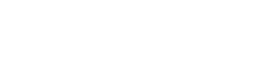
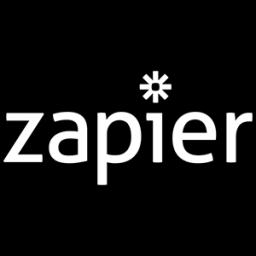
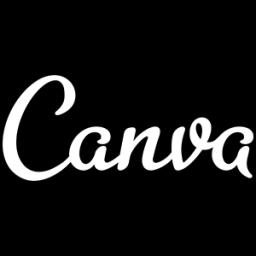
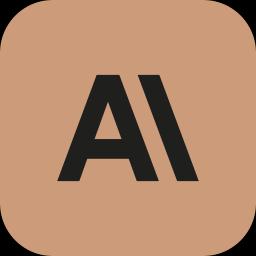
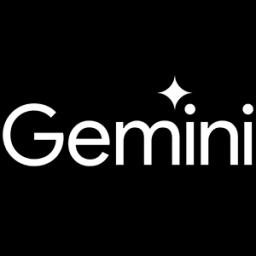
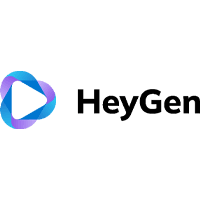
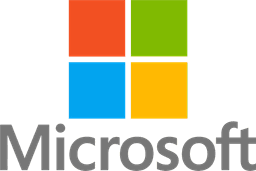
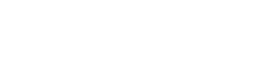
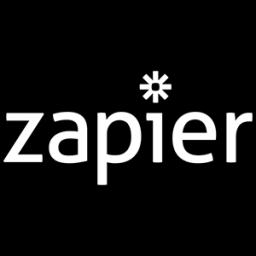
The growing awareness of AI's limitations regarding negation urges a re-examination of current regulatory models. This includes revisiting guidelines on data quality, model transparency, and accountability mechanisms to ensure AI systems are not only accurate but also interpretable and trustable by end-users. As researchers continue to explore solutions, such as improved algorithms and training techniques, regulators must ensure that these advancements are incorporated into actionable policies that safeguard public welfare while encouraging technological progress.
Broader Impact of AI's Negation Problem
The challenge of AI's inability to process negation extends far beyond the confines of medical settings. This deficiency can significantly impede the effectiveness of AI applications across various domains, highlighting the broader impact of this issue. In high-stakes fields such as finance and legal jurisdictions, where nuanced understanding of negative statements may influence critical decisions, the consequences of misinterpretation could be severe and far-reaching [1](https://www.newscientist.com/article/2480579-ai-doesnt-know-no-and-thats-a-huge-problem-for-medical-bots/). A financial AI might fail to correctly interpret a statement like 'No pending debts,' leading to erroneous credit assessments, while a legal AI could misjudge the presence of a 'not guilty' statement, potentially influencing case outcomes.
Beyond direct economic impacts, the inability of AI systems to handle negation effectively could result in trust erosion among users and clients. As these systems are increasingly deployed in customer service or contractual agreement scenarios, reliable negation comprehension becomes essential [3](https://radiologybusiness.com/topics/artificial-intelligence/insufficient-governance-ai-no-2-patient-safety-threat-2025). Any failure in this respect could not only lead to malpractice but also fuel skepticism about AI reliability, thereby affecting the overall market and slowing technological adoption.
The socio-cultural implications are equally significant, as AI systems misinterpreting negated phrases could inadvertently perpetuate biases. Instances where the AI misjudges medical assessments due to negation issues could exacerbate existing health inequalities, particularly affecting marginalized communities that already face healthcare access challenges [2](https://pmc.ncbi.nlm.nih.gov/articles/PMC11880872/). The repeated occurrence of such errors could undermine initiatives aimed at reducing these disparities, forcing policymakers to reassess AI's role in bridging the gap.
Moreover, the defense and security sectors face notable challenges, as affirming or negating statements could significantly impact strategic decisions. AI's struggle with understanding negation may lead to incorrect threat assessments or mishandling of intelligence, posing national security risks [4](https://karlobag.eu/en/science/fatal-ai-blind-spot-visual-language-models-dont-understand-no-study-finds-2z78k). This underlines the need for robust AI systems with heightened comprehension capabilities and the potential necessity for human oversight in critical analysis tasks to avoid compromising safety priorities.
Expert Perspectives on AI and Negation Bias
Artificial Intelligence (AI) has become a ubiquitous presence in various sectors, from healthcare to industrial applications. However, one of the persistent challenges AI faces is the understanding of negation. This problem becomes particularly evident in high-stakes fields like medicine, where the ability to comprehend negative statements can dramatically affect outcomes. For instance, medical AI systems that are unable to distinguish between 'no signs of disease' and 'signs of disease' can lead to serious misdiagnoses. The inability to process such critical nuances not only undermines the reliability of AI in medical diagnostics but also poses significant risks to patient safety. Recent insights from experts underline that this shortcoming is a major barrier to the effective implementation of AI technologies in healthcare, calling for more intelligent and nuanced algorithms that can better handle negation.
Learn to use AI like a Pro
Get the latest AI workflows to boost your productivity and business performance, delivered weekly by expert consultants. Enjoy step-by-step guides, weekly Q&A sessions, and full access to our AI workflow archive.
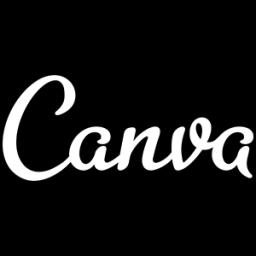
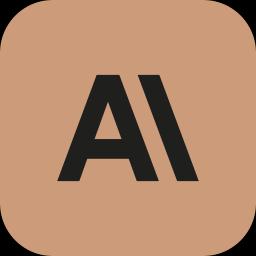
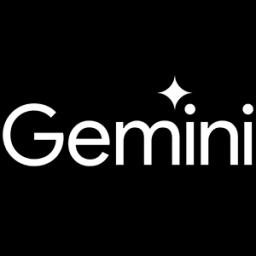
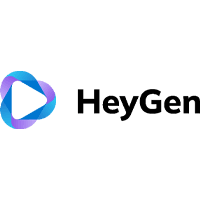
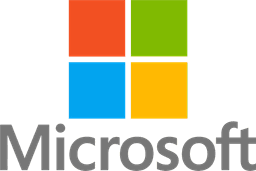
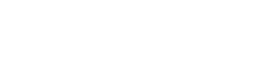
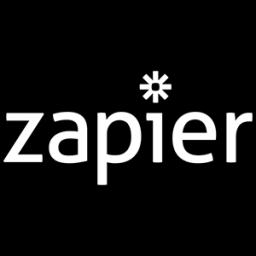
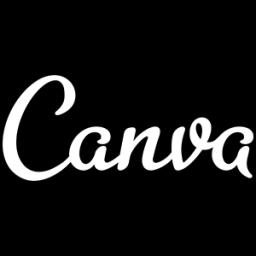
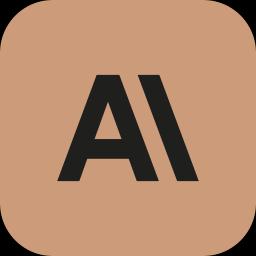
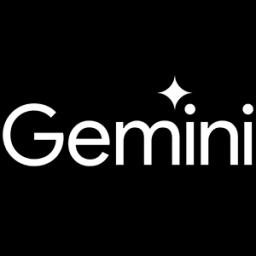
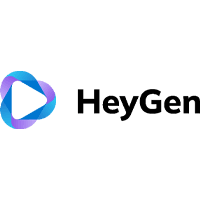
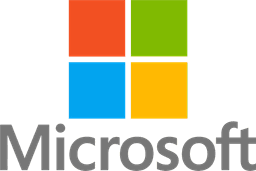
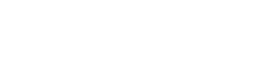
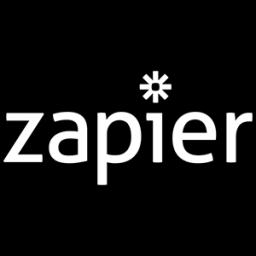
The issue with processing negation is not just a matter of technology but also a fundamental limitation in current AI training methods. Most AI models are developed by identifying patterns within massive datasets; however, these models often overlook linguistic nuances like negation, which requires understanding the context in which information is presented. This lack of comprehension in negation is particularly problematic in fields dealing with complex data, such as medical imaging. Industry experts suggest that a re-evaluation of the datasets used to train AI systems is necessary, emphasizing the inclusion of linguistic constructs that involve negation to ensure more accurate AI interpretations. The ongoing challenge is to enhance AI's semantic understanding to a level where it can make reliable judgments akin to human cognition.
To address AI's difficulties with negation, there is a growing focus on improving the algorithms that underpin these systems. Researchers are exploring new methodologies such as data augmentation techniques, which can enrich training sets specifically to include various examples of negation. Moreover, establishing clearer guidelines and robust testing protocols for AI systems can help verify their ability to handle negations before widespread deployment, particularly in sensitive environments like healthcare. Fostering interdisciplinary collaboration among linguists, computer scientists, and clinicians is crucial for developing AI models that align closer with human language understanding. These targeted efforts reflect an awareness of the problem and the potential solutions necessary to mitigate the risks associated with AI's misinterpretation of negation.
In light of AI's current limitations with negation, experts in the field argue for a shift towards systems that prioritize transparency and explainability. Ensuring that AI models can provide clear reasoning for their outputs will empower users, especially in the medical field, to identify and rectify errors that might arise from misinterpretations. The push for transparent AI systems is coupled with calls for heightened regulatory measures that can enforce accountability and ensure patient safety. As the technology evolves, aligning these systems with ethical standards and societal expectations becomes increasingly critical. By addressing these challenges, the integration of AI into clinical settings can be conducted with greater confidence and security.
Strategies for Mitigating AI Biases in Healthcare
Mitigating AI biases in healthcare, particularly in addressing the misunderstanding of negation, requires a comprehensive approach combining technology, policy, and education. A critical strategy involves the development of more sophisticated algorithms capable of better processing complex linguistic structures like negation. This demands rigorous research and development to create AI models that can discern subtle differences in medical language, such as distinguishing between 'no signs of pneumonia' and 'signs of pneumonia' [1](https://www.newscientist.com/article/2480579-ai-doesnt-know-no-and-thats-a-huge-problem-for-medical-bots/). Enhancing the training datasets with a diverse range of scenarios, including various syntactic structures and demographic data, is essential. This could involve the use of natural language processing techniques specifically geared towards understanding negative constructs to prevent misinterpretations in critical medical applications [1](https://www.newscientist.com/article/2480579-ai-doesnt-know-no-and-thats-a-huge-problem-for-medical-bots/).
Another important strategy is to implement robust validation frameworks that ensure AI systems in healthcare undergo thorough testing before deployment. This process should include simulations of real-world scenarios with a focus on high-risk applications where the accurate understanding of medical instructions and diagnoses is crucial [2](https://pmc.ncbi.nlm.nih.gov/articles/PMC11880872/). Additionally, adopting verification steps and post-deployment audits could help in early identification and correction of biases in AI behavior, thereby enhancing reliability and trust [2](https://pmc.ncbi.nlm.nih.gov/articles/PMC11880872/). Moreover, creating interdisciplinary teams including AI specialists, healthcare professionals, and ethicists can contribute to a more holistic view of AI development, ensuring technology serves the diverse needs of all patient groups while maintaining ethical standards [2](https://pmc.ncbi.nlm.nih.gov/articles/PMC11880872/).
To combat bias in AI effectively, healthcare institutions could also focus on fostering diverse data representation in AI model development. This means using datasets that reflect the broad spectrum of patient demographics to reduce instances of misdiagnosis linked to underrepresented groups [2](https://pmc.ncbi.nlm.nih.gov/articles/PMC11880872/). The inclusion of varied linguistic and cultural contexts in the datasets helps AI systems become more adaptable and accurate in diverse healthcare environments.
Learn to use AI like a Pro
Get the latest AI workflows to boost your productivity and business performance, delivered weekly by expert consultants. Enjoy step-by-step guides, weekly Q&A sessions, and full access to our AI workflow archive.
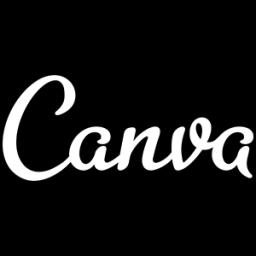
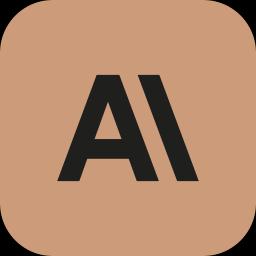
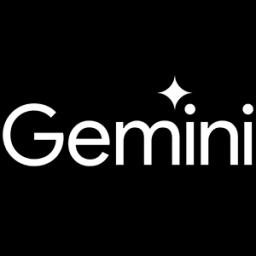
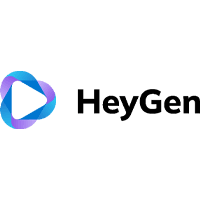
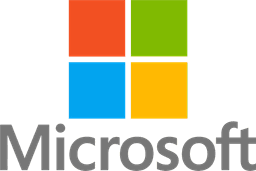
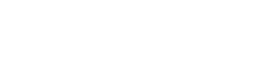
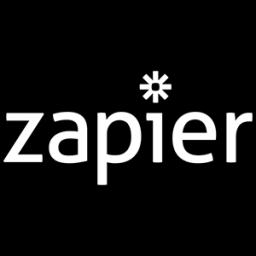
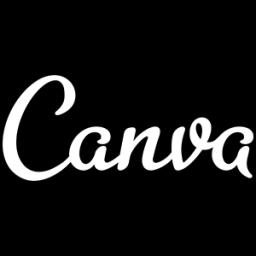
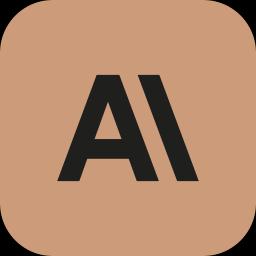
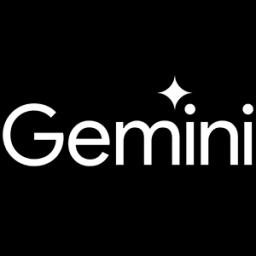
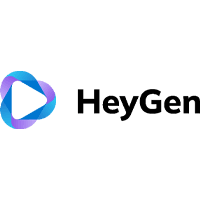
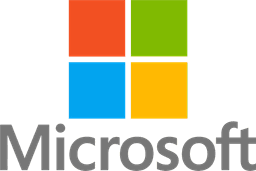
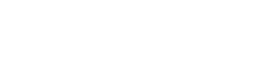
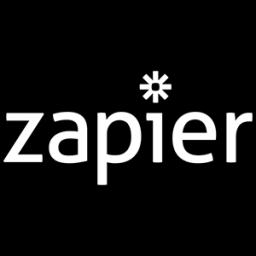
In parallel, education plays a critical role in mitigating biases by training healthcare professionals to understand the limitations and potential pitfalls of AI systems. By increasing awareness of the biases present in AI and the technological complexities involved, clinicians can make informed decisions and utilize AI as an assistive tool rather than a definitive solution [2](https://pmc.ncbi.nlm.nih.gov/articles/PMC11880872/). This perspective encourages medical practitioners to maintain a balanced approach when interpreting AI-generated insights, relying on their expertise while leveraging AI capabilities.
Finally, strong regulatory frameworks are essential to govern the ethical use of AI in healthcare. Policies that mandate transparency in AI decision-making processes and require regular updates to reflect new medical insights and technological advancements are crucial [3](https://pmc.ncbi.nlm.nih.gov/articles/PMC7973477/). Regulators must ensure that AI systems are not only technically robust but also socially responsible, minimizing potential harms and promoting equitable healthcare access [3](https://pmc.ncbi.nlm.nih.gov/articles/PMC7973477/).
Public Trust and AI in Medicine
The integration of artificial intelligence (AI) in medicine is a promising frontier, yet it also brings significant challenges, especially concerning public trust. The reliance on AI for medical diagnoses, image interpretation, and other critical applications means that any potential flaw in these systems can have serious repercussions. One of the central issues highlighted in recent discussions is AI's difficulty with understanding negation. This seemingly innocuous weakness can translate into critical errors in medical settings, where a simple misunderstanding can lead to misdiagnoses and inappropriate treatment plans. For instance, as highlighted in a recent article from New Scientist, AI struggles to distinguish between statements like 'no signs of pneumonia' and 'signs of pneumonia,' leading to potentially dangerous misinterpretations [1](https://www.newscientist.com/article/2480579-ai-doesnt-know-no-and-thats-a-huge-problem-for-medical-bots/). Such errors could diminish public confidence in AI technologies in healthcare, threatening to slow down adoption and innovation in this crucial field.
These difficulties with negation cross the boundary from mere technical challenges to issues of public trust and safety. The medical community is increasingly concerned about the implications of AI systems making undetected errors, especially when these systems are involved in life-and-death decisions. The potential for AI to misinterpret medical data raises not only immediate safety concerns but also long-term trust issues. Public trust in medical AI is vital for its success; without it, advancements could stall, and the benefits these systems offer could be significantly delayed. As raised in Radiology Business, without sufficient governance and a robust framework for validating AI systems, they pose a significant patient safety threat [3](https://radiologybusiness.com/topics/artificial-intelligence/insufficient-governance-ai-no-2-patient-safety-threat-2025). This situation underscores the need for enhanced regulatory measures to ensure that AI can be safely integrated into healthcare systems without compromising patient trust or safety.
Efforts to bolster public trust in AI-based medical systems are underway, with researchers advocating for more robust and transparent systems. As experts in the field suggest, improving algorithms to better handle negation and ensuring these are rigorously tested within diverse datasets are crucial steps [1](https://www.newscientist.com/article/2480579-ai-doesnt-know-no-and-thats-a-huge-problem-for-medical-bots/). Additionally, enhancing system transparency can help clinicians understand AI decisions, potentially catching errors that might currently go unnoticed. This is part of a broader movement that includes developing ethical guidelines and regulatory frameworks to guide the safe deployment of AI in healthcare. By addressing these challenges head-on, it is possible not only to enhance the reliability of medical AI but also to strengthen public trust in these increasingly integral technologies.
Future Directions and Challenges in AI Research
The field of AI research is at a critical juncture, with several exciting paths for future exploration as well as formidable challenges that need addressing. One of the foremost challenges is the issue of negation understanding in AI models, especially within medical contexts. These models have historically struggled to process negative statements, leading to significant misinterpretations in crucial areas like medical diagnosis. The inability to discern between phrases such as "signs of pneumonia" and "no signs of pneumonia" can have severe consequences on patient safety and healthcare outcomes. Such failings underscore the necessity for developing more sophisticated algorithms that can comprehend these subtle linguistic distinctions. As pointed out in a New Scientist article, this misunderstanding is a major obstacle in advancing medical bots into more reliable diagnostic tools (source).
Learn to use AI like a Pro
Get the latest AI workflows to boost your productivity and business performance, delivered weekly by expert consultants. Enjoy step-by-step guides, weekly Q&A sessions, and full access to our AI workflow archive.
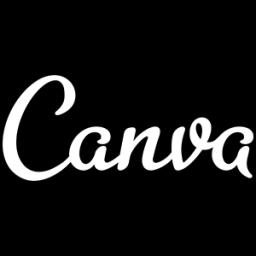
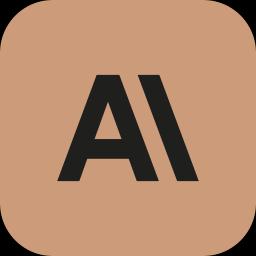
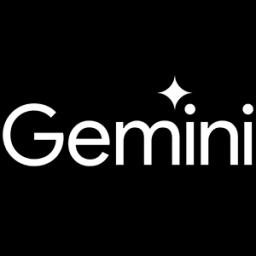
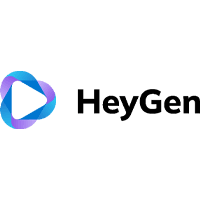
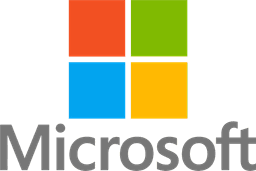
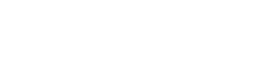
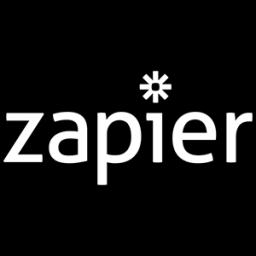
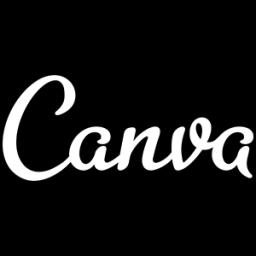
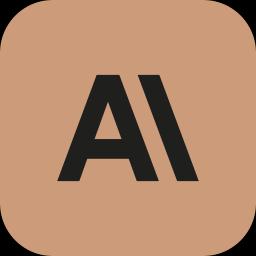
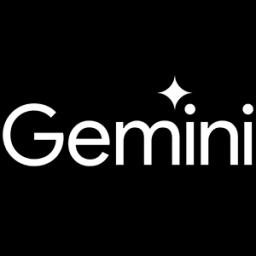
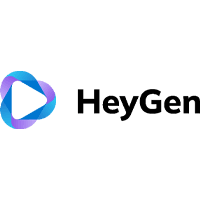
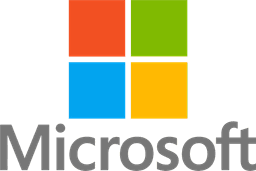
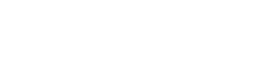
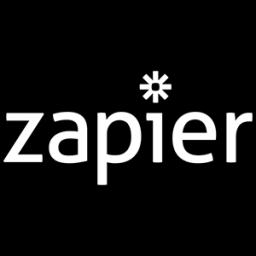
Further, the reinforcement of biases through AI systems remains a pressing concern in AI research. Models trained on skewed datasets can perpetuate or amplify existing inequalities, particularly in healthcare. This is particularly troubling given the diverse populations AI is being applied to, and the potential for bias to lead to misdiagnosis or inappropriate treatment across different demographic groups. To combat this, researchers are advocating for diverse development teams and robust bias detection methods, aiming to create more equitable systems. MIT researchers have highlighted the critical nature of this "blind spot," emphasizing the need for comprehensive datasets that incorporate negation and other challenging aspects to avert harmful outcomes (source).
Another critical area facing both opportunity and challenge is the development of governance and regulatory frameworks for AI, particularly in high-stakes applications like healthcare. Currently, the landscape is marred by a lack of sufficient regulatory oversight, which heightens risks related to patient safety. There's an urgent need for governance structures that can ensure AI systems are both ethical and effective. Existing reports underline the dire need for strong ethical guidelines and regulatory frameworks to reduce the risks posed by inaccuracies in AI systems (source). Establishing such frameworks is vital to safeguard against potential mishaps and to bolster trust in AI technologies.
Finally, the economic implications of the current limitations in AI cannot be ignored. Misinterpretations caused by inadequacies in negation understanding have ramifications that extend beyond healthcare, affecting fields like manufacturing, where AI models are used for quality control. Failures here could lead to unnoticed defects, costly recalls, and potential safety hazards, highlighting the need for meticulous development and validation processes. This cross-industry challenge demands comprehensive solutions, from advanced algorithm designs to enhanced training protocols, emphasizing the economic burden of AI inaccuracies (source).