AI Whiz Tackling Complex Equations
DeepMind's AlphaEvolve Surpasses Expectations in Solving Math and Science Problems
Last updated:

Edited By
Mackenzie Ferguson
AI Tools Researcher & Implementation Consultant
DeepMind's latest AI marvel, AlphaEvolve, is proving itself as a master in math and science problem-solving by leveraging Gemini models to generate, critique, and evaluate its own solutions. Though it currently focuses on numerical problems, it has already optimized Google's data centers and sped up Gemini model training. The AI community eagerly awaits its early access academic program.
DeepMind's AlphaEvolve: Revolutionizing Math and Science Problem Solving
DeepMind's innovation, AlphaEvolve, represents a significant leap forward in the realm of artificial intelligence, particularly in solving complex mathematical and scientific problems. This state-of-the-art AI system leverages the power of Gemini models to not only generate potential solutions but also to critique and refine them autonomously. By processing and evaluating its solutions, AlphaEvolve minimizes inaccuracies, akin to performing a self-audit, which greatly reduces the risk of 'hallucinations' or erroneous results. This meticulous approach ensures that its outputs are both accurate and reliable, a critical factor when applying AI to fields where precision is paramount, such as scientific research and advanced mathematics. You can read more about this breakthrough on TechCrunch.
While AlphaEvolve's capabilities might sound like science fiction, its real-world applications have shown tangible benefits, particularly for Google. One of its key achievements has been the optimization of Google's data centers, effectively recovering 0.7% of global computing resources that were previously untapped. This optimization does not only enhance computational efficiency but also significantly contributes to reducing energy consumption and environmental impact. Moreover, AlphaEvolve accelerated the training of Gemini models by 1%, exemplifying the potential of AI to streamline operations in technology-intensive industries. Such advancements underline the potential economic impacts, including reductions in operational costs and improved productivity across various sectors. Learn more about these achievements on TechCrunch.
Learn to use AI like a Pro
Get the latest AI workflows to boost your productivity and business performance, delivered weekly by expert consultants. Enjoy step-by-step guides, weekly Q&A sessions, and full access to our AI workflow archive.
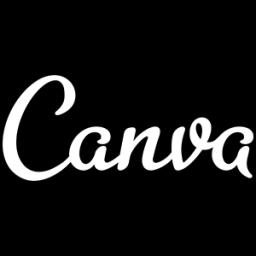
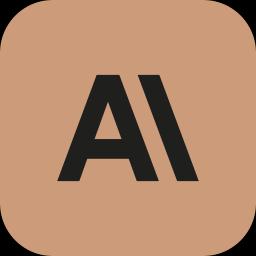
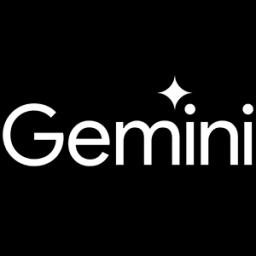
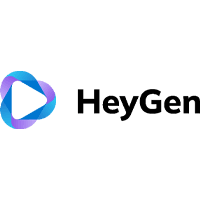
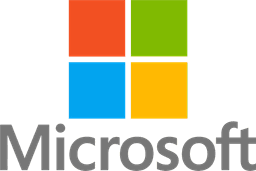
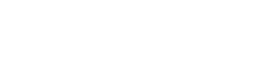
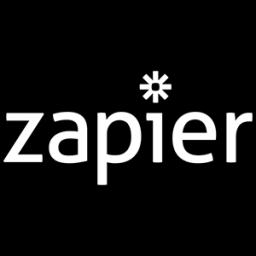
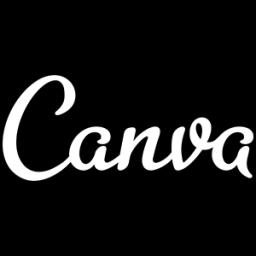
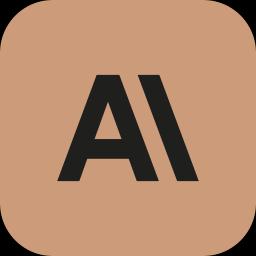
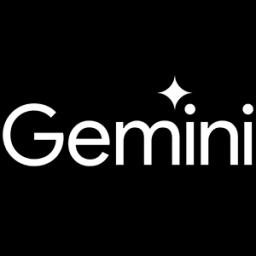
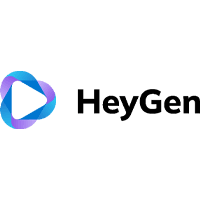
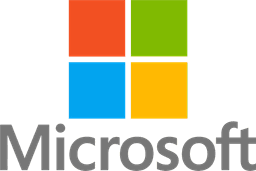
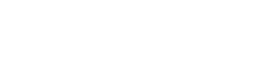
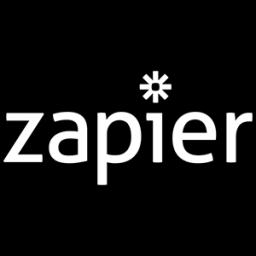
However, AlphaEvolve's journey is not without limitations and challenges. Its focus on solving numerical problems that clearly lend themselves to 'machine-gradable' solutions means that its applicability is somewhat constrained. This specificity could limit its use in fields where qualitative analysis or creative solution-finding is required. Additionally, the fact that its code is not openly available hinders independent verification and may raise transparency concerns among the academic and scientific communities. These barriers need addressing to expand its influence and applicability. The insights into these challenges can be explored further in the full article.
Preventing AI Hallucinations: How AlphaEvolve Ensures Solution Accuracy
DeepMind's AlphaEvolve is at the forefront of addressing 'AI hallucinations,' a phenomenon where AI models generate incorrect or nonsensical outputs. This issue is particularly challenging in complex domains like mathematics and science, where precision is paramount. AlphaEvolve leverages Gemini models to automatically evaluate and critique its solutions, ensuring higher accuracy and reliability. As described in TechCrunch, this capability helps AlphaEvolve to significantly reduce errors, making it an effective tool for tackling numerical problems that require machine-gradable solutions.
The efficacy of AlphaEvolve in preventing hallucinations is largely due to its algorithmic approach. Rather than merely generating answers, it employs a feedback loop of solution generation and evaluation, enhancing its capacity to debug its own outputs. This method not only safeguards against inaccurate solutions but also enables AlphaEvolve to refine its problem-solving processes continuously. TechCrunch highlights its impact on optimizing Google's data centers by reducing inefficient computing resource usage by 0.7%, underlining the effectiveness of its algorithmic accuracy in real-world applications.
By focusing on numerical problems with well-defined parameters and machine-gradable results, AlphaEvolve sidesteps many of the pitfalls associated with broader AI systems that might be prone to hallucinations due to ambiguities in unstructured data. The system's design inherently limits the scope of potential errors, creating a more controlled environment for problem-solving. As TechCrunch discusses, the automation of solution creation, evaluation, and improvement through Gemini models is a testament to its sophisticated design and operational efficacy.
Learn to use AI like a Pro
Get the latest AI workflows to boost your productivity and business performance, delivered weekly by expert consultants. Enjoy step-by-step guides, weekly Q&A sessions, and full access to our AI workflow archive.
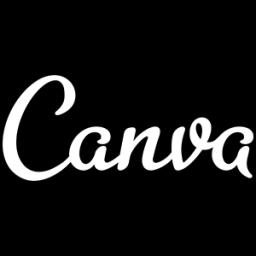
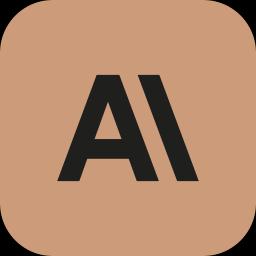
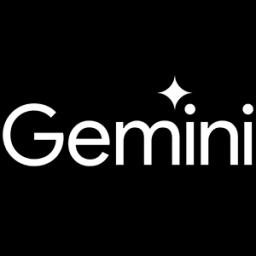
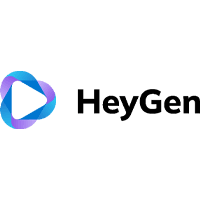
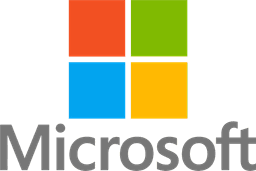
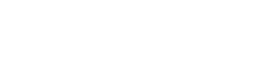
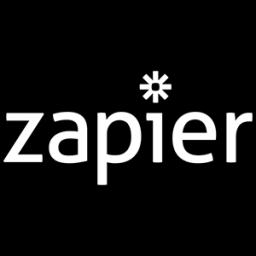
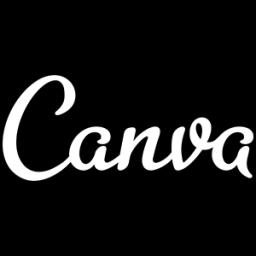
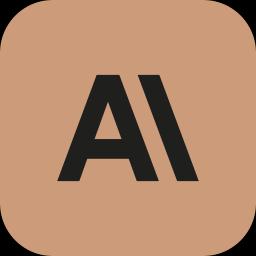
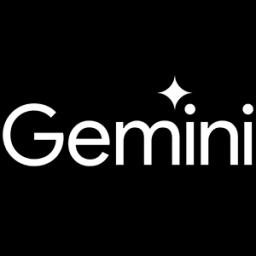
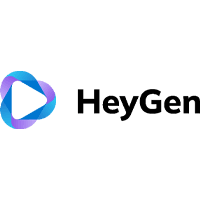
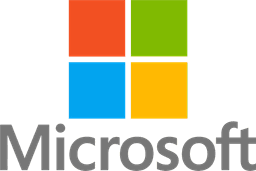
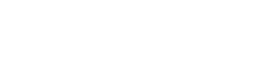
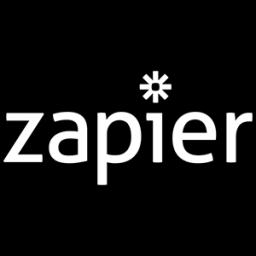
Furthermore, AlphaEvolve's impact extends beyond theory and into practical applications. The system has accelerated Gemini model training by 1%, showcasing how mitigating hallucinations translates into tangible time and resource savings. As these models become more efficient, they offer a dual advantage: enhancing computational problem-solving capacities while also conserving resources—both critical in tech development and operational environments, as noted in TechCrunch. This efficiency positions AlphaEvolve as a crucial asset in fields requiring precise algorithmic solutions.
The Scope of AlphaEvolve: Numerical and Algorithmic Problem Solving
DeepMind's AlphaEvolve, powered by the groundbreaking Gemini models, marks a significant leap in the realm of numerical and algorithmic problem-solving. This AI system is designed to tackle complex math and science problems with a high degree of accuracy. Through a process of generating, critiquing, and evaluating its own solutions, AlphaEvolve is tailored to excel in solving 'machine-gradable' numerical problems that can be expressed as algorithms. This focus on numerical problems represents both its greatest strength and a notable limitation [TechCrunch](https://techcrunch.com/2025/05/14/deepmind-claims-its-newest-ai-tool-is-a-whiz-at-math-and-science-problems/).
What sets AlphaEvolve apart is its ability to autonomously create solutions from scratch, ensuring that each solution is critically evaluated by leveraging the intricacies of Gemini models to minimize errors or 'hallucinations.' This autonomous nature makes AlphaEvolve a formidable tool in fields that depend heavily on data-driven problem-solving, such as optimizing computational resources and accelerating model training. Google's harnessing of AlphaEvolve led to recovering 0.7% of global compute resources and a 1% increase in Gemini model training efficiency, underscoring the practical benefits already realized [Scientific American](https://www.scientificamerican.com/article/new-google-ai-chatbot-tackles-complex-math-and-science/).
Although AlphaEvolve is not yet publicly available, its implications for academia and industry are vast. An early access program is planned to broaden its reach to academic researchers, providing them with the tools needed to confront and solve complex mathematical challenges. This could revolutionize how problems are approached and solved in scientific research and beyond, potentially speeding up advancements in diverse fields such as drug discovery and materials science [DeepMind Blog](https://deepmind.google/discover/blog/alphaevolve-a-gemini-powered-coding-agent-for-designing-advanced-algorithms/).
The existence of AlphaEvolve also raises pivotal questions about the future direction of AI in problem-solving. By automating the creation of algorithms, it threatens job displacement in fields that rely heavily on technical skills in algorithm design and optimization. On the other hand, there exists a great potential for economic benefit, as businesses could significantly reduce costs and improve efficiency. There is, however, an urgent need for reskilling programs to help those displaced by such technologies adapt to new roles [VentureBeat](https://venturebeat.com/ai/meet-alphaevolve-the-google-ai-that-writes-its-own-code-and-just-saved-millions-in-computing-costs/).
While AlphaEvolve's capabilities are impressive, its development also prompts discussion on ethical AI use, particularly concerning bias and fairness in algorithmic outputs. The lack of open-source access to its code has fueled calls for increased transparency to allow external verification and trust-building. Furthermore, as AlphaEvolve's optimization algorithms could conceivably be adapted for military applications, there are geopolitical worries regarding autonomous weapons and international regulatory measures to prevent misuse [Defense News](https://www.defensenews.com/artificial-intelligence/2024/07/10/pentagon-pushes-for-responsible-ai-use-amid-autonomous-weapons-concerns/).
Learn to use AI like a Pro
Get the latest AI workflows to boost your productivity and business performance, delivered weekly by expert consultants. Enjoy step-by-step guides, weekly Q&A sessions, and full access to our AI workflow archive.
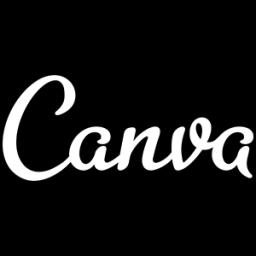
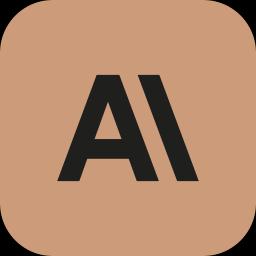
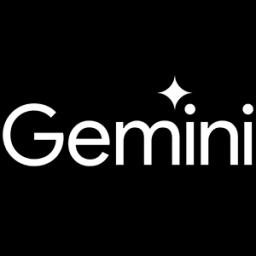
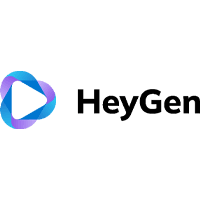
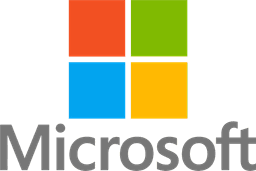
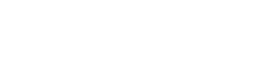
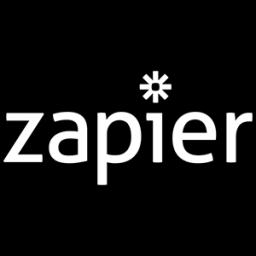
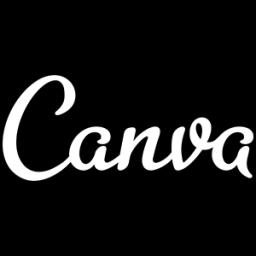
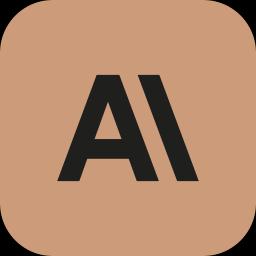
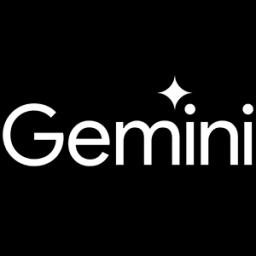
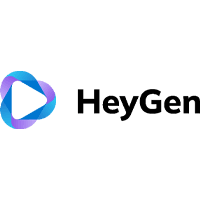
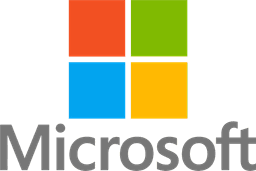
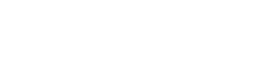
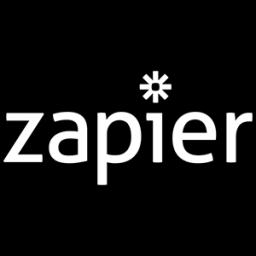
AlphaEvolve's Impact on Google's Infrastructure and Gemini Training
AlphaEvolve, an advanced AI system developed by DeepMind, is reshaping Google's infrastructure by optimizing data center operations and enhancing the training of Gemini models. Leveraging its ability to tackle complex numerical problems, AlphaEvolve has promoted efficiency within Google's vast network of servers, enabling a notable 0.7% reduction in the global compute resource usage. This optimization is crucial, as it represents a sizeable achievement in reducing energy consumption and operational costs across Google's data centers. Furthermore, AlphaEvolve's impact extends to the acceleration of Gemini model training by 1%, demonstrating its proficiency in refining AI training processes. Such enhancements not only augment Google's computational capabilities but also highlight the potential of AI in streamlining operations across various sectors. Read more.
The introduction of AlphaEvolve marks a significant milestone in AI development, particularly in the context of Google's infrastructure and Gemini training. By automating the solution generation, critique, and evaluation processes, AlphaEvolve has transcended traditional AI boundaries, offering a glimpse into the future of autonomous optimization tools. This advancement facilitates not only increased efficiency but also positions Google at the forefront of AI-driven innovation. The ramifications of such technology extend beyond mere performance improvements, suggesting broader applications in industries requiring complex problem-solving capabilities. As Google continues to integrate AlphaEvolve, the potential for reshaping computing, research, and development landscapes becomes increasingly apparent, shedding light on AI's transformative potential. Learn more.
While AlphaEvolve's impact on Google's infrastructure is making headlines, its influence on Gemini model training underscores the critical role of AI in advancing machine learning frameworks. By reducing training time by 1%, AlphaEvolve ensures that Google can deploy new models more swiftly, which is vital in maintaining a competitive edge in the fast-evolving field of AI. This advancement is not just about speed; it's about the quality and efficiency of training processes that lead to more robust, capable AI systems. The synergy between AlphaEvolve and the Gemini models exemplifies how specialized AI tools can synergize to overcome computational challenges, paving the way for future innovations in AI research and application. Explore further.
As AlphaEvolve continues to optimize and innovate within Google's frameworks, it lays the groundwork for broader societal benefits. The capability to refine Google's infrastructure suggests significant economic implications, potentially reducing operational costs and paving the way for sustainable growth. Moreover, as part of a planned early access program for academics, AlphaEvolve promises to be a catalyst for research and discovery across numerous disciplines. This accessibility fosters an academic synergy that could accelerate innovation in fields like drug discovery, materials science, and beyond. However, such advancements also necessitate dialogue around ethical considerations, ensuring that the deployment of such transformative technologies remains fair and transparent. Discover more.
Availability of AlphaEvolve: Early Access and Future Release Plans
DeepMind has unveiled plans for AlphaEvolve, an AI system renowned for tackling complex math and science problems, by initially launching an early access program. This initiative targets academics and schools, allowing them to explore the system's capabilities in a controlled environment before its broader availability. The early access phase signifies a deliberate approach to fine-tuning and validating AlphaEvolve's problem-solving prowess, ensuring it delivers reliable results when applied to real-world scenarios. According to a recent article, this phase will provide insights into AlphaEvolve's potential adaptations and improvements for further optimization [source].
Following the early access period, DeepMind plans to roll out a wider release of AlphaEvolve, aiming to bring its advanced AI capabilities to broader markets and industries. This strategic move comes on the heels of successful trials in academic settings, reinforcing confidence in its practicality and effectiveness. The anticipation of a larger release also stirs excitement within industries eager to leverage AI for enhanced computational solutions and operational efficiencies. This progressive launch strategy not only positions AlphaEvolve as a leader in AI problem-solving tools but also underscores DeepMind's commitment to ensuring its systems are adaptable and trustworthy before wide-scale deployment [source].
Learn to use AI like a Pro
Get the latest AI workflows to boost your productivity and business performance, delivered weekly by expert consultants. Enjoy step-by-step guides, weekly Q&A sessions, and full access to our AI workflow archive.
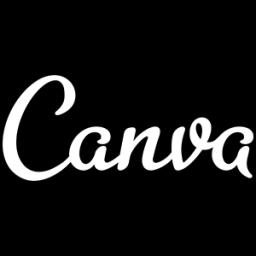
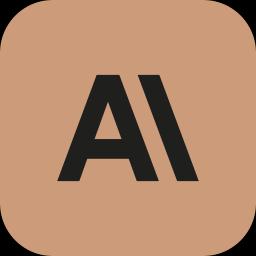
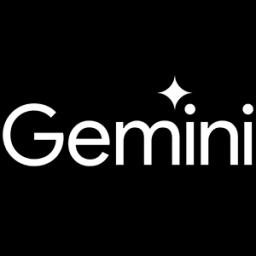
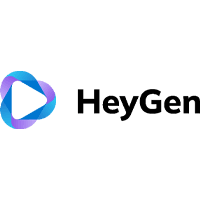
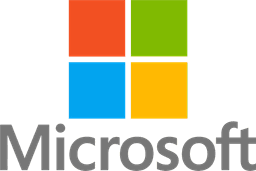
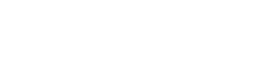
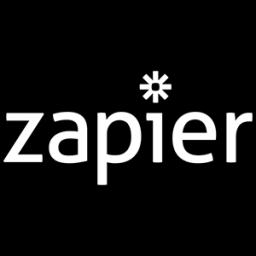
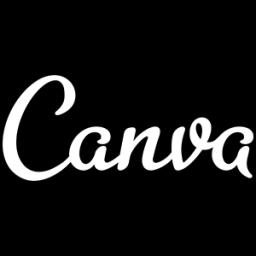
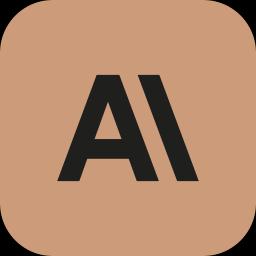
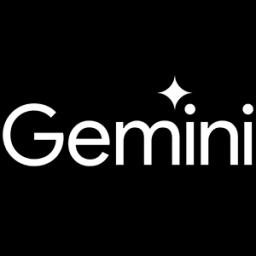
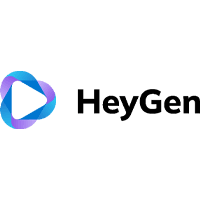
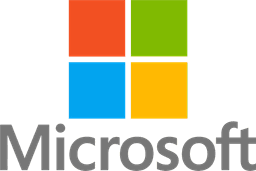
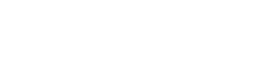
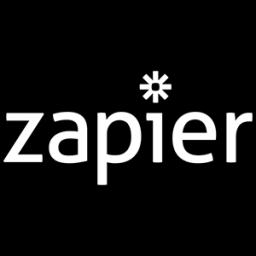
AlphaEvolve in Comparison: Novelty and Limitations
DeepMind's AlphaEvolve represents a significant advancement in artificial intelligence, particularly in tackling complex math and science problems. While its novel ability to self-generate, critique, and evaluate solutions sets it apart from traditional AI tools, there are limitations to consider. One of the most impressive aspects is its optimization capabilities, which have notably enhanced Google's data center efficiency and accelerated the training of Gemini models . Such enhancements underscore AlphaEvolve's potential in optimizing large-scale computational resources, yet its utility is primarily confined to numerical problems due to its dependency on algorithmic and machine-gradable solutions. This focus, while empowering in specific domains, restricts its application breadth, making it less versatile in non-numerical contexts .
Despite its constraints, AlphaEvolve contributes a strategic advantage in environments where optimization of mathematical and computational tasks is paramount. By effectively sustaining high-level performance in these areas, it demonstrates a robust problem-solving capacity that is enviable among AI systems. However, the scope of its novel discoveries has been met with skepticism. Many experts point out that the tool does not fundamentally pioneer new solutions but excels at refining and automating existing ones. This distinction is crucial, especially as the AI community weighs its contributions against other tools that might offer groundbreaking insights into unsolved problems .
There is ongoing debate about the accessibility and transparency of AlphaEvolve, as its proprietary nature limits independent verification and broader academic adoption. The call for an open-source variant is growing, driven by researchers who advocate for enhanced scrutiny and collaboration. Such openness could facilitate more rigorous testing and validation in diverse environments, potentially broadening its impact beyond its current capabilities . Nevertheless, DeepMind's emphasis on controlled academic testing through an early access program promises to alleviate some concerns, fostering a gradual, yet secure, evaluation of its effectiveness in real-world applications .
The balanced view of AlphaEvolve is that it is a tool of significant capability and limitation. While public reaction has measured both impressment and reservations, the future implications of its deployment are vast. Its optimization prowess holds economic promise, particularly in operational efficiencies and resource management within large enterprises. Nevertheless, social and ethical considerations must guide its evolution, especially regarding its fairness and the potential for bias in automated decision-making processes . Politically, the technology should be carefully regulated to prevent misuse in sensitive areas such as military applications, ensuring that AlphaEvolve evolves as a tool for positive global advancement rather than contention .
AI Tools in Math Competitions: Achievements of AlphaProof and AlphaGeometry 2
The realm of math competitions has witnessed a transformative shift with the rise of AI tools, exemplified by DeepMind's AlphaProof and AlphaGeometry 2. These cutting-edge systems have demonstrated their prowess by achieving a silver medal-equivalent score at the prestigious International Mathematical Olympiad (IMO). This achievement underscores the potential of AI in tackling complex reasoning and problem-solving tasks that are characteristic of high-level mathematical contests.
Google's AI Success in Medical Diagnosis and Data Center Optimization
Google's substantial investments in artificial intelligence have borne fruit, as exemplified by their significant successes in both medical diagnosis and data center optimization. At the forefront of these achievements is DeepMind, a subsidiary of Alphabet, which has pioneered some groundbreaking AI systems like AlphaEvolve. This system specializes in solving intricate numerical problems, leveraging its capability to generate, critique, and refine its solutions autonomously. These capabilities have proven invaluable not just in theoretical setups, but in practical applications like optimizing Google's massive data center operations. AlphaEvolve's introduction led to a notable recovery of 0.7% in global compute resources by optimizing data processes, which is a testament to the profound impact AI can have on infrastructure and energy efficiency. [TechCrunch](https://techcrunch.com/2025/05/14/deepmind-claims-its-newest-ai-tool-is-a-whiz-at-math-and-science-problems/).
Learn to use AI like a Pro
Get the latest AI workflows to boost your productivity and business performance, delivered weekly by expert consultants. Enjoy step-by-step guides, weekly Q&A sessions, and full access to our AI workflow archive.
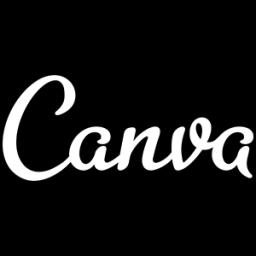
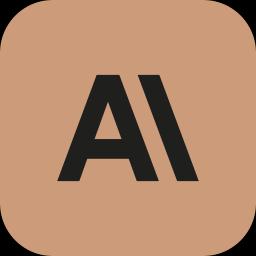
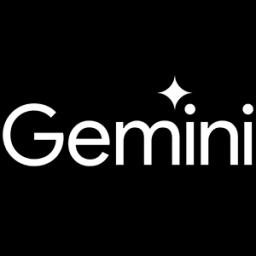
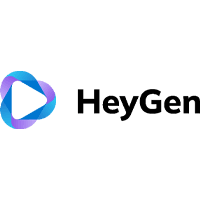
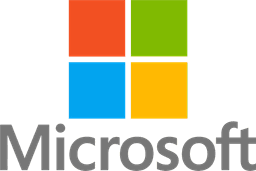
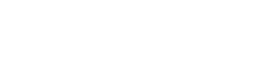
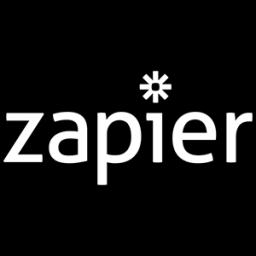
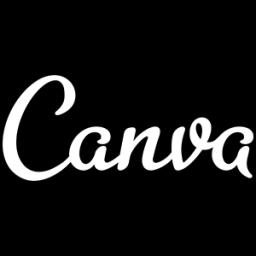
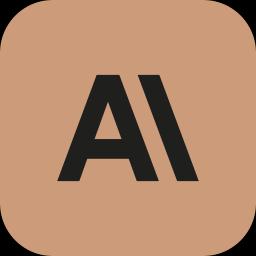
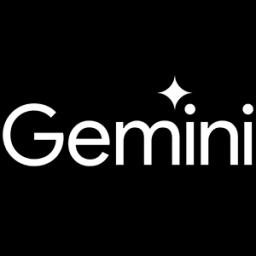
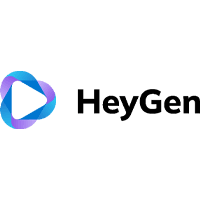
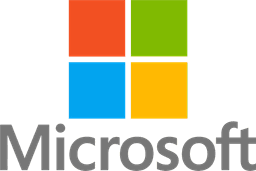
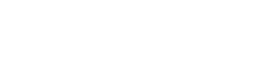
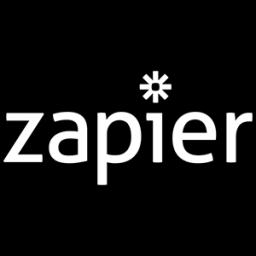
In the medical field, Google's AI initiatives have also made groundbreaking strides. Their AI systems have been developed to diagnose skin rashes by analyzing images with greater accuracy than human doctors, showcasing the power of artificial intelligence in medical diagnostics. Such advancements underscore AI's potential in complex pattern recognition and analysis, paving the way for more precise and rapid diagnostics which can significantly improve patient outcomes. This development is a part of a larger movement towards integrating AI with healthcare to enhance the efficiency and accuracy of diagnostic processes, as reported by [Nature](https://www.nature.com/articles/d41586-025-01523-z).
The ability of Google's AI technologies to optimize and innovate within data centers speaks volumes about its strategic importance in the tech industry. The Keysight AI Data Center Builder, for instance, has been designed specifically to enhance the validation and optimization of network architecture, reflecting a growing trend of using AI to boost efficiency in data center operations. By making data centers smarter and more efficient, Google is not only cutting costs but also reducing its energy footprint, making strides towards sustainable computing solutions [BusinessWire](https://www.businesswire.com/news/home/20250401898311/en/Keysight-Introduces-AI-Data-Center-Builder-to-Validate-and-Optimize-Network-Architecture-and-Host-Design).
The dual focus on health and operational efficiency by Google's AI research highlights a strategic alignment with global needs for both health innovations and sustainable technology practices. By pushing the boundaries of AI capabilities, particularly through innovations like AlphaEvolve, Google isn't just solving today's problems but setting the stage for tomorrow's technological landscape. These advancements also emphasize the cross-sector applicability of AI, demonstrating its potential to revolutionize industries by making diagnostics more accurate and operations more efficient. The widespread implications of such technologies indicate that AI can drive significant socio-economic benefits while also prompting discussions on ethical standards and regulatory measures to ensure responsible use [Scientific American](https://www.scientificamerican.com/article/new-google-ai-chatbot-tackles-complex-math-and-science/).
Expert Opinions on AlphaEvolve's Methodology and Applicability
The introduction of DeepMind's AlphaEvolve has sparked interest and excitement across the academic and technological communities, particularly due to its unique approach to problem-solving. Experts have lauded its ability to not just solve complex math and science problems but to evaluate and refine its solutions autonomously. Jakob Moosbauer, a renowned mathematician at the University of Warwick, finds the methodology behind AlphaEvolve's algorithmic exploration groundbreaking. By focusing on algorithms that produce specific outcomes, rather than merely finding solutions, AlphaEvolve offers a broader scope for experimentation and potential breakthroughs in both mathematical and computational research. This capability is seen as a significant step forward, supporting the acceleration of research and potentially transforming academic approaches to problem-solving .
Despite these accolades, there is a blend of skepticism mixed with appreciation among experts regarding AlphaEvolve's impact and applicability. While the system has achieved substantial success in enhancing Google's data efficiency and optimizing computational resources, its primary limitation lies in its focus—that is, its application is restricted to numerical problems with machine-gradable solutions. Such constraints raise questions about its adaptability to broader, less structured domains. Some experts have voiced concerns that the lack of public access to AlphaEvolve's coding framework hampers independent validation of its efficacy, which could limit widespread application and acceptance .
The anticipation surrounding AlphaEvolve's early access program for academics underlines the cautious optimism within the scientific community. While the potential for groundbreaking discoveries enhances excitement, many researchers remain wary of the practical and ethical implications of deploying such powerful AI systems. There are calls for stringent controls and broader transparency to ensure fair and unbiased outputs. Ultimately, the success of this program will be pivotal in determining the broader applicability and reliability of AlphaEvolve's methodologies in the scientific domain .
Learn to use AI like a Pro
Get the latest AI workflows to boost your productivity and business performance, delivered weekly by expert consultants. Enjoy step-by-step guides, weekly Q&A sessions, and full access to our AI workflow archive.
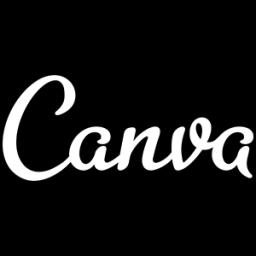
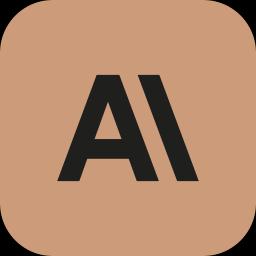
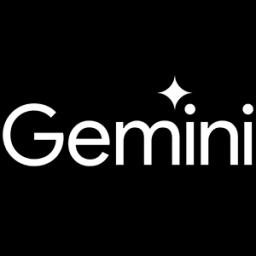
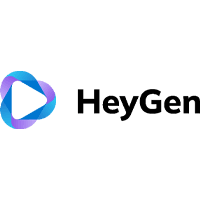
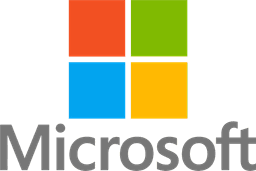
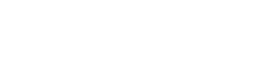
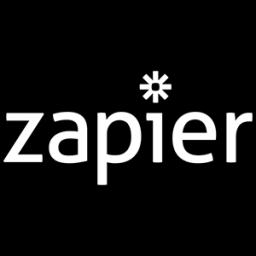
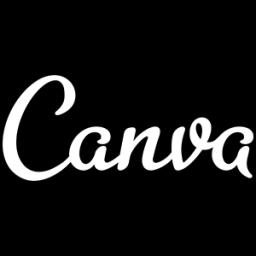
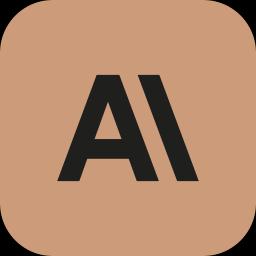
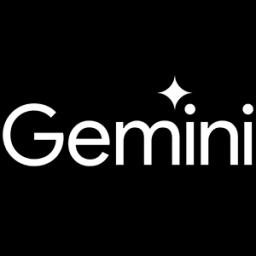
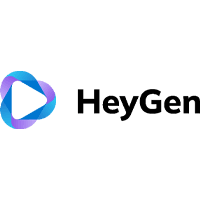
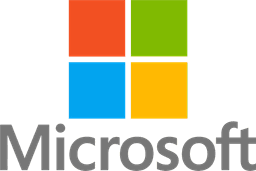
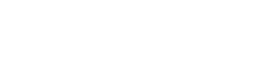
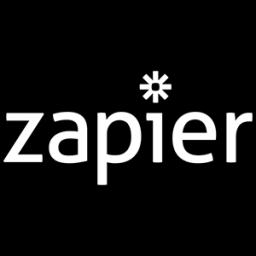
Public Reactions and Skepticism Towards AlphaEvolve's Capabilities
Public reactions to DeepMind's AlphaEvolve have been a mix of awe and skepticism. While some people are impressed with the AI's ability to self-generate, critique, and evaluate solutions, others express concern about its practical utility outside of theoretical realms. For instance, AlphaEvolve's remarkable success in optimizing Google's data centers, contributing to a significant reduction in computing resource usage, has sparked admiration and has been highlighted in various media, such as in a piece by TechCrunch. However, its perceived limitation to only numerical and machine-gradable problems leaves some experts doubtful about its broader applicability.
Skepticism also surfaces from the planned early access program aimed at academics, which has fueled both anticipation and doubt. Enthusiasm is tempered by the system's confinement to controlled environments within DeepMind, which some experts fear could bias its perceived effectiveness. An article from Scientific American captures these mixed reactions, noting the reservations of researchers who call for testing in unmoderated, real-world scenarios to truly assess its capabilities.
The lack of an open-source code version of AlphaEvolve has led to further skepticism, particularly regarding the novelty and reproducibility of its results. As noted on Hacker News, there is a persistent debate among experts and enthusiasts alike about the true innovation presented by the system. While some argue that AlphaEvolve is a pioneering advancement in algorithm design, others view it as an incremental step rather than a groundbreaking leap.
Despite these concerns, many believe in AlphaEvolve's potential to transform significant sectors if the limitations can be addressed. Experts quoted in articles on Technology Review argue for its substantial promise in research and computational fields, provided that challenges regarding transparency and verification are tackled. This duality in perspectives highlights a broader discourse on the prospects and challenges of AI in mathematical and scientific problem-solving.
Future Implications: Economic, Social, and Political Impact of AlphaEvolve
AlphaEvolve, DeepMind's latest AI innovation, stands on the brink of revolutionizing the economic, social, and political landscapes with its breakthrough capabilities in solving intricate numerical challenges using Gemini models. On the economic front, the AI's prowess in enhancing data center efficiency and accelerating AI model training has already shown substantial cost savings and power usage reduction at Google. This echoes a broader potential for transforming sectors such as drug discovery, where accelerated data processing could significantly reduce development timelines. However, such advancements could lead to job displacement, particularly in fields related to algorithm design and optimization, necessitating proactive measures for workforce reskilling. An in-depth analysis of these implications can be accessed [here](https://techcrunch.com/2025/05/14/deepmind-claims-its-newest-ai-tool-is-a-whiz-at-math-and-science-problems/).
Socially, AlphaEvolve's applicability promises to democratize access to advanced problem-solving tools, offering academic researchers a quantum leap in their ability to tackle complex mathematical and scientific problems. This democratization could greatly enhance collaborative scientific inquiry and discovery, pushing the boundaries of current knowledge. Yet it also poses ethical challenges. Issues of fairness, bias, and accessibility must be addressed to prevent the amplification of existing inequalities. Comprehensive coverage on AlphaEvolve's societal impact can be found within DeepMind's [blog](https://deepmind.google/discover/blog/alphaevolve-a-gemini-powered-coding-agent-for-designing-advanced-algorithms/).
Learn to use AI like a Pro
Get the latest AI workflows to boost your productivity and business performance, delivered weekly by expert consultants. Enjoy step-by-step guides, weekly Q&A sessions, and full access to our AI workflow archive.
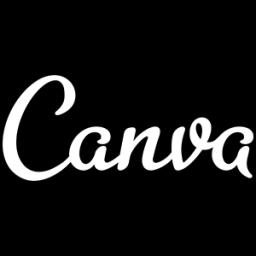
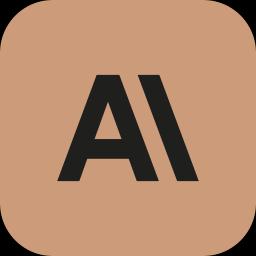
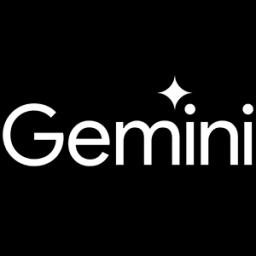
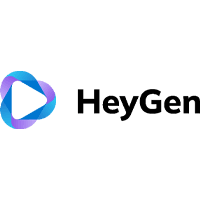
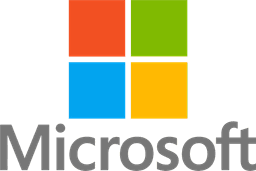
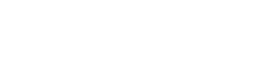
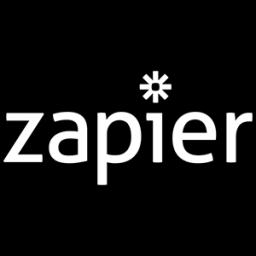
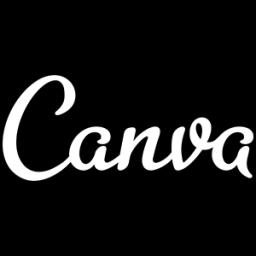
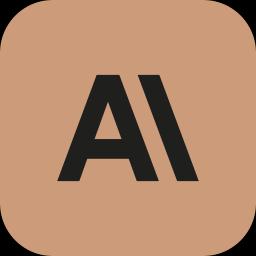
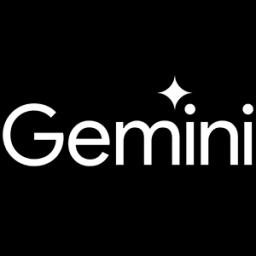
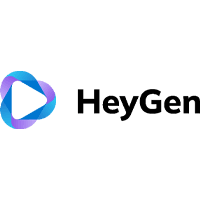
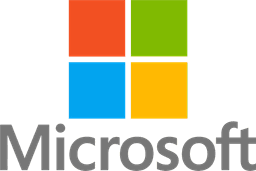
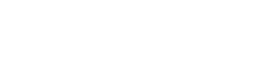
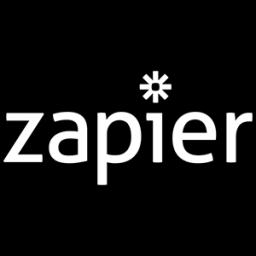
Politically, AlphaEvolve's technology could influence global dynamics, particularly if its optimization capabilities find applications in defense sectors. The potential for military utilization raises critical questions about the governance of AI technologies and the urgent need for international regulation to curb risks associated with autonomous systems. Moreover, the ability of such technology to heighten competitiveness in AI-driven economies could shift geopolitical power balances. For a detailed exploration of these political dimensions, see this analysis on [Brookings Institution's](https://www.brookings.edu/articles/regulating-ai-what-explainer/) site. As AlphaEvolve undergoes early-access trials in academic settings, these political considerations will undoubtedly shape discussions on its widespread integration.