AI Controversy Unveiled
Benchmark Battle: xAI's Grok 3 Model Under Fire in Accuracy Dispute
Last updated:

Edited By
Mackenzie Ferguson
AI Tools Researcher & Implementation Consultant
xAI faces backlash over claims about Grok 3's performance on the AIME 2025 math benchmark, as critics point out the omission of the crucial 'consensus@64' metric in comparisons with OpenAI.
Introduction to xAI's Grok 3 Benchmark Controversy
The AI industry is currently embroiled in a debate over xAI's Grok 3 benchmark claims, especially concerning its performance on the AIME 2025 math benchmark in relation to OpenAI's models. The controversy stems from xAI's decision to leave out the 'consensus@64' metric in comparison charts, a move OpenAI argues misrepresents the true competitive landscape. The 'consensus@64' metric allows models to attempt problems multiple times and choose the most common answer, significantly boosting accuracy on complex tasks. xAI's decision to exclude this metric has been criticized as a way to artificially inflate Grok 3's apparent superiority. This incident is more than just a technical detail, as it raises broader questions about transparency in the AI benchmarking process. The industry lacks standardized reporting protocols, and this controversy highlights the urgent need for comprehensive metrics that include computational costs and a detailed assessment of real-world applications.
Significance of the Consensus@64 Metric
The Consensus@64 metric plays a crucial role in the landscape of AI model evaluations, particularly in the assessment of complex mathematical tasks. By allowing models to attempt equations 64 times and to select the most common answer, this metric can significantly enhance accuracy. Its strategic inclusion ensures a level playing field that accounts for the variance in model outputs, which can be particularly pronounced with challenging problems that have nuanced solutions. The omission of Consensus@64 in the assessment of xAI’s Grok 3 model versus OpenAI’s offerings has sparked debate about the veracity of reported performance metrics. Critics, including experts from Stanford and the AI Benchmarking Institute, argue that without this metric, performance comparisons are incomplete and potentially misleading, skewing the perception of a model's capabilities .
Learn to use AI like a Pro
Get the latest AI workflows to boost your productivity and business performance, delivered weekly by expert consultants. Enjoy step-by-step guides, weekly Q&A sessions, and full access to our AI workflow archive.
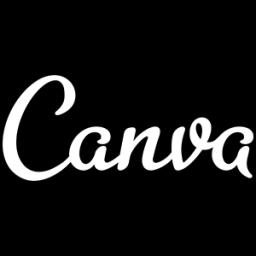
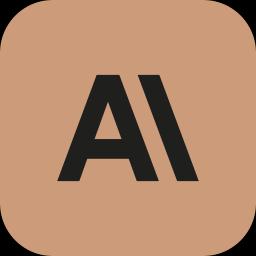
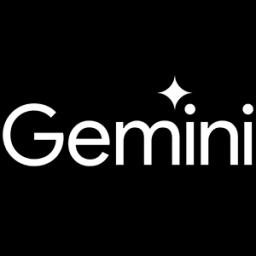
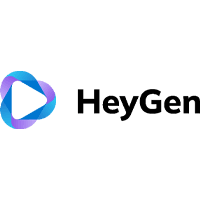
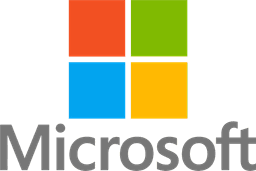
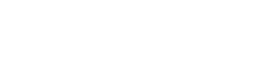
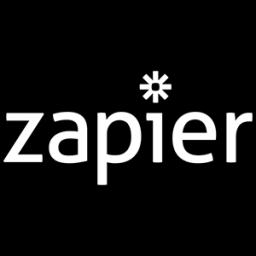
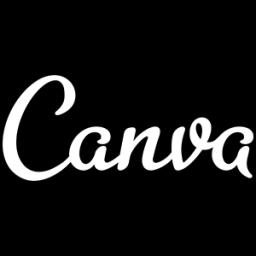
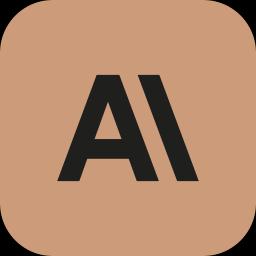
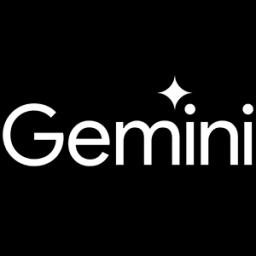
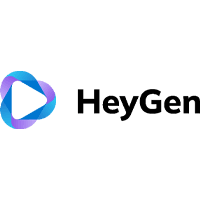
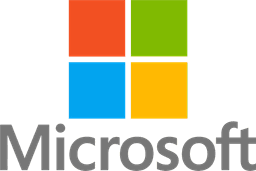
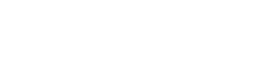
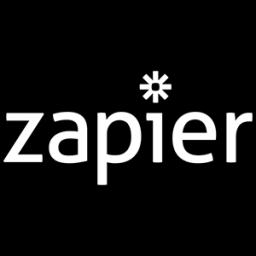
The significance of the Consensus@64 metric is underscored by its ability to capture the real-world potential of AI models in solving mathematical problems. By permitting 64 attempts, this metric provides a more thorough analysis of a model's aptitude, allowing for the correction of outlier mistakes and an enhancement in predictive accuracy. In the context of AI benchmarking, such detailed scrutiny is vital given the competitive nature of AI development. Ommitting Consensus@64 not only risks inflating the perceived superiority of certain models but also highlights broader transparency issues in AI benchmarking. As Dr. Sarah Chen from Stanford pointed out, the lack of standardized reporting in model evaluations could lead to a distortion of capabilities, which might mislead stakeholders relying on these assessments for decision-making .
Furthermore, the controversy surrounding the Consensus@64 metric reveals critical insights into AI industry practices. The selective presentation of data, as seen with xAI's performance claims, emphasizes the need for standardization in benchmarking protocols. As the AI industry grapples with the consequences of such controversies, industry leaders are advocating for comprehensive evaluation frameworks that integrate Consensus@64. Establishing universal standards may not only improve transparency but also enhance the reliability of AI models when deployed in critical real-world applications. This shift could further result in a collective movement towards ethical and transparent AI development, boosted by regulatory efforts for mandatory independent verification of AI performance claims .
xAI's Response to Criticism
In addressing the criticisms surrounding Grok 3's performance claims, xAI has adopted a defensive position, emphasizing that selective benchmark showcasing is not an uncommon practice in the industry. Co-founder Igor Babushkin highlighted that other companies, such as OpenAI, have similar histories of selective metric usage in their comparative analyses, often omitting results that might show their models in an unfavorable light. This response suggests that xAI sees the benchmarking dispute not as a unique misstep but as part of broader industry norms, where the intent is to present models in the best possible light, even if it means omitting less favorable data [source](https://www.techi.com/xai-grok3-benchmarks-accuracy-dispute/).
However, this defense does not address the core of the criticism, which centers on transparency and completeness in reporting AI performance metrics. The specific omission of the 'consensus@64' metric, a significant indicator of model performance in mathematical benchmarks like AIME 2025, has been perceived as a deliberate attempt to inflate Grok 3's competitive standing. The metric allows models to achieve higher accuracy by selecting the most common answer over multiple attempts, a capability that can significantly skew performance perceptions when omitted. Babushkin's remarks have sparked further debate over whether industry practices should change to include more comprehensive evaluations rather than focusing on selective performance highlights [source](https://www.techi.com/xai-grok3-benchmarks-accuracy-dispute/).
Learn to use AI like a Pro
Get the latest AI workflows to boost your productivity and business performance, delivered weekly by expert consultants. Enjoy step-by-step guides, weekly Q&A sessions, and full access to our AI workflow archive.
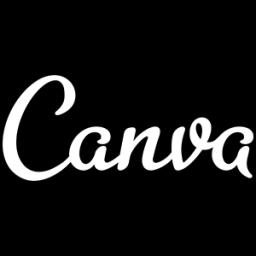
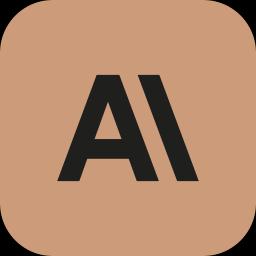
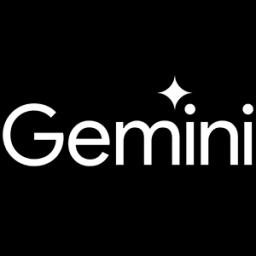
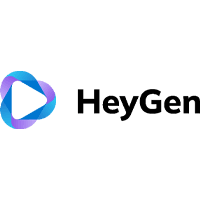
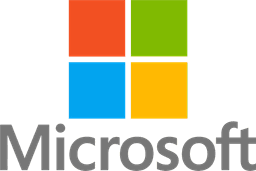
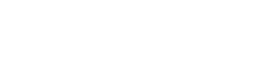
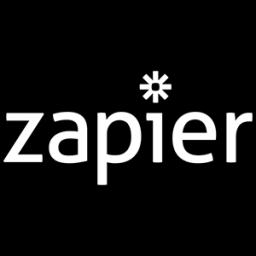
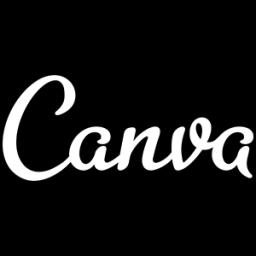
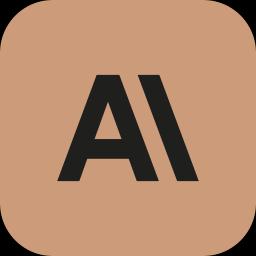
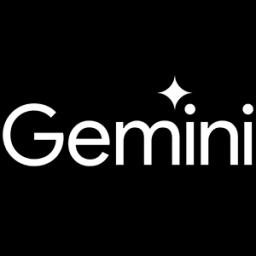
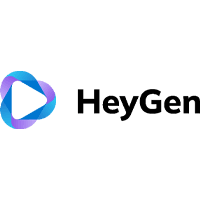
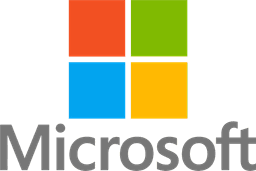
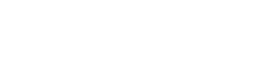
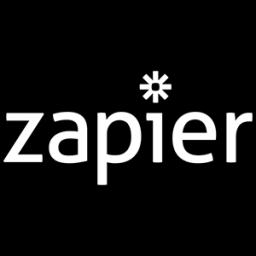
AIME 2025: Evaluating AI with Human Benchmarks
In the ever-evolving landscape of artificial intelligence, the AIME 2025 benchmark has emerged as a pivotal evaluation tool, stirring both intrigue and controversy among experts and enthusiasts alike. As an advanced mathematics competition benchmark, AIME 2025 is designed to test AI models against high-level math problems, offering insights into their reasoning and problem-solving capabilities. However, the benchmark's relevance has been questioned by some researchers who argue that it lacks the breadth required to comprehensively assess AI's real-world potential. Despite these criticisms, AIME 2025 remains influential, serving as a reference point for comparing different AI models and their mathematical prowess. The ongoing debate over its utility underscores a broader conversation about the need for diverse and holistic evaluation metrics in AI.
The recent controversy surrounding xAI's Grok 3 model and its performance claims on the AIME 2025 benchmark has brought to light crucial issues within the AI industry. One key point of contention is the omission of the "consensus@64" metric, which allows models multiple attempts to solve a problem and select the most frequent answer. OpenAI has critiqued xAI for not including this metric, arguing that it skews the perceived effectiveness of Grok 3 when compared to other models, particularly their own. The omission could misrepresent Grok 3’s actual ability to solve complex math tasks, emphasizing the necessity for transparent and comprehensive benchmarking practices. This incident highlights the competitive pressures within the AI field that sometimes lead to compromised reporting of performance metrics.
xAI's handling of the Grok 3 benchmark results has not gone without defense or context. Co-founder Igor Babushkin pointed out that selective presentation of benchmark data is not uncommon in the tech industry, citing instances where OpenAI has employed similar strategies. While some observers might find such practices misleading, others argue that they reflect a broader lack of standardized protocols in AI benchmarking. This absence of universal benchmarks and reporting standards complicates the landscape, fostering an environment where companies might choose selective narratives to bolster their standing in the competitive AI market. As the call for transparency and standardization in AI reporting grows louder, the Grok 3 controversy remains a focal point for ongoing discussions about ethical practices in AI model evaluation.
Beyond outlining the deficiencies in current AI evaluation methodologies, the Grok 3 controversy underscores the broader implications of benchmarking disputes in the AI sector. Public reactions have been polarized, with users on platforms like X (formerly Twitter) lambasting xAI for their perceived lack of transparency and advocating for more robust, standardized testing protocols. These debates have crystallized the demands of AI skeptics and reform advocates for ethical guidelines and comprehensive benchmarking practices. By shining a light on these issues, the Grok 3 incident has catalyzed discussions about the importance of accurate, transparent, and meaningful performance evaluations, all of which are essential for maintaining public trust and fostering innovation in AI technologies.
The fallout from the Grok 3 benchmark dispute may act as a catalyst for significant shifts in industry practices and regulatory frameworks. Analysts suggest that the scrutiny xAI faces could lead investors to demand greater transparency and verification of AI performance claims, shifting investment toward companies that prioritize and demonstrate rigorous, independently verified benchmarks. Additionally, there is a growing call for regulatory bodies to enforce stricter oversight, which might include standardized methodologies that consider factors beyond mere performance metrics, such as computational efficiency and bias concerns. As discussions around these issues evolve, the AI community is poised for potentially transformative changes that could define the future landscape of artificial intelligence evaluation and regulation.
Broader Industry Issues Uncovered
The unveiling of broader industry issues in the wake of the xAI Grok 3 benchmark controversy underscores significant challenges within the AI sector. One prominent concern is the absence of standardized benchmarking protocols, which has fueled inconsistent performance claims and comparisons. This lack of uniformity allows companies to selectively highlight favorable metrics, such as omitting the consensus@64 metric, which can skew perceptions of a model's capabilities . As AI models become more advanced, the need for transparent and fair benchmarking methods becomes increasingly critical, ensuring that performance data is both accurate and comprehensible.
Learn to use AI like a Pro
Get the latest AI workflows to boost your productivity and business performance, delivered weekly by expert consultants. Enjoy step-by-step guides, weekly Q&A sessions, and full access to our AI workflow archive.
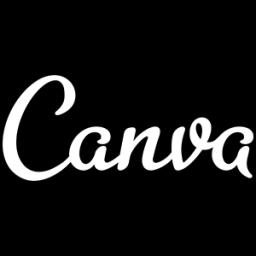
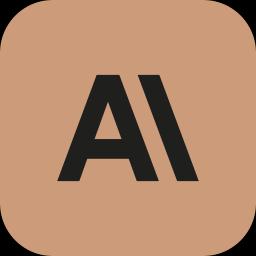
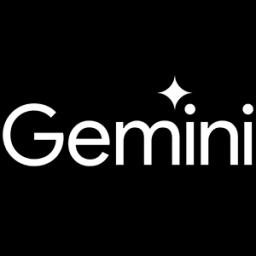
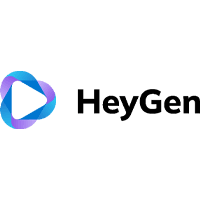
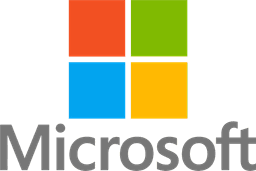
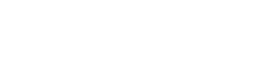
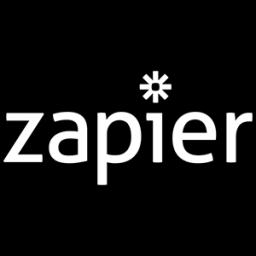
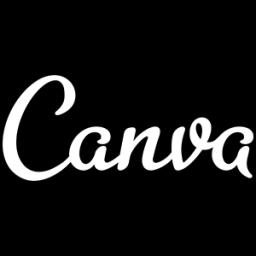
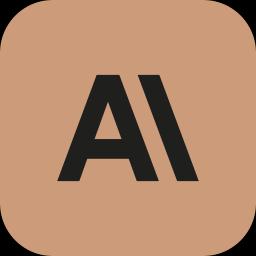
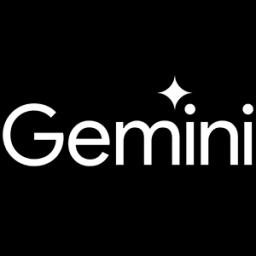
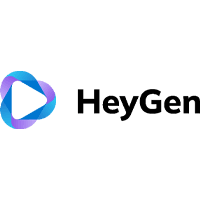
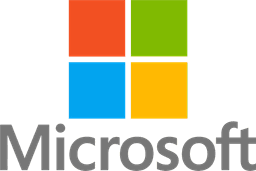
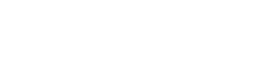
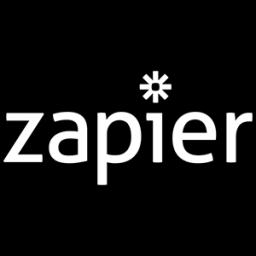
The necessity for transparency in AI performance metrics is part of a broader call for ethical practices in the technology industry. The selective presentation of benchmarks, illustrated by xAI's approach, highlights a competitive environment where companies might feel pressured to compromise on ethical standards to maintain an edge in the market . This issue not only impacts individual companies but also the industry’s reputation as a whole, potentially eroding public trust in AI technologies. Therefore, developing universal benchmarking standards, as suggested by industry experts, could foster a more honest and reliable landscape in AI development.
Another critical issue unveiled through the controversy is the overwhelming emphasis on performance metrics without adequate consideration of computational costs and real-world application measures. AI models are typically evaluated based on their ability to score high on specific tasks, such as the AIME 2025 math benchmark, yet this narrow focus can lead to the oversight of practical efficiency and application within real-world scenarios . To address these gaps, experts advocate for the inclusion of comprehensive evaluation metrics that assess models not only on accuracy but also on computational resource utilization and practical utility. This approach could ensure a more holistic assessment of AI technologies.
The competitive landscape of the AI industry intensifies the need for regulated benchmarking practices. As companies like xAI and OpenAI compete for dominance, the temptation to embellish performance results can lead to misleading claims and diminish the value of AI innovations . Addressing this issue involves not just setting new standards but also ensuring adherence through independent verification and transparency. By implementing these changes, the industry can move towards a future where AI advancements are accurately represented and confidently adopted by a wary public.
In response to controversies like the one surrounding xAI's Grok 3, the AI industry faces mounting pressure to reform its benchmarking methodologies. Initiatives such as the AI Transparency Initiative reflect growing momentum towards establishing universal testing protocols and reporting standards . By prioritizing openness and independent validation, these efforts aim to create an environment where AI capabilities are portrayed truthfully, ultimately aiding in the ethical advancement of AI technologies.
What's Missing from Current AI Evaluations?
AI evaluations often lack transparency and standardized criteria, leading to disputes like the one surrounding xAI's Grok 3 model. A key issue is the absence of metrics that reflect real-world application capabilities, such as the 'consensus@64' metric. This metric, which allows AI models to solve problems by selecting the most frequent answer from multiple attempts, significantly influences performance perception. Its omission in xAI's evaluations suggests a skewed advantage of Grok 3 over competitors like OpenAI's models, as reported by sources discussing the xAI controversy [here](https://www.techi.com/xai-grok3-benchmarks-accuracy-dispute/).
Current AI benchmarking protocols often ignore computational costs and practicality in favor of raw performance metrics. This oversight results in benchmarks that do not fully reflect a model's efficiency and applicability in diverse situations. Calls for standardized testing protocols are growing, particularly after incidents where selective data presentation has misled stakeholders about an AI model's capabilities, such as in the xAI Grok 3 case [here](https://www.techi.com/xai-grok3-benchmarks-accuracy-dispute/).
Learn to use AI like a Pro
Get the latest AI workflows to boost your productivity and business performance, delivered weekly by expert consultants. Enjoy step-by-step guides, weekly Q&A sessions, and full access to our AI workflow archive.
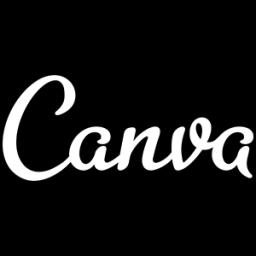
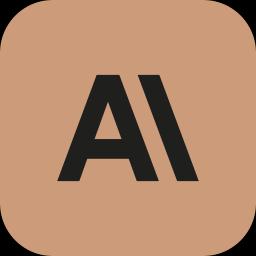
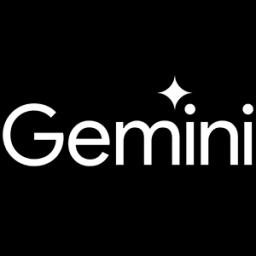
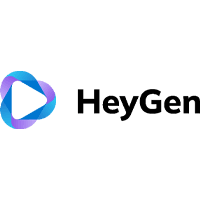
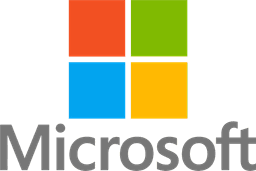
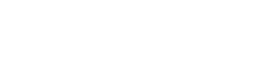
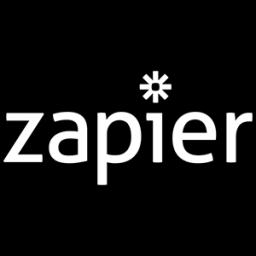
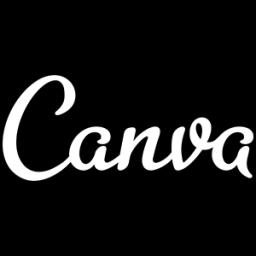
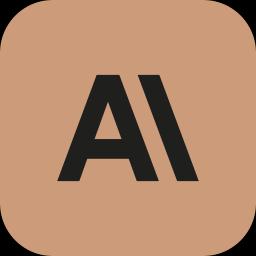
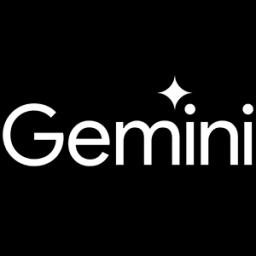
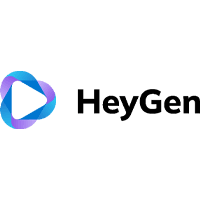
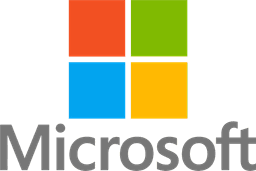
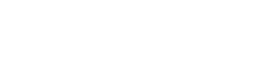
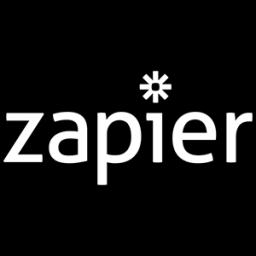
Further complicating AI evaluations is the limited scope of widely-used benchmarks like AIME 2025. These benchmarks, while influential, often cater to specific domains, such as high-level mathematics, without providing insight into a model's broader, real-world capabilities. Critics argue for the need for industry-wide evaluation standards that could offer a more accurate assessment of AI models across different domains, echoing concerns from industry experts [here](https://www.techi.com/xai-grok3-benchmarks-accuracy-dispute/).
Related Events in the AI Benchmarking Landscape
The landscape of AI benchmarking has witnessed a series of high-profile events that reflect ongoing challenges and shifts in the field. The controversy surrounding xAI's Grok 3 model and its benchmarking claims has become a pivotal moment in illustrating the complexities of AI performance evaluation. This event is part of a broader trend, as seen in the Gemimi Ultra Benchmark Controversy involving DeepMind, where similar accusations of selective reporting surfaced, highlighting discrepancies in claimed versus actual performance [1](https://ai-watch.org/deepmind-gemini-ultra-benchmark-dispute). As these controversies unfold, they expose fundamental issues within the industry regarding the lack of standardized benchmarking protocols and the need for transparency in performance metrics.
In response to these challenges, major tech companies have taken significant steps to address the benchmarking issues. In January 2025, the launch of the AI Transparency Initiative saw companies like Google, Meta, and Anthropic come together to form the AI Benchmarking Standards Coalition. This coalition aims to establish universal testing protocols and reporting requirements to ensure clarity and consistency in evaluating AI model capabilities [3](https://tech-standard.org/ai-transparency-initiative). Their efforts reflect a broader industry recognition of the importance of transparency and the establishment of ethical guidelines in AI development.
Globally, AI models from Chinese tech giants such as Baidu and ByteDance have faced intense scrutiny regarding their claimed performance metrics. Independent verifications have revealed discrepancies between reported and actual capabilities, indicating a wider issue of overstatement in AI claims [5](https://asia-ai-news.com/chinese-ai-models-verification). Meanwhile, regulatory discussions in Europe, exemplified by the EU AI Act Implementation Debates, are focusing on the need for transparency and mandatory third-party verification of AI benchmarks [7](https://eu-tech-policy.com/ai-act-benchmarks). Such regulations are poised to create a more accountable environment for AI performance declarations.
The debate around xAI's Grok 3 has not only raised questions about specific claims but has also catalyzed broader industry movements toward reform. By emphasizing the importance of ethical benchmarking and accurate reporting, industry leaders and regulatory bodies alike are pushing for a transformation in how AI technologies are assessed and communicated to the public. These changes are critical, as they aim to enhance investor confidence and maintain public trust in AI technologies. The ongoing discussions underscore a critical juncture for the AI field, where the pursuit of standardized and reliable benchmarks may define the future trajectory of AI development [3](https://www.techi.com/xai-grok3-benchmarks-accuracy-dispute).
Expert Opinions on xAI's Benchmark Practices
Experts in artificial intelligence are expressing serious concerns about the benchmark practices employed by xAI in promoting their Grok 3 model. Dr. Sarah Chen, an AI Ethics Researcher at Stanford, has particularly criticized the selective presentation of benchmark data by xAI. In the comparisons released by xAI, the omission of the 'consensus@64' metric, which significantly impacts performance accuracy on complex mathematical tasks, has raised red flags. According to her, xAI's decision to omit this metric skews the comparison with OpenAI's models, as Grok 3 tends to underperform on first-attempt scores, commonly known as @1 scores, when compared with competitors like OpenAI's models. Chen stresses that such practices can mislead stakeholders into believing in superior performance that does not hold up under comprehensive scrutiny. More detailed information on this issue can be found [here](https://techcrunch.com/2025/02/22/did-xai-lie-about-grok-3s-benchmarks/).
Learn to use AI like a Pro
Get the latest AI workflows to boost your productivity and business performance, delivered weekly by expert consultants. Enjoy step-by-step guides, weekly Q&A sessions, and full access to our AI workflow archive.
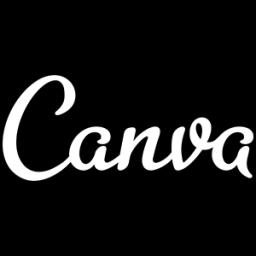
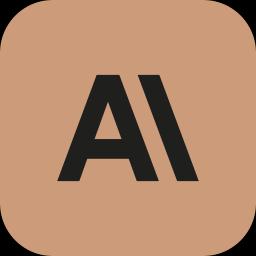
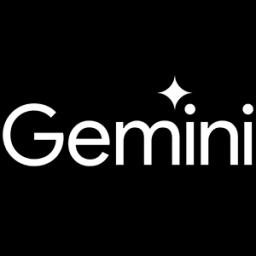
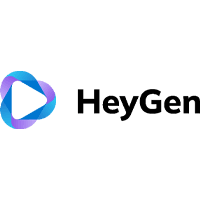
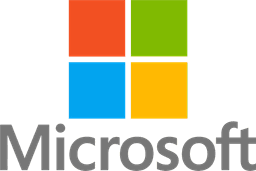
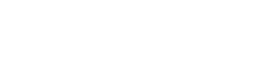
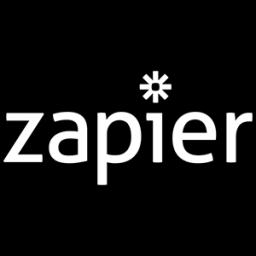
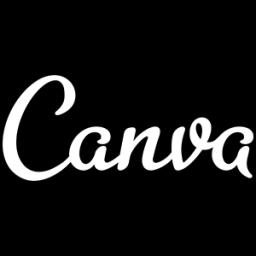
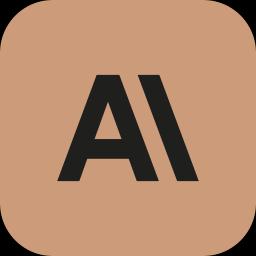
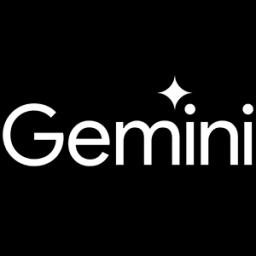
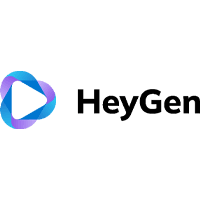
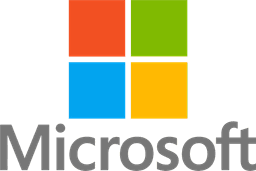
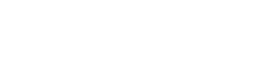
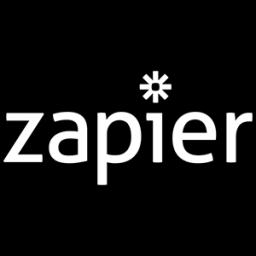
Dr. Marcus Thompson, Director of the AI Benchmarking Institute, has voiced concerns regarding the very framework used for AI benchmarking, like the AIME 2025. As an academic examination predominantly designed for human cognitive assessments, he argues that its application in AI evaluations is inherently flawed. Thompson calls for the development and adoption of AI-specific evaluation metrics that can better assess the true capabilities and limitations of AI models. This perspective is especially vital given the ongoing debate about appropriate measures for assessing AI performance realistically in diverse scenarios, emphasized further in discussions [here](https://techcrunch.com/2025/02/22/did-xai-lie-about-grok-3s-benchmarks/).
Overall, the critical outlook shared by AI experts reflects a wider industry need for standardized and transparent benchmarking practices. The scrutiny surrounding xAI’s Grok 3 illustrates broader issues of selective data presentation and metric omission, which have recurring implications across the AI landscape. Experts highlight the urgency for consensus on universal benchmarking standards, encouraging the industry's participation in collaborative efforts to develop frameworks that ensure transparency, fairness, and accountability in AI performance evaluations. This move is seen as increasingly necessary to maintain trust and realism in AI advancements, a topic further explored in related articles [here](https://www.techi.com/xai-grok3-benchmarks-accuracy-dispute/).
Public Reactions to the Grok 3 Controversy
The public reactions to the Grok 3 controversy have been intense and widespread, particularly on social media platforms. Three main camps have emerged amid the debate. AI benchmark skeptics are increasingly vocal, expressing distrust in current AI benchmarking practices and calling for more standardized and transparent testing protocols. Critics, especially on platforms like X (formerly Twitter), have lambasted xAI for what they view as "deliberately misleading" data presentations, demanding greater accountability and transparency from AI companies [1](https://medium.com/@bytefulsunday/xai-lying-about-grok-3-performance-while-openai-exposes-chinese-surveillance-network-fba04a3dff1a).
As expected, xAI has its defenders. Some supporters argue that xAI's presentation of data is in line with standard industry methodologies and emphasize the fact that selective benchmark presentations are a norm across the sector. They also pointed to similar precedent cases where competing companies have employed analogous strategies to evidence their claims [2](https://www.newsbytesapp.com/news/science/openai-employee-accuses-xai-of-fudging-ai-benchmark-results/story). Much of this support also points to alternative graphs and validations as further proof of Grok 3's competitive performance output [8](https://saipien.org/grok-3-benchmark-battle-scrutinizing-inflated-metrics-to-reveal-true-ai-impact/).
Industry reform advocates form another significant group in this conversation, engaging in dialogues that emphasize establishing ethical guidelines and more rigorous protocols for AI marketing and reporting. These advocates focus discussions towards the flaws perceived in benchmarks like the AIME 2025, urging for alterations that could prevent misrepresentation in AI evaluations [6](https://coinstats.app/news/5d434216138bd83f87f424aca2369c3f6170ed51dfdb593e0d28202156e11323_Shocking-Claims-Did-xAI-Mislead-the-World-with-Grok-3-AI-Benchmarks/). Technical forums have been especially critical, discussing the foundational issues with AIME 2025's efficacy as a metric [5](https://ca.finance.yahoo.com/news/did-xai-lie-grok-3s-225514063.html).
The controversy surrounding Grok 3 has spurred broader debates not only about AI model transparency but also about the broader ethical implications of AI benchmarking and development [8](https://saipien.org/grok-3-benchmark-battle-scrutinizing-inflated-metrics-to-reveal-true-ai-impact/). As these discussions continue, they underline the importance of establishing universal AI performance standards, which might require collaboration between technology companies, academic researchers, and regulators to ensure both transparency and integrity in this rapidly evolving field.
Learn to use AI like a Pro
Get the latest AI workflows to boost your productivity and business performance, delivered weekly by expert consultants. Enjoy step-by-step guides, weekly Q&A sessions, and full access to our AI workflow archive.
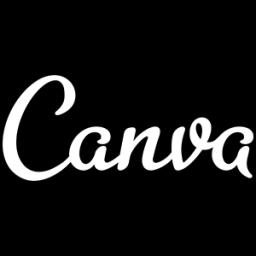
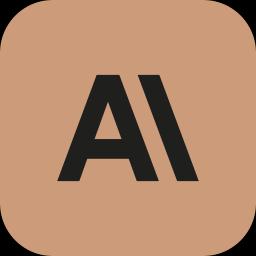
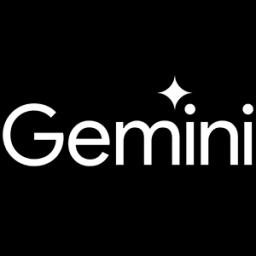
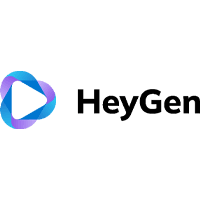
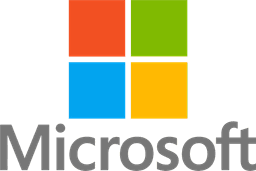
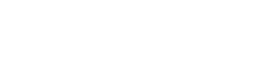
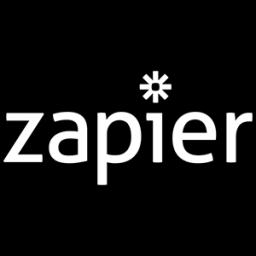
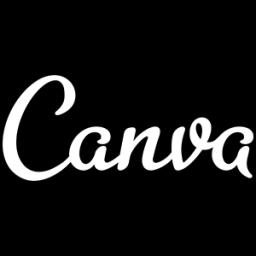
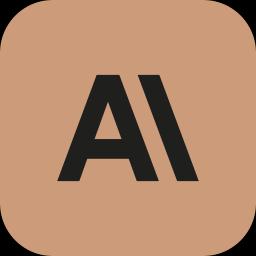
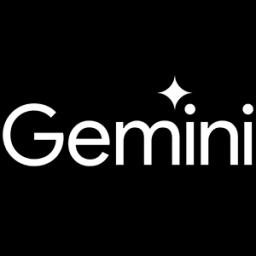
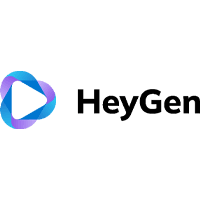
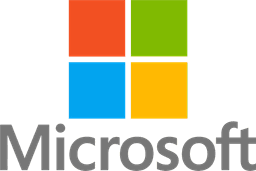
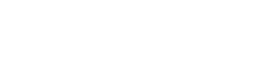
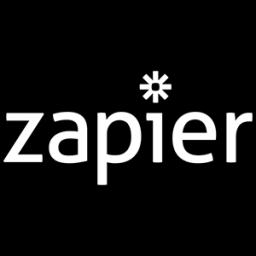
Future Implications for the AI Industry
The ongoing dispute over xAI's Grok 3 benchmark results has cast a spotlight on future developments within the AI industry, particularly in the realm of benchmark transparency and standardization. As the demand for high-level accountability in AI capabilities grows, the industry must confront its reliance on selective presentation, as seen in controversies like the Grok 3 incident. The scrutiny this case has brought will likely lead to significant reforms in how benchmarks are reported and verified. Investors, wary of the accuracy of company claims, might start diverting resources to entities that prioritize comprehensive and unbiased performance metrics, thereby reshaping funding patterns across the sector.
A key takeaway from the Grok 3 controversy is the pressing need for the AI industry to adopt more uniform benchmarking practices. Such a move would address not only public doubt but also the community's internal call for ethical standards in marketing and assessment of AI models. The implementation of these practices could force AI developers to adhere to stricter standards in both design and disclosure of AI capabilities, ensuring that publicly released performance data is as accurate and representative as possible.
Furthermore, heightened regulatory oversight is expected to emerge in response to this controversy. Regulatory bodies, recognizing the potential for misleading benchmark claims to erode public trust, may implement mandatory standards for performance reporting. This could include requirements for independent verification and comprehensive evaluations that account for computational costs and biases. Such stringent measures would not only protect stakeholders but also foster a healthier, more transparent AI environment.
The dialogue spurred by the Grok 3 benchmark dispute has galvanized calls for international collaboration among AI researchers, developers, and regulatory agencies to establish universal benchmarking standards. These efforts aim to produce a sophisticated framework that accurately evaluates AI model capabilities, transcending the limitations of current benchmarks like AIME 2025. This push towards a global standard highlights the industry's shift towards responsibility and precision, potentially ushering in an era of technological introspection and innovation growth.
As these changes take shape, the industry may witness a shift in AI implementation, with increased focus on showcasing truthful capabilities that withstand rigorous, independent scrutiny. This adjustment could further influence AI adoption rates across diverse sectors, restoring some degree of public confidence in innovative technologies. Ultimately, this evolution could redefine productivity benchmarks, transparency, and trust in AI capabilities, paving the way for a new era of advancement grounded in ethical and transparent research practices.