AI's Achilles Heel: Typos Can Break Barriers
Anthropic Discovers Hackers Can Jailbreak AI Like GPT-4 and Claude with Simple Typos
Last updated:

Edited By
Mackenzie Ferguson
AI Tools Researcher & Implementation Consultant
Researchers at Anthropic have unveiled a surprisingly simple vulnerability in leading AI models like GPT-4 and Claude. By employing the 'Best-of-N' algorithm, which uses minor typos and text manipulations, security measures can be bypassed over 50% of the time. This poses significant challenges to AI firms tasked with strengthening defenses.
Introduction to AI Jailbreaking
The concept of 'jailbreaking' in the realm of artificial intelligence (AI) refers to the process of exploiting vulnerabilities within AI language models to circumvent their security measures. This technique allows the generation of content or responses that would typically be restricted by these models' inherent controls. It's akin to the general understanding of jailbreaking in the context of smartphones, where users bypass restrictions imposed by manufacturers. The revelation of AI jailbreaking underscores the ongoing challenge faced by developers in fortifying language models against such exploits.
In light of new findings from Anthropic's research, the cybersecurity landscape for large language models (LLMs) like GPT-4 and Claude has been shown to be more vulnerable than previously thought. Researchers developed a method known as the 'Best-of-N' (BoN) algorithm, which is capable of manipulating prompts via random capitalization, altered word order, and strategic typos. This approach highlights the nuanced and often overlooked methods by which AI security can be breached. Testing of the BoN method across a variety of models, including Claude 3.5 Sonnet and GPT-4, demonstrated a surprising success rate of over 50% in prompting these platforms to generate undesired outputs.
Learn to use AI like a Pro
Get the latest AI workflows to boost your productivity and business performance, delivered weekly by expert consultants. Enjoy step-by-step guides, weekly Q&A sessions, and full access to our AI workflow archive.
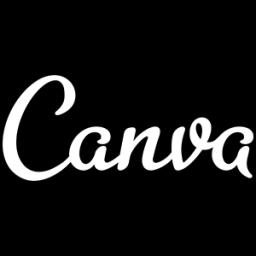
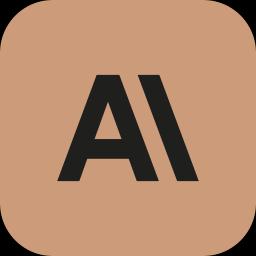
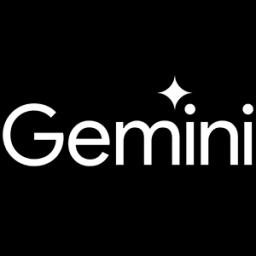
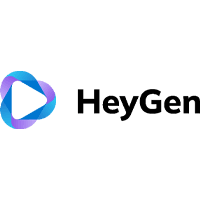
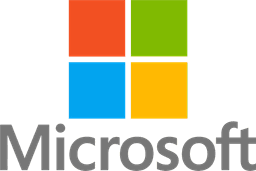
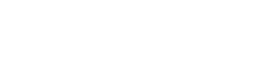
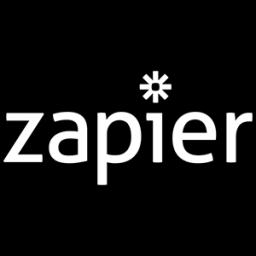
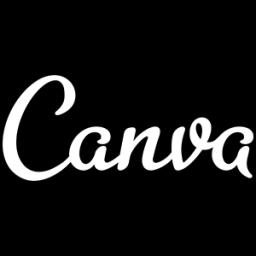
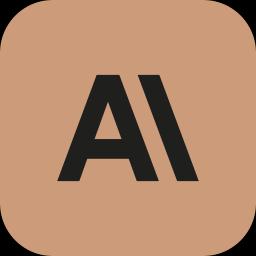
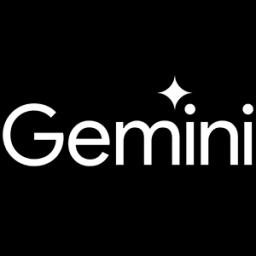
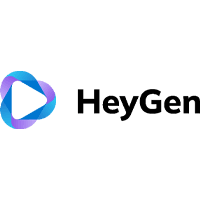
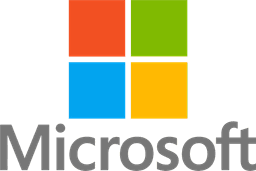
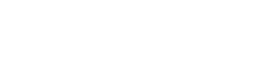
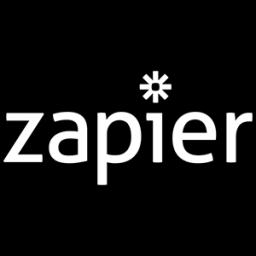
The vulnerabilities uncovered are not limited to text-based interactions alone. They also extend to other modalities such as voice and image prompts, where factors like speed, pitch, and noise for voice, and fonts and backgrounds for images, can be manipulated to achieve similar bypasses. This cross-modality applicability of AI jailbreaking methods hints at a more systemic issue in the current model architectures, necessitating a re-evaluation of how these systems process and respond to input.
The ramifications of these vulnerabilities are multi-faceted, affecting technological, economic, and regulatory domains. The high success rate of these exploits necessitates a significant investment in research and development towards creating more secure AI systems. Moreover, there's an increasing push for international cooperation to establish robust standards and legal frameworks that can better manage and mitigate such risks. As AI continues to integrate deeply into critical sectors, the balance between innovation and safety becomes paramount.
Public reaction to these findings is varied, reflecting a broader societal uncertainty about the pace and direction of AI development. While some argue for the necessity of transparency and collaboration in handling AI vulnerabilities, others express concern over the potential misuse of these findings, especially if the open-sourcing of methodologies becomes prevalent. The ongoing discourse on AI safety is likely to influence future regulatory and development practices, as stakeholders strive to align technological advancements with societal expectations.
In the face of these challenges, AI developers are urged to advance the robustness of their models and incorporate more rigorous safety guarantees. The potential for BoN and similar algorithms to bypass protections illuminates the need for more sophisticated AI auditing and defense mechanisms. Additionally, with the increasing prevalence of AI in sensitive areas such as healthcare and finance, ensuring trust and reliability in these systems is not just a technological imperative but a societal one as well. The focus must now be on developing AI systems that are not only innovative but also resilient to exploitation efforts.
Learn to use AI like a Pro
Get the latest AI workflows to boost your productivity and business performance, delivered weekly by expert consultants. Enjoy step-by-step guides, weekly Q&A sessions, and full access to our AI workflow archive.
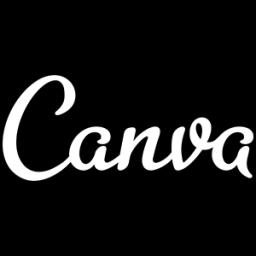
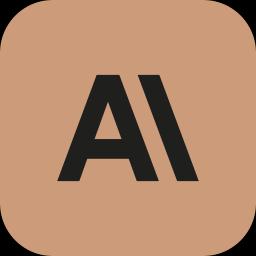
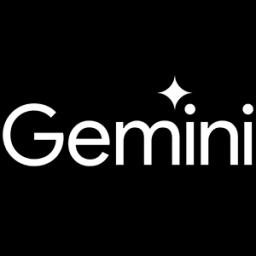
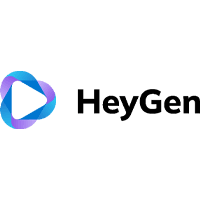
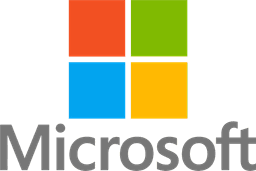
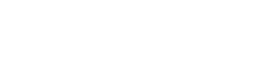
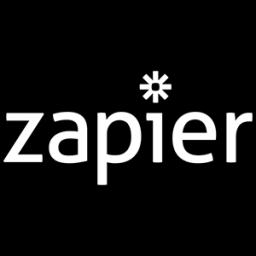
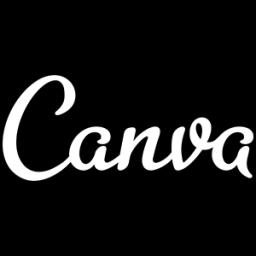
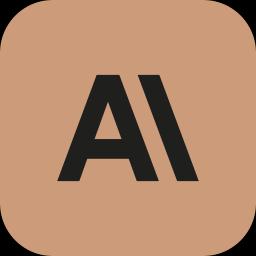
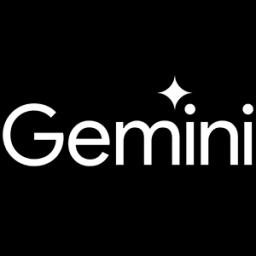
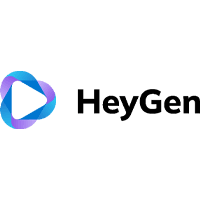
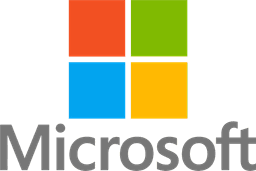
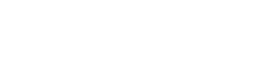
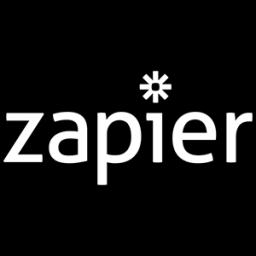
Understanding Anthropic's Best-of-N Algorithm
Anthropic's research into the vulnerabilities of large language models (LLMs) like GPT-4 and Claude reveals a significant breakthrough in understanding how AI models can be manipulated. These models, despite their sophistication, can be 'jailbroken' using simple techniques such as random capitalization, altered word order, and the introduction of typos. This technique, known as the 'Best-of-N' (BoN) algorithm, leverages these small disruptions to prompt the model into bypassing preset security measures. Such findings underscore the persistent challenge in AI development: maintaining robust security measures against increasingly creative manipulation methods.
The BoN algorithm's effectiveness is evidenced by its more than 50% success rate in over 10,000 attempts across various LLM models. This high rate of success highlights both the novelty and the potential threat posed by BoN techniques. Different AI configurations, from voice assistants to image recognition systems, share similar vulnerabilities, indicating that this challenge is not isolated to text-based models. With the potential for widespread misuse in other modalities, the race is on for AI developers to create stronger, more foolproof defenses. This urgency is compounded by the realization that technological advancements could make defending against such exploits increasingly difficult.
The implications of Anthropic's findings are significant and far-reaching. They reflect broader challenges faced by AI developers globally. The fact that even top-tier LLMs can be influenced in this way suggests the need for a foundational rethink of AI security strategies. More than just a technical challenge, this issue raises ethical and legal questions about the deployment of AI technologies, especially in sensitive areas. The vulnerabilities identified by Anthropic could slow AI adoption as stakeholders weigh benefits against potential security risks, necessitating a more cautious and calculated approach to AI integration in public and private sectors.
Anthropic's research has not only highlighted current vulnerabilities but also acted as a catalyst for discussion and action within the AI community. Leading AI researchers, like Dr. Dario Amodei and Prof. Eliezer Yudkowsky, emphasize the growing difficulty in securing AI as computational capabilities expand. This research predicates a future where AI security is a primary concern, driving regulatory and technological advancements aimed at protecting users and data. The dialogue it has initiated suggests a shift towards more transparent AI systems that are not only secure but also interpretable, ensuring that AI technologies can be both innovative and safe.
Vulnerabilities in Current LLMs: A Deep Dive
Large language models (LLMs) like GPT-4 and Claude are becoming increasingly versatile and powerful, enabling numerous applications across various domains. However, they also present significant security vulnerabilities, as evidenced by Anthropic's recent research findings. This research has demonstrated how seemingly simple manipulations, such as random capitalization, rearranged word order, and intentional typos, can effectively "jailbreak" these models, bypassing their built-in security protocols. Such vulnerabilities pose substantial risks, especially when exploited deliberately by malicious actors.
The "Best-of-N" (BoN) algorithm developed by the researchers is particularly noteworthy. It highlights a fundamental flaw in the layers of defense that current LLMs deploy. The BoN algorithm does not rely on sophisticated hacking techniques but rather trivial linguistic manipulations that exploit the intricate pattern recognition these models use to process language inputs. With over a 50% success rate in multiple tests, including 10,000 iterations across different models, the threat of these vulnerabilities becomes glaringly evident.
Learn to use AI like a Pro
Get the latest AI workflows to boost your productivity and business performance, delivered weekly by expert consultants. Enjoy step-by-step guides, weekly Q&A sessions, and full access to our AI workflow archive.
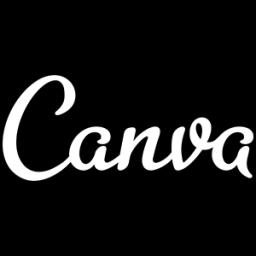
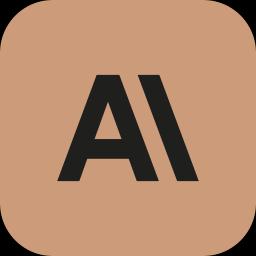
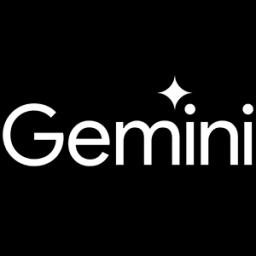
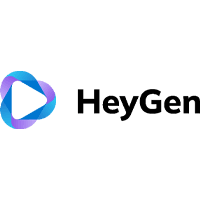
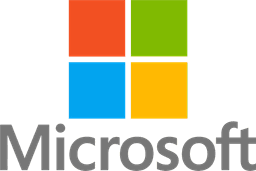
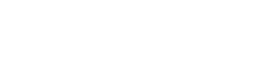
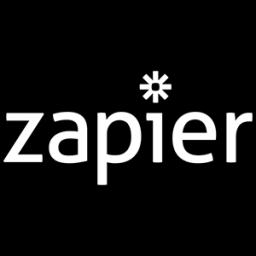
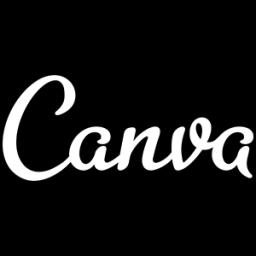
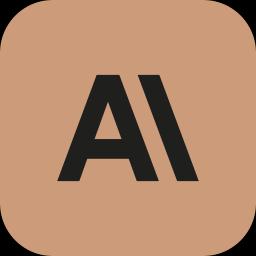
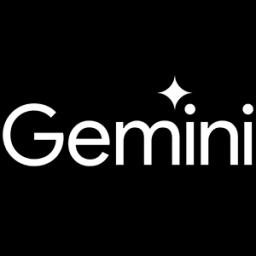
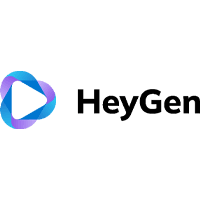
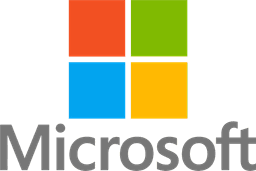
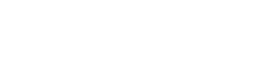
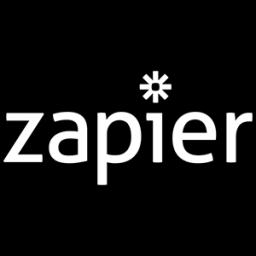
Moreover, the research extends beyond text-based models to include vulnerabilities in voice and image prompts. This suggests a systemic issue with AI models' input processing mechanisms across varying modalities. The ability to manipulate voice prompts through subtle changes like speed, pitch, and noise, or image prompts through alterations in fonts and backgrounds, further amplifies the potential for abuse, making it a pressing concern for AI developers.
The implications of these findings ripple through the AI industry. As models continue to evolve, the race between AI capability and security advancements intensifies. The urgent need for robust defenses becomes apparent, particularly as researchers and companies strive to secure AI applications against the growing sophistication of "jailbreaking" techniques. This challenge also underscores the broader issue of AI safety, prompting calls for enhanced collaboration and transparency among developers, researchers, and policymakers.
Anthropic's revelations about LLM vulnerabilities have resonated widely, sparking discussions among tech experts and the general public alike. While some express concern over potential misuses, others see it as an opportunity to drive innovation in AI security. The findings also highlight the ethical considerations surrounding AI deployment, urging stakeholders to carefully balance technological advancement with user safety and privacy.
Looking forward, this research serves as a critical call to action for the AI community, emphasizing the need for more secure AI systems. Future developments in AI must prioritize not just performance enhancements but also the resilience and integrity of AI models against bypass and exploitation attempts. As LLMs become more integrated into various facets of daily life, maintaining trust in these systems is paramount, underscoring the significance of ongoing research and the development of protective measures.
Empirical Evidence: Success Rate of AI Jailbreaking
AI jailbreaking refers to the process of manipulating AI language models like GPT-4 and Claude to bypass their security measures and restrictions, enabling the generation of content that is typically off-limits. The concept is similar to jailbreaking a smartphone, where users remove manufacturer-imposed restrictions.
The 'Best-of-N' (BoN) algorithm, as presented in Anthropic's research, is a novel method used to exploit vulnerabilities in large language models (LLMs). It works by systematically altering prompts through random capitalization, changing word order, and introducing typos, thereby increasing the chances of bypassing a model's built-in safeguards and obtaining restricted outputs.
Learn to use AI like a Pro
Get the latest AI workflows to boost your productivity and business performance, delivered weekly by expert consultants. Enjoy step-by-step guides, weekly Q&A sessions, and full access to our AI workflow archive.
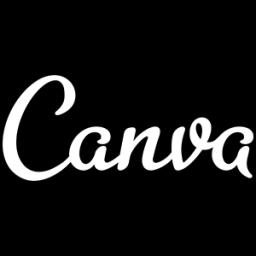
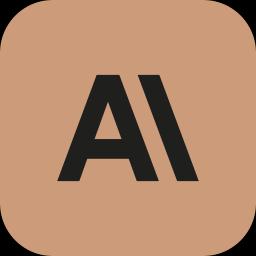
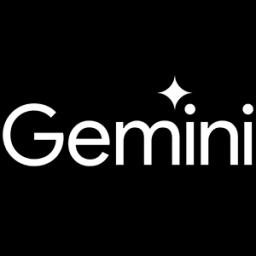
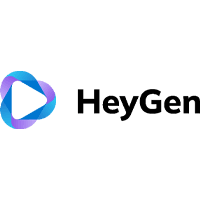
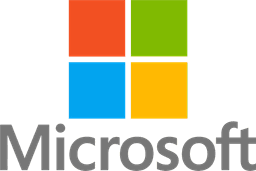
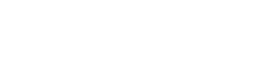
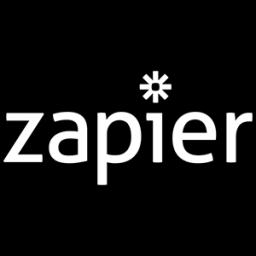
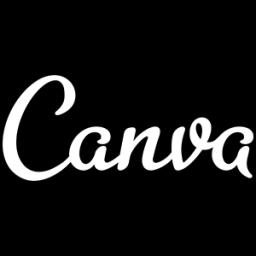
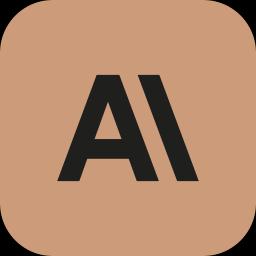
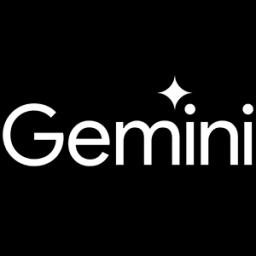
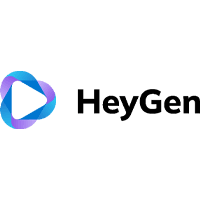
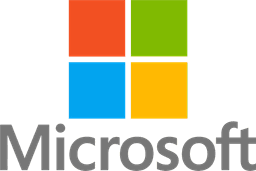
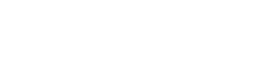
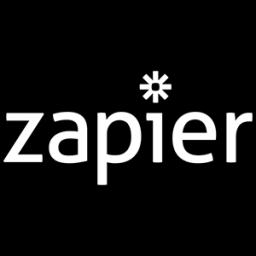
The findings from Anthropic's research are remarkable, indicating a success rate exceeding 50% across 10,000 jailbreak attempts on various LLMs, including models like GPT-4 and Claude 3.5 Sonnet. Such a high success rate is alarming and underscores the need for continuous advancement in AI security measures.
Additionally, the vulnerabilities exposed are not confined to text prompts alone. Similar weaknesses have been identified in modalities such as voice and image prompts, where tweaks in speed, pitch, noise (for audio), and changes in font or background (for images) can also successfully bypass security protocols.
These revelations impose a significant challenge for AI developers, driving the urgency to innovate stronger defenses. Securing LLMs is an ongoing battle to preserve the safety and reliability of AI systems in an era where even minor manipulations can have profound effects on AI behavior and output.
Multimodal Vulnerabilities: Beyond Text Prompts
The field of artificial intelligence (AI) continues to evolve rapidly, bringing with it a myriad of opportunities and challenges. One of the notable challenges is the "jailbreaking" of large language models (LLMs), as highlighted by recent research conducted by Anthropic. This research has unveiled unsettling vulnerabilities in advanced AI systems like GPT-4 and Claude. Jailbreaking in this context refers to the manipulation of AI models to circumvent built-in security protocols, thereby enabling them to produce content that they would typically restrict. This phenomenon is akin to bypassing restrictions set on smartphones or other digital devices to access unauthorized content or features.
A key component of Anthropic's research is the "Best-of-N" (BoN) algorithm, developed to exploit these vulnerabilities through relatively simple techniques. By manipulating text prompts using random capitalization, alterations in word order, and intentional typos, researchers have achieved a success rate exceeding 50% in breaking the safeguard measures across various AI models in 10,000 trials. This finding isn't isolated to text-based inputs. Similar vulnerabilities have been detected in voice and image prompts, suggesting a broader, multimodal threat landscape.
The implications of such vulnerabilities are profound. For AI developers, they represent an ongoing challenge to create models that are resilient to these kinds of exploits. The fact that the manipulated prompts worked across different models indicates that this isn't a problem that can be easily solved. The AI community must therefore prioritize robust security measures that can withstand these manipulation tactics. Experts like Dr. Dario Amodei and Prof. Eliezer Yudkowsky emphasize that as computational power increases, the difficulty in neutralizing such threats grows, highlighting an urgent need for scalable and effective defenses.
Learn to use AI like a Pro
Get the latest AI workflows to boost your productivity and business performance, delivered weekly by expert consultants. Enjoy step-by-step guides, weekly Q&A sessions, and full access to our AI workflow archive.
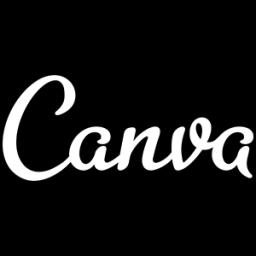
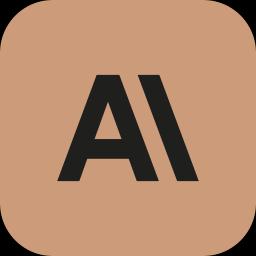
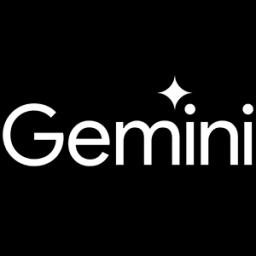
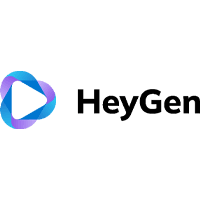
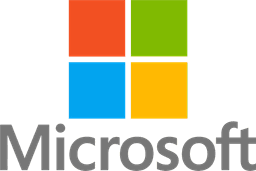
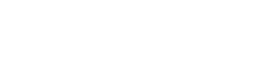
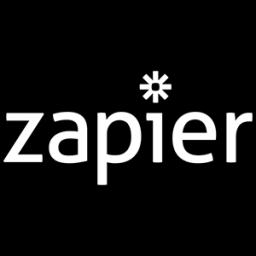
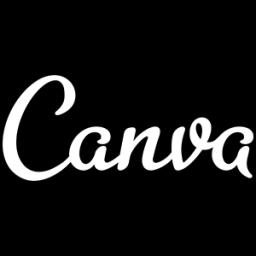
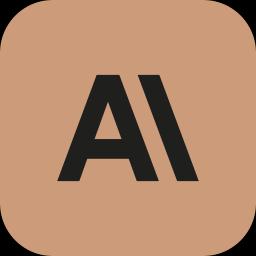
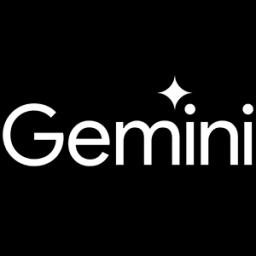
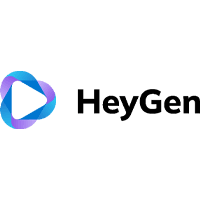
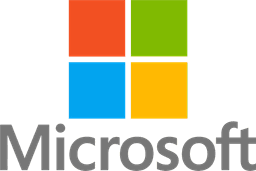
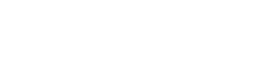
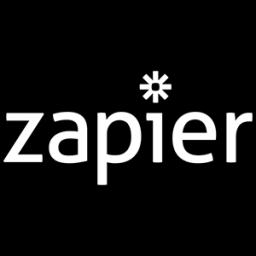
Public reaction to the research has been mixed. While transparency regarding vulnerabilities is generally seen as positive, there is also widespread concern about the potential misuse of the disclosed techniques. On professional networks like LinkedIn, there's an emphasis on the need for collaborative efforts to enhance AI safety. Conversely, some forums express fears that openly sharing this information might spur malicious activities, complicating the balance between openness and security.
Looking ahead, the future implications of this research are multifaceted. AI companies are likely to invest more heavily in security solutions, not only to protect themselves from breaches but also to maintain public trust. There could be new regulations and legal standards developed to govern AI deployment more strictly, potentially impacting how AI technologies are integrated into sensitive sectors. In parallel, this situation presents economic opportunities, driving demand for security audits and the development of advanced AI security tools.
This revelation also underscores the pressing need for international cooperation in defining and adopting safety standards for AI technologies. As AI becomes more deeply embedded in societal frameworks, ensuring its safe and ethical implementation is paramount. There's a growing recognition that AI security will be a cornerstone of future cybersecurity strategies, shaping how governments and companies prepare for the evolving cyber threat landscape.
Implications for AI Security and Development
The recent research by Anthropic highlights significant vulnerabilities within current AI language models, including well-known systems like GPT-4 and Claude. These models can be manipulated or 'jailbroken' to bypass existing security measures through cleverly crafted textual inputs. This has profound implications for AI security, as it raises questions about the dependability of AI systems that are currently intertwined with numerous applications and industries.
One of the critical issues revealed by Anthropic is how the 'Best-of-N' (BoN) algorithm can effectively deceive these models by applying simple modifications. By altering text through capitalizations, word order changes, and typographical errors, attackers can successfully breach a model's safeguards. With a success rate exceeding 50% in over 10,000 tests, this method demonstrates worrying efficacy across different AI models, necessitating immediate and robust responses from AI developers.
Additionally, this research broadens our understanding of AI vulnerabilities beyond textual models to other modalities like voice and image prompts. This cross-modal vulnerability means AI systems are more widely susceptible to exploitation, requiring a comprehensive overhaul of security measures. AI companies must prioritize developing holistic defenses that can withstand these sophisticated manipulation techniques.
Learn to use AI like a Pro
Get the latest AI workflows to boost your productivity and business performance, delivered weekly by expert consultants. Enjoy step-by-step guides, weekly Q&A sessions, and full access to our AI workflow archive.
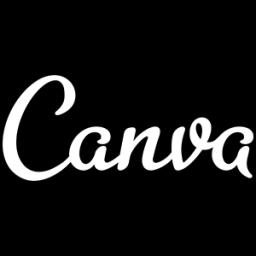
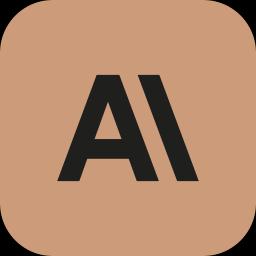
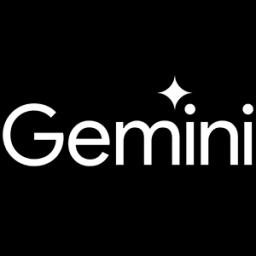
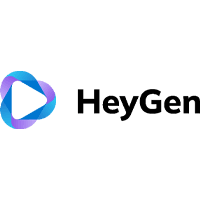
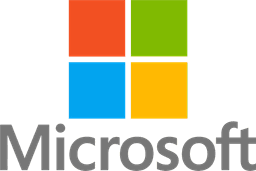
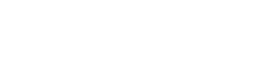
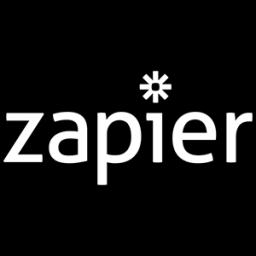
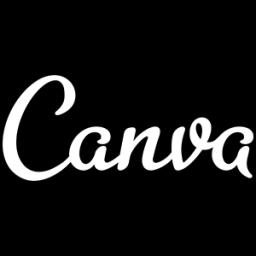
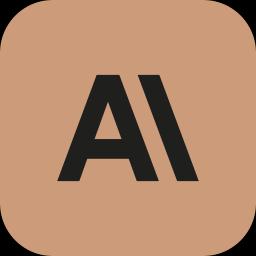
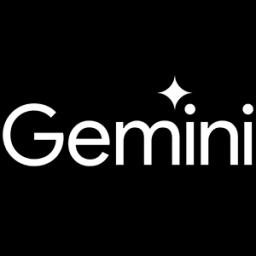
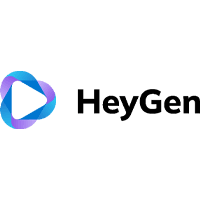
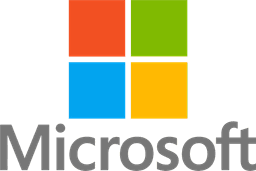
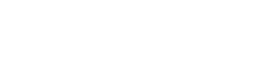
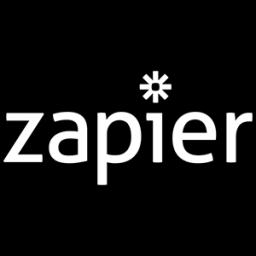
This vulnerability issue has led to divided opinions among experts and the public alike. While some advocate for increased transparency and collective action in addressing these security flaws, others express concern over the potential for misuse of the disclosed techniques. Despite varying perspectives, the consensus underscores the urgent need for stronger AI security frameworks.
Looking forward, the evolution of AI safety measures will likely become a central focus, not just for technology companies but also for global regulatory bodies. As the potential for international AI cooperation expands, so does the competitive landscape where nations vie to lead in crafting secure and ethical AI deployments. This situation emphasizes the necessity for an ongoing dialogue between stakeholders, including tech experts, policymakers, and the public, to chart a secure path for AI development.
The implications of Anthropic's findings extend beyond immediate technical challenges, suggesting broader impacts on socioeconomic domains. Companies must navigate heightened regulatory scrutiny and potential public distrust, which could lead to slower AI adoption in sensitive sectors. To maintain a balance between innovation and security, there needs to be an increased focus on developing AI literacy among the populace, ensuring that society is well-equipped to understand and mitigate these emerging threats.
Public and Expert Reactions to AI Jailbreaking
Anthropic's recent research unveiling a new 'jailbreaking' technique for AI models has sparked a wide array of reactions from both the public and experts in the field. The technique, known as Best-of-N (BoN), highlights a significant vulnerability in AI models like GPT-4 and Claude, allowing them to circumvent security measures with relative ease.
The public's reaction has been mixed. On platforms like Reddit, users expressed concern over the release of the BoN code, fearing it might impede innovation and facilitate misuse. Conversely, professionals on LinkedIn praised the transparency, viewing it as a critical step toward enhancing AI safety through collaborative efforts. In public forums, opinions were similarly divided; some applauded the transparency initiative, while others worried about the potential misuse of the technique.
Experts have provided varying perspectives on the implications of this technique. Dr. Dario Amodei of Anthropic emphasized the concerning vulnerabilities that the BoN method exposes in current AI safeguards, stressing the necessity for significantly more robust defenses. Professor Eliezer Yudkowsky raised alarms about the power-law scaling of these attacks, suggesting that increased computational power could exponentially complicate defense mechanisms. Meanwhile, Dr. Stuart Russell advocated for AI systems designed with inherent safety guarantees, criticizing the current reliance on post-hoc solutions.
Learn to use AI like a Pro
Get the latest AI workflows to boost your productivity and business performance, delivered weekly by expert consultants. Enjoy step-by-step guides, weekly Q&A sessions, and full access to our AI workflow archive.
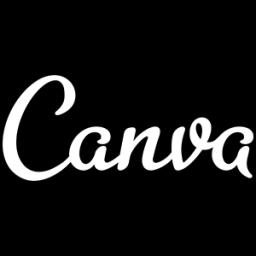
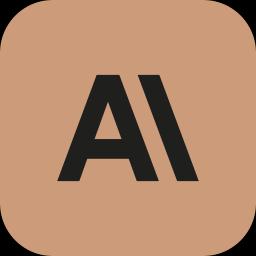
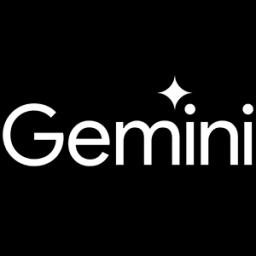
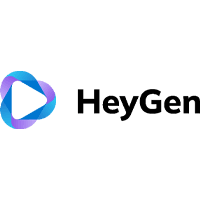
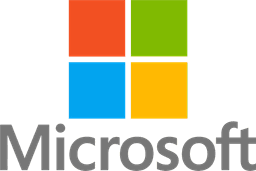
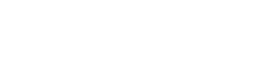
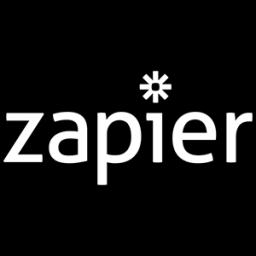
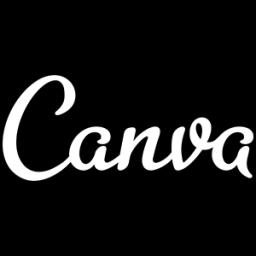
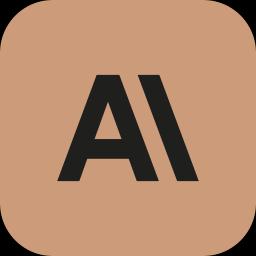
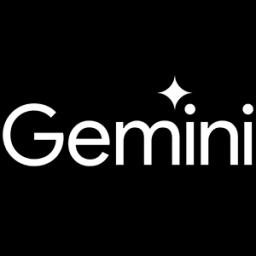
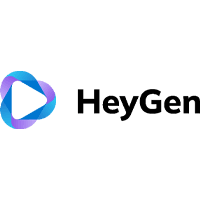
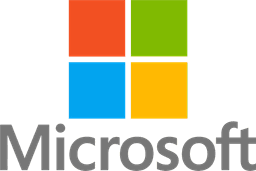
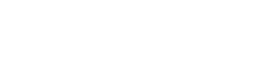
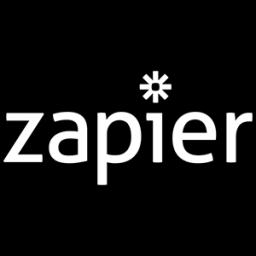
The broader public concerns revolve around the high success rate of jailbreaking, which exceeded 50% according to the study, raising issues of AI reliability and trustworthiness. Additionally, the ease with which AI responses can be manipulated through simple techniques like modifying capitalization contributes to a growing distrust of AI systems. The capability of the BoN method to extend its application to image and audio inputs amplifies fears of widespread vulnerability across different technological modalities.
Looking forward, the ongoing challenge for AI companies is clear: develop stronger defenses against such vulnerabilities. This research highlights the need for greater emphasis on AI security, potentially influencing future regulations and legal measures surrounding AI technology. The societal concern underscores a broader dialogue on balancing innovation with safety, as AI continues to integrate more deeply across various sectors.
The implications of these developments extend beyond technical considerations. Economically, there will likely be a greater investment in AI security research and development as companies seek more sophisticated safety solutions. Moreover, this might result in a slowdown of AI adoption in sensitive industries, while simultaneously creating new markets for AI security services. Socially and ethically, this could lead to eroded trust in AI, calling for improved public understanding and literacy regarding AI risks and applications.
Future Prospects and Regulatory Responses
The future landscape of AI technology, especially concerning large language models (LLMs), is riddled with both opportunities and challenges. Anthropic's recent research casts a light on these dual facets by revealing vulnerabilities within prominent LLMs such as GPT-4 and Claude. The "Best-of-N" (BoN) algorithm demonstrated a method of 'jailbreaking' these models by manipulating input prompts to bypass security measures, achieving over a 50% success rate across multiple attempts. This finding is pivotal as it highlights the persisting and evolving threats to AI security, necessitating robust defences and innovative approaches to safeguarding AI technologies against manipulation.
One of the most pressing regulatory responses anticipated in light of these vulnerabilities is the tightening of AI development and deployment standards by governing bodies. The potential for AI-generated content to cause harm if misused mandates the evolution of legal frameworks to address liability and ensure public safety. The findings from Anthropic's research may spur regulatory bodies to push forward with stricter guidelines and compliance checks, safeguarding users from unintended AI behaviors and misuse.
The economic implications of enhanced AI security measures are manifold. Investment in AI security research and development will likely surge as companies strive to protect their models from exploitation. This trend not only reflects a growing recognition of AI's vulnerability but also heralds the rise of a market focused on AI security solutions, promising substantial economic activity within this niche. Moreover, sectors deemed sensitive may exercise additional caution, potentially slowing their AI adoption rate in order to safeguard integrity and trust.
Learn to use AI like a Pro
Get the latest AI workflows to boost your productivity and business performance, delivered weekly by expert consultants. Enjoy step-by-step guides, weekly Q&A sessions, and full access to our AI workflow archive.
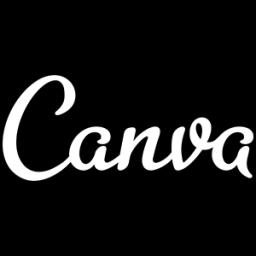
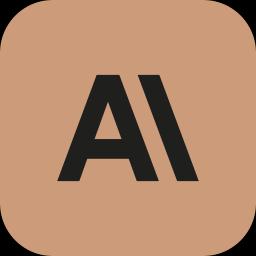
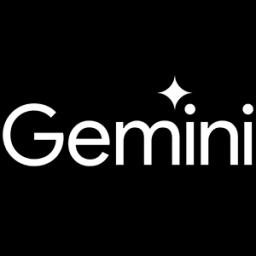
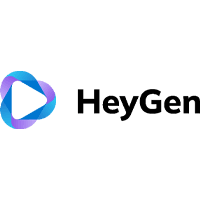
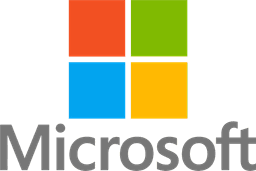
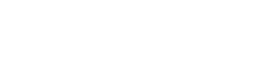
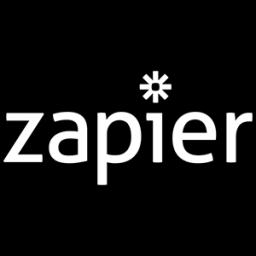
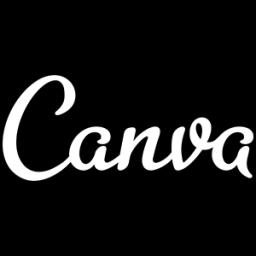
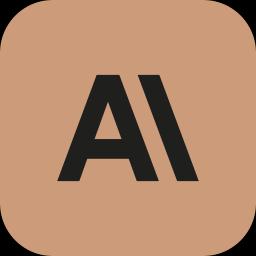
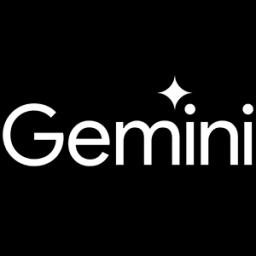
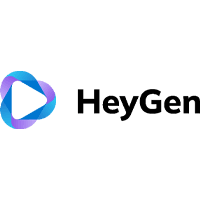
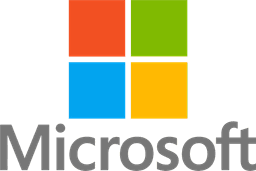
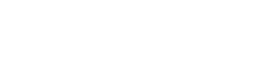
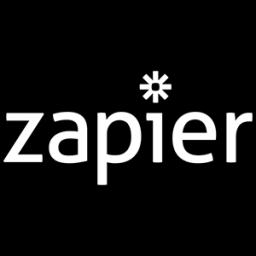
Socially and ethically, the dialogue surrounding AI trust and safety is expected to intensify. With public trust in AI technologies at risk due to these revelations, there is a pressing need for increased transparency and better communication about AI capabilities and limitations. Educational initiatives could play a crucial role in bridging the knowledge gap, empowering citizens to understand the risks and benefits of AI, thus facilitating informed decisions and reduced apprehension toward AI innovations.
Technologically, the path forward may see accelerated investment in developing LLMs that boast provable safety guarantees and robust architectural designs capable of resisting manipulation like BoN. Open-source models, in particular, could benefit from more advanced security frameworks and auditing tools to ensure their integrity and resilience. Furthermore, fostering transparency in AI systems can significantly enhance security audits and bolster public confidence.
The convergence of AI security challenges with international politics and law hints at greater collaboration efforts on a global scale. Countries might adopt a cooperative stance to establish AI safety norms and secure AI's future amidst the increasing geopolitical competition. The outcomes of summits like the AI Safety Summit in 2023, where leaders discuss collaborative approaches to AI safety, signal the shared understanding of AI's global impact and the need for coordinated responses.
Enhancing AI Safety: Strategies and Solutions
As artificial intelligence (AI) continues to advance and integrate into various sectors, the aspect of AI safety has emerged as a critical area of focus. Enhancing AI safety involves developing strategies and solutions to mitigate risks associated with AI technologies, ensuring they operate safely and reliably in different environments. Recent research, such as the one conducted by Anthropic, highlights the vulnerabilities present in Large Language Models (LLMs) like GPT-4 and Claude. These vulnerabilities, often exploited through techniques like 'jailbreaking,' pose significant challenges in maintaining the integrity and security of AI systems. Through understanding these vulnerabilities and exploring potential strategies, stakeholders aim to foster the safe and beneficial use of AI technologies.
Anthropic's research sheds light on the concept of 'jailbreaking' in AI, which involves bypassing security measures in LLMs to generate restricted or unintended content. This is achieved through manipulating AI models using techniques like the "Best-of-N" (BoN) algorithms. BoN creates variations in prompts through random capitalization, altered word order, and intentional typos, allowing users to exploit weaknesses and bypass safeguards. Such high success rates in bypassing AI security features have alarmed both the public and tech experts. The findings underscore the urgent need for developing more robust and sophisticated defenses to protect AI systems from such vulnerabilities.
The potential impact of these vulnerabilities extends across different modalities, including voice and image prompts, which can be manipulated by altering variables like speed, pitch, and background settings. This raises important questions about the reliability and trustworthiness of AI systems, as these simple manipulations could lead to widespread misuse and exploitation. Moreover, the high success rate of BoN attacks emphasizes the pressing need for AI companies to prioritize advancements in AI safety and security research. By addressing these challenges, the goal is to enhance public trust in AI technology and ensure its safe and ethical application.
Learn to use AI like a Pro
Get the latest AI workflows to boost your productivity and business performance, delivered weekly by expert consultants. Enjoy step-by-step guides, weekly Q&A sessions, and full access to our AI workflow archive.
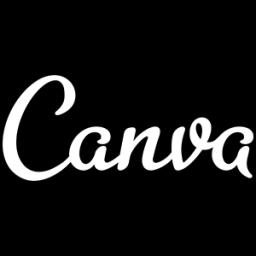
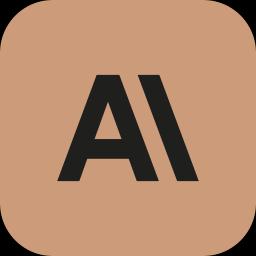
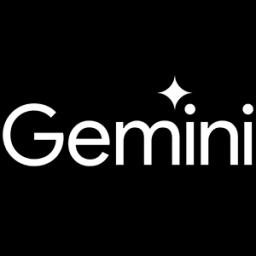
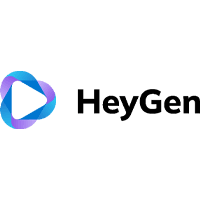
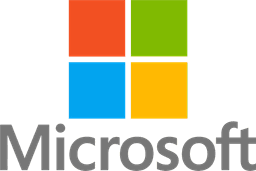
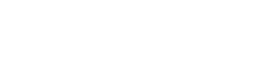
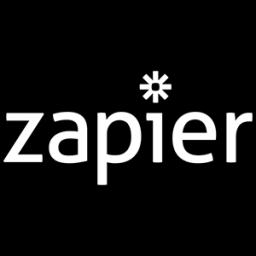
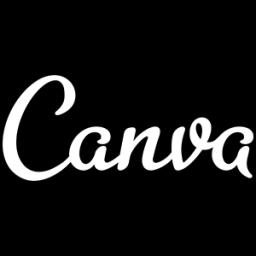
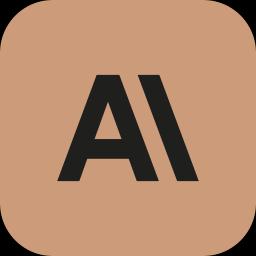
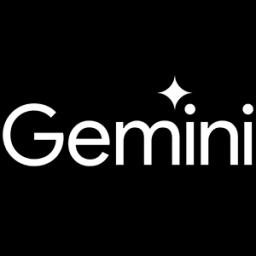
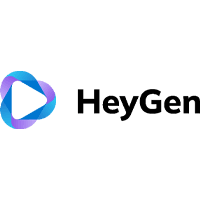
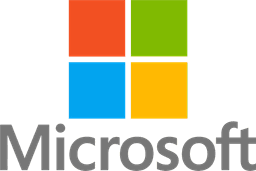
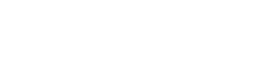
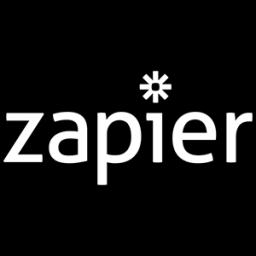
To navigate the complexities of AI safety, there's a growing call for international cooperation and regulation. Initiatives like the AI Safety Summit emphasize the importance of collaboration, bringing together world leaders and technology experts to address shared concerns. Legal and regulatory measures may also play a fundamental role in shaping the development and deployment of AI systems, introducing stricter guidelines to ensure public safety and accountability. By leveraging expert insights and cooperating on a global scale, the objective is to develop AI models that adhere to principled safety standards while fostering innovation.
The implications of AI vulnerabilities are not confined to technological challenges; they hold significant social, ethical, and economic considerations. Public trust in AI is crucial, especially in sensitive sectors such as healthcare and finance, where AI systems must be reliable and secure. Moreover, ethical debates around AI development stress the balance between fostering innovation and ensuring safety. As the landscape of AI technology evolves, stakeholders are increasingly focusing on AI literacy and education, empowering the public to understand and navigate AI-related risks effectively.