Benchmark Overhaul?
Are AI Benchmarks Becoming Obsolete? A Call for Real-World Evaluation
Last updated:

Edited By
Mackenzie Ferguson
AI Tools Researcher & Implementation Consultant
In an era of rapidly advancing AI, a TechCrunch article questions the relevance of current AI benchmarks, which are often self-reported and lack real-world applicability. The article suggests moving towards evaluation methods that focus on economic impact and practical utility rather than solely on benchmark scores, calling into question the current industry standard.
Introduction: The Benchmark Dilemma
In recent years, the AI industry has grown significantly, partly driven by the pursuit of 'benchmarks' that purportedly measure the technical prowess and capabilities of artificial intelligence systems. However, as highlighted in an insightful TechCrunch article, there is growing skepticism about the true value of these benchmarks. The article emphasizes a critical issue at the heart of the AI field: the disconnect between performance on standardized tests and real-world application. This "Benchmark Dilemma" questions whether these scores reflect genuine innovation or merely create an illusion of progress.
Currently, AI benchmarks tend to favor narrow expertise, often tailored to specific tasks, lacking the broader examinations necessary for gauging actual efficacy in diverse situations. The reliance on these scores can lead to a perilous tunnel vision, where developers and companies might optimize algorithms to 'game' the benchmark system rather than focus on producing meaningful, applicable solutions. This self-reported nature of benchmarks, as noted in the TechCrunch article, heightens these risks due to limited independent verification, making it nearly impossible to objectively evaluate the integrity and applicability of claims.
Learn to use AI like a Pro
Get the latest AI workflows to boost your productivity and business performance, delivered weekly by expert consultants. Enjoy step-by-step guides, weekly Q&A sessions, and full access to our AI workflow archive.
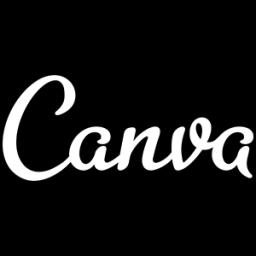
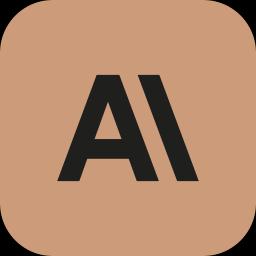
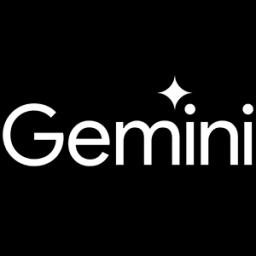
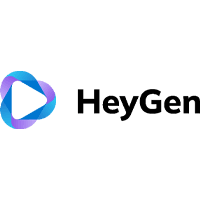
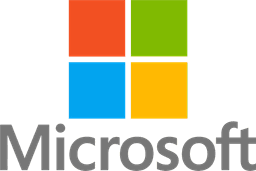
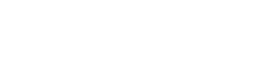
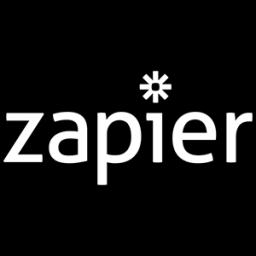
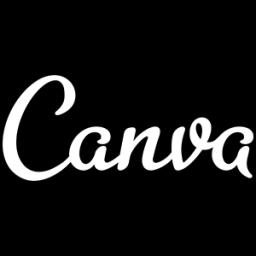
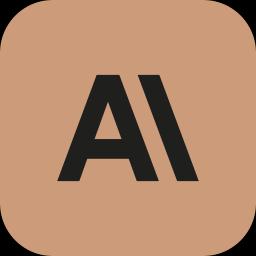
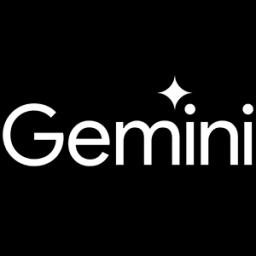
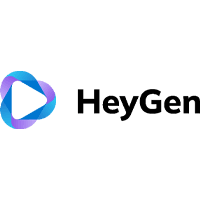
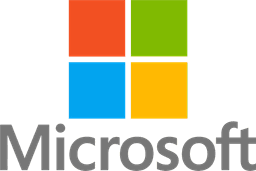
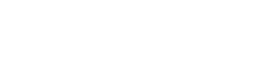
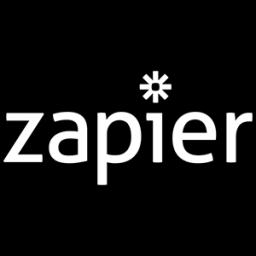
Furthermore, as industry experts, such as Wharton professor Ethan Mollick, stress, the real-world economic impact and practical utility of AI technologies must become the focal points of evaluation rather than abstract performance scores. The call for new assessment strategies is echoed by many aiming for metrics that genuinely represent an AI system's productivity and social contributions. These suggestions include adopting economic impact measurements and standardized benchmarks facilitated by independent bodies to ensure transparency and reliability.
The burden of AI benchmarks as the primary evaluative tools has led to a myriad of issues, ranging from inflated investor expectations to misallocated resources within AI-driven projects. As initiatives like the EU AI Act begin enforcing clearer standards for testing and transparency, the industry faces the imperative to evolve beyond traditional metrics. Such transformations promise a landscape where AI developments are critically aligned with tangible real-world needs, enabling actual technological advancements instead of mere statistical triumphs.
Understanding AI Benchmarks: Strengths and Weaknesses
AI benchmarks have been a cornerstone of evaluating artificial intelligence systems, providing a quantitative measure of capabilities and progress. However, these benchmarks often present both strengths and weaknesses. According to a comprehensive article on TechCrunch, while AI models like xAI's Grok 3 show impressive benchmark performances, the broader relevance and application of these metrics are questionable. One of the primary criticisms is that benchmarks do not always correlate with real-world applicability, often failing to encompass the vast array of scenarios and problems these models are designed to tackle. This disconnection raises significant concerns about how much weight these numerical scores should carry in determining an AI model's effectiveness and readiness for practical deployment.
The self-reported nature of benchmarks is another major concern. Companies often publish performance data without independent verification, leading to skepticism about the authenticity of these claims. As critics point out, these benchmarks are not just tests of AI capabilities, but also reflections of strategic marketing by companies looking to position their models favorably in a competitive market. The TechCrunch article underscores the need for independently assessed benchmarks that hold companies accountable and ensure the integrity of the AI's reported performance.
Learn to use AI like a Pro
Get the latest AI workflows to boost your productivity and business performance, delivered weekly by expert consultants. Enjoy step-by-step guides, weekly Q&A sessions, and full access to our AI workflow archive.
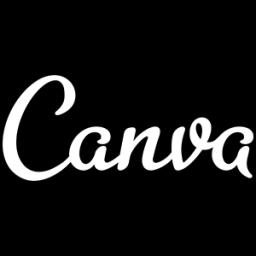
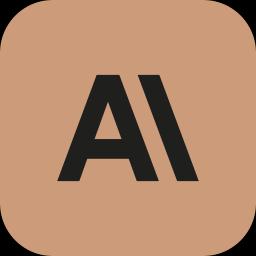
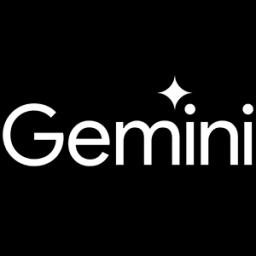
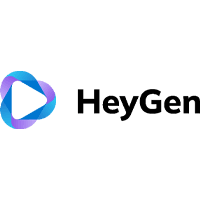
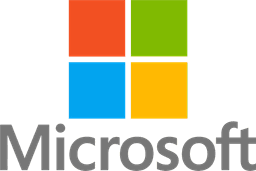
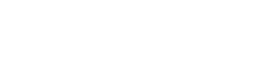
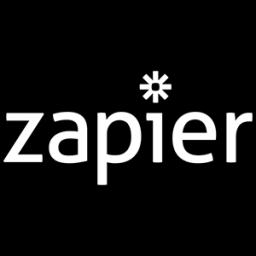
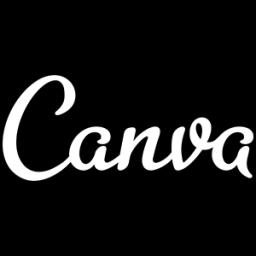
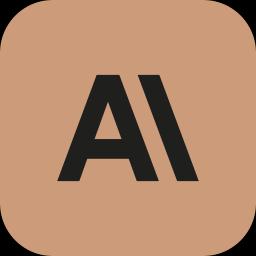
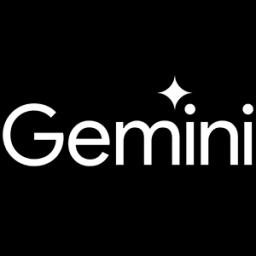
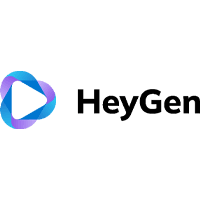
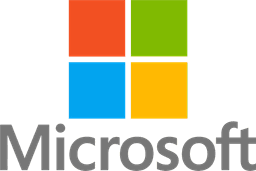
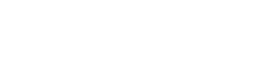
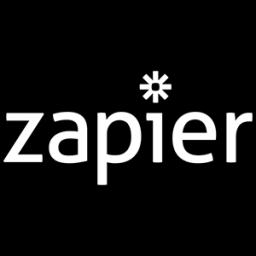
Moreover, the limited scope of current benchmarks often means that they focus on narrow domains of knowledge, which can inflate the perceived competence of AI models in those areas while neglecting their performance in others. This specificity limits the benchmarks' ability to fully represent a model's potential across diverse tasks and environments. Critics advocate for alternative methods of evaluation that emphasize real-world impact and economic utility over sheer computational prowess. This approach could bridge the gap between theoretical performance and actual deployment scenarios, providing a more nuanced understanding of an AI's capabilities and drawbacks.
Despite their criticisms, benchmarks remain a vital tool in AI development, offering a standardized way to compare models and track technological progress. For instance, the European Union's recent regulations requiring more rigorous testing and transparency have been seen as a significant step towards improving benchmark reliability. By mandating independent verification and requiring all models to undergo standardized tests, the EU AI Act aims to enhance the trustworthiness of AI evaluations, aligning them more closely with practical applications and societal needs. This shift towards better governance of AI benchmarks suggests a future where benchmarks align more closely with practical relevance and ethical standards.
The Grok 3 Case: A Benchmark Performance Paradox
The Grok 3 case presents a compelling example of the complexities and contradictions inherent in current AI benchmark evaluations. This scenario is underscored by a TechCrunch article that questions the validity and relevance of AI benchmarks in measuring true technological capability and utility. Despite Grok 3's impressive performance on various established benchmarks, the article suggests that these scores might not accurately reflect the model's real-world applicability or usefulness. The discourse around Grok 3 underscores a broader industry issue: the reliance on benchmarks that may not capture practical utilities or innovations necessary for AI's long-term success .
The situation with Grok 3 also highlights the pitfalls of self-reported benchmarks. While Grok 3 has outperformed in certain areas, it's crucial to approach these results with caution due to the lack of independent verification. The TechCrunch piece emphasizes the need for more transparent and reliable testing methods that better align with economic impacts and tangible outcomes, rather than abstract numerical rankings. This reflects a growing consensus among experts and the public pushing for benchmarks that matter not just in theory but in practice .
Furthermore, Grok 3's performance has fueled debates over how AI should be evaluated. Critics argue that benchmarks should not be the ultimate measure of technological worth but rather one of many tools used to assess a model's potential impact. The TechCrunch discussion points to alternative metrics, such as economic impact assessments and real-world adoption rates, which could offer a more rounded evaluation framework. These alternatives are proposed to bridge the gap between AI capabilities and actual societal benefits .
In conclusion, while the Grok 3 model excels on paper, the case illustrates the significant limitations inherent in traditional benchmarking approaches. This situation serves as a call to action for the broader AI community to develop and adopt more meaningful evaluation standards. As this narrative develops, it becomes apparent that the future of AI lies not just in attaining high benchmark scores but in achieving real-world relevance and utility. As the TechCrunch article advocates, ignoring AI benchmarks might be prudent until they truly reflect a model's comprehensive applicability and effectiveness .
Learn to use AI like a Pro
Get the latest AI workflows to boost your productivity and business performance, delivered weekly by expert consultants. Enjoy step-by-step guides, weekly Q&A sessions, and full access to our AI workflow archive.
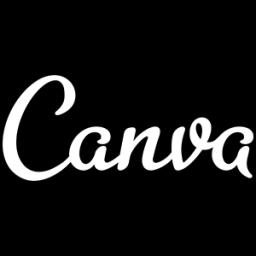
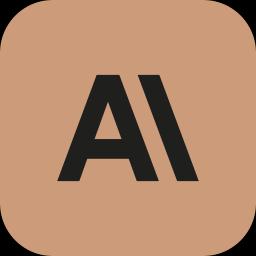
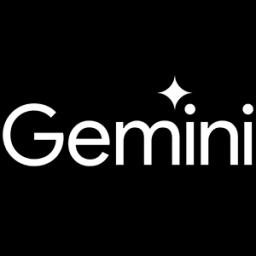
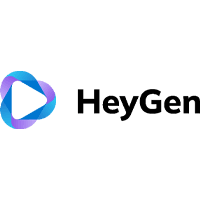
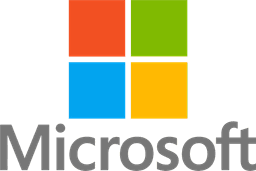
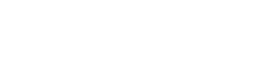
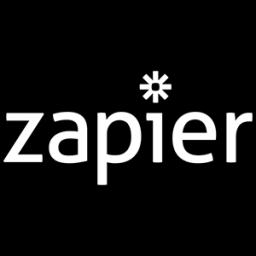
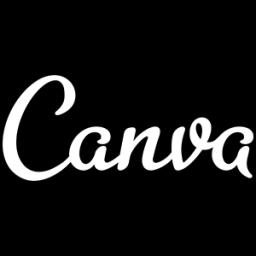
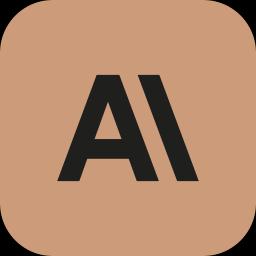
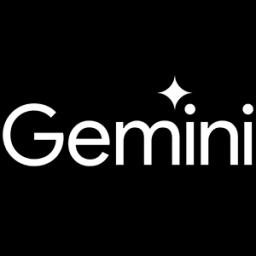
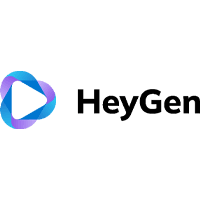
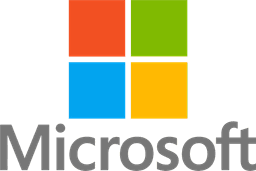
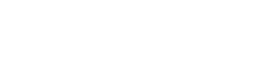
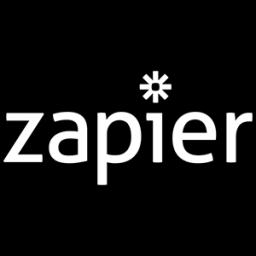
Criticisms Against Current Benchmarks
The validity of current AI benchmarks has increasingly come under scrutiny for several glaring issues, as scrutinized in a recent TechCrunch article. These benchmarks, often self-reported by companies, lack independent verification, leading to questions about their reliability. Additionally, they tend to focus narrowly on specific domains, which does not adequately reflect an AI system's general applicability across diverse real-world scenarios. This narrow scope often fails to capture the nuances of practical utility, rendering high benchmarks more symbolic than substantive. Moreover, the benchmarks' emphasis on theoretical performance metrics often neglects their disconnect from real-world applications, where AI's true value is operationalized.
Proposed Alternatives for Evaluating AI
The current discourse surrounding AI benchmarks shines a spotlight on their inadequacy in assessing AI systems' true efficacy. As raised in a TechCrunch article, benchmarks are problematic due to issues such as self-reporting bias and their narrow focus that does not translate into real-world applications. The limitations of these benchmarks are underscored by their inability to provide meaningful insights into AI's practical utility, prompting a call for alternative evaluation methods.
One of the most discussed alternatives to traditional AI benchmarks is the measurement of economic impact. This alternative focuses on assessing how AI technologies contribute to economic growth and productivity, rather than merely excelling in rigid test conditions. As emphasized by experts, aligning AI evaluation metrics with economic outcomes can offer a clearer picture of an AI system's value and impact on the industry, shifting focus from abstract scores to tangible economic benefits.
Real-world adoption metrics serve as another viable alternative for AI evaluation. By focusing on how widely and effectively AI technologies are used across various industries, stakeholders can gauge the true success and applicability of these technologies. Widespread adoption and integration into existing workflows can demonstrate an AI system's capability to deliver practical benefits, thus offering a more holistic perspective than traditional benchmarks. This approach resonates with recent industry insights, advocating for a finer balance between theoretical performance and actual utility.
There is a growing consensus about the need for independent testing bodies to oversee and standardize AI evaluation methods. This proposal envisions an objective and transparent testing process, free from the biases and limitations plaguing current benchmark systems. Establishing such independent authorities could lead to the development of standardized benchmark suites, ensuring all AI systems undergo consistent and fair evaluation processes. Such organizational oversight would address both transparency and verification issues, garnering trust from stakeholders and enhancing the reliability of AI assessments.
Moreover, some argue for practical utility assessments as a robust alternative to current benchmarks. These assessments would evaluate AI systems based on their effectiveness in real-world applications, bridging the gap between technical performance and everyday functionality. By highlighting how AI influences real-world scenarios beyond controlled testing environments, practical utility assessments would enable practitioners to make informed decisions based on an AI model's actual capabilities rather than theoretical perfection. This approach aligns with calls for prioritizing concrete benefits over speculative, benchmark-driven claims, as outlined in the TechCrunch article.
Learn to use AI like a Pro
Get the latest AI workflows to boost your productivity and business performance, delivered weekly by expert consultants. Enjoy step-by-step guides, weekly Q&A sessions, and full access to our AI workflow archive.
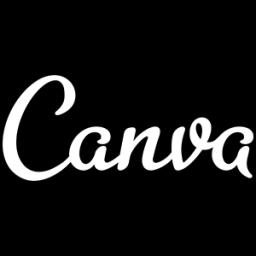
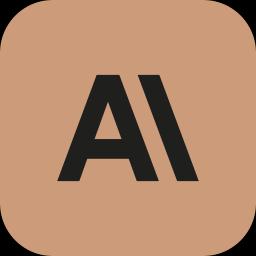
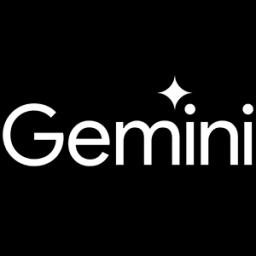
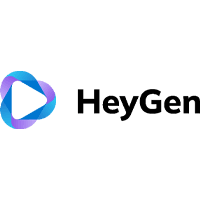
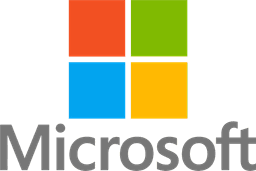
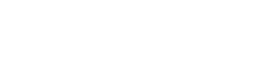
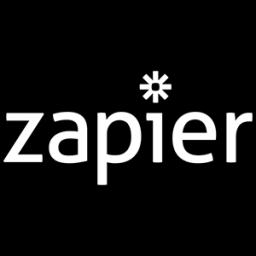
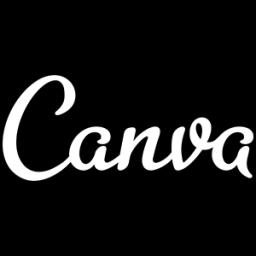
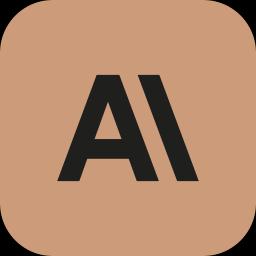
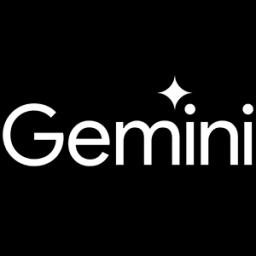
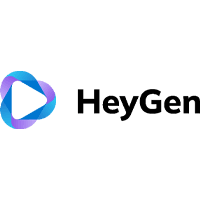
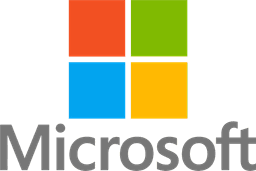
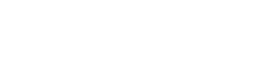
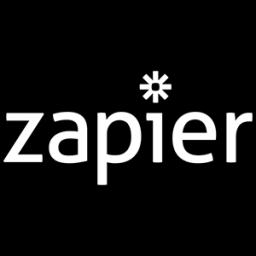
The Role of Independent Verification
In the rapidly evolving landscape of artificial intelligence, independent verification has emerged as a cornerstone of credible evaluation processes. A prominent issue with existing AI benchmarks is their reliance on self-reported data, which often lacks third-party oversight and questioning their validity. In an era where AI systems significantly impact society, having independent entities verify these benchmarks ensures that the claims made by organizations are accurate and unbiased. The call for independent verification is not merely a procedural necessity; it's a foundational pillar to forge trust among stakeholders, be it consumers, regulatory bodies, or industry peers.
The limitations of current AI benchmarks underscore the urgent need for independent verification. Most AI models today are assessed based on narrowly defined criteria, which may not necessarily reflect their real-world applicability. The Grok 3 model, for instance, demonstrated excellent performance in selective tests, yet these scores were met with skepticism, highlighting the incongruence between benchmark outcomes and functional efficiency in practical settings. By integrating independent verification, stakeholders can focus on comprehensive assessments that encompass a wider array of performance metrics tailored to real-world applications.
Independent verification not only addresses the credibility gap in AI benchmarking but also fosters innovation. When benchmarks are verified independently, it creates an equitable playing field where companies are incentivized to genuinely enhance their AI models rather than game the metrics. This shift encourages a move towards performance metrics aligned with economic impact and societal benefits, rather than abstract scores. Such focus on meaningful improvement can drive innovation that directly benefits users, making AI technologies more reliable and applicable across diverse domains.
Apart from enhancing transparency and accountability, independent verification in AI benchmarks aligns with global regulatory trends. The European Union's introduction of standardized testing protocols as part of the AI Act exemplifies this shift towards rigorous independent scrutiny. By mandating independent verification, regulation can help standardize benchmarks across the industry, reducing discrepancies and ensuring that AI claims are not just hyperbolic assertions but are backed by verifiable data. Such measures can accelerate the adoption and integration of AI technologies in critical sectors with heightened assurance of their safety and effectiveness.
Public reaction towards AI benchmarks has been predominantly skeptical, particularly when claims of groundbreaking performance fail to translate into tangible benefits. Independent verification can bridge this trust chasm by ensuring that performance metrics reflect genuine capabilities. As echoed by industry experts and reflected in public discussions, there is a pressing demand for benchmarks that are not only rigorous but also representative of how AI systems perform in everyday applications. Thus, embracing independent verification is a crucial step towards achieving transparency and instilling confidence in AI advancements.
Industry Case Studies Illustrating Benchmark Issues
Industry case studies often reveal the stark reality behind AI benchmark issues, highlighting a recurring theme of inconsistency between reported metrics and actual performance. One notable example is illustrated by DeepMind's Gemini 1.5 model, which faced scrutiny over its selective benchmark reporting practices. Initially, DeepMind provided results that seemed to position Gemini 1.5 as a frontrunner in AI capabilities. However, the subsequent public pressure forced them to release additional results that were less flattering. This incident, reported by The Verge, underscores a broader issue in the industry: the lack of transparency and the propensity for companies to cherry-pick data to present their products in the best possible light.
Learn to use AI like a Pro
Get the latest AI workflows to boost your productivity and business performance, delivered weekly by expert consultants. Enjoy step-by-step guides, weekly Q&A sessions, and full access to our AI workflow archive.
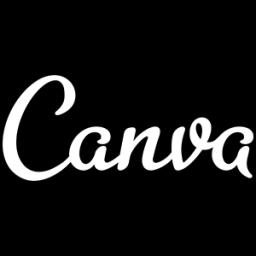
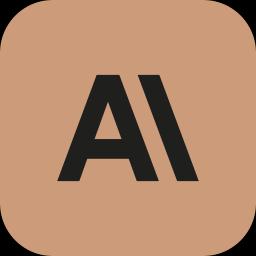
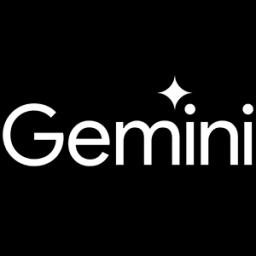
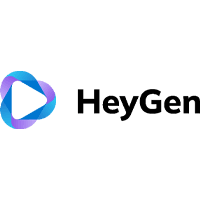
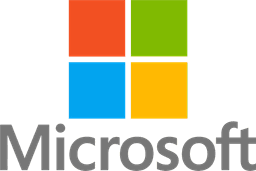
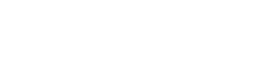
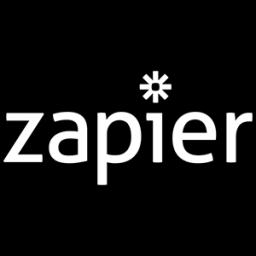
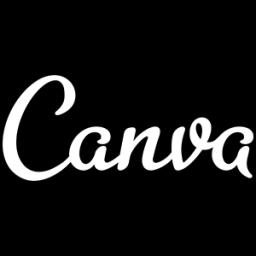
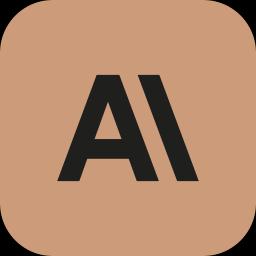
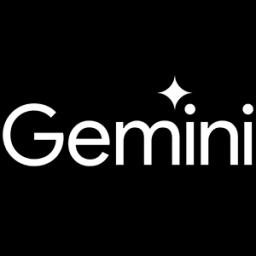
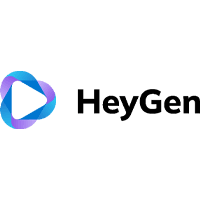
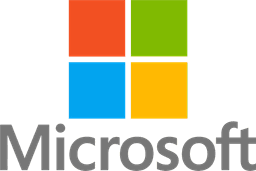
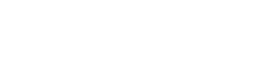
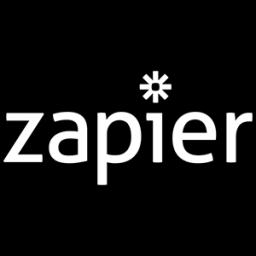
Similarly, the partnership between Microsoft and Inflection AI brought benchmark manipulation issues to the forefront. Despite Inflection AI's Pi model achieving remarkable scores in specific tests, independent researchers identified gaps between these results and the model's performance in real-world scenarios. An ArXiv study pointed out discrepancies that suggest some benchmarks are being tailored to inflate perceived capabilities. This case highlights the critical need for independent verification of AI models to ensure that benchmark scores are not only accurate but also reflective of genuine operational effectiveness.
The introduction of the European Union's AI Act demonstrates a regulatory approach to addressing benchmark issues by mandating standardized testing and transparency. This legislative move requires companies to submit their AI models for independent testing before they can enter the market, as noted in an article by Digital Strategy. This act represents a significant shift towards accountability in AI development, aiming to align benchmark results more closely with real-world impact and safety considerations.
Furthermore, OpenAI’s decision to pause the development of GPT-5, as detailed on OpenAI's blog, reflects growing internal recognition of benchmarking's limitations. By redirecting focus toward developing more meaningful evaluation metrics, OpenAI aims to ensure that its AI systems not only pass tests but exercise practical utility. This move is part of a larger industry trend where AI developers recognize that benchmarks, while useful, do not fully capture an AI system's value or differences in real-world environments.
These case studies highlight an evolving discourse in the AI industry, where companies, regulators, and the public are re-evaluating the effectiveness of traditional benchmarks. The demand for more transparent, real-world applicable metrics is leading to a transformation in both the development and evaluation processes of AI technologies. As noted in several industry reports, these movements signal a critical turning point where economic impact and practical application take precedence over abstract scores.
Public Perception and Skepticism Surrounding AI Benchmarks
Public perception of AI benchmarks has become increasingly skeptical, as highlighted in an article from TechCrunch. This skepticism stems from the self-reported nature of these benchmarks, which often lack independent verification. Such practices raise concerns about the transparency and reliability of AI performance claims, as they could be manipulated to present favorable outcomes without reflecting actual capabilities. The public's distrust is further fueled by the limited scope of current benchmarks, which tend to focus on narrow domains rather than providing a holistic view of an AI model's utility in real-world applications [0](https://techcrunch.com/2025/02/19/this-week-in-ai-maybe-we-should-ignore-ai-benchmarks-for-now/).
The limited scope of existing AI benchmarks contributes to a disconnect between benchmark scores and practical utility, a point made clear in the discussion surrounding xAI's Grok 3 model. Despite its impressive benchmark performance, there is ongoing debate about the significance of these scores in assessing real-world applications. Critics argue that AI benchmarks should extend beyond abstract performance metrics to include factors like economic impact and real-world applicability. These concerns underscore the need for alternative methods to evaluate AI that prioritize tangible outcomes over theoretical achievements [0](https://techcrunch.com/2025/02/19/this-week-in-ai-maybe-we-should-ignore-ai-benchmarks-for-now/).
Learn to use AI like a Pro
Get the latest AI workflows to boost your productivity and business performance, delivered weekly by expert consultants. Enjoy step-by-step guides, weekly Q&A sessions, and full access to our AI workflow archive.
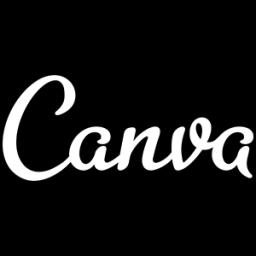
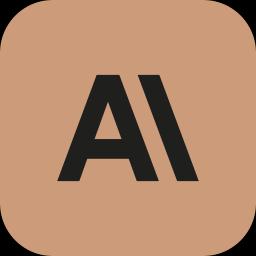
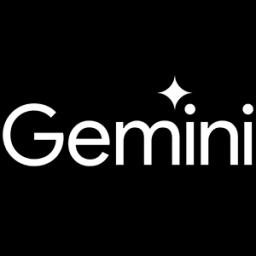
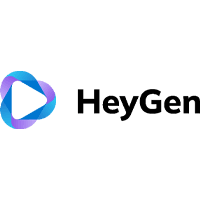
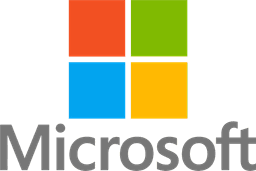
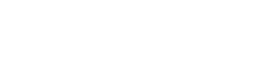
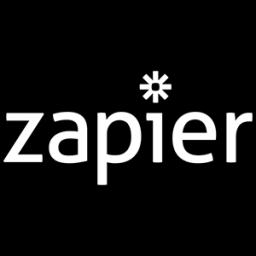
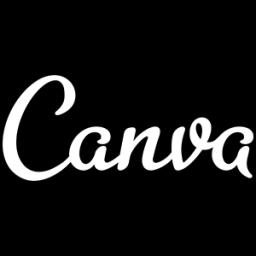
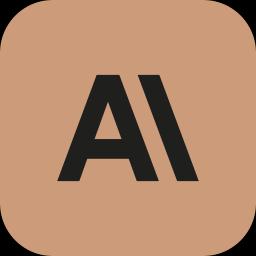
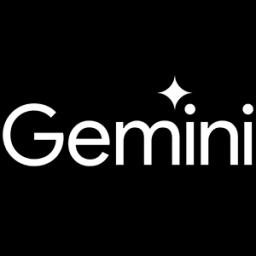
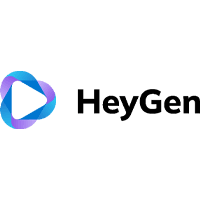
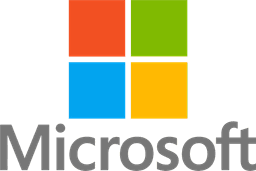
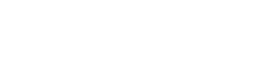
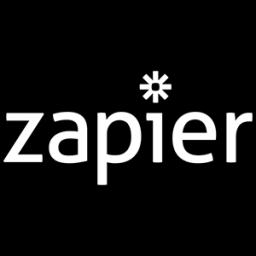
The public discourse surrounding AI benchmarks is also shaped by related controversies, such as DeepMind's Gemini 1.5 launch. The company faced backlash for selective reporting of benchmark data, which spurred discussions about the importance of full transparency in AI testing. Such incidents amplify the call for standardized benchmark suites by independent organizations, which could ensure that AI evaluation is both comprehensive and impartial. This push for transparency is aligned with evolving regulatory landscapes, like the EU AI Act, that aim to enforce rigorous testing standards and verification processes [1](https://www.theverge.com/2024/2/15/gemini-deepmind-benchmark-controversy).
The broader skepticism towards AI benchmarks is mirrored by industry experts who call for a shift in focus towards real-world efficiency and societal impacts. As noted by Wharton professor Ethan Mollick, benchmarks often resemble subjective reviews more than objective assessments. This comparison highlights the industry's struggle to establish reliable and meaningful measures of AI performance. Experts suggest aligning benchmarks with economic and practical utility measures, a change that could transform how AI systems are perceived by both the market and the society [5](https://techcrunch.com/2025/02/19/this-week-in-ai-maybe-we-should-ignore-ai-benchmarks-for-now/).
Social media platforms are abuzz with discussions on AI benchmarks, where users advocate for evaluations anchored in real-world applications. This sentiment reflects a growing movement that demands transparency and accountability from AI developers. Calls for independent verification of benchmark results are loud on these platforms, where users express their desire for benchmarks that genuinely reflect AI's potential contributions to critical sectors such as healthcare and education. Such public pressure indicates a significant shift in how AI progress and success are defined and perceived [3](https://techcrunch.com/2025/02/19/this-week-in-ai-maybe-we-should-ignore-ai-benchmarks-for-now/).
Economic Implications of Relying on Benchmarks
In an era where artificial intelligence (AI) is becoming an integral part of various industries, the reliance on benchmarks to measure AI performance has deep economic implications. These benchmarks, often self-reported and narrowly focused, fail to capture the practical utility of AI systems, leading to misguided investments and resource allocation. This was notably highlighted in a recent TechCrunch article which underscores the problematic nature of AI benchmarks and advocates for a shift towards more meaningful evaluation methods that focus on real-world impact and economic significance [TechCrunch](https://techcrunch.com/2025/02/19/this-week-in-ai-maybe-we-should-ignore-ai-benchmarks-for-now/).
The economic landscape of AI is heavily influenced by how performance metrics are perceived by investors and stakeholders. Traditional benchmarks, criticized for their lack of independent verification, often mislead stakeholders into prioritizing technologies that may not yield practical results. This misalignment can lead to inefficiencies in capital allocation, as investments are driven more by benchmark scores than by tangible benefits or return on investment (ROI). By shifting focus towards economic impact measurements and practical utility assessments, companies can ensure that resources are directed towards AI developments that deliver measurable value.
Moreover, the persistent use of benchmarks as a primary measure of AI success creates a disconnect between theoretical performance and real-world utility. This disconnect has significant economic implications, as it affects industry adoption rates and shapes public perception of AI technologies [TechCrunch](https://techcrunch.com/2025/02/19/this-week-in-ai-maybe-we-should-ignore-ai-benchmarks-for-now/). To mitigate these effects, experts call for the establishment of standardized testing and independent verification mechanisms, which would help align benchmark metrics with real-world applications and economic productivity.
Learn to use AI like a Pro
Get the latest AI workflows to boost your productivity and business performance, delivered weekly by expert consultants. Enjoy step-by-step guides, weekly Q&A sessions, and full access to our AI workflow archive.
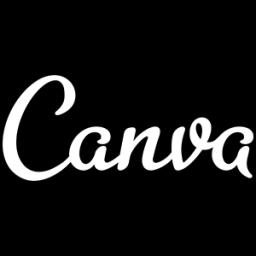
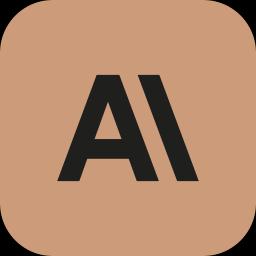
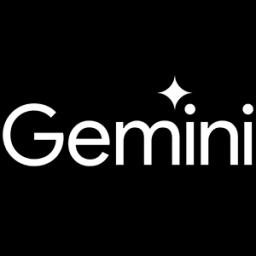
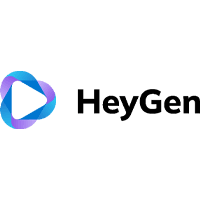
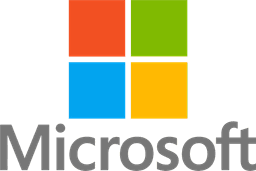
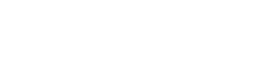
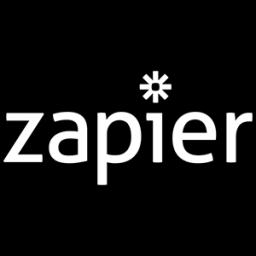
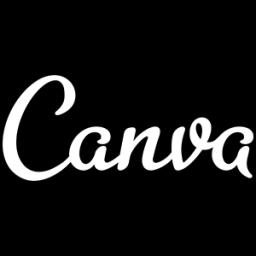
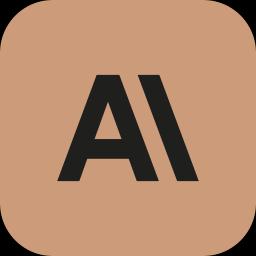
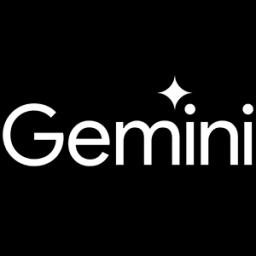
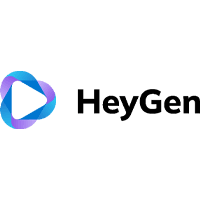
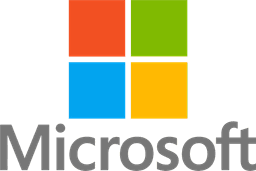
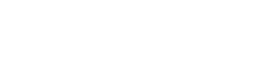
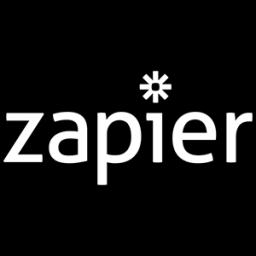
As industries continue to grapple with the complexities of AI integration, the need for alternative evaluation approaches becomes increasingly apparent. Experts emphasize the importance of aligning benchmarks with economic impacts and real-world productivity gains [Bitcoin World](https://bitcoinworld.co.in/truth-about-ai-benchmarks/). By focusing on metrics such as real-world application success, adoption rates, and economic return, companies and investors can better assess the practicality and effectiveness of AI technologies, leading to more strategic and ROI-focused investment decisions.
Social and Regulatory Shifts: A Call for Change in AI Evaluation
The landscape of artificial intelligence evaluation is undergoing crucial transformation due to myriad social and regulatory shifts. Traditional benchmarks, often used as the yardsticks for AI capability, are facing critical scrutiny. The inadequacies of these benchmarks are increasingly apparent as they tend to prioritize narrow-domain expertise and self-reported metrics, which lack independent validation as suggested in a recent article on TechCrunch. The public and expert community alike are advocating for a paradigm shift in how AI efficacy is assessed, focusing more on economic impact and practical utility rather than theoretical prowess.
Significant regulatory changes are amplifying the call for overhaul in AI performance evaluation. For instance, the European Union's introduction of the AI Act mandates standardized testing and greater transparency in benchmark reporting, thereby setting a precedent for global standards (as noted by European Digital Strategy). This regulatory pressure is driving tech companies to adopt more comprehensive and transparent testing methodologies before releasing their AI models to market.
Amidst these shifts, there emerges a growing consensus among tech innovators and academics for the establishment of independent verification bodies. Such authorities could ensure the integrity of AI performance claims, effectively mitigating the marketing hype often found in the industry. This sentiment echoes the critiques laid out by Wharton professor Ethan Mollick, who compared existing benchmarks to subjective reviews and called for objective, real-world assessments (TechCrunch).
Moreover, the industry's move towards improved evaluation methods aligns closely with ethical and social expectations. By concentrating on the genuine impacts of AI technologies in diverse environments, companies not only comply with forthcoming regulations but also address the skepticism among consumers and technologists. This sentiment is also mirrored in public forums where discussions about prioritizing real-world utility over benchmarks are frequently debated (TechCrunch).
These changes are anticipated to reshape the investment strategies within the AI sector. By shifting focus from superficial benchmark achievements to verifiable real-world outputs, investors are expected to align their evaluations with practical applications and tangible returns. This transition is reflective of a more mature and judicious market approach, as discussed in strategic insights on AI investments available from financial institutions (BNY Insights).
Learn to use AI like a Pro
Get the latest AI workflows to boost your productivity and business performance, delivered weekly by expert consultants. Enjoy step-by-step guides, weekly Q&A sessions, and full access to our AI workflow archive.
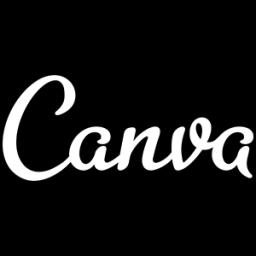
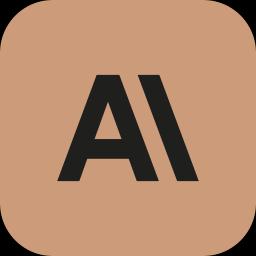
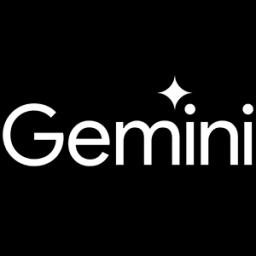
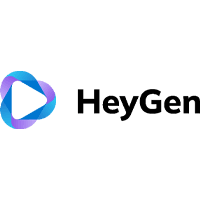
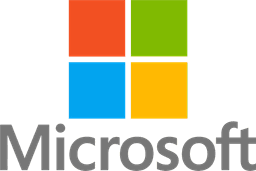
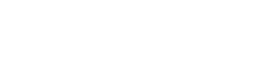
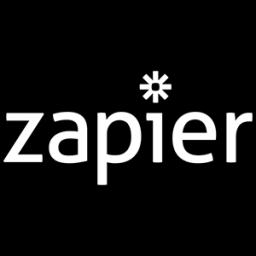
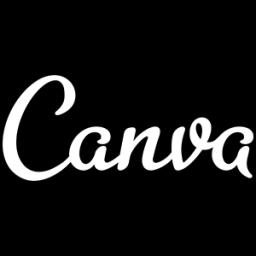
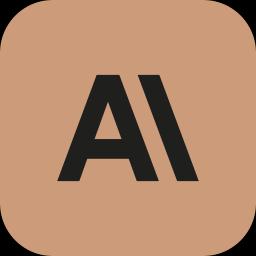
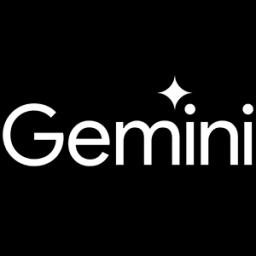
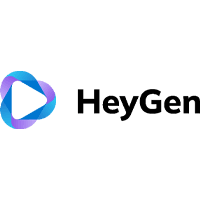
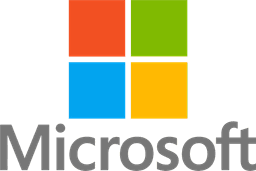
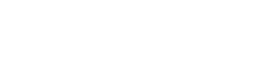
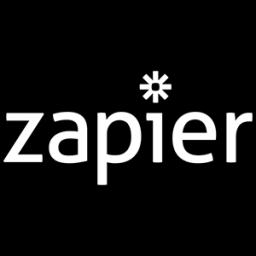
Future Prospects: Moving Beyond Benchmarks
As the AI sector ventures into new frontiers, it's becoming increasingly apparent that the reliance on traditional benchmarks needs to be reevaluated. The potential for growth and innovation is immense, but this progression requires a shift in how success is measured and what is prioritized. The current fixation on benchmark scores is criticized not only for its self-reported nature and limited scope but also for its disconnect from practical applications [0](https://techcrunch.com/2025/02/19/this-week-in-ai-maybe-we-should-ignore-ai-benchmarks-for-now/). Moving beyond these benchmarks necessitates the development of evaluation methods that are more attuned to economic and societal impacts.
Alternative approaches are gaining traction among experts who see the need for evaluation strategies that align with real-world adoption and economic impact. New models of assessment could include metrics like economic contributions and improvements in productivity rather than solely relying on traditional benchmarks which are often criticized for their inadequacies [0](https://techcrunch.com/2025/02/19/this-week-in-ai-maybe-we-should-ignore-ai-benchmarks-for-now/). Such evaluations would not only provide a clearer picture of an AI model's utility but also guide investment and innovation towards more tangible benefits.
The AI market is on the cusp of potentially revolutionary changes, particularly as leading organizations recognize the importance of real-world impact over theoretical performance measures. This shift is reflected in moves by major entities, such as the EU's new regulations requiring transparency and standardized testing, which promise to make AI development more accountable [5](https://digital-strategy.ec.europa.eu/en/ai-act-2024). The focus on independent verification and the measure of actual impact underscore a broader desire for tools and methods that can truly represent an AI model's capabilities in practical, everyday scenarios.
In response to growing concerns about the reliability of AI benchmarks, there's a collective push for new frameworks that emphasize ethical and societal consequences. This involves recalibrating AI evaluation methods to better reflect their real-world implications and contributions. By prioritizing transparency and reproducibility in AI systems' performance claims, stakeholders aim to restore confidence and focus on the technology's substantial potential for generating value [1](https://www.cmacrodev.com/ai-and-the-future-of-government-unexpected-effects-and-critical-challenges/). Such realignment not only promises more sustainable growth but also encourages more strategic industry advancements.
Conclusion: Toward Meaningful AI Evaluation Methods
As we navigate the complex landscape of artificial intelligence evaluation, it becomes evident that traditional benchmark metrics no longer suffice. These conventional methods, often touted as the measure of an AI model's capability, fail to reflect the practical utilities that end-users experience in real-world applications. Instead, experts suggest that metrics like economic impact and user adoption rates offer a more honest appraisal of a model's influence and potential. In recent discussions, the necessity for AI evaluation to transcend beyond abstract scores and align more closely with tangible results has gained traction, urging a reconsideration of how success is measured in the AI arena. This shift in focus is not only essential for accurate assessment but pivotal to fostering trust and transparency within the industry.
The ongoing discourse calls for a reevaluation of the emphasis placed on AI benchmarks, which, as highlighted by the recent performance of xAI's Grok 3 model, can often be misleading. This model's success on paper did little to quell debates about the true value of benchmark scores, emphasizing instead the allure of practical capabilities over numerical triumphs. TechCrunch has been at the forefront in advocating for alternative evaluation paradigms that prioritize real-world impact and user relevance. The argument for a pivot away from self-reported and unverifiable metrics highlights a growing consensus among experts about the necessity of robust, standardized testing mechanisms.
Learn to use AI like a Pro
Get the latest AI workflows to boost your productivity and business performance, delivered weekly by expert consultants. Enjoy step-by-step guides, weekly Q&A sessions, and full access to our AI workflow archive.
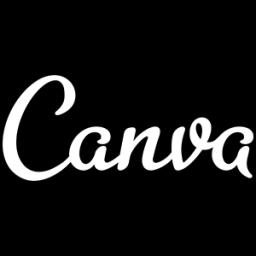
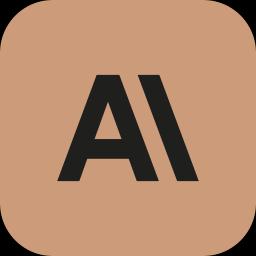
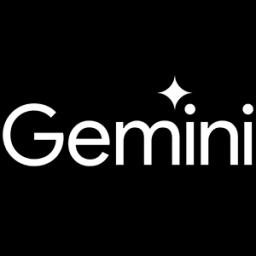
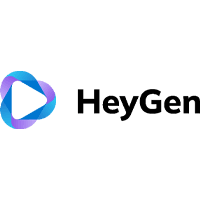
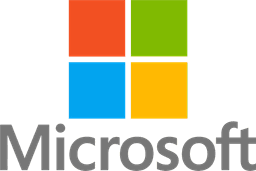
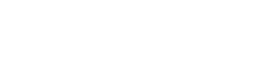
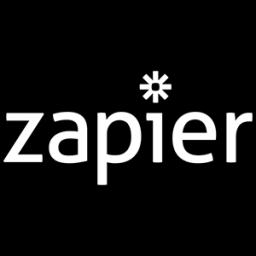
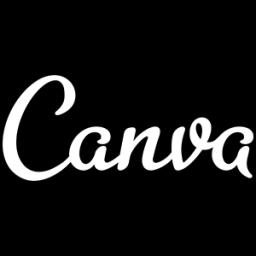
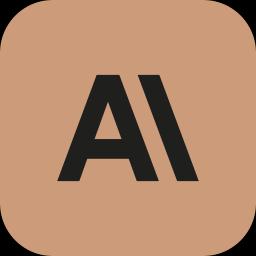
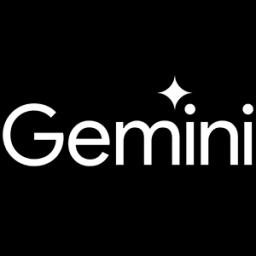
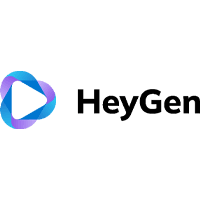
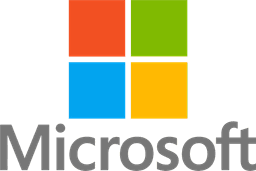
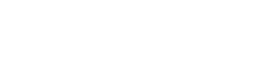
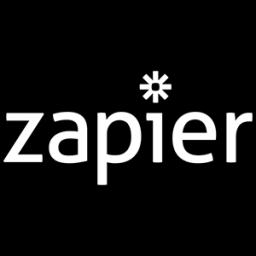
In line with this evolving perspective, the AI industry is increasingly recognizing the importance of developing alternative evaluation frameworks. These frameworks would ideally be governed by independent authorities to ensure unbiased and transparent assessments, thus mitigating the prevalent issues of self-reporting and lack of verification. Industry insiders and researchers alike propose a shift that favors marketplace applications and economic performance indicators over isolated benchmark achievements. As industries align more closely with these principles, the potential for more meaningful assessments becomes achievable, enhancing AI's role in society.
Ultimately, the movement toward redefined AI evaluation methods seeks to establish a new gold standard that prioritizes genuine performance over hollow metrics. As more stakeholders advocate for this transformation, it is imperative to embrace not just the capability, but the responsibility, of crafting tools that truly resonate with, and benefit, the broader community. This comprehensive approach not only elevates the standards of AI evaluation but ensures that technological advancements translate into real-world enhancements, benefiting society at large.