AI Meets Its Fact-Checker
AWS Launches Automated Reasoning to Curb AI Hallucinations
Last updated:

Edited By
Mackenzie Ferguson
AI Tools Researcher & Implementation Consultant
Amazon Web Services has unveiled a new service aiming to put an end to AI hallucinations by using Automated Reasoning checks. Like similar tools from Microsoft and Google, this service aims to ensure AI models stay factual and reliable. AWS also introduced features like Model Distillation and multi-agent collaboration, hoping to redefine AI efficiency and accuracy. But as AWS steps into this frontier, questions about the tools' effectiveness and AWS's lack of supporting data keep the intrigue alive.
Introduction: AWS Tackles AI Hallucinations with New Service
Amazon Web Services (AWS) recently unveiled an innovative service, Automated Reasoning checks, aimed at tackling the notorious issue of AI hallucinations—occurrences where AI systems generate incorrect or misleading responses. Similar in intent to Microsoft's "Correction" feature and Google's verification tool in Vertex AI, the unique selling point of AWS's service lies in its ability to scrutinize and verify the logical steps an AI model uses to reach its conclusions, aligning them with a predefined ground truth provided by the client. This approach not only enhances the reliability of AI outputs but also empowers users to apply bespoke rules that refine the validation process.
AWS has complemented this new feature with the introduction of Model Distillation, a capability that facilitates the transfer of knowledge from expansive and sophisticated AI models to their more compact, cost-effficient counterparts. This mirrors Microsoft's Azure AI Foundry's strategy and underscores AWS's commitment to making AI more accessible without compromising on the power and efficiency of the tools. Additionally, the rollout of a "multi-agent collaboration" feature on AWS Bedrock allows tasks to be broken down among AI agents under the oversight of a 'supervisor agent,' optimizing task management in intricate projects.
Learn to use AI like a Pro
Get the latest AI workflows to boost your productivity and business performance, delivered weekly by expert consultants. Enjoy step-by-step guides, weekly Q&A sessions, and full access to our AI workflow archive.
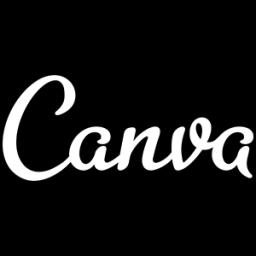
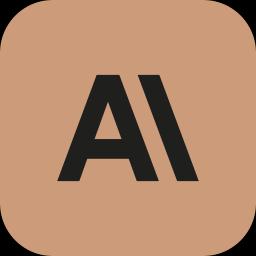
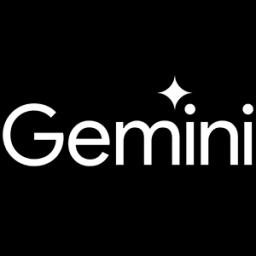
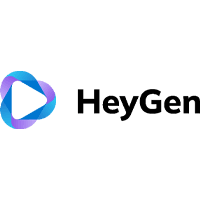
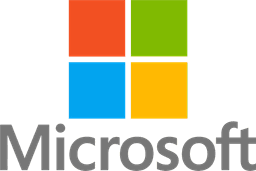
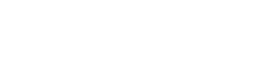
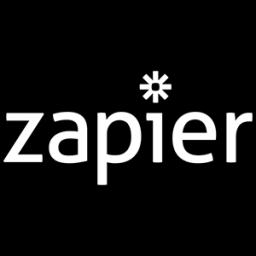
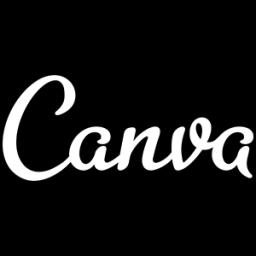
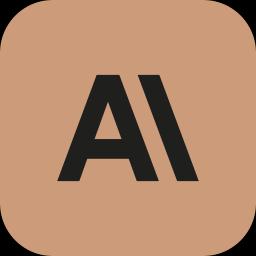
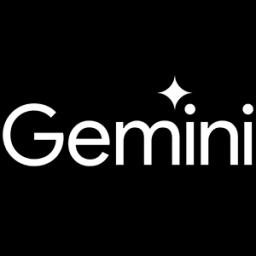
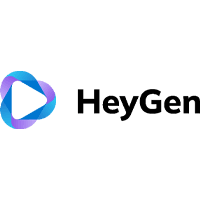
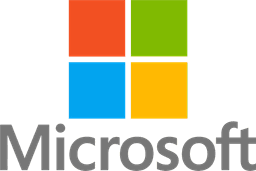
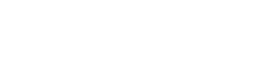
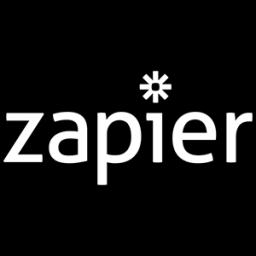
While these innovations position AWS competitively against giants like Microsoft and Google, they also invite scrutiny about their efficacy. There is keen interest in understanding whether Automated Reasoning checks can genuinely diminish AI hallucinations, given the complex nature of AI's prediction mechanisms. Critics and analysts alike are also probing the absence of direct empirical evidence substantiating AWS's claims of reliability, raising questions about the robustness and trustworthiness of these new tools in real-world applications.
The broader implications of these breakthroughs are multifaceted. Economically, advances in Model Distillation and multi-agent collaboration could significantly reduce operational costs and boost performance in real-time settings, particularly lucrative for industries heavily reliant on computational resources. Socially, if AWS's tools manage to enhance the accuracy and reliability of AI systems, they might bolster public confidence in AI-based services—a fundamental step for their integration into critical fields such as healthcare. However, given the nascent stage of these technologies and the accompanying lack of empirical validation, skepticism regarding their immediate effectiveness remains a barrier to widespread acceptance.
On the regulatory front, the emergence of such sophisticated AI safeguards is likely to prompt legislative bodies to revisit and potentially reshape the norms governing AI applications. As AWS, Microsoft, and Google continue to expand their AI capabilities, there may be an increasing push towards establishing standardized practices that ensure AI reliability and safety, influencing how future AI technologies are developed and implemented. This burgeoning interest in regulatory standards could also dictate how tech companies innovate and market their AI solutions.
Automated Reasoning Checks: An Overview
Amazon Web Services (AWS) has introduced an innovative service named Automated Reasoning checks that specifically targets the issue of AI 'hallucinations'. These hallucinations arise when AI models deliver responses that are not grounded in reality or correct information. The Automated Reasoning checks function similarly to certain features offered by other major tech companies such as Microsoft's Correction feature and Google's Vertex AI tools. The key functionality involves the service's capability to thoroughly analyze the reasoning path taken by an AI model to reach a particular decision and verify its accuracy against a provided ground truth. This capability allows stakeholders to ensure model outputs align with trusted and pre-determined truths, which can significantly enhance the reliability of AI outputs in critical applications.
Learn to use AI like a Pro
Get the latest AI workflows to boost your productivity and business performance, delivered weekly by expert consultants. Enjoy step-by-step guides, weekly Q&A sessions, and full access to our AI workflow archive.
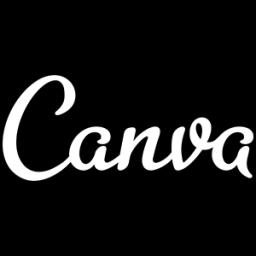
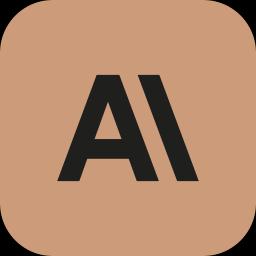
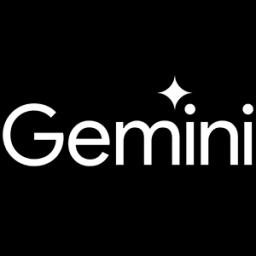
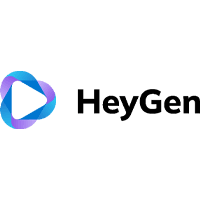
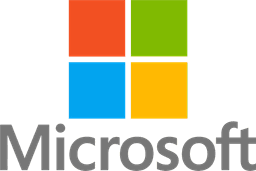
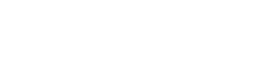
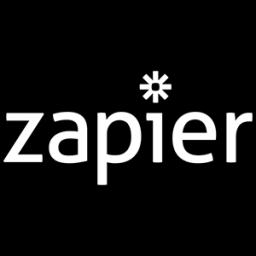
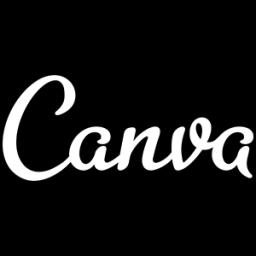
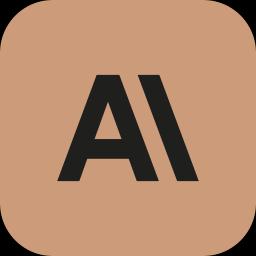
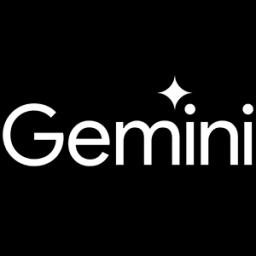
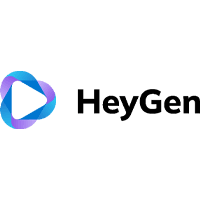
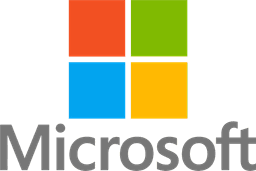
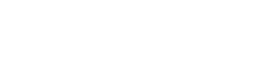
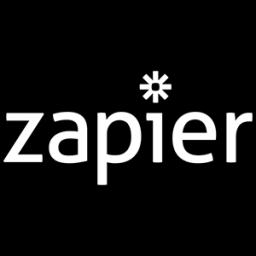
Comparisons with Microsoft and Google: A Competitive Landscape
The competitive landscape of AI technology sees a significant interplay between major players like Amazon Web Services (AWS), Microsoft, and Google as they develop solutions to enhance AI reliability and efficiency. These companies are innovating to address a common issue in AI technology known as 'hallucinations,' where AI systems generate incorrect or unreliable responses. AWS's new Automated Reasoning checks, similar to Microsoft's 'Correction' feature and Google's models in Vertex AI, aim to provide reliable AI outputs by validating responses against pre-established truths, bringing into question how these tools stack up against each other and their effectiveness in reducing inaccuracies.
AWS's introduction of Model Distillation presents a new vector in AI development, allowing for the downsizing of AI models without significantly compromising their capabilities. This innovation echoes the approaches seen in Microsoft's Azure AI Foundry. Google, meanwhile, is creating systems to ground AI responses in verified data, as exemplified by their DataGemma models. The comparison among these tech giants showcases varying methodologies toward achieving efficient and reliable AI, reflecting how these companies tailor their technologies to meet the evolving demands of the AI sector.
The multi-agent collaboration feature announced by AWS is another prominent aspect of the competitive landscape, allowing AI agents to handle specific tasks within larger workflows. This mirrors Microsoft's Magentic-One system designed for task specialization across domains. Such capabilities symbolize the cutting edge of collaborative AI systems and their potential in improving task management and execution efficiency.
However, within this competitive setting, each feature presents its own challenges. Automated Reasoning checks from AWS, while promising increased reliability, falter due to a lack of data supporting their accuracy claims. Similarly, while Model Distillation heralds cost and speed efficiency, concerns arise regarding potential accuracy loss with model scaling. These limitations highlight the balance AWS must strike between innovation and practical application to firmly establish its position in the competitive AI market.
As these companies continue to develop and enhance their AI capabilities, they not only push each other to innovate but also help define the standards of reliability and efficiency expected in advanced AI systems. This dynamic competition among AWS, Microsoft, and Google fuels advancements that offer broader implications for industries reliant on AI technology, influencing future trends and developments within the tech sector as they vie for market leadership.
Understanding Model Distillation and Its Impact
Model distillation refers to a process where a larger, complex machine learning model is used to train a smaller one. The idea is that the smaller model will acquire the essential knowledge or abilities of the larger model, allowing it to perform similar tasks with equal efficacy but using fewer computational resources.
Learn to use AI like a Pro
Get the latest AI workflows to boost your productivity and business performance, delivered weekly by expert consultants. Enjoy step-by-step guides, weekly Q&A sessions, and full access to our AI workflow archive.
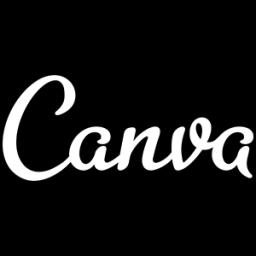
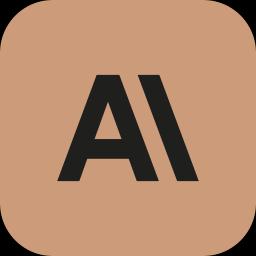
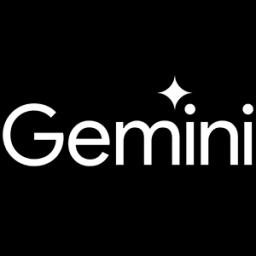
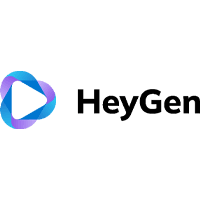
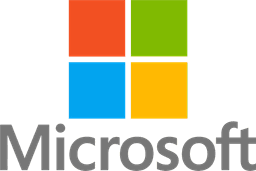
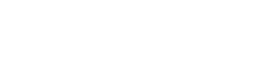
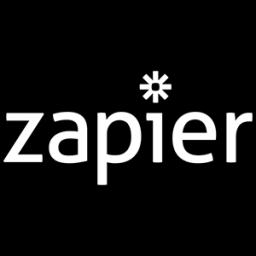
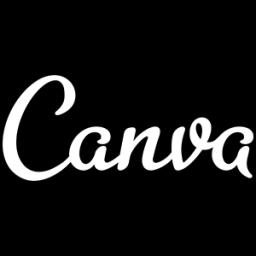
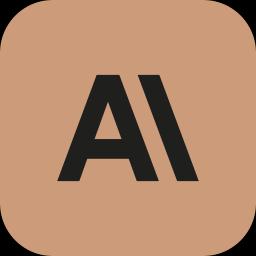
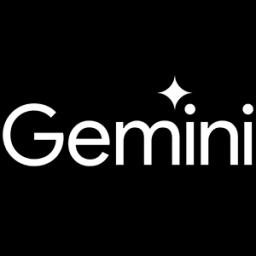
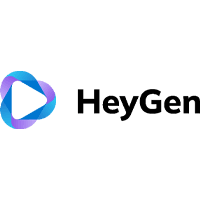
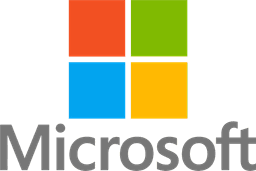
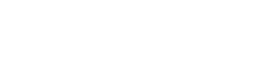
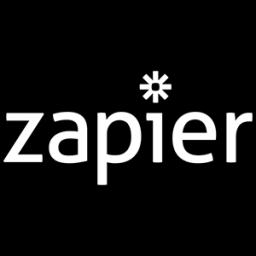
The concept has gained traction as the AI field rapidly grows and the need for efficient deployment of AI models becomes more pronounced. Large AI models are computationally expensive and often impractical for real-world applications, especially when those applications require real-time processing or must function on hardware with limited capabilities.
Model distillation not only makes AI applications more accessible by reducing their resource requirements but also reduces costs associated with deploying these models at scale. This is particularly beneficial for companies looking to implement AI solutions without investing heavily in expensive infrastructure.
Despite its advantages, model distillation comes with challenges. One significant issue is the potential loss of accuracy or capabilities when transferring knowledge from a larger model to a smaller one. This trade-off between model size and performance can sometimes limit the applicability of distilled models.
Compatibility is another concern, as not all knowledge from a large model can be easily or effectively transferred to a smaller model. This could result in limitations where only certain types or aspects of data are well-suited for distillation.
Multi-Agent Collaboration: A New Frontier in AI Task Management
In the rapidly evolving landscape of artificial intelligence, the concept of multi-agent collaboration is gaining momentum as an innovative approach to task management. This method involves the assignment of multiple AI agents, each tasked with handling specific subtasks within a larger project, under the supervision of a central coordinator often referred to as a 'supervisor agent.' This collaborative framework enables more efficient task execution, reduces redundancies, and enhances the overall effectiveness of AI systems in complex processes.
The application of multi-agent collaboration extends to various industries, where the division of labor among AI agents can lead to significant productivity gains. For instance, in sectors like finance, healthcare, and supply chain management, this approach can streamline operations by allowing agents to specialize in tasks that align with their capabilities. This specialization not only optimizes resources but also ensures a higher degree of accuracy and reliability in task outcomes, as agents can focus on functions tailored to their designed strengths.
Learn to use AI like a Pro
Get the latest AI workflows to boost your productivity and business performance, delivered weekly by expert consultants. Enjoy step-by-step guides, weekly Q&A sessions, and full access to our AI workflow archive.
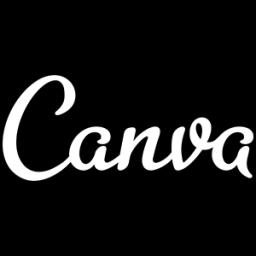
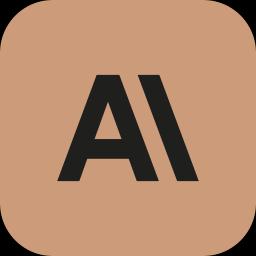
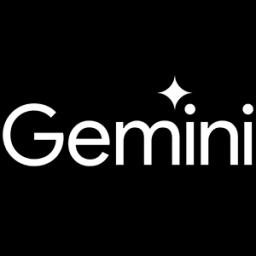
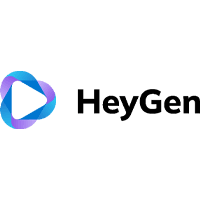
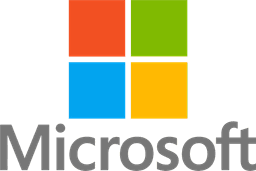
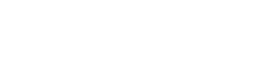
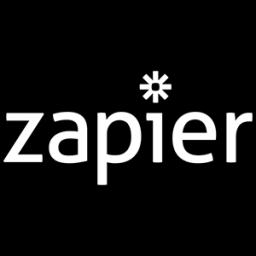
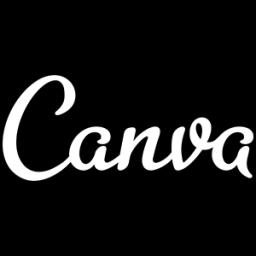
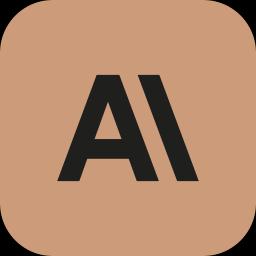
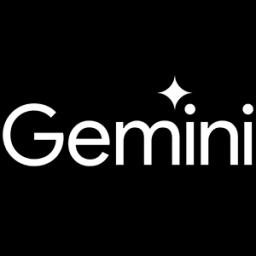
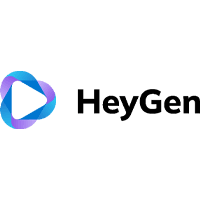
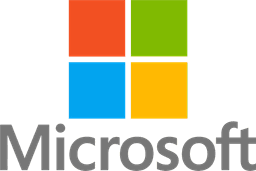
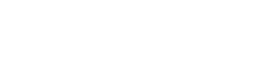
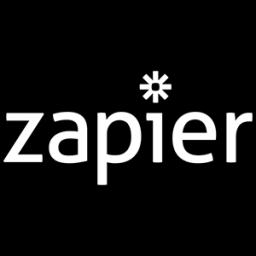
Moreover, multi-agent collaboration facilitates scalability in AI applications. As businesses grow and their operational demands become more complex, the ability to assign and reassign tasks among AI agents helps maintain efficiency and performance. This adaptability is crucial in dynamic environments where rapid changes can affect project timelines and resource allocation. By deploying a network of specialized agents, organizations can quickly adjust to new requirements, ensuring continuity and competitiveness.
Nevertheless, the implementation of multi-agent systems comes with its challenges. Managing the complexity of interactions between agents, data privacy concerns, and integration into existing IT infrastructure are key issues that need to be addressed. Effective coordination mechanisms are required to prevent conflicts between agents and ensure that tasks are executed seamlessly without duplication of effort. Organizations must also consider the security aspects of allowing multiple agents to access and process sensitive information, necessitating robust data protection measures.
The future of multi-agent collaboration in AI task management looks promising, with advancements in technology continually improving the capability and efficiency of such systems. As AI continues to integrate deeper into various sectors, the need for sophisticated task management techniques will rise. Organizations adopting multi-agent systems can expect not only operational efficiencies but also the opportunity to innovate new solutions tailored to their specific needs, potentially reshaping industry standards and practices.
Expert Insights: Praises and Pitfalls of AWS's AI Innovations
Amazon Web Services (AWS) has recently introduced a new AI service called Automated Reasoning checks, aimed at reducing the occurrence of AI "hallucinations." These hallucinations occur when AI models produce incorrect or misleading responses. Automated Reasoning checks work by analyzing the AI model's thought process and verifying it against a predefined standard of truth established by the client. In essence, it checks how a model arrives at a particular conclusion and the logic behind its answers, thereby ensuring they align with established facts. This service is seen as a competitor to Microsoft's Correction feature and Google's tool in Vertex AI, both designed to improve the reliability of AI-generated content.
AWS's Model Distillation is another innovative feature that facilitates the transfer of capabilities from larger AI models to smaller, more cost-effective, and efficient models. This technique is similar to Microsoft's approach in Azure AI Foundry. By distilling a model, AWS aims to maintain accuracy while reducing computational requirements, thus enabling broader accessibility and affordability of AI technologies. However, there are concerns regarding potential incompatibilities and loss of accuracy in the distilled models, which might limit widespread adoption.
A significant advancement is the introduction of multi-agent collaboration within AWS's Bedrock platform. This feature allows different AI agents to work together on segmented tasks within a broader project, managing these interactions through a centralized "supervisor agent." By enabling AI specialization in distinct tasks, it increases overall system efficiency and productivity. While the potential for complex task facilitation is evident, challenges such as operational complexity and data management could impede seamless implementation, especially in industries where data security and control are paramount.
Learn to use AI like a Pro
Get the latest AI workflows to boost your productivity and business performance, delivered weekly by expert consultants. Enjoy step-by-step guides, weekly Q&A sessions, and full access to our AI workflow archive.
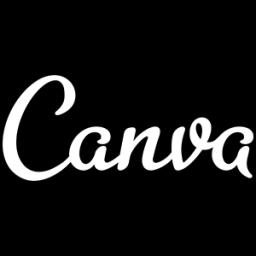
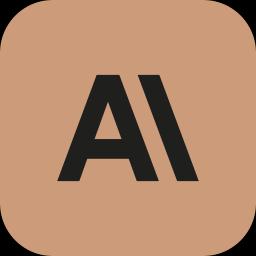
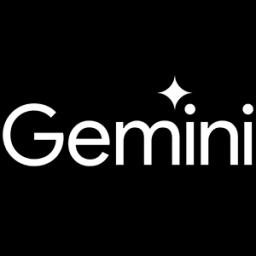
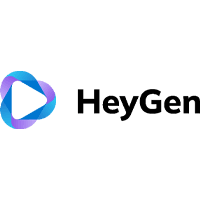
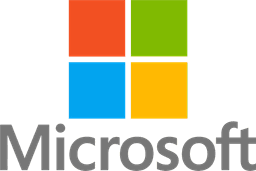
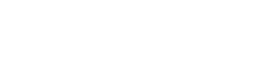
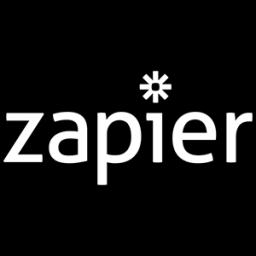
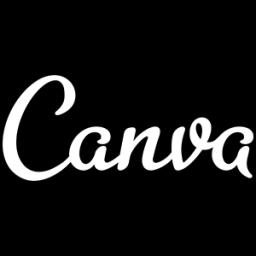
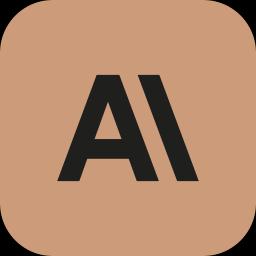
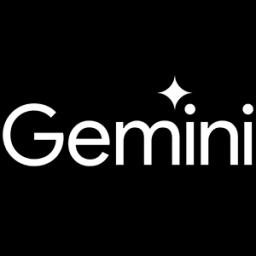
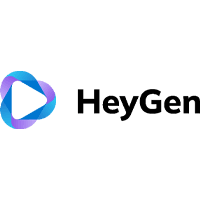
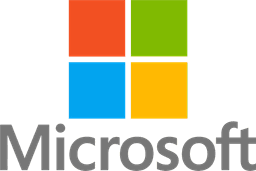
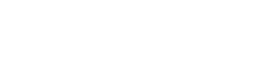
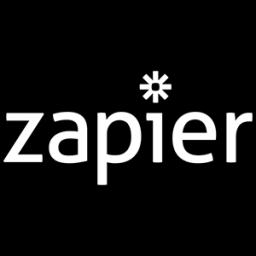
The introduction of these revolutionary tools by AWS raises pertinent questions about their practicality and effectiveness in solving prevalent AI challenges. Automated Reasoning checks are designed to increase output reliability, particularly benefiting sectors requiring stringent verification processes like healthcare and finance. But, experts argue about its uniqueness compared to existing solutions from Google and Microsoft, given the absence of quantitative data supporting AWS's reliability claims.
Model Distillation offers significant economic benefits by reducing operational costs and improving the speed of real-time applications. It is notably praised in financial sectors for optimizing resource usage while enhancing performance metrics. Nevertheless, the risk of reduced accuracy in distilled models remains a concern, potentially affecting the quality and applicability across diverse domains where precision is critical.
The public's reception of AWS's announcements is not well-documented due to insufficient data from social platforms. Typical reactions to such technological advancements often include a mix of enthusiasm for new efficiencies and skepticism about the ability to fully address AI challenges like hallucinations. Accurate public sentiment can be more fully understood through analyses of responses on platforms like Twitter, Reddit, or relevant tech forums.
Looking ahead, AWS's AI innovations may significantly impact various sectors. Economically, cost reductions and improved performance could provide AWS with a competitive advantage in the rapidly growing AI industry. Socially, as these tools become more reliable, public trust in critical applications might increase, particularly in sensitive sectors such as healthcare. Politically, the growing reliance on AI could lead to stricter regulatory oversight to ensure these technologies are used responsibly, prompting tech giants like AWS to align their products with global standards in AI reliability and safety.
Public Reactions: Enthusiasm and Skepticism
Amazon Web Services (AWS) recently unveiled a service known as Automated Reasoning checks, targeted at mitigating AI 'hallucinations'—instances when AI models produce unreliable results. The service scrutinizes the reasoning behind AI outputs, comparing them with a customer-provided ground truth to verify correctness, akin to strategies from Microsoft and Google. While Automated Reasoning checks potentially improve the dependability of AI models, questions arise regarding their efficacy, particularly without supporting data from AWS to validate these claims. Public sentiment appears mixed, with excitement over potential improvements tempered by skepticism about the effectiveness of these solutions.
Alongside Automated Reasoning checks, AWS introduced Model Distillation, a function to streamline the transfer of capabilities from larger models to smaller, more efficient ones. This innovation promises to decrease operational costs and enhance efficiency, aligning with Microsoft’s methods in Azure AI Foundry. Despite financial experts praising model distillation for potential economic benefits, concerns about loss of accuracy and compatibility issues persist, which may influence its broader adoption. Tech enthusiasts and critics alike show a keen interest in seeing real-world data and applications that demonstrate the tangible benefits of both features amidst these challenges.
Learn to use AI like a Pro
Get the latest AI workflows to boost your productivity and business performance, delivered weekly by expert consultants. Enjoy step-by-step guides, weekly Q&A sessions, and full access to our AI workflow archive.
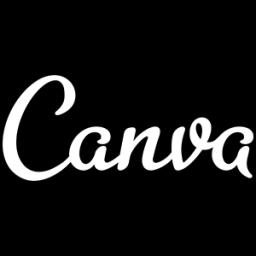
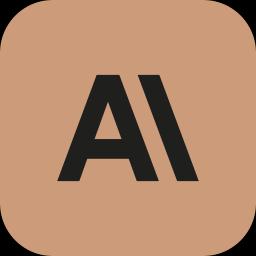
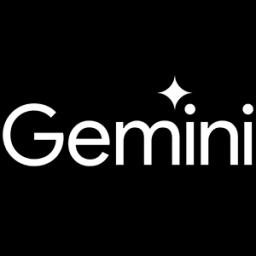
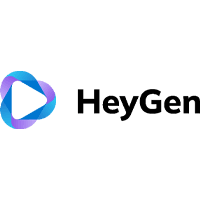
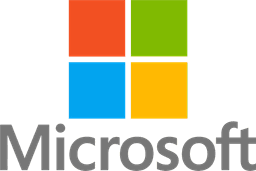
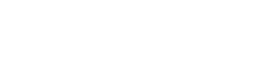
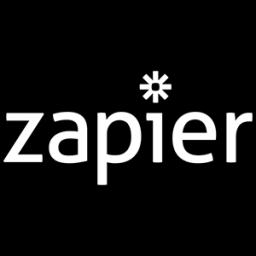
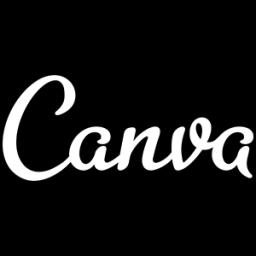
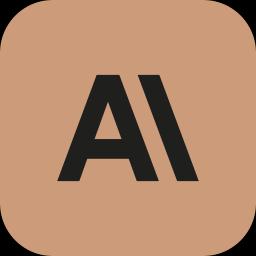
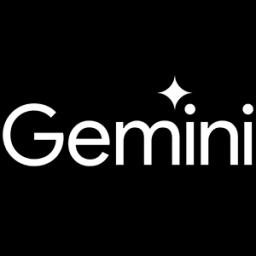
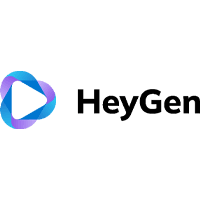
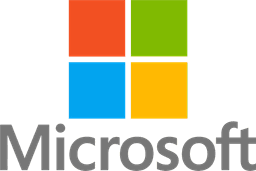
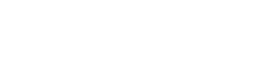
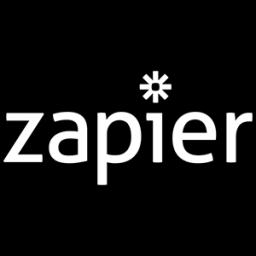
Multi-agent collaboration in AWS's Bedrock feature represents another significant shift, offering the ability to allocate specific AI agents to tasks, governed by a supervisory agent. It aims to innovate task management across complex systems, thereby augmenting productivity. Practical applications in sectors like finance illustrate its promise but also highlight the intricate balance required in maintaining data integrity and system efficiency. While experts recognize this leap forward, they remain cautiously optimistic, underscoring the importance of overcoming data control and complexity management issues to ensure successful implementation.
General public reactions are diverse yet speculative due to a dearth of direct responses on platforms like Twitter or Reddit. Typically, technology enthusiasts exhibit enthusiasm for these advancements, highlighting potential efficiency gains, while skeptics question the realistic efficacy of completely eliminating AI hallucinations. There's acknowledgment that examining concrete user experiences and discourse from community-driven forums will be essential to understanding the true impact of these new AWS tools. The dual perspective underscores the importance of nuanced, evidence-backed discourse in the evolving field of AI.
The introduction of these AI services by AWS signifies potentially transformative effects economically, socially, and politically. Economically, if model distillation and multi-agent collaboration succeed, they promise to significantly cut costs while enhancing real-time processing capacities, granting AWS a competitive advantage within the AI industry.
Socially, the efforts to build trust through AI verification mechanisms may reassure users, especially in critical fields such as healthcare, thereby fostering greater public acceptance. Yet, the absence of empirical data revealing the actual effectiveness of these solutions may hinder trust and the pace of adoption. Politically, as AI's role pervades decision-making processes, regulatory scrutiny intensifies, necessitating standardization of AI reliability and ethics. This landscape compels tech firms like AWS to align innovations with emerging regulations to sustain growth and acceptance across various sectors.
Economic and Social Implications of AWS's AI Advancements
AWS's recent advancements in AI innovations hold profound implications both economically and socially. The introduction of 'Automated Reasoning checks' is set to change the landscape by potentially reducing AI 'hallucinations'. This new service allows for the cross-verification of AI model outputs with an established ground truth, which could significantly enhance the reliability of AI in critical sectors like finance and healthcare. While this holds promise for increased trust in AI-generated data, the challenge remains as AWS has yet to provide empirical evidence of the system's effectiveness.
Economic ramifications of these innovations are substantial, particularly regarding cost efficiency. Model Distillation allows knowledge transfer from larger to smaller models, which is projected to cut computational costs. This is not just about saving expenses, but also about increasing the speed and applicability of AI, crucial for industries relying on rapid data processing. Additionally, multi-agent collaboration could elevate task efficiency, particularly in complex operational tasks, albeit with some concerns over complexity management and data control.
Learn to use AI like a Pro
Get the latest AI workflows to boost your productivity and business performance, delivered weekly by expert consultants. Enjoy step-by-step guides, weekly Q&A sessions, and full access to our AI workflow archive.
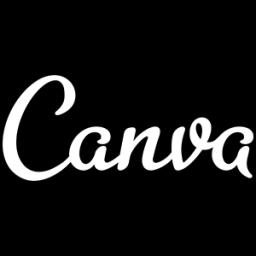
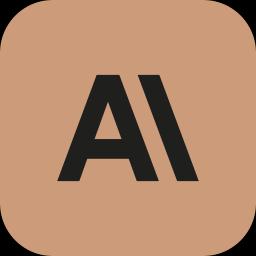
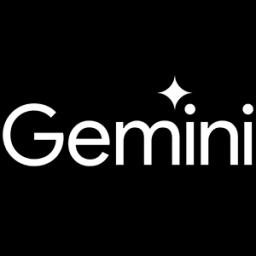
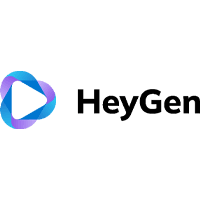
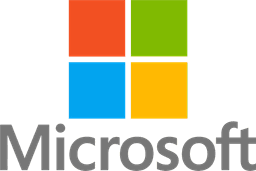
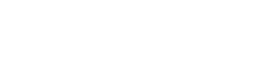
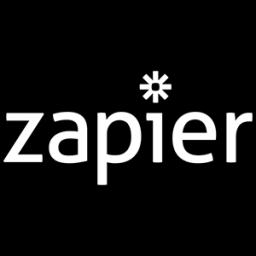
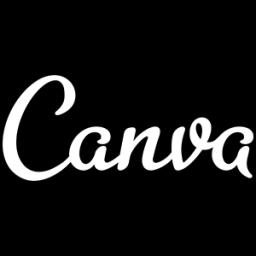
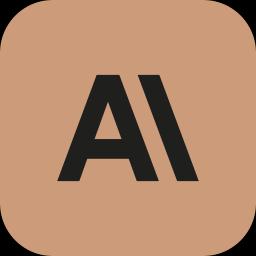
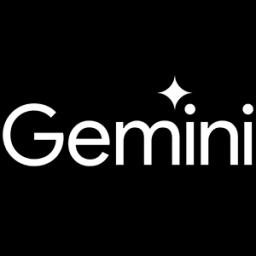
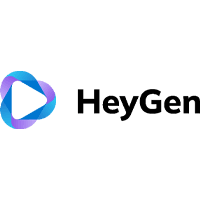
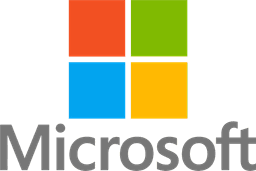
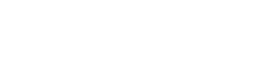
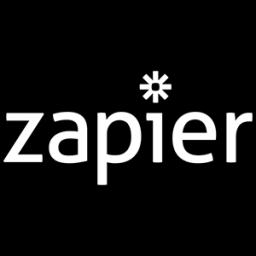
Socially, the integration of these technologies could reshape public perception and reliance on AI systems. By providing verifiable, accurate AI outputs, AWS aims to mitigate public skepticism, nurturing trust especially in sensitive areas like healthcare, where accuracy and reliability are paramount. However, without public validation of AWS's Automated Reasoning system's claims, skepticism might persist among users who are wary of over-relying on AI for critical decision-making.
The influence of such technological advancements may also resonate politically, as they push regulatory bodies to rethink and possibly reframe the oversight on AI decision-making uses. The convergence of similar safeguards from big players like AWS, Microsoft, and Google may pressure these institutions to establish standardized norms for AI reliability, which in turn could dictate future paths for AI development and deployment strategies.
Public reactions, though not explicitly documented, are expected to range from enthusiasm to skepticism. The excitement stems from enhancements in AI efficiency and reduction in operational challenges. Nevertheless, there is an underlying layer of cautious optimism, especially when considering the historical challenges associated with fully curbing AI hallucinations.
Future Regulatory Changes: The Political Repercussions
The introduction of AWS's Automated Reasoning checks represents a critical step toward addressing the problem of AI hallucinations, which has become a significant concern given the AI models' increasing integration into various sectors. By comparing model outputs with a customer-provided ground truth, AWS aims to enhance the reliability of AI-generated responses. This initiative mirrors similar efforts by Microsoft and Google, showcasing an industry-wide push towards improving the veracity of AI systems. However, questions remain about the absolute effectiveness of these tools, as the nature of AI prediction inherently involves uncertainty and potential inaccuracies.
Additionally, Amazon's Model Distillation feature seeks to optimize AI operations by facilitating the transfer of capabilities from larger models to smaller, more cost-effective ones. This approach not only aims to cut down operational costs but also improves the efficiency of AI systems without significantly compromising accuracy. However, the challenge of maintaining model compatibility and the potential for reduced accuracy in distilled models could pose obstacles for widespread implementation. Notably, AWS's ability to demonstrate empirical evidence for the reliability of these features will be essential to address skeptics' concerns and promote broader adoption.
The potential of the 'multi-agent collaboration' feature introduced by AWS is significant, particularly in improving task management within complex projects. This development allows for the specialization of AI agents in specific subtasks, thus enhancing workflow efficiency under the guidance of a supervisor agent. However, managing the complexity of such systems and ensuring robust data control presents challenges that need addressing to maximize their utility across diverse industry applications. As AWS progresses with these integrations, clear data demonstrating their efficacy will be crucial to gaining user trust and driving adoption.
Learn to use AI like a Pro
Get the latest AI workflows to boost your productivity and business performance, delivered weekly by expert consultants. Enjoy step-by-step guides, weekly Q&A sessions, and full access to our AI workflow archive.
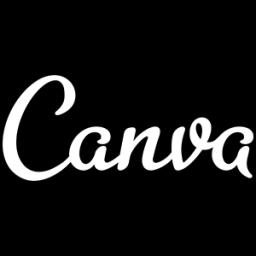
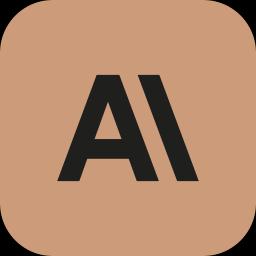
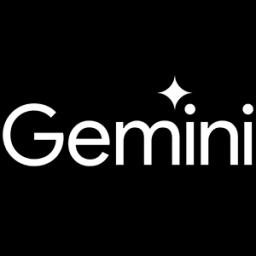
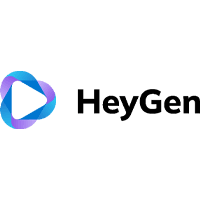
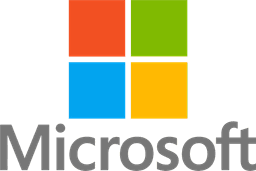
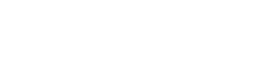
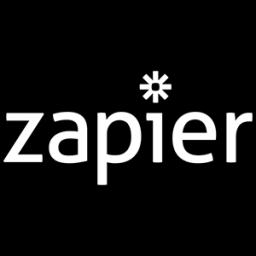
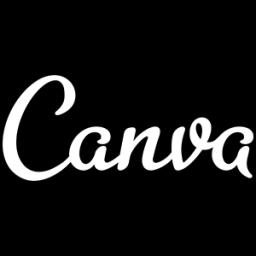
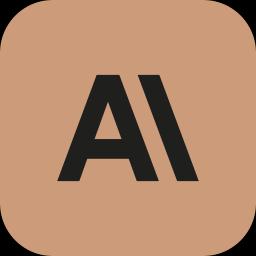
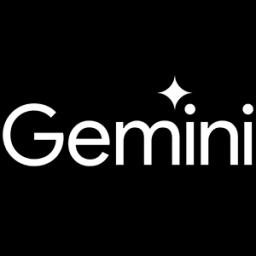
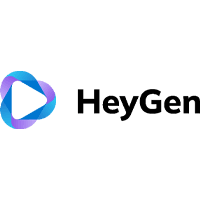
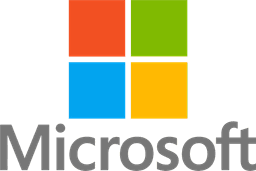
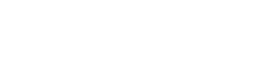
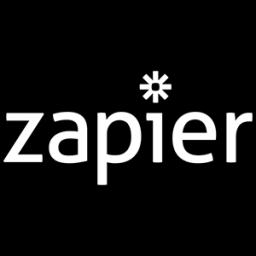
With these technological enhancements, regulatory implications loom large. As AI tools become more pivotal in decision-making processes, there is a foreseeable push from regulatory bodies to implement standardized frameworks ensuring AI's reliability and transparency. This is underscored by the competitive landscape where companies like AWS, Microsoft, and Google are continuously unveiling AI safeguards. Future regulations could significantly influence how these organizations develop and deploy AI solutions, potentially establishing new industry norms that ensure both innovation and accountability.
The political repercussions of these advancements are profound. Growing reliance on AI and its potential to streamline various sectors can lead to both opportunities and challenges. On one hand, improved efficiency and cost reductions can bolster economic growth and enhance service delivery, offering competitive advantages to early adopters. On the other hand, it raises concerns regarding data privacy, security, and the ethical use of AI, demanding careful consideration from policymakers. As these innovations unfold, a balance between technological progress and regulation will be paramount to harness their full potential while safeguarding public interests.