A Game-Changer in Open-Source AI
DeepSeek R1: The Reinforcement Learning Marvel Outpacing OpenAI at a Fraction of the Cost!
Last updated:

Edited By
Mackenzie Ferguson
AI Tools Researcher & Implementation Consultant
Discover how DeepSeek R1, the trailblazing AI model leveraging pure reinforcement learning, has matched OpenAI's performance at just 3-5% of the cost. Unveiling a new era in cost-efficient AI development, this open-source model challenges industry giants and democratizes high-tech access. However, concerns over potential biases and security implications loom as discussions ignite across the AI community.
Introduction to DeepSeek R1
DeepSeek R1 represents a significant breakthrough in the field of artificial intelligence, with its innovative use of pure reinforcement learning (RL) that largely bypasses traditional supervised fine-tuning (SFT) methods. This approach has enabled the open-source AI model to achieve performance parity with OpenAI's offerings at a mere 3-5% of the cost. The development of DeepSeek R1 marks a pivotal moment as it challenges existing assumptions about the economics of state-of-the-art AI technology, potentially reshaping the industry's landscape.
One of the critical advancements of DeepSeek R1 is its pioneering RL-focused approach that facilitates independent reasoning and task prioritization. While limited supervised fine-tuning was later introduced to enhance readability and reduce language mixing, the model's backbone remains centered around reinforcement learning, setting it apart from other AI systems. This breakthrough not only democratizes access to advanced AI through its open-source nature but also places it in direct competition with other prominent open-source models like Meta's Llama and Mistral.
Learn to use AI like a Pro
Get the latest AI workflows to boost your productivity and business performance, delivered weekly by expert consultants. Enjoy step-by-step guides, weekly Q&A sessions, and full access to our AI workflow archive.
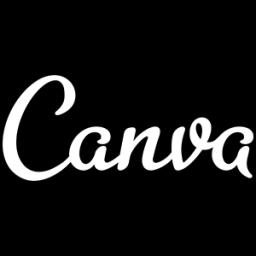
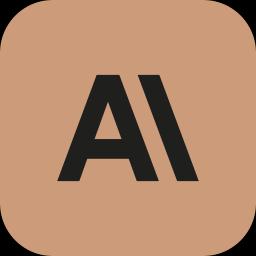
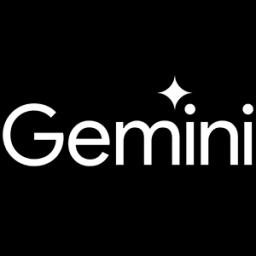
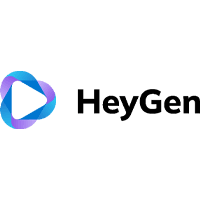
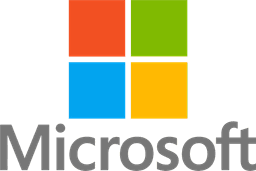
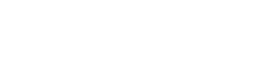
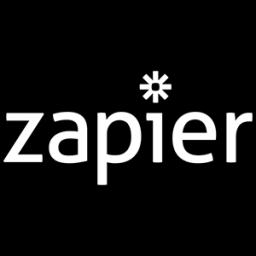
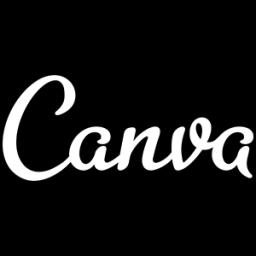
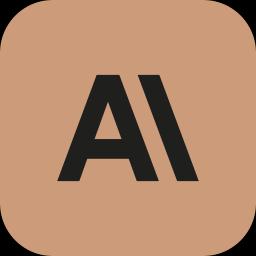
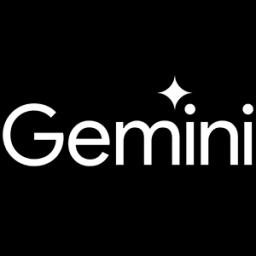
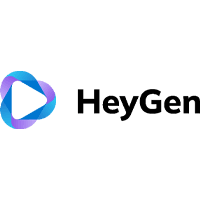
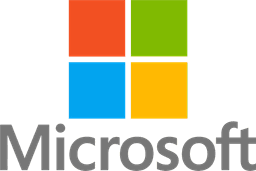
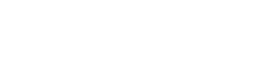
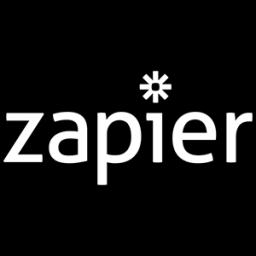
DeepSeek R1's cost efficiency is attributed to its reliance on reinforcement learning instead of the more expensive supervised fine-tuning, bringing down the training costs significantly. Furthermore, across various performance benchmarks, DeepSeek R1 has managed to match the outputs of OpenAI's counterparts, showcasing impressive parity in both capability and efficiency. However, it is important to note the challenges DeepSeek R1 faces, particularly regarding potential biases influenced by Chinese regulatory standards and undisclosed specifics of its training data.
The impact of DeepSeek R1 on the industry is profound, as it presents a counter-narrative to the market's dominant proprietary models that often come with massive budgets. By operating at a fraction of the cost, this model could lead to a democratization of AI technology, opening new opportunities for smaller organizations and researchers who have previously been obstructed by economic barriers.
Related significant events in the AI industry have been shaped by players recognizing the potential of reinforcement learning and open-source models. ByteDance has similarly showcased its ability to outperform with less, releasing a new AI model that claims better performance compared to OpenAI's benchmark tests. Concurrently, Meta has doubled down on its commitment to open-source AI development with a substantial $60 billion investment, driving the momentum further away from costly proprietary models.
Experts in artificial intelligence, such as Alex Zhavoronkov and Dr. Sarah Chen, highlight both the innovative and contentious aspects of DeepSeek R1. Zhavoronkov points out the model's ability to promote chain-of-thought reasoning and enable self-verification through its RL-based methodology. Nevertheless, concerns around biases and ethical considerations, especially those related to its training data and compliance with regional regulations, remain prominent in discussions surrounding the model.
Learn to use AI like a Pro
Get the latest AI workflows to boost your productivity and business performance, delivered weekly by expert consultants. Enjoy step-by-step guides, weekly Q&A sessions, and full access to our AI workflow archive.
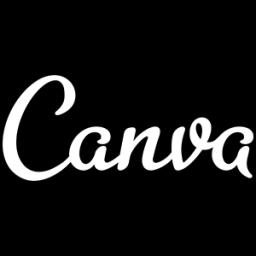
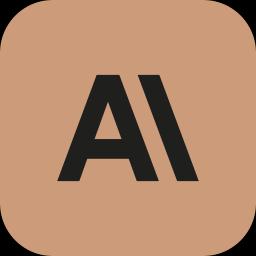
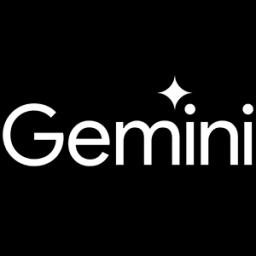
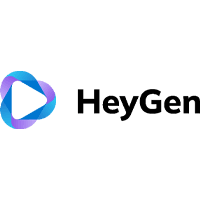
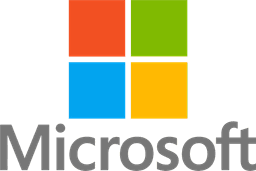
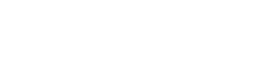
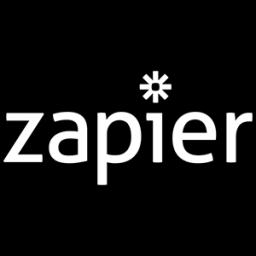
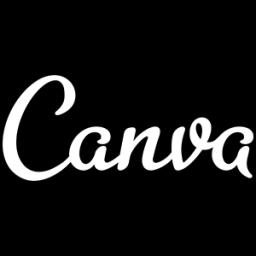
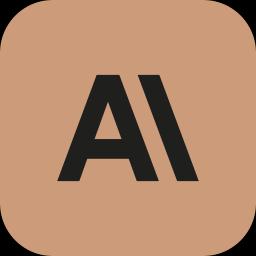
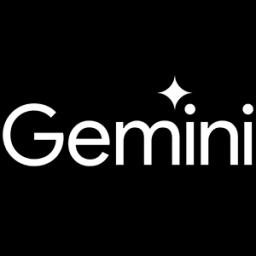
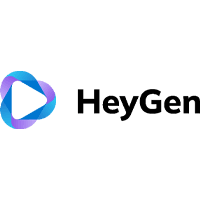
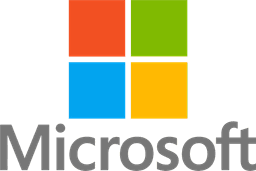
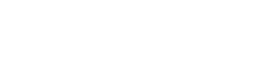
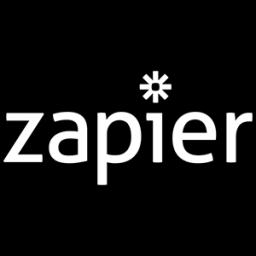
Public responses have been mixed, with many lauding DeepSeek R1's cost-effective performance and accessibility. However, these positives are counterbalanced by concerns regarding the originality and possible biases inherent in its training. Additionally, issues related to security in open-source models highlight the ongoing debate about the ethical implications of increasingly accessible and powerful AI tools.
Looking ahead, the implications of DeepSeek R1's emergence in the AI landscape could be transformative. By dramatically reducing the cost of AI model development, it holds the potential to disrupt existing market dynamics and challenge the expansive business models of proprietary leaders like OpenAI and Google. This shift toward more cost-effective AI solutions may accelerate adoption across industries, fundamentally changing how businesses approach AI integration in their operations.
Beyond technical and economic impacts, geopolitical considerations are also at play as AI leadership dynamics between China and the US evolve. With OpenAI's and DeepSeek's advancements, global AI strategies might reflect not only the need for compliance with local regulations but also the broader implications of AI capabilities on the international stage. As AI technology continues to mature, the global community must also contend with its societal and ethical ramifications.
The Reinforcement Learning Approach
The article delves into the innovative reinforcement learning (RL) approach undertaken by DeepSeek R1. This open-source AI model has made headlines for achieving performance levels on par with OpenAI's o1, all while operating at a fraction of the cost—between 3-5% of its counterparts. DeepSeek R1's strength lies in its unique methodology; rather than relying heavily on traditional supervised fine-tuning, it embraces a pure reinforcement learning strategy. This enables the model to independently reason and prioritize tasks effectively.
One of the key developments highlighted is DeepSeek R1's pioneering RL-focused approach which allows it to perform with minimal human intervention. This model encapsulates the ability to analyze tasks and choose pathways that maximize the desired outcomes, showcasing an advanced degree of independence and efficiency. While supervised fine-tuning was later integrated to enhance language clarity and coherence, DeepSeek R1 primarily stands out for its cost-effective RL-driven framework.
With an open-source platform, DeepSeek R1 democratizes access to cutting-edge AI technology, providing a valuable resource for smaller businesses and researchers with limited budgets. Despite its advantages, the model faces stiff competition from other open-source rivals like Meta's Llama and Mistral which are also advancing quickly in the AI landscape.
Learn to use AI like a Pro
Get the latest AI workflows to boost your productivity and business performance, delivered weekly by expert consultants. Enjoy step-by-step guides, weekly Q&A sessions, and full access to our AI workflow archive.
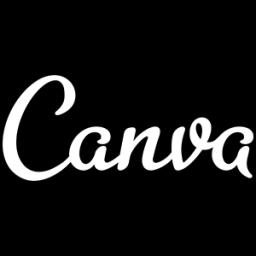
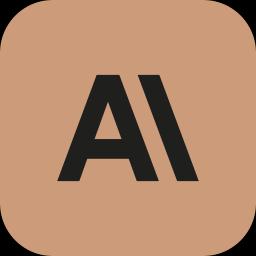
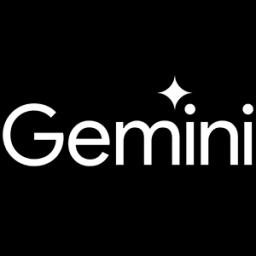
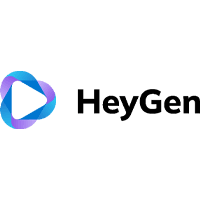
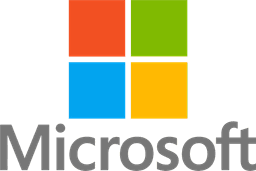
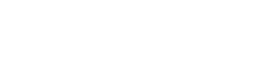
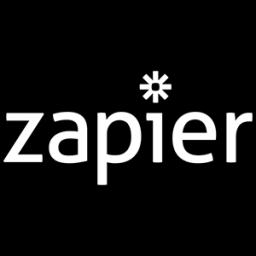
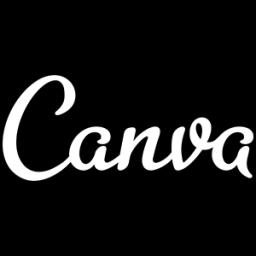
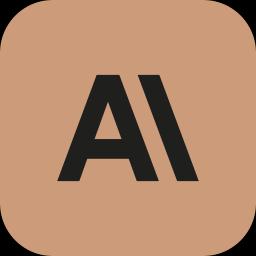
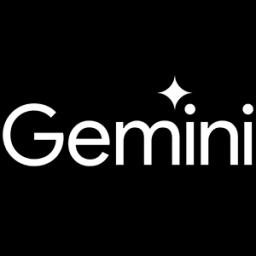
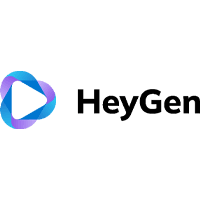
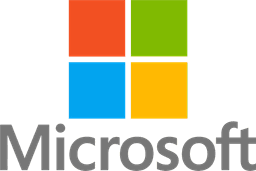
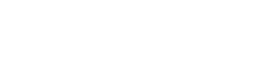
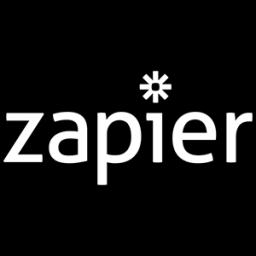
The cost efficiency of DeepSeek R1 largely stems from its focus on reinforcement learning, which circumvents the expensive process of supervised fine-tuning. This strategic choice reduces training costs substantially, enabling the model to operate at about 3-5% of what its competitors incur. Performance benchmarks reveal that DeepSeek R1 successfully competes with OpenAI's offerings, especially in terms of task execution and cost management.
However, DeepSeek R1 is not without its criticisms. Concerns have emerged regarding potential biases, especially given the undisclosed nature of its training data. There are also apprehensions about how the model's development aligns with regulatory standards, particularly those in China, and whether such alignments affect its impartiality and credibility.
The broader impact of DeepSeek R1 on the AI industry is significant. By challenging the notion that state-of-the-art AI models require large financial investments, it disrupts existing market dynamics and pushes for a reevaluation of how AI developments can be made accessible and sustainable globally. Its open-source nature encourages innovation but also raises questions about security and ethical governance. As the model continues to gain traction, it represents a paradigm shift in how advanced AI can be developed and deployed with economic prudence.
Cost Efficiency Compared to OpenAI
DeepSeek R1 has achieved remarkable cost efficiency by leveraging a predominantly reinforcement learning-based approach, significantly reducing expenses compared to its competitors like OpenAI. The model's reliance on reinforcement learning over supervised fine-tuning minimizes training costs, allowing DeepSeek R1 to operate at just 3-5% of OpenAI's expenditure. This cost effectiveness not only highlights the potential of alternative AI training methodologies but also challenges the prevailing notion that substantial budgets are required to achieve state-of-the-art performance.
The open-source nature of DeepSeek R1 further enhances its cost efficiency by democratizing technology access and fostering a collaborative development environment. The model's transparency allows smaller organizations and researchers with limited resources to utilize advanced AI capabilities without incurring the high costs typically associated with proprietary models. This shift is particularly advantageous for encouraging innovation and experimentation within the AI community, paving the way for new applications and enhancements driven by diverse contributors.
In addition to its economic advantages, DeepSeek R1's cost-efficient model is strategically positioned to disrupt the market dominance of established proprietary models such as OpenAI's offerings. By providing comparable performance at a fraction of the cost, DeepSeek R1 introduces competitive pressure on larger AI companies to reevaluate their pricing models and development strategies. This competition could potentially lead to more affordable AI solutions globally, benefiting a wide array of industries eager to integrate AI technologies.
Learn to use AI like a Pro
Get the latest AI workflows to boost your productivity and business performance, delivered weekly by expert consultants. Enjoy step-by-step guides, weekly Q&A sessions, and full access to our AI workflow archive.
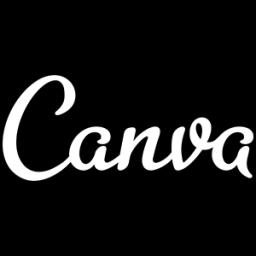
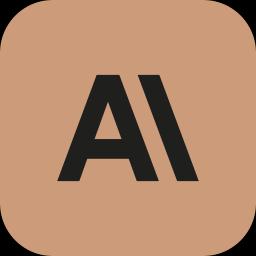
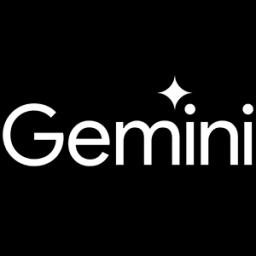
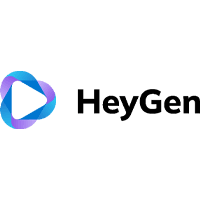
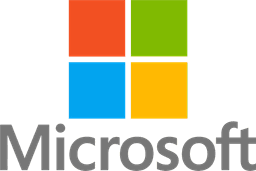
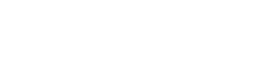
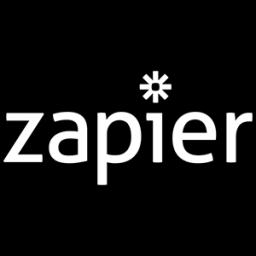
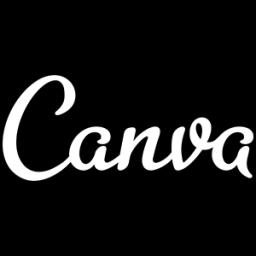
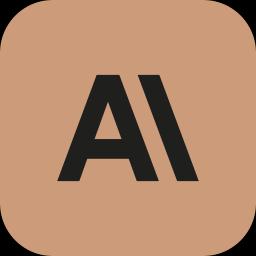
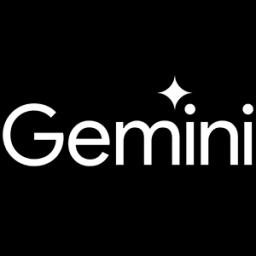
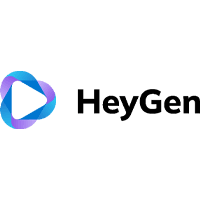
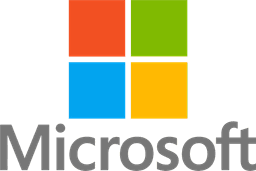
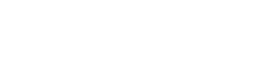
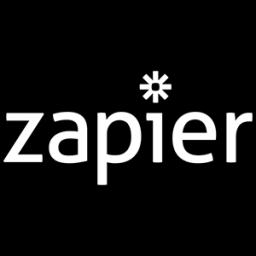
However, despite its cost benefits, DeepSeek R1 must navigate potential challenges, including biases influenced by regional regulations and concerns about the model's compliance with local censorship laws. These factors may affect its widespread acceptance and deployment. Yet, by maintaining a commitment to cost efficiency and open accessibility, DeepSeek R1 exemplifies how innovative training approaches can make advanced AI more attainable and practical across different economic landscapes.
Overall, DeepSeek R1's cost efficiency not only underscores the transformative potential of reinforcement learning but also sets a new precedent for AI development. By reducing the financial barriers to access, the model fosters a more inclusive AI landscape, where cutting-edge technology is within reach for a broader spectrum of users and developers. This shift may drive accelerated AI adoption, technological advancement, and exploration of novel AI applications that were previously constrained by cost.
Limited Use of Supervised Fine-Tuning (SFT)
The DeepSeek R1 model represents a significant departure from conventional methods in AI development by minimizing the use of supervised fine-tuning (SFT) in favor of reinforcement learning (RL). This strategic choice underlines a growing trend in AI research, where RL is increasingly leveraged to enhance independent reasoning and task prioritization within AI models. By primarily focusing on RL, DeepSeek R1 has achieved cost efficiency and performance levels comparable to leading proprietary models without the financial burden typically associated with extensive SFT processes.
The integration of limited SFT into DeepSeek R1 was a calculated enhancement to address specific shortcomings like readability and language mixing. While RL excels in providing the model with capabilities such as self-verification and independent decision-making, supervised fine-tuning ensures that the output remains coherent and contextually accurate. This dual approach facilitates a balance between autonomous learning and content reliability, showcasing how could AI systems optimize both efficacy and efficiency, breaking away from heavy reliance on costly fine-tuning processes.
This innovative model showcases the potential for RL to fundamentally change the financial landscape of AI development. By reducing the need for large-scale SFT, DeepSeek R1 drastically cuts down on training expenditures. This reduction can democratize access to high-performance AI systems, providing significant advantages to smaller enterprises and academic communities that are often limited by budget constraints. The cost-effective nature of DeepSeek R1 challenges larger corporations' dominance in the AI industry, fostering an environment ripe for competition and innovation.
The broader implication of DeepSeek R1's limited use of SFT redefines expectations in AI capabilities and resource allocation. As the model achieves impressive results within the constraints of significantly reduced training budgets, it invites a reassessment of resource distribution in AI development. This shift could prompt a transition towards more scalable and financially accessible AI solutions, aligning with the open-source movement's objectives of inclusivity and widespread technological advancement.
Learn to use AI like a Pro
Get the latest AI workflows to boost your productivity and business performance, delivered weekly by expert consultants. Enjoy step-by-step guides, weekly Q&A sessions, and full access to our AI workflow archive.
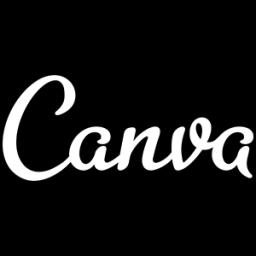
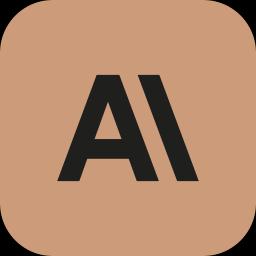
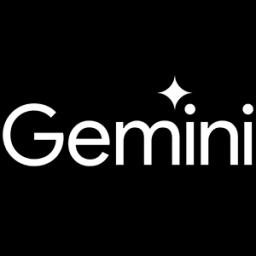
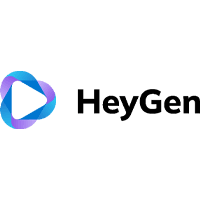
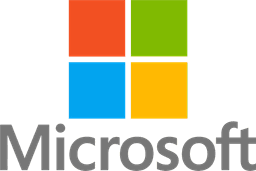
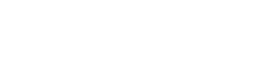
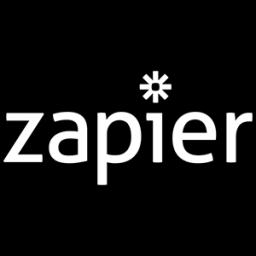
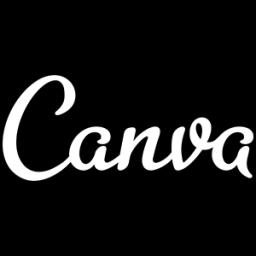
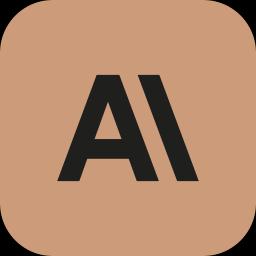
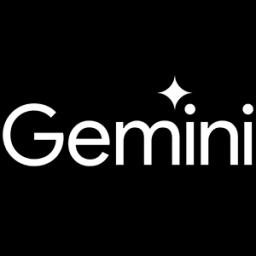
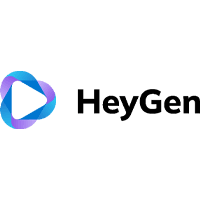
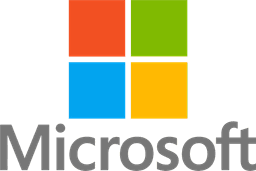
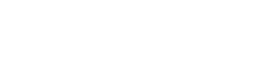
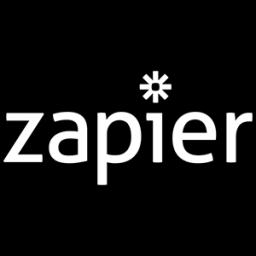
In summary, DeepSeek R1 exemplifies a pivotal shift away from dependency on comprehensive SFT processes, highlighting how innovative learning methodologies can achieve comparable or superior results in a cost-effective manner. This paradigm shift not only sets new benchmarks in AI development but also drives forward the conversation on sustainable and equitable access to AI capabilities across diverse sectors globally.
Democratization and Open Source Impact
The emergence of open-source AI models like DeepSeek R1 is reshaping the landscape of artificial intelligence by significantly lowering the barriers to entry for advanced technology. This democratization is highlighted by DeepSeek R1's ability to rival expensive, proprietary models like OpenAI's o1 while operating at a fraction of the cost. The reliance on reinforcement learning instead of traditional supervised fine-tuning has not only reduced development expenses but also promoted independent reasoning capabilities, challenging the status quo within the AI community.
DeepSeek R1 exemplifies how open-source initiatives are decentralizing access to cutting-edge AI technologies, thereby empowering smaller companies and researchers who previously might have been sidelined in a high-cost environment dominated by big tech firms. Furthermore, this approach has ignited a competitive spirit among open-source developers, encouraging innovation and broader participation in AI advancements.
However, the open-source movement is not without its challenges. Concerns about data biases and the implications of regional censorships, particularly within the context of global usage, pose potential ethical dilemmas. Moreover, the increased accessibility to AI capabilities necessitates the establishment of new ethical guidelines to mitigate risks associated with misuse or unintended consequences of powerful AI systems.
The ripple effects of open-source AI democratization extend into economic, geopolitical, and social realms. Economically, reduced AI development costs could disrupt traditional market dynamics, offering smaller players a chance to innovate and compete. Geopolitically, as models like DeepSeek gain traction, countries may reconsider their AI strategies and regulations, potentially altering the global balance of technology leadership. Socially, the accessibility of AI tools could accelerate automation, transforming industries but also raising questions about employment and ethical use.
In conclusion, the impact of democratization through open-source AI models like DeepSeek R1 is profound, setting the stage for more inclusive innovation and a diversification of AI contributors. As these models continue to evolve, so too must the frameworks that govern them, ensuring they align with the broader societal values of safety, fairness, and responsibility.
Learn to use AI like a Pro
Get the latest AI workflows to boost your productivity and business performance, delivered weekly by expert consultants. Enjoy step-by-step guides, weekly Q&A sessions, and full access to our AI workflow archive.
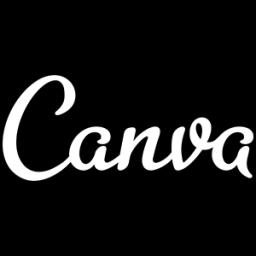
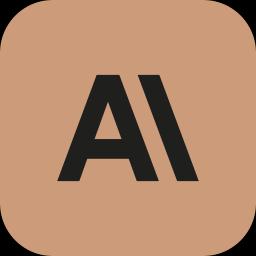
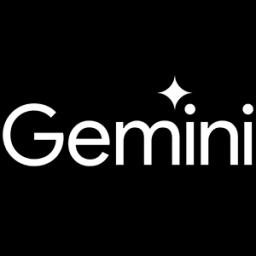
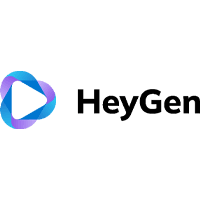
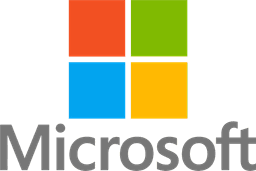
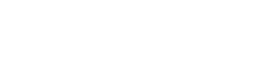
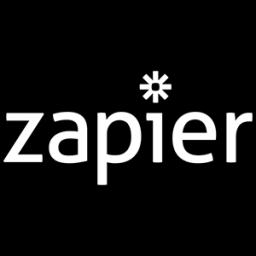
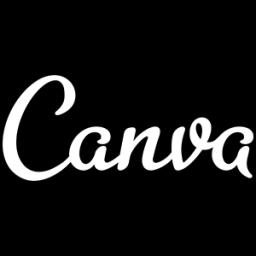
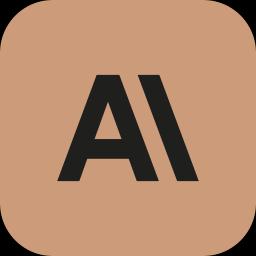
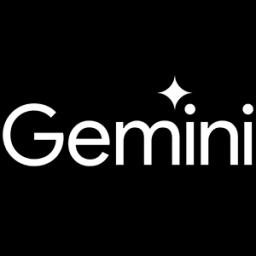
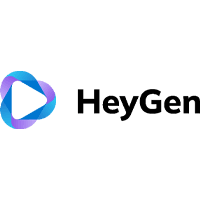
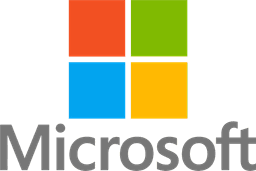
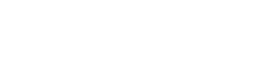
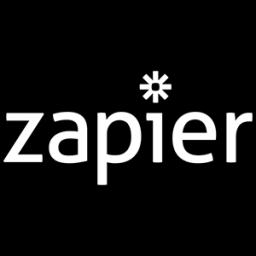
Comparison with Competitors
DeepSeek R1 has set a new benchmark in AI with its open-source model matching competitors like OpenAI’s model in performance, while being remarkably cost-effective. This breakthrough is largely credited to its innovative use of reinforcement learning (RL), which starkly contrasts the industry-standard method of supervised fine-tuning (SFT) that other models like OpenAI conventionally employ. The minimal use of SFT not only enhances the model’s ability to independently reason and prioritize tasks but also slashes training expenses to a fraction of competitors’ costs.
Despite its cost efficiency and high performance, DeepSeek R1 faces significant competition from other open-source AI models, notably Meta’s Llama and the Mistral, which are renowned in the industry for their robustness and adaptability. These models, like DeepSeek, leverage the democratization of AI technology, making advanced capabilities accessible to a broader audience without the associated high costs.
DeepSeek R1’s open-source nature stands as both a strength and a vulnerability when compared with competitors. On one hand, it broadens access to AI technology, fostering innovation and inclusivity among smaller players in the environment that were previously sidelined due to budget constraints. On the other hand, it invites scrutiny over potential security vulnerabilities and bias risks that open-source models face, an issue less prevalent in proprietary counterparts from companies like Google or OpenAI.
The release of such a cost-efficient, high-performing model has provoked a spectrum of reactions within the industry. While there is enthusiasm about challenging the economic status quo of AI deployments—which traditionally demand considerable investments—there are concerns regarding regulatory compliance and ethical considerations, especially under regional legislation influences like that of China.
Looking forward, the impact of DeepSeek R1 could reshape AI market dynamics dramatically. Lower barriers to entry may spur widespread adoption of advanced AI systems across industries, destabilizing established market players who rely heavily on proprietary models. Additionally, the emphasis on reinforcement learning could herald a shift in AI development, potentially igniting a trend that moves away from traditional models that require extensive supervised fine-tuning.
Industry Impact and Disruption
The adoption of reinforcement learning (RL) as the primary method for training DeepSeek R1 represents a significant shift in AI development strategies, challenging the established reliance on supervised fine-tuning. This innovative approach not only reduces operational costs but also leads to comparable performance against industry leaders like OpenAI. By achieving such levels of efficiency and capability at a fraction of the cost, DeepSeek R1 disrupts the preconceived notions about the financial and technological requirements for developing state-of-the-art AI models.
Learn to use AI like a Pro
Get the latest AI workflows to boost your productivity and business performance, delivered weekly by expert consultants. Enjoy step-by-step guides, weekly Q&A sessions, and full access to our AI workflow archive.
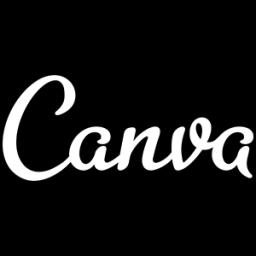
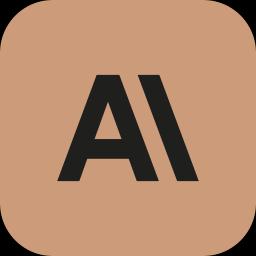
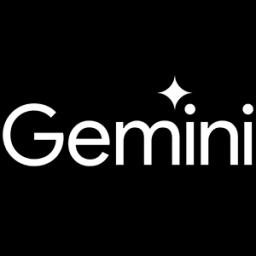
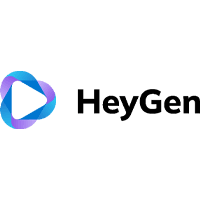
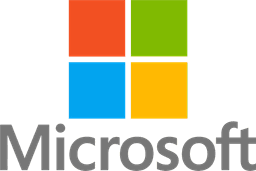
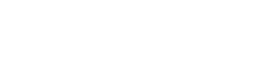
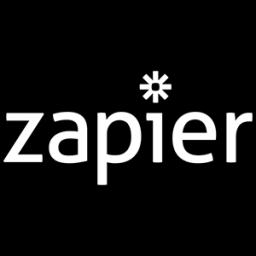
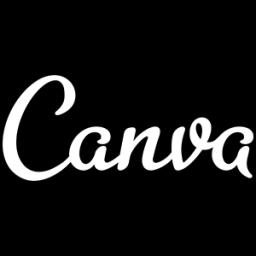
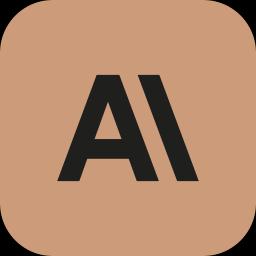
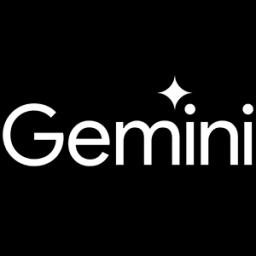
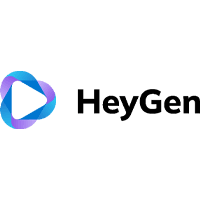
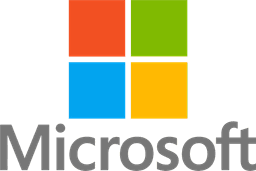
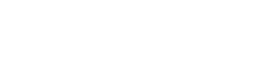
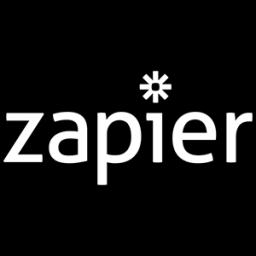
DeepSeek R1's open-source nature is poised to democratize AI technology, making advanced AI capabilities accessible to researchers and smaller organizations who previously could not compete with tech giants. This democratization can foster innovation across various sectors, as smaller players now have the tools to develop custom AI solutions tailored to their specific needs. This shift could effectively alter the competitive landscape of the AI industry, encouraging a more diverse range of participants and potentially leading to unexpected breakthroughs.
Despite its advantages, DeepSeek R1 faces challenges, notably its susceptibility to biases due to the limited transparency of its training data and compliance with local regulations. These concerns highlight the need for ongoing scrutiny and evaluation to ensure ethical use, especially as AI technologies become more entrenched in daily operations across industries worldwide. Addressing these issues is critical to maintaining trust and ensuring the responsible deployment of AI.
The development of DeepSeek R1 underlines the potential for AI growth even in the face of hardware restrictions, as demonstrated by its performance using less powerful Nvidia H800 chips. This capability suggests that significant AI advancements can occur without relying on the most advanced hardware, thus reducing barriers to entry for AI developers and opening possibilities for innovation in regions with restricted technology access.
Furthermore, the emergence of DeepSeek R1 raises important geopolitical questions regarding AI leadership, particularly between the US and China, with implications for international collaboration and competition. As AI models increasingly align with regional regulations, they may redefine global deployment strategies, emphasizing the importance of developing universally applicable ethical frameworks.
Expert Opinions on DeepSeek R1
Alex Zhavoronkov, CEO of Insilico Medicine, believes that DeepSeek R1's emphasis on reinforcement learning (RL) provides it with a distinct edge over conventional supervised approaches. He argues that RL promotes a chain-of-thought reasoning that is integral for capabilities such as self-verification and error correction. This approach, he suggests, is more aligned with organic learning processes as opposed to the more rigid frameworks provided by supervised fine-tuning.
AI specialists at MarktechPost commend DeepSeek R1 for its cost-effectiveness, highlighting the substantial reduction in training costs compared to OpenAI's models. They attribute this cost efficiency to its unique RL-based training methods, which while maintaining high performance levels across various benchmarks, remains affordable and accessible.
Learn to use AI like a Pro
Get the latest AI workflows to boost your productivity and business performance, delivered weekly by expert consultants. Enjoy step-by-step guides, weekly Q&A sessions, and full access to our AI workflow archive.
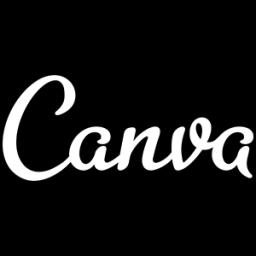
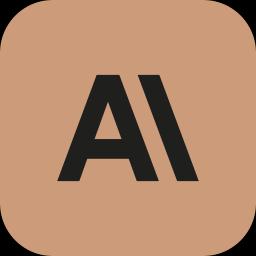
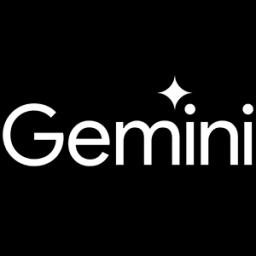
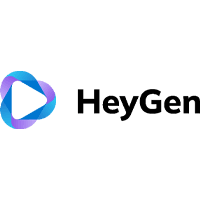
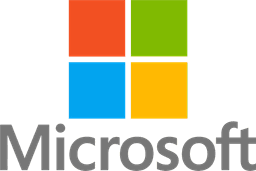
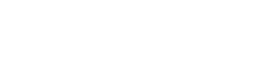
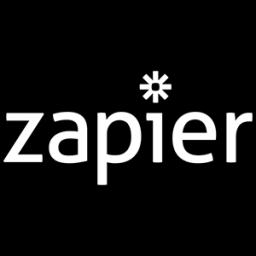
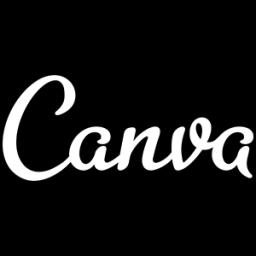
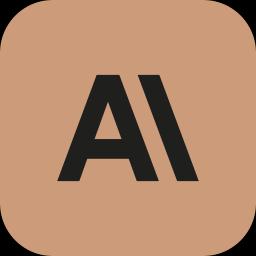
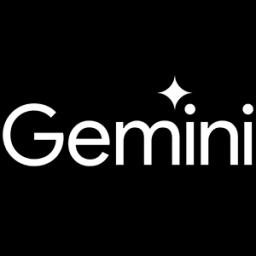
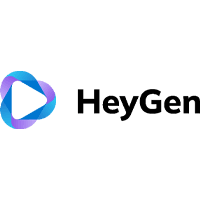
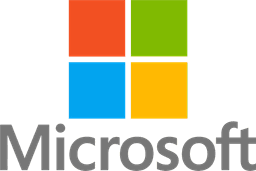
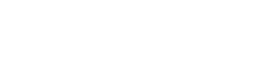
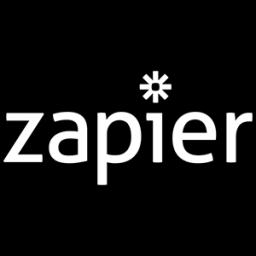
Dr. Sarah Chen, an AI ethics expert, raises potential issues with DeepSeek R1, particularly concerning biases within its training data. She expresses concerns about the limitations of RL in human-like reasoning, emphasizing that while DeepSeek R1 can simulate decision-making processes, it lacks genuine understanding and consciousness.
Industry analysts from RD World highlight the potential democratizing effect DeepSeek R1's open-source nature could have. They suggest that by making advanced AI capabilities more accessible, smaller enterprises and researchers who typically face resource constraints stand to benefit, potentially disrupting the AI development landscape.
Public sentiment around DeepSeek R1's release has been varied. There is significant enthusiasm for its cost-effective performance that rivals leading AI models, as indicated by its rapid uptake on developer platforms like HuggingFace. Nevertheless, concerns about inherent biases and security vulnerabilities remind of the ongoing debates around open-source software and its implications.
Looking ahead, DeepSeek R1's approach could drive economic impacts, notably among small to medium enterprises that traditionally struggle with the high costs of AI model development. By drastically reducing costs, it sets a precedent for AI model affordability that could shift market dynamics considerably.
Public Reactions and Analysis
The unveiling of DeepSeek R1, an open-source AI model developed using reinforcement learning (RL), has sparked considerable interest and debate within the AI community and beyond. On the positive side, a significant portion of the public regards DeepSeek R1's ability to match the performance of OpenAI's models at a fraction of the cost as groundbreaking. This cost-effectiveness, achieved by utilizing RL rather than expensive supervised fine-tuning, has gained widespread acclamation across social media platforms and AI forums.
Many developers are particularly enthusiastic about the open-source nature of DeepSeek R1, which has led to over 109,000 downloads on HuggingFace, a prominent platform for AI models. This enthusiasm is fueled by the model's impressive benchmark results, such as a 79.8% score on the AIME 2024 exams, and its superior search functionality compared to competitors. These achievements have generated a buzz around the potential democratization of AI technology, as smaller businesses and independent developers gain access to advanced capabilities once reserved for industry giants like OpenAI.
Learn to use AI like a Pro
Get the latest AI workflows to boost your productivity and business performance, delivered weekly by expert consultants. Enjoy step-by-step guides, weekly Q&A sessions, and full access to our AI workflow archive.
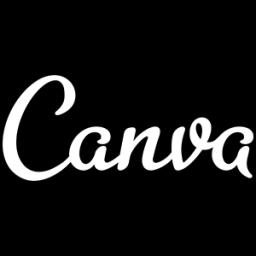
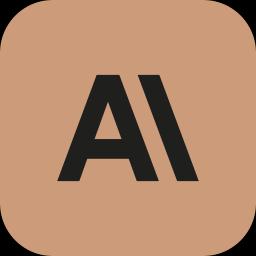
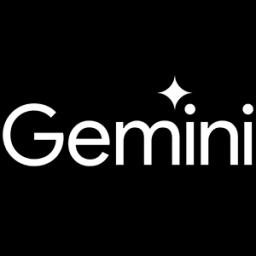
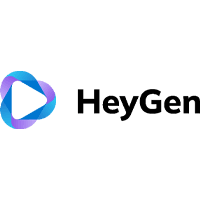
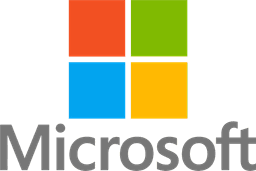
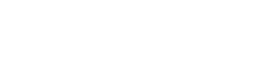
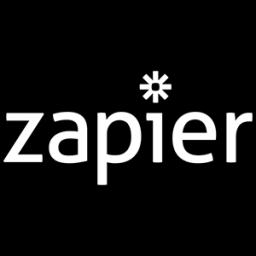
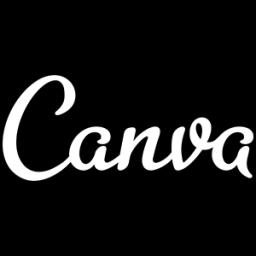
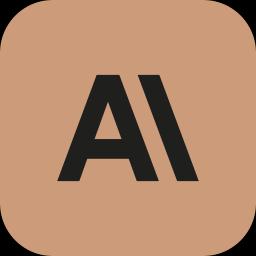
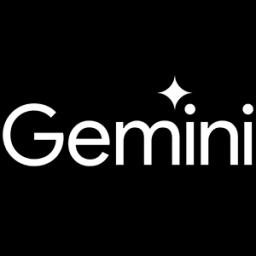
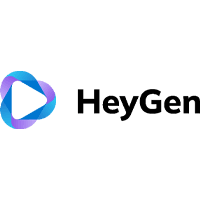
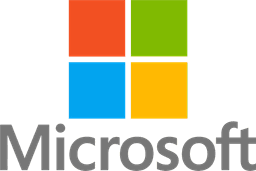
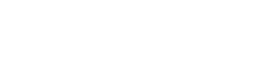
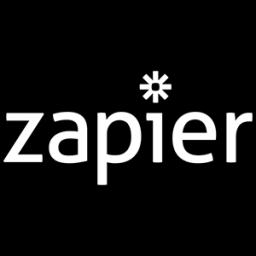
Despite these positive reactions, DeepSeek R1 has faced its share of concerns and criticisms. Some users have raised questions regarding the originality of the model, particularly due to allegations of compliance with Chinese regulations that might bias the AI's training. Critiques have emerged over the model's apparent refusal to address certain political topics, leading to discussions around its potential biases. Furthermore, there are security concerns about the vulnerabilities inherent in open-source models, as noted in discussions on platforms like Hacker News.
Meanwhile, AI ethics researchers express apprehension about the limitations of RL training. While this method enables DeepSeek R1 to simulate human-like reasoning, it lacks genuine consciousness and the ability to evolve understanding over time. These criticisms highlight the ongoing debate about the ethical implications and safety of making such powerful AI capabilities accessible to the masses, sparking discussions on the need for new ethical frameworks to govern the development and deployment of open-source AI models.
Overall, the public's reaction to DeepSeek R1 underscores a broader conversation about the future of AI and its role in society. As the boundaries of AI technology expand, so do the complexities associated with its governance, raising important questions about how to balance innovation with ethical responsibility. The discourse surrounding DeepSeek R1 reflects a pivotal moment in the journey toward more inclusive and widely accessible AI solutions.
Future Implications in AI Industry
The future implications of advancements such as DeepSeek R1 are multifaceted and could have significant impacts across various domains. Economically, the drastic reduction in AI development costs signifies a potential democratization of access to advanced AI capabilities. Smaller businesses and startups could greatly benefit from these reductions, leveling the playing field against larger, well-funded enterprises. Moreover, the disruption of current AI market dynamics is likely, challenging the stranglehold of giants like OpenAI and Google. This may usher in a new era of AI innovations as proprietary models face competition from cost-effective open-source alternatives.
Technologically, DeepSeek R1’s reinforcement learning breakthrough might catalyze a shift in AI development paradigms, moving away from traditional supervised fine-tuning approaches. This shift could lead to accelerated advancements in AI capabilities, even under hardware constraints, as evidenced by DeepSeek's performance using less powerful processing units.
From a geopolitical perspective, this technological stride could alter the global AI leadership landscape, especially concerning the US and China. As these models gain sophistication, there will be heightened attention to compliance with regional regulations, influencing global deployment strategies.
Learn to use AI like a Pro
Get the latest AI workflows to boost your productivity and business performance, delivered weekly by expert consultants. Enjoy step-by-step guides, weekly Q&A sessions, and full access to our AI workflow archive.
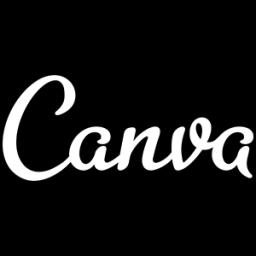
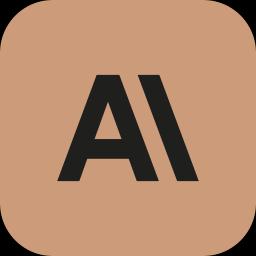
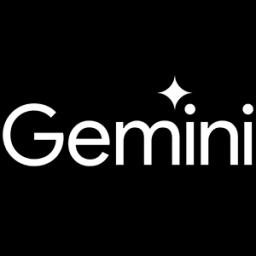
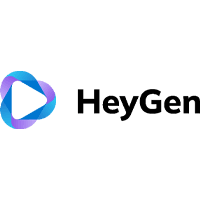
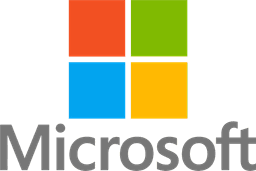
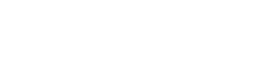
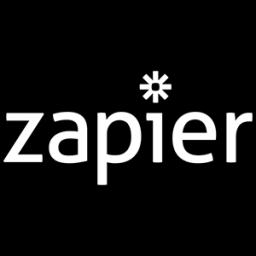
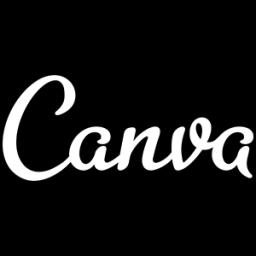
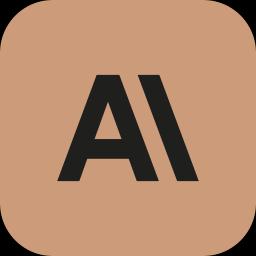
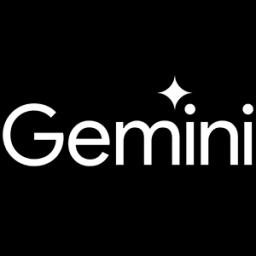
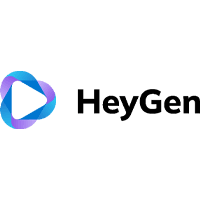
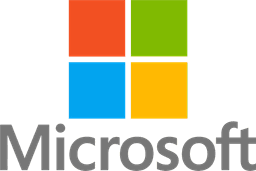
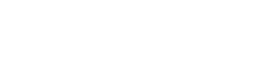
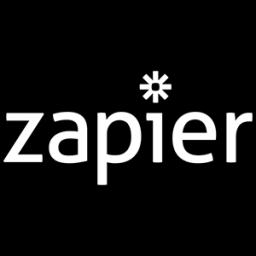
Socially, wider access to AI could fuel widespread automation across sectors, prompting discussions about the ethical ramifications and safety of AI technologies becoming ubiquitous. Developing new ethical guidelines to govern the open-source AI landscape may prove essential to ensure fair and safe utilization. As powerful AI tools become more accessible, concerns around biases, transparency, and accountability will necessitate robust debates and solutions.
Conclusion
In conclusion, DeepSeek R1 represents a significant shift in the AI landscape, challenging conventional wisdom about the economics of AI model development. By achieving performance comparable to OpenAI's o1 at a fraction of the cost, DeepSeek R1 not only proves the viability of pure reinforcement learning but also paves the way for more accessible AI technologies. This shift could democratize AI development, allowing smaller companies and startups to benefit from cutting-edge AI without prohibitive costs.
The open-source nature of the DeepSeek R1 model further amplifies its potential impact, as it invites contributions from the global developer community while also raising questions about security and ethical implications. As the AI market becomes increasingly competitive with new entrants like DeepSeek R1 and other open-source models, traditional proprietary models may need to re-evaluate their strategies in order to maintain their dominance.
DeepSeek R1's approach highlights a critical reevaluation of AI innovation methodologies, emphasizing the importance of efficiency and adaptability in training processes. The success of reinforcement learning in replacing costly supervised fine-tuning methods could inspire a broader industry shift towards more sustainable AI model development practices.
As AI technologies proliferate and become integrated into diverse aspects of society, concerns around bias, ethical guidelines, and geopolitical implications will likely persist. The development of such sophisticated models will necessitate robust, globally recognized frameworks to ensure ethical standards and equitable access across different regions.
Ultimately, DeepSeek R1's rise reflects a transformative period in AI development, characterized by rapid technological advances and a growing emphasis on open-source approaches. This evolution not only disrupts existing market dynamics but also underscores the need for continued dialogue and collaboration among technologists, policymakers, and ethicists to navigate the challenges and opportunities ahead.
Learn to use AI like a Pro
Get the latest AI workflows to boost your productivity and business performance, delivered weekly by expert consultants. Enjoy step-by-step guides, weekly Q&A sessions, and full access to our AI workflow archive.
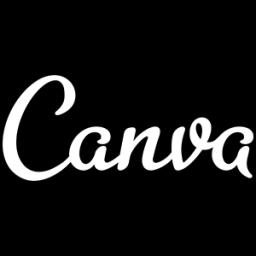
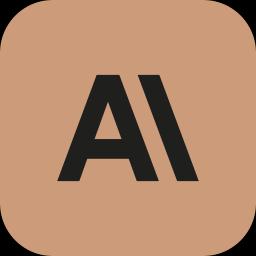
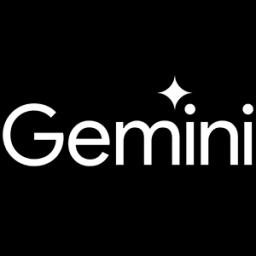
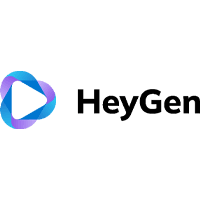
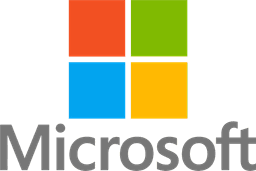
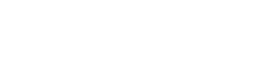
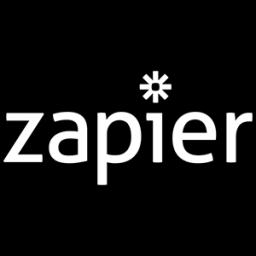
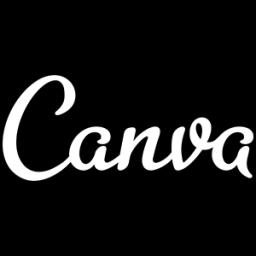
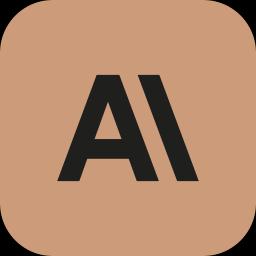
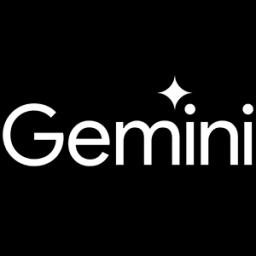
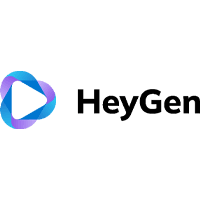
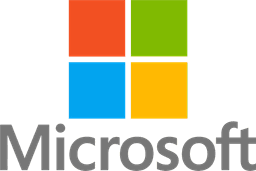
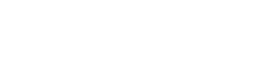
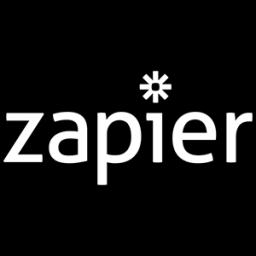