AI Innovation
Alibaba's New LLM QwQ-32B Shakes Up AI Landscape: A Small But Mighty Contender
Last updated:

Edited By
Mackenzie Ferguson
AI Tools Researcher & Implementation Consultant
Alibaba has just launched QwQ-32B, a compact yet powerful language model poised to rival industry giants such as DeepSeek-R1 and OpenAI's latest offerings. This new model, boasting only 32 billion parameters, achieves comparable performance by leveraging reinforcement learning and other optimization techniques, marking a significant shift towards more efficient AI solutions. Openly available under the Apache 2.0 license, QwQ-32B sets the stage for broader accessibility and collaboration in the AI field. However, ethical questions around potential biases and regulatory alignment remain points of concern.
Introduction to Alibaba's QwQ-32B
The introduction of Alibaba's QwQ-32B marks a significant milestone in the realm of artificial intelligence, particularly in the development of large language models (LLMs). As outlined in the background information, QwQ-32B stands out due to its compact size, boasting 32 billion parameters compared to its larger counterparts like DeepSeek-R1 and OpenAI's o1. Despite its smaller scale, Alibaba claims that QwQ-32B delivers comparable performance. This remarkable achievement leverages a blend of advanced techniques, including reinforcement learning and training with a general reward model, which significantly enhances its reasoning capabilities.
One of the defining features of QwQ-32B is its open-weight availability under the Apache 2.0 license, which encourages wide accessibility and collaboration within the AI community. This open-source nature not only fosters innovation but also invites scrutiny, which is essential to address any concerns regarding bias and data governance. The model is positioned as a versatile tool for various applications, from industrial to academic settings, making it a crucial asset for developers and businesses seeking to harness AI's potential without the corresponding computational costs typically associated with larger models.
Learn to use AI like a Pro
Get the latest AI workflows to boost your productivity and business performance, delivered weekly by expert consultants. Enjoy step-by-step guides, weekly Q&A sessions, and full access to our AI workflow archive.
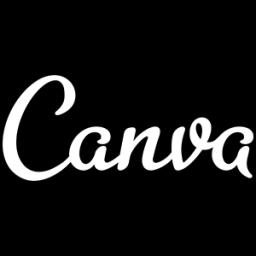
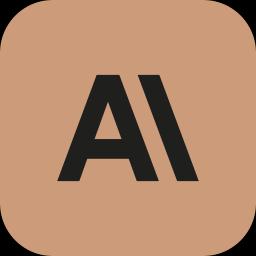
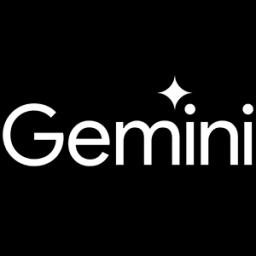
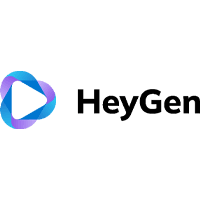
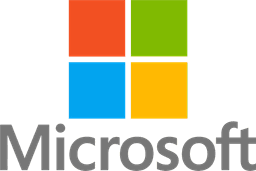
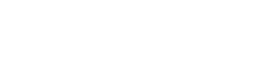
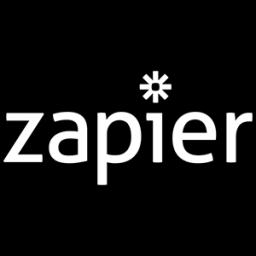
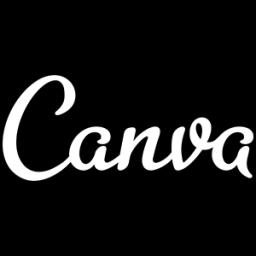
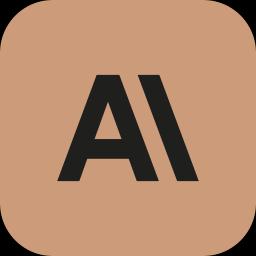
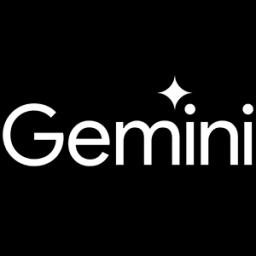
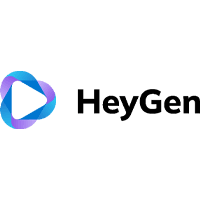
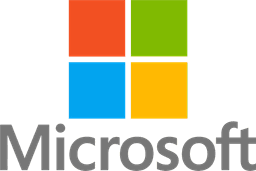
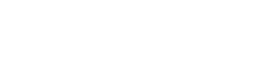
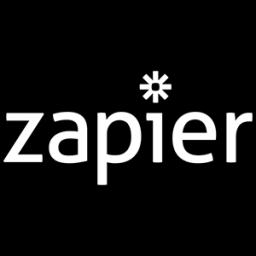
In terms of impact, QwQ-32B is poised to influence the competitive landscape of LLMs profoundly. It challenges the prevailing notion that bigger is better, demonstrating that efficiency in design and training can yield high performance without the need for extensive computational resources. This shift is potentially transformative, opening up new possibilities for smaller enterprises and researchers by reducing barriers to entry in AI development. As detailed in the source, the model's development aligns with a broader trend towards creating more resource-conscious AI solutions.
The Technological Edge: How Reinforcement Learning Powers QwQ-32B
Reinforcement learning has been a pivotal element in the design and success of Alibaba's QwQ-32B, a sophisticated model in the increasingly competitive landscape of large language models (LLMs). Reinforcement learning (RL), a form of machine learning in which agents learn optimal actions through trial and error by interacting with their environment, plays a critical role in enabling QwQ-32B to deliver highly efficient performance with its relatively compact structure of 32 billion parameters. The application of RL allows this model to execute complex reasoning tasks with efficiency typically associated with models of greater magnitude, like DeepSeek-R1 and OpenAI's o1. By leveraging RL's capacity to iteratively improve through feedback mechanisms, QwQ-32B excels in decision-making processes, thereby achieving performance metrics that impressively rival its more parameter-heavy counterparts. This strategic application of RL underscores Alibaba's commitment to pushing technological boundaries while optimizing computational resources.
Alibaba's innovative use of reinforcement learning in powering QwQ-32B showcases a paradigm shift from the traditional belief that model size directly correlates with performance. This shift highlights how strategic algorithmic enhancements can compensate for relying solely on sheer scale. Reinforcement learning enables QwQ-32B to conduct intricate logical operations and process vast arrays of information in a structured manner, thus broadening its utility across diverse applications from natural language processing to intricate problem-solving scenarios. The model's design draws on RL's fundamental principles, focusing on maximizing cumulative reward, which in QwQ-32B translates into refined outputs and enhanced predictive capabilities. This not only ensures resource-efficient execution but also broadens access to advanced AI technologies by reducing the cost barriers associated with high-performance computing, which traditionally restricted such capabilities to only well-funded entities.
Central to QwQ-32B's architecture is its incorporation of a general reward model bolstered by rule-based verifiers, which are integral to the reinforcement learning framework. By using these evaluative components, the model continuously calibrates its outputs to optimize accuracy and relevance, ensuring that each iteration of input processing leads to progressively enhanced decision paths. This approach allows the model to maintain a high level of precision and adapt to an array of contexts, making it highly versatile in its applications. Furthermore, the rule-based verifiers act as a safeguard, ensuring that the model's learning does not deviate into less desirable outcomes, thus maintaining alignment with intended ethical standards and practical objectives. This thoughtful integration exemplifies Alibaba's strategic intention to not only innovate but also address potential biases and ethical considerations in the use of AI.
Learn to use AI like a Pro
Get the latest AI workflows to boost your productivity and business performance, delivered weekly by expert consultants. Enjoy step-by-step guides, weekly Q&A sessions, and full access to our AI workflow archive.
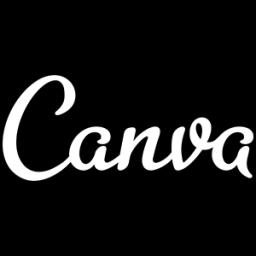
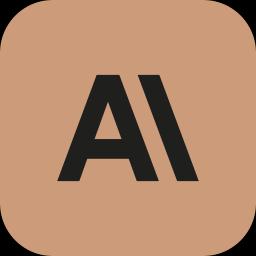
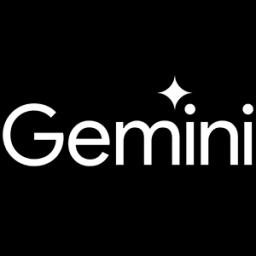
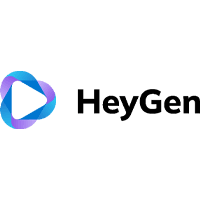
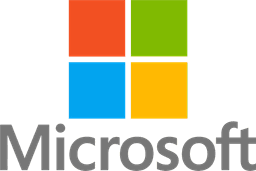
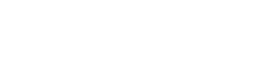
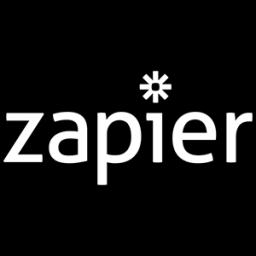
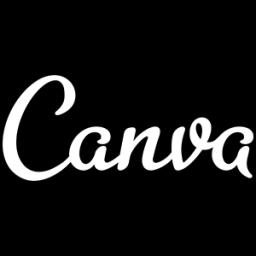
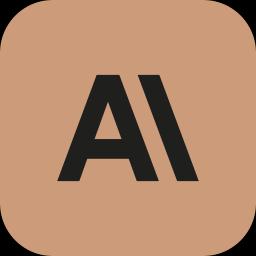
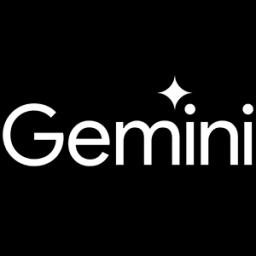
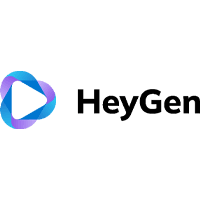
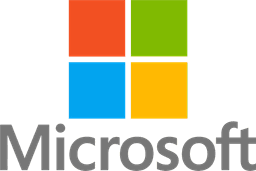
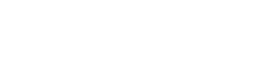
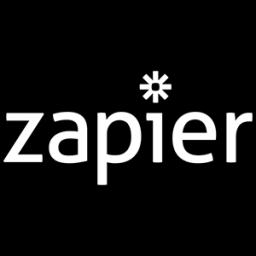
Availability and Licensing: Open Sourcing the Model
Alibaba's QwQ-32B model, an open-weight language model based on its Qwen2.5-32b large language model (LLM), is making waves with its strong performance despite its compact size. Open-sourcing the model plays a crucial role in Alibaba's strategy to foster innovation and collaboration within the AI community. Given its release under the Apache 2.0 license, QwQ-32B encourages developers to explore its code and contribute to enhancing its capabilities. This licensing model allows users to freely use, modify, and distribute the software, making it accessible to a wide range of developers and researchers who can implement their own modifications based on specific needs and challenges. As part of the open-source movement, QwQ-32B stands to democratize access to powerful AI tools, enabling smaller companies and academic institutions to leverage cutting-edge technology without incurring substantial costs associated with proprietary models.
The decision to open-source the QwQ-32B model under the Apache 2.0 license is a strategic move by Alibaba to assert its presence in the global LLM landscape. In doing so, Alibaba not only promotes transparency and collaboration but also positions itself as a forward-thinking leader in AI development. The model's open-source nature allows for continuous improvements driven by a global community of experts and enthusiasts who can address potential limitations and biases. By releasing QwQ-32B as open-source software, Alibaba challenges the traditional dominance of larger and often more costly models, thus leveling the playing field for emerging players in the AI space. The open access to QwQ-32B fosters an ecosystem where innovation is shared, and solutions to complex problems are developed collaboratively.
With its open-source launch, QwQ-32B provides a unique opportunity to explore efficient AI development. The model's compact size, combined with its competitive performance, may drive a shift in how developers approach AI architectures, emphasizing efficiency over sheer size. By sharing the model openly, Alibaba signals its commitment to inclusivity and advancement in AI technologies, encouraging a range of applications across different industries. This could stimulate new developments in areas like healthcare, where smaller, cost-effective models can be particularly valuable, or in education, where personalized learning approaches are increasingly in demand. Open sourcing QwQ-32B not only sparks innovation but also invites scrutiny and input that could address ethical concerns surrounding AI use, particularly in terms of privacy and bias, ensuring these technologies are used responsibly and effectively in the future.
Open-weight availability of models like QwQ-32B is crucial as it accelerates research and application in AI by removing barriers typically associated with proprietary models. By opting for an open-source approach, Alibaba reduces access restrictions, potentially expediting the pace of advancements in AI technology. This kind of accessibility encourages more robust data governance practices and ethical compliance, as a broader range of stakeholders can participate in its enhancement and application. Under the Apache 2.0 license, developers worldwide can utilize QwQ-32B without legal or financial constraints, which fosters an environment of inclusivity and collective growth. In doing so, Alibaba not only contributes to the technical evolution of AI but also sets precedence for how technology companies can lead with openness and trust as foundational principles.
The competitive advantage of open-sourcing QwQ-32B is multifaceted. It poses a challenge to the paradigm that larger models, requiring significant computational resources, are inherently superior. By offering comparable performance, QwQ-32B undercuts the computational demands typically associated with premium models like DeepSeek-R1 and OpenAI's o1-series, showcasing the potential for leaner, more resource-efficient models that do not compromise on capability. Such strides redefine competition in the AI market, compelling other companies to reevaluate their strategies in model development and deployment. Alibaba's strategic move towards open-source licensing provides it with a unique positioning in the market, promoting its ethos of collaboration, community-driven progress, and sustainable innovation. With QwQ-32B, Alibaba effectively addresses industry demand for accessible, high-caliber AI solutions while driving a narrative that prioritizes shared learning and resource-conscious development.
Ethical Implications in Chinese AI Initiatives
The rise of AI technology in China, spearheaded by initiatives like Alibaba's QwQ-32B model, presents significant ethical implications. As these advanced technologies become more integrated into global systems, the governance and ethical standards they adhere to come under scrutiny. China's approach to AI, while impressive in innovation, often incites debate around issues of transparency, bias, and data governance. These concerns are compounded by the Chinese government's stringent regulatory environment, which may not align with more globally accepted ethical standards.
Learn to use AI like a Pro
Get the latest AI workflows to boost your productivity and business performance, delivered weekly by expert consultants. Enjoy step-by-step guides, weekly Q&A sessions, and full access to our AI workflow archive.
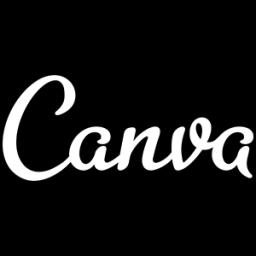
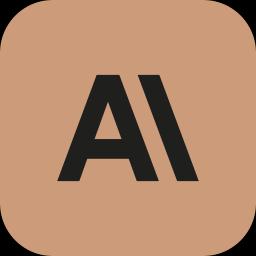
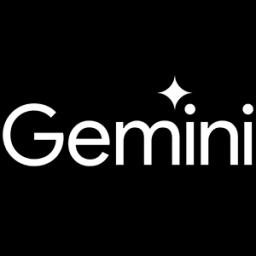
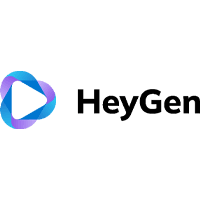
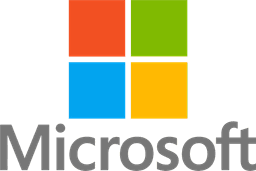
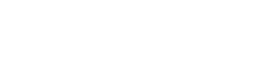
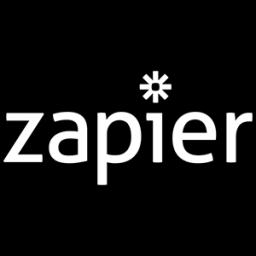
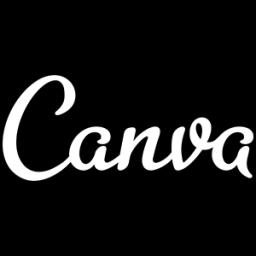
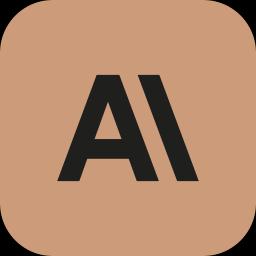
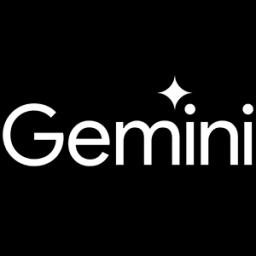
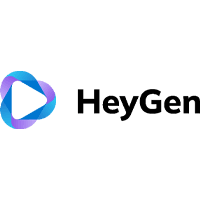
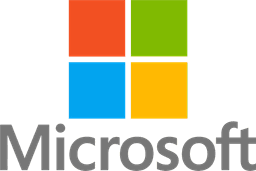
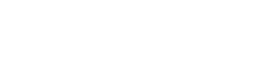
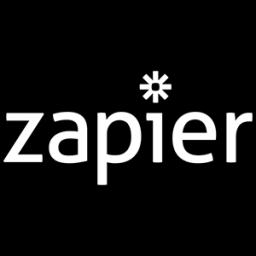
One prominent ethical concern is the lack of transparency in the development and deployment of Chinese AI models. While Alibaba's QwQ-32B is open-source, there are still significant worries about how these models are trained, particularly regarding the datasets used and the potential biases these datasets may introduce. The alignment of these models with state-imposed regulatory standards can also lead to outputs that reflect political biases, which is particularly concerning in a model that might be used globally. The potential for the model to propagate these biases into critical decision-making processes raises substantial questions about fairness and objectivity.
Another major ethical implication is related to data privacy and security. The use of large language models (LLMs) requires vast amounts of data, often including personal information. In a country where state surveillance is a significant concern, the deployment of AI technologies raises alarms about how data is collected, stored, and used. The ethical handling of data becomes even more critical when these technologies are used beyond their country of origin, potentially impacting global standards of data privacy.
Furthermore, the competitive nature of AI development could push companies to prioritize performance over ethics. With Alibaba's QwQ-32B challenging the status quo by outperforming larger models with fewer parameters, there is a risk that companies worldwide might overlook ethical practices in pursuit of competitive advantage. This pursuit can lead to a "race to the bottom" in ethics, especially if regulatory frameworks are weaker or less enforced. The responsibility, therefore, not only lies with developers but also with international bodies to ensure that AI innovation does not compromise ethical principles.
Lastly, the societal implications of biases inherent in AI models like QwQ-32B cannot be overlooked. When these models are used in sectors such as law enforcement or healthcare, unchecked biases can lead to serious consequences, such as discrimination or unequal treatment. Ensuring that AI systems are developed and implemented with strict ethical guidelines is vital for preventing harm and fostering trust in AI technologies. Global collaboration in establishing robust ethical standards and frameworks will be essential to navigate these challenges sustainably and equitably.
Comparative Analysis: Baidu vs Alibaba vs DeepSeek
In the landscape of artificial intelligence (AI), the competition among giants like Baidu, Alibaba, and DeepSeek is increasingly heating up. Alibaba has recently emerged as a formidable contender with the release of its QwQ-32B model, which has set a new benchmark in efficiency and performance ([source](https://www.infoworld.com/article/3840580/alibaba-says-its-new-ai-model-rivals-deepseekss-r-1-openais-o1.html)). This model, which boasts only 32 billion parameters, challenges the traditional reliance on sheer scale as the sole determinant of a model's prowess. By incorporating advanced reinforcement learning techniques and rule-based verification, Alibaba's QwQ-32B offers capabilities on par with much larger models like DeepSeek-R1, which contains 671 billion parameters, marking a shift towards more resource-efficient AI development ([source](https://venturebeat.com/ai/alibabas-new-open-source-model-qwq-32b-matches-deepseek-r1-with-way-smaller-compute-requirements/)).
Baidu, another key player in the AI domain, launched its Ernie model to much anticipation; however, it has not captured global attention to the same extent as Alibaba and DeepSeek's models have. Baidu's strategy appears more regionally focused, positioning its technology to cater primarily to domestic markets within China. Despite this, the Ernie model's integration within Baidu's ecosystem demonstrates its commitment to leveraging AI for broad-based consumer applications ([source](https://www.infoworld.com/article/3840580/alibaba-says-its-new-ai-model-rivals-deepseekss-r-1-openais-o1.html)). The contrasting scales of impact between these companies underline a strategic divergence in their approaches to AI globalization.
Learn to use AI like a Pro
Get the latest AI workflows to boost your productivity and business performance, delivered weekly by expert consultants. Enjoy step-by-step guides, weekly Q&A sessions, and full access to our AI workflow archive.
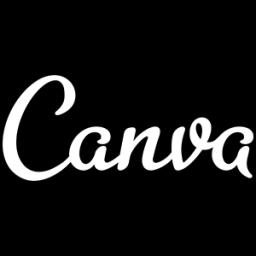
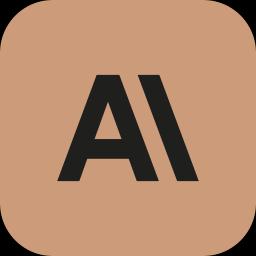
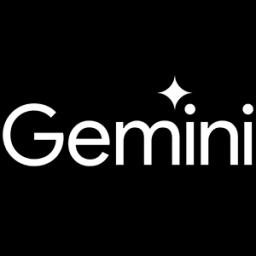
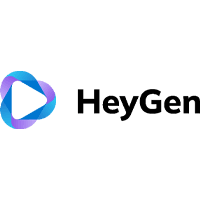
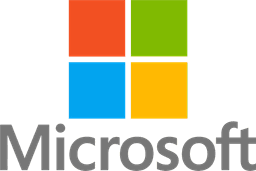
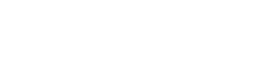
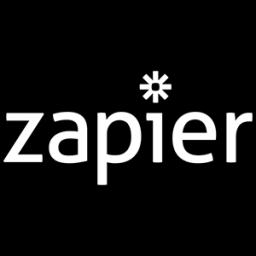
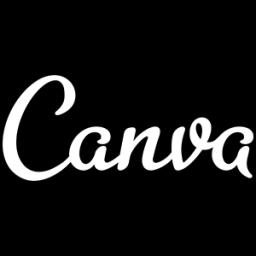
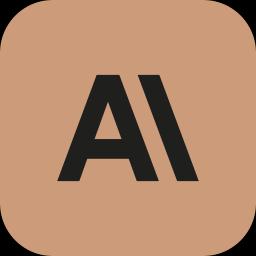
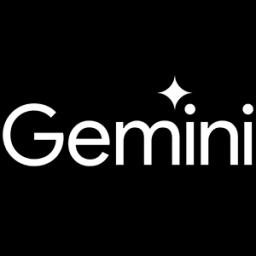
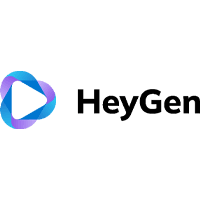
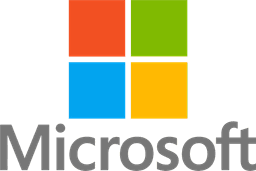
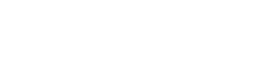
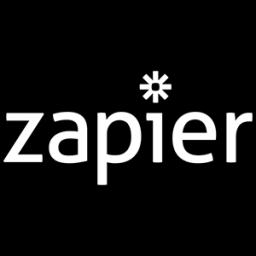
On the other hand, DeepSeek's R-1 model stands as a testament to the potential of expansive AI architectures. As part of this comparative analysis, DeepSeek has maintained its reputation by prioritizing high parameter counts to achieve intricate deep learning capabilities. The expansive model design ensures that DeepSeek can address complex, nuanced tasks across diverse domains. This approach, while more computationally intensive, highlights the traditional belief in AI development that "bigger is better" ([source](https://www.analyticsindiamag.com/ai-news-updates/alibabas-new-qwq-32b-model-is-as-good-as-deepseek-r1-outperforms-openais-o1-mini/)).
The introduction of Alibaba's QwQ-32B into this competitive landscape has significant implications for the future of large language models (LLMs). It has prompted a reevaluation of how parameters correlate with performance. This challenges current AI industry norms, which often equate larger models with enhanced capabilities ([source](https://opentools.ai/news/alibabas-qwq-32b-a-new-contender-in-the-ai-arena-sparking-a-stock-surge)). With QwQ-32B's success, there is a growing recognition that efficiency, coupled with smart design, can offset the advantages traditionally gained by models of more significant size, potentially heralding a new era in AI that favors nuanced innovation over brute computational force.
While the excitement around these developments is palpable, critical analysis must address potential ethical and practical challenges. Alibaba’s model, due to its alignment with Chinese regulatory standards, may carry implicit biases, a factor warranting attention by users and developers globally ([source](https://opentools.ai/news/alibabas-qwq-32b-a-new-contender-in-the-ai-arena-sparking-a-stock-surge)). These concerns highlight the importance of transparency and ethical guidelines in AI development, ensuring that AI tools serve broader societal interests without compromising on fairness and objectivity. As these companies continue to push the AI frontiers, balancing innovation with responsibility remains a core challenge.
Global Trends: Efficient Models and Proprietary LLMs
The global landscape of large language models (LLMs) is undergoing a transformative shift, driven by the increasing focus on efficiency and the rise of proprietary models. Alibaba's release of QwQ-32B, a compact reasoning model, is a testament to this trend. Despite having only 32 billion parameters, QwQ-32B is designed to rival much larger models like DeepSeek-R1 and OpenAI's o1. This compactness is achieved through reinforcement learning techniques and a robust reward model, marking a significant advancement in making high-performance AI more accessible and cost-efficient. The model's availability under the open-weight Apache 2.0 license further supports its widespread adoption, fostering a spirit of collaboration and innovation within the AI community. This development highlights a broader industry movement towards creating models that are not only powerful but also resource-efficient and flexible for diverse applications.
Alibaba's QwQ-32B illustrates the growing trend of companies investing in proprietary models to cement their place in the competitive AI landscape. While major players like Alibaba are pushing the boundaries with innovative approaches, smaller companies are motivated to develop their own LLMs to meet specific industry needs and maintain control over their data. This trend is observed across sectors, including law firms and tech startups, that increasingly seek to gain a competitive edge through customized AI solutions. Alibaba's strategic focus on efficiency not only challenges the dominant narrative that larger models offer unparalleled performance but also stimulates new research directions that prioritize scalable and economical AI development. Moreover, the contributions from other tech giants, including Tencent with its Hunyuan Turbo S model and Mistral's OCR API, underscore a collective shift towards specialization and innovative model architectures.
The release of QwQ-32B has also sparked significant discussion regarding the ethical implications of relying on Chinese-developed AI models. Concerns related to data governance and regulatory alignment have been raised, particularly in western markets where the ethical framework surrounding AI development remains a topic of considerable debate. Although QwQ-32B's potential biases due to its alignment with Chinese regulatory standards might pose risks, the open-source policy invites global scrutiny and collaboration, offering a platform for shared advancements in tackling these issues. As businesses and developers navigate the complexities of deploying such models, balancing innovation with ethical responsibility becomes crucial to ensuring fair and unbiased AI applications. This context emphasizes the importance of establishing international guidelines and frameworks that promote ethical use and development of AI systems.
Learn to use AI like a Pro
Get the latest AI workflows to boost your productivity and business performance, delivered weekly by expert consultants. Enjoy step-by-step guides, weekly Q&A sessions, and full access to our AI workflow archive.
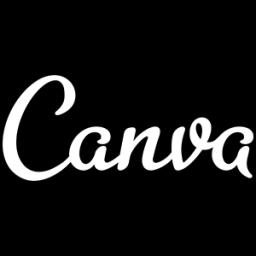
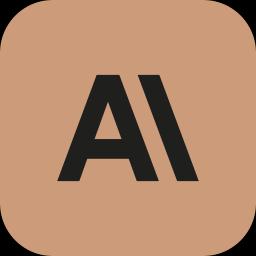
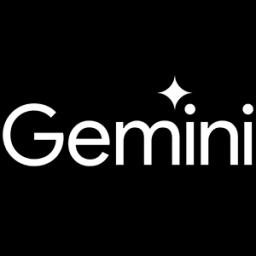
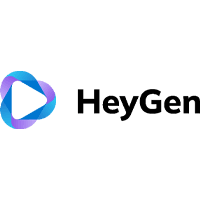
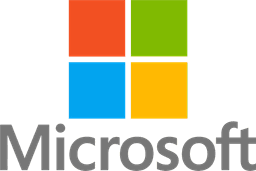
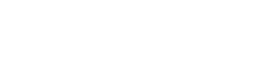
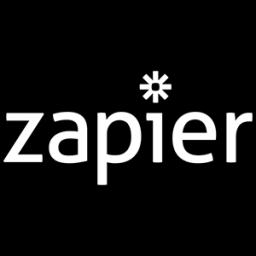
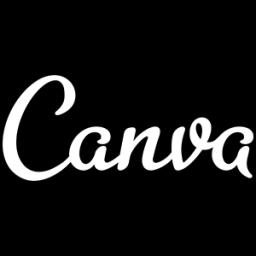
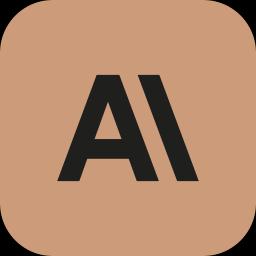
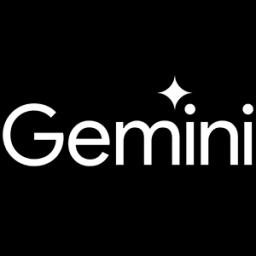
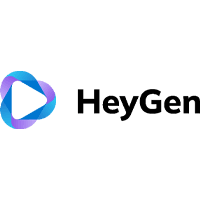
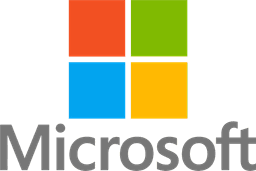
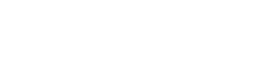
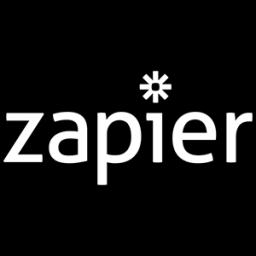
Public reaction to Alibaba's QwQ-32B has been notably varied, reflecting the model's dual role as a technological breakthrough and a point of contention regarding its origin and ethical considerations. Enthusiasts praise its performance efficiency, particularly given its comparatively low parameter count, and its open-source nature under the Apache 2.0 license has been met with approval, catalyzing innovation and broader community engagement. However, the model has also faced criticism over its challenges with common-sense reasoning and its alignment with Chinese regulations. These mixed reactions underscore the complex interplay between technological capabilities and societal acceptance, emphasizing the need for transparency and continued dialogue between developers, policymakers, and the public to align technological progress with ethical standards.
The future implications of innovations like QwQ-32B are profound, extending beyond technical achievements to influence economic and geopolitical landscapes. Its development signifies a potential shift in the global AI ecosystem, challenging the dominance of western tech companies and highlighting China's growing influence in the field. Economically, the model's efficiency could democratize AI by reducing costs and broadening access, empowering smaller entities to leverage cutting-edge technology. Politically, however, it raises questions about data sovereignty and international norms, necessitating careful consideration by governments and regulatory bodies to ensure that advancements in AI contribute positively to global technological and ethical standards. Addressing these challenges with a collaborative and ethical approach will be crucial in guiding the future course of AI development.
Expert Opinions: The Impact of QwQ-32B's Launch
Alibaba Cloud's recent launch of QwQ-32B has sparked varied expert opinions, underscoring its potential to reshape the AI landscape. With a mere 32 billion parameters, QwQ-32B is designed to efficiently rival models that boast significantly higher parameter counts, such as DeepSeek-R1 and OpenAI's o1 series. This has elicited admiration from industry analysts, who view it as a monumental step towards more resource-efficient artificial intelligence. According to a report by InfoWorld, Alibaba argues that QwQ-32B delivers comparable performance to these larger models by utilizing advanced reinforcement learning and comprehensive rule-based evaluation mechanisms, all within an open-source framework.
Experts in the field are particularly captivated by the reduced computational demands of QwQ-32B, emphasizing its potential to democratize access to sophisticated AI technologies. This could lead to substantial cost savings and foster innovation among smaller enterprises and researchers who previously found the costs prohibitive. Analyst discussions highlighted in InfoWorld also point to the open-access nature of QwQ-32B under the Apache 2.0 license as a strategic move that encourages widespread adoption and collaborative advancements within the artificial intelligence community.
Nevertheless, some experts approach Alibaba's claims with skepticism, questioning whether QwQ-32B can effectively navigate complex recursive reasoning and the ethical issues it might raise, especially in light of its alignment with Chinese regulatory norms. As highlighted by a critical analysis, the potential for bias, particularly in politically sensitive issues, poses significant challenges. This skepticism reflects broader concerns about the balance between technological innovation and ethical responsibility in the burgeoning field of AI.
The introduction of QwQ-32B into the competitive arena also signals a shift in prevailing perceptions about model size and capability. By challenging the notion that bigger is always better, Alibaba's compact model could set a new benchmark for efficiency and innovation in AI development. Experts recognize this potential as a catalyst for a paradigm shift, inspiring new architectures and methodologies across the AI landscape. The model's open-access framework further invites the global research community to explore new possibilities, driving forward an era of collaborative technological advancement.
Learn to use AI like a Pro
Get the latest AI workflows to boost your productivity and business performance, delivered weekly by expert consultants. Enjoy step-by-step guides, weekly Q&A sessions, and full access to our AI workflow archive.
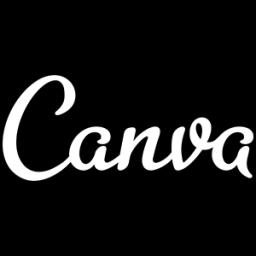
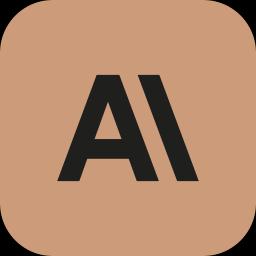
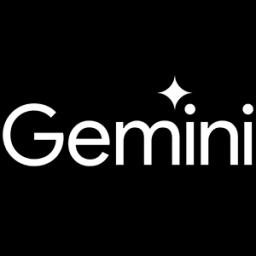
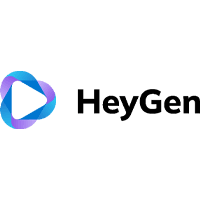
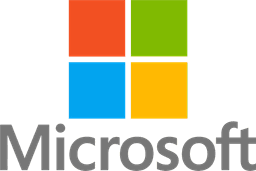
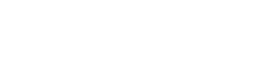
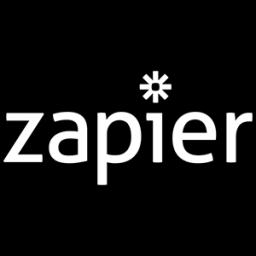
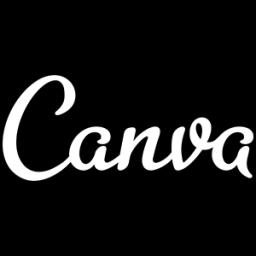
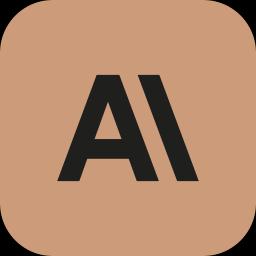
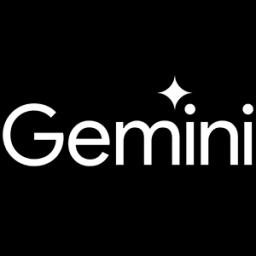
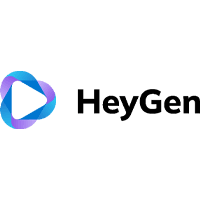
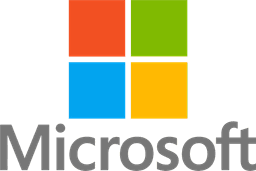
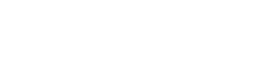
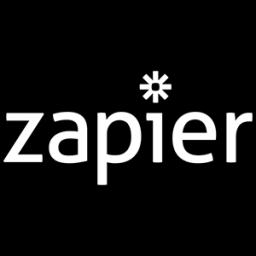
Public Reception and Initial Reactions
The public reception to Alibaba's QwQ-32B model has been a mix of enthusiasm and scrutiny, as discussions around its compact and efficient design capture the attention of the tech industry. Since its introduction, QwQ-32B has been lauded for its competitive performance in AI modeling despite its smaller size, drawing comparisons to giants like DeepSeek-R1 and OpenAI’s o1 models. Enthusiasts and AI developers have particularly appreciated its reasoning capabilities, which manage to rival larger models with significantly fewer resources, thus promising to democratize access to advanced AI technologies like never before [source].
However, the model's introduction has not been without its concerns. Skeptics have pointed out potential issues related to bias and alignment with Chinese regulatory standards. There are discussions around the model's propensity to be less effective in common-sense reasoning tasks and the lack of transparency in certain components, which some attribute to its regulatory and developmental framework [source]. These apprehensions hint at the broader conversations in the AI community regarding ethical standards and governance, especially with open-source models that carry significant influence on global AI development.
Future Implications and Economic Impact
The release of Alibaba's QwQ-32B model marks a significant shift in the landscape of large language models (LLMs). By delivering competitive performance with a substantially smaller parameter count compared to models like DeepSeek-R1 and OpenAI's o1, QwQ-32B highlights a move toward more efficient artificial intelligence solutions. This efficiency is pivotal in reducing the costs associated with deploying LLMs, thus enabling smaller businesses and emerging markets to leverage high-performance AI without prohibitive infrastructure investments. Such democratization of technology could spur innovation across diverse sectors, prompting economic growth and an evolution in industrial paradigms. The economic implications of this development are vast, as access to advanced AI capability can redefine competitiveness and operational methodologies across industries such as finance, healthcare, and education. [More information](https://www.infoworld.com/article/3840580/alibaba-says-its-new-ai-model-rivals-deepseekss-r-1-openais-o1.html).
Socially, the QwQ-32B model's open accessibility under the Apache 2.0 license heralds a new era of collaborative AI advancement. Its potential applications in enhancing educational tools and medical diagnostics demonstrate its capacity to deliver personalized solutions and improved service delivery across public sectors. However, the emphasis on addressing ethical concerns related to inherent biases is critical. Given the model's development within a Chinese regulatory framework, ensuring objectivity and fairness in its applications is crucial to avoid unintended biases or discriminatory practices. Therefore, strategies to incorporate transparent and balanced decision-making algorithms are necessary to support its ethical deployment across global platforms. [Explore further](https://www.dlapiper.com/en-us/insights/publications/2024/09/china-releases-ai-safety-governance-framework).
Politically, QwQ-32B's emergence challenges the hegemony of Silicon Valley giants by illustrating the capability of Chinese firms to produce competitive AI technologies. This development could recalibrate the geopolitical landscape, influencing AI governance and international collaborations. As these models become instrumental in shaping political and economic policies globally, understanding and integrating international data governance standards will be imperative. Training such models to align ethically while promoting transparency can help mitigate potential geopolitical tensions and encourage constructive international dialogues on AI policies. The model's open-source nature serves as both an opportunity for global integration and a point of scrutiny regarding oversight and ethical standards, essential for sustaining balanced global AI progress. [Read more](https://venturebeat.com/ai/alibabas-new-open-source-model-qwq-32b-matches-deepseek-r1-with-way-smaller-compute-requirements/).
The impact of efficient models like QwQ-32B on the competitive landscape of AI highlights the ongoing transition towards models that optimize resources without sacrificing performance. This shift encourages new research directions in AI, focusing on refining reinforcement learning techniques and leveraging compact architectures to achieve high performance. By challenging the assumption that larger parameter counts equate to superior AI, Alibaba's model motivates a reexamination of AI developmental methodologies. This advancement not only positions Alibaba as a formidable challenger in the AI race but also fosters an environment for innovative breakthroughs in how artificial intelligence is constructed and operationalized globally. The broader implications of these developments necessitate careful navigation of its potential ethical and regulatory challenges to fully harness the technologies' capabilities for societal benefit. [Discover more](https://opentools.ai/news/alibabas-qwq-32b-a-new-contender-in-the-ai-arena-sparking-a-stock-surge).
Learn to use AI like a Pro
Get the latest AI workflows to boost your productivity and business performance, delivered weekly by expert consultants. Enjoy step-by-step guides, weekly Q&A sessions, and full access to our AI workflow archive.
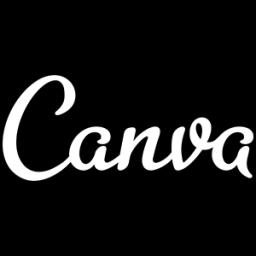
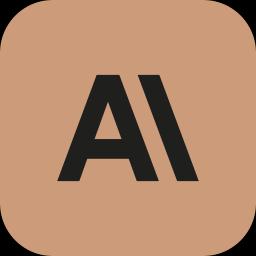
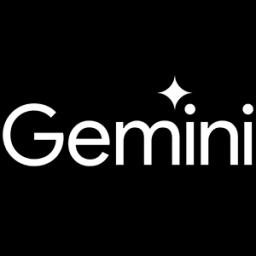
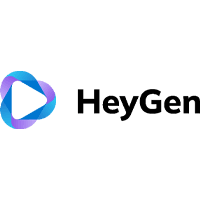
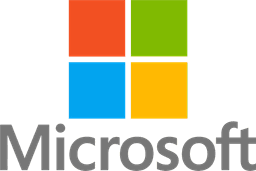
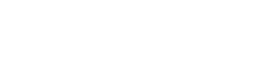
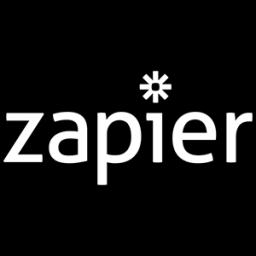
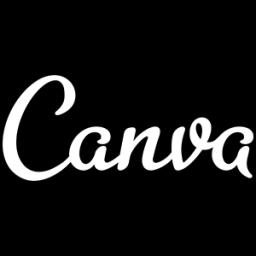
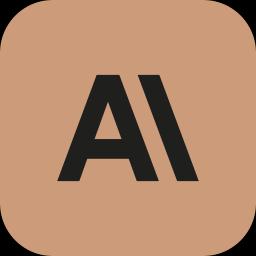
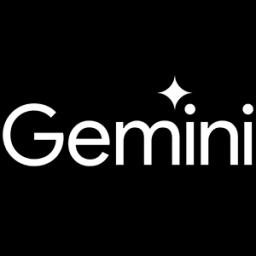
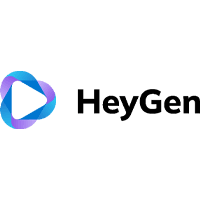
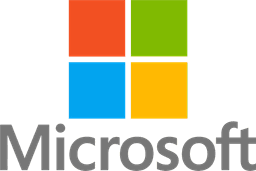
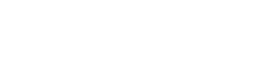
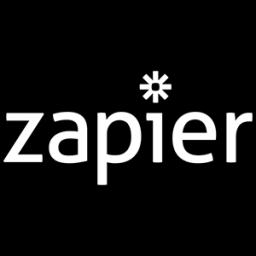
In conclusion, QwQ-32B stands as a testament to the rapid evolution and ongoing reshaping of AI technologies. Its implementation promotes a forward-thinking approach that balances efficiency with performance, creating numerous pathways for industry-wide applications. However, to capitalize on its advantages, comprehensive strategies addressing biases, data governance, and international cooperation must be prioritized. As the international community grapples with these challenges, Alibaba's QwQ-32B could become a pivotal force in crafting the future narrative of global AI deployment and integration, provided its strategies align with sustainable and equitable technological development. [Learn more](https://www.law.com/legaltechnews/2025/03/06/firms-are-building-their-own-llms-to-stay-ahead-of-innovation-competition-/).
Social and Political Dimensions of AI Advancements
The rapid advancement of AI technology has paved the way for significant transformations in both social and political spheres. Breakthroughs like Alibaba's QwQ-32B model highlight not just technological progress but also raise critical questions about global power dynamics and ethical responsibilities. The model's capacity to perform with fewer parameters challenges the traditional belief that bigger is always better, positioning Alibaba as a formidable contender in the AI landscape, traditionally dominated by Western companies like OpenAI. This shift in technological prowess exemplifies the changing geopolitical narratives and the rise of China as a tech leader, underscoring the need for international dialogue on AI standards and regulations [InfoWorld](https://www.infoworld.com/article/3840580/alibaba-says-its-new-ai-model-rivals-deepseekss-r-1-openais-o1.html).
As AI models like QwQ-32B become more prevalent, their societal impacts must be carefully scrutinized. Tools that enhance efficiency and accessibility in AI can democratize technology, making advanced capabilities available to more diverse groups, including smaller enterprises and research organizations. However, this democratization comes with a caveat; the potential for embedding biases and enforcing certain cultural or political leanaries needs to be addressed. The concern over QwQ-32B's alignment with Chinese regulatory norms illustrates the broader debate around how AI ethics are modeled across different national perspectives. The global community must collaborate to create ethical AI frameworks that transcend borders while respecting cultural and political contexts [InfoWorld](https://www.infoworld.com/article/3840580/alibaba-says-its-new-ai-model-rivals-deepseekss-r-1-openais-o1.html).
Politically, the development and release of QwQ-32B represent China's strategic investment in AI, aimed at diminishing the technological gap with the West. This investment is not merely a technological pursuit but also a tool for gaining soft power and geopolitical influence. The open-source nature of the QwQ-32B, touted as a major step in fostering innovation, also raises concerns about intellectual property and data sovereignty, complicating international relations in technology. These geopolitical implications necessitate careful analysis and collaborative efforts in governance to prevent misuse and ensure that AI serves a global good, rather than exacerbating divides or fostering monopolistic control by few countries or corporations [InfoWorld](https://www.infoworld.com/article/3840580/alibaba-says-its-new-ai-model-rivals-deepseekss-r-1-openais-o1.html).
Despite the potential advantages, the ethical and political challenges posed by AI advancements like Alibaba's QwQ-32B cannot be ignored. Ensuring that these technologies are implemented responsibly requires extensive international cooperation. Regulatory frameworks must be crafted that ensure fairness, transparency, and respect for privacy across all applications. Furthermore, mitigating the risk of biases inherent in model training, particularly those reflecting the developer's regional stances, is essential for maintaining objectivity and neutrality in AI applications. The open dialogue between nations and tech companies could pave the way for a future where AI technologies are developed with a keen sense of ethics and responsibility, benefiting all of humanity [InfoWorld](https://www.infoworld.com/article/3840580/alibaba-says-its-new-ai-model-rivals-deepseekss-r-1-openais-o1.html).
Challenges and Considerations for Ethical Deployment
The ethical deployment of AI models, especially large language models like Alibaba's QwQ-32B, involves a multitude of challenges and considerations. One primary concern is data governance, which refers to how data is collected, stored, and utilized. This is particularly significant for models developed in regions with stringent regulatory environments, such as China. Ensuring compliance with local data protection laws while maintaining global applicability is crucial for gaining trust and wider acceptance. Huawei, for instance, faced similar challenges, needing to align its innovations with both Chinese regulations and international standards to expand its AI solutions globally ().
Learn to use AI like a Pro
Get the latest AI workflows to boost your productivity and business performance, delivered weekly by expert consultants. Enjoy step-by-step guides, weekly Q&A sessions, and full access to our AI workflow archive.
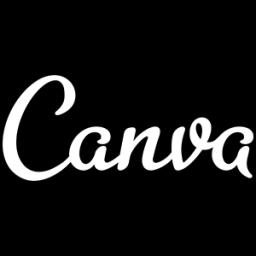
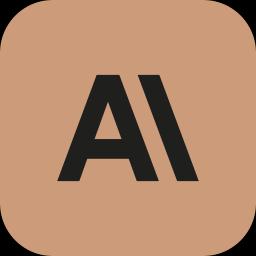
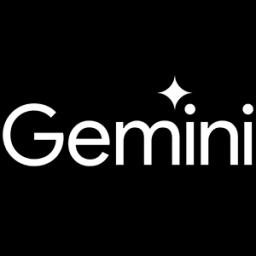
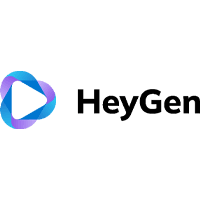
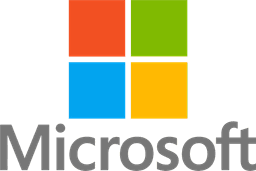
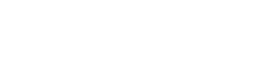
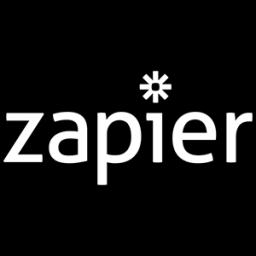
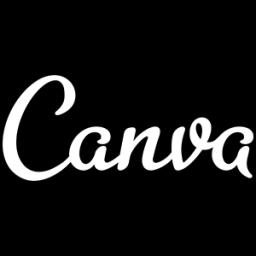
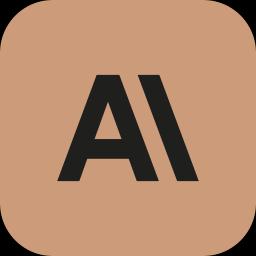
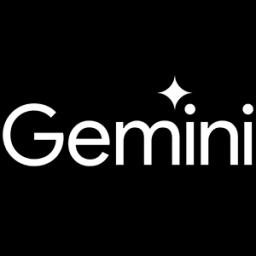
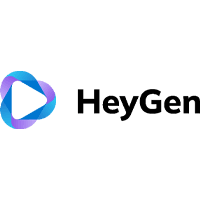
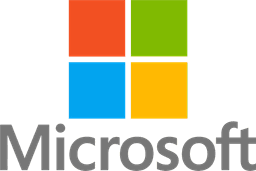
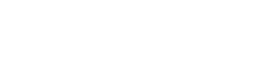
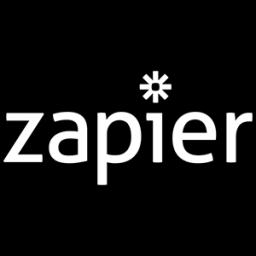
Another significant consideration is bias and transparency. AI models like QwQ-32B must be meticulously evaluated to deter biases that could arise from their training datasets, which may inadvertently reflect political, cultural, or social prejudices inherent in the data source region. Addressing these biases is fundamental to ethical AI deployment, especially for AI models deployed across diverse geographies. Leveraging techniques such as augmenting training datasets with varied cultural perspectives can help. Moreover, the transparency of AI operations through open-source frameworks is a strategic approach to inviting scrutiny and collaborative improvements from the global AI community. This sharing of knowledge, although advancing innovation, also needs careful governance to prevent misuse ().
The potential for misuse is a major ethical concern that must be addressed through robust oversight and regulation. Open-weight models like QwQ-32B, while promoting innovation and accessibility, also pose risks of being adopted for unintended malicious purposes if not properly controlled. The model’s alignment with ethical guidelines and international standards must be meticulously managed to circumvent its application for harmful activities. Establishing stringent ethical frameworks and international collaboration on AI governance can help in mitigating such risks and fostering a balanced technological landscape.
Ultimately, the deployment of AI models such as Alibaba’s QwQ-32B requires a holistic approach that considers economic, social, and political ramifications. This involves not only technological advancements but also ethical foresight to ensure that the models enhance human capabilities without undermining societal norms and regulations. The discourse on ethical AI deployment must continue to evolve alongside technological progress, ensuring a future where AI technologies are inclusive, transparent, and aligned with global societal values ().