Open Source vs Proprietary AI Showdown
DeepSeek-R1 vs. OpenAI's o1: The Cost-Effective Competitor Cometh!
Last updated:

Edited By
Mackenzie Ferguson
AI Tools Researcher & Implementation Consultant
Introducing DeepSeek-R1, an open-source AI model from China that challenges OpenAI's proprietary o1, boasting comparable performance at just 5% of the cost. By leveraging a unique training methodology known as GRPO for enhanced reasoning, DeepSeek-R1 shows exceptional math and coding proficiency, all while maintaining transparency through its open-source nature. Dive into our exploration of what this means for the AI industry, cost comparisons, and the balance between innovation and safety.
Introduction to DeepSeek-R1 and OpenAI's o1
DeepSeek-R1 is a groundbreaking open-source language model emerging from China, which presents a formidable challenge to OpenAI's proprietary o1 model by offering similar performance at a fraction of the cost. This development marks a significant leap in the landscape of language models, leveraging innovative training methods to enhance reasoning capabilities. By employing a novel three-stage pipeline that integrates cold-start data, reinforcement learning (specifically a method known as GRPO), and supervised fine-tuning, DeepSeek-R1 shifts focus towards reasoning as opposed to traditional supervised learning models.
The cost efficiency of DeepSeek-R1 is particularly notable, achieving operational performance at only 5% of the cost of OpenAI's o1, thanks primarily to its resource-efficient architecture and its open-source model. This allows for broader accessibility and could potentially disrupt existing pricing structures in the industry.
Learn to use AI like a Pro
Get the latest AI workflows to boost your productivity and business performance, delivered weekly by expert consultants. Enjoy step-by-step guides, weekly Q&A sessions, and full access to our AI workflow archive.
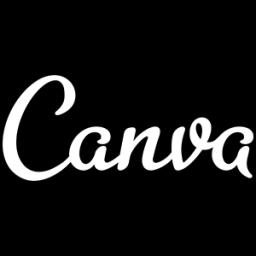
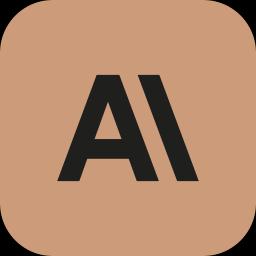
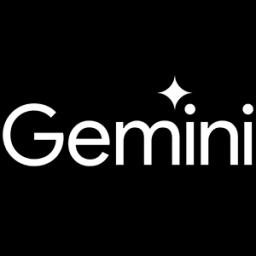
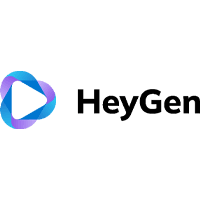
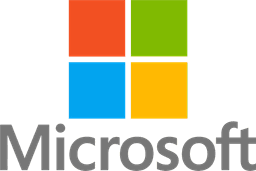
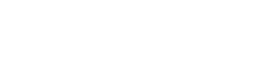
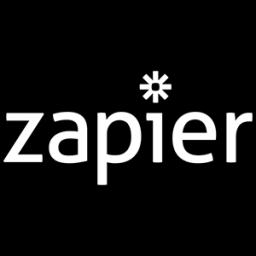
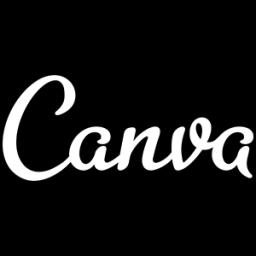
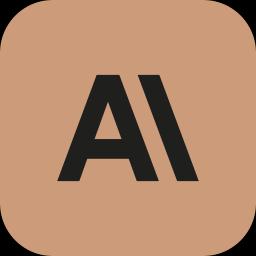
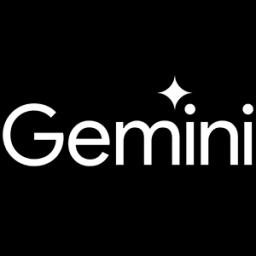
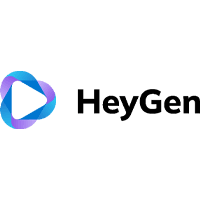
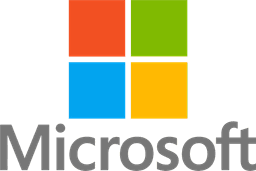
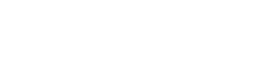
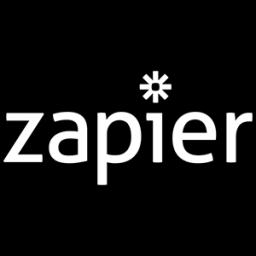
While DeepSeek-R1 is highly cost-effective and specialized in particular tasks such as math and coding (as evidenced by superior performance in benchmarks like MATH-500 and Codeforces), OpenAI's o1 provides a more comprehensive range of general capabilities along with robust safety protocols. This contrast highlights the ongoing trade-offs between open-source and proprietary AI development: transparency and customization against comprehensive testing and security.
Training Innovations in DeepSeek-R1
DeepSeek-R1, an open-source language model from China, presents a formidable challenge to OpenAI's proprietary o1 model by delivering comparable performance at significantly reduced costs. The model operates at approximately five percent of o1’s cost, largely due to its efficient architecture and the open-source nature, which allows broader accessibility and customization. This cost-effectiveness is critical in democratizing access to advanced AI technologies, enabling smaller businesses and developers to leverage such capabilities without prohibitive expenses.
One of the key innovations in DeepSeek-R1’s training methodology is the use of a three-stage pipeline that combines initial data collection (cold-start), reinforcement learning using Generalized Reinforcement Policy Optimization (GRPO), and supervised fine-tuning. This approach prioritizes reasoning and cognitive capabilities over traditional supervised learning methods. This innovative process has led to remarkable performance in mathematical and coding benchmarks, scoring 97.3% on MATH-500 and gaining an Elo rating of 2029 on Codeforces, exhibiting its prowess in complex reasoning tasks.
Comparatively, while DeepSeek-R1 displays excellent cost-performance efficiency and specialized task handling, OpenAI’s o1 model maintains advantages in safety protocols and broader general capabilities. The transparency offered by DeepSeek-R1's open-source code contrasts with o1's proprietary approach, reflecting different priorities in development philosophies between open-source initiatives and traditional proprietary models.
Learn to use AI like a Pro
Get the latest AI workflows to boost your productivity and business performance, delivered weekly by expert consultants. Enjoy step-by-step guides, weekly Q&A sessions, and full access to our AI workflow archive.
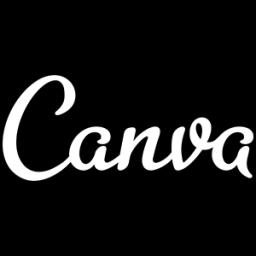
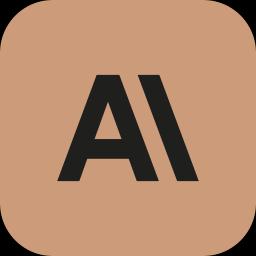
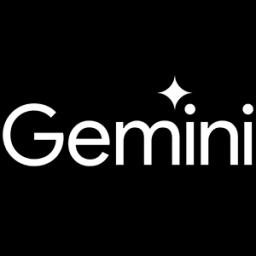
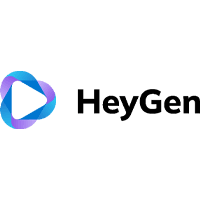
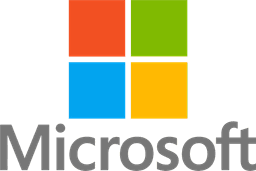
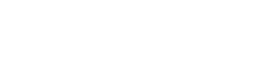
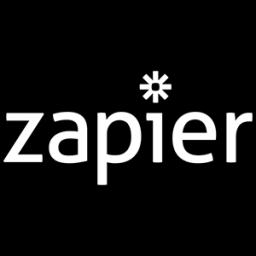
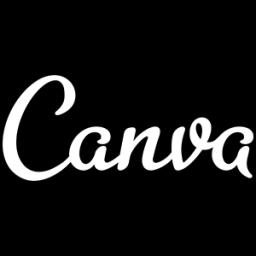
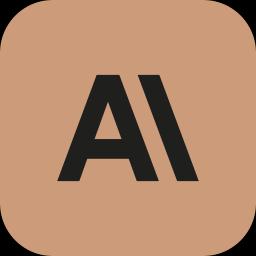
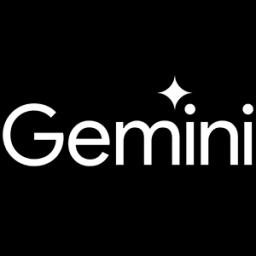
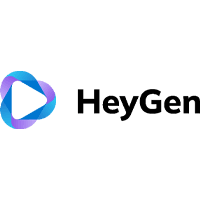
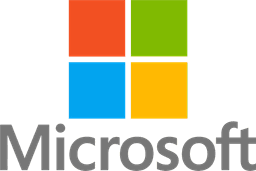
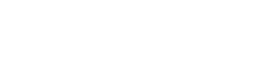
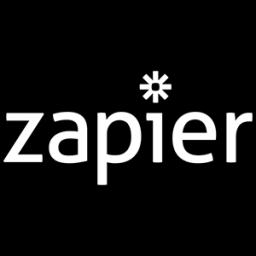
The implications of DeepSeek-R1's development are profound for the AI field, signaling a shift towards open-source models competing at high levels with their proprietary counterparts. Not only does this challenge traditional cost structures, but it also raises important discussions about the balance between innovation, accessibility, and the risks associated with deploying such transformative technologies on a wider scale.
AI industry experts like Dr. Sarah Chen and Prof. James Miller have praised DeepSeek-R1's approach as a game changer for AI democratization, highlighting its efficient use of resources to achieve high performance without depending on extensive computational power. Meanwhile, Dr. Michael Chang urges a balanced evaluation of the model’s capabilities across varied benchmarks, emphasizing the need for continuous assessment of its knowledge scope and reasoning skills relative to other models.
In the public sphere, reactions to DeepSeek-R1 are mixed. Some celebrate its cost-effectiveness and the potential for open-source models to democratize AI access, while others express concerns over security vulnerabilities and compliance with regional regulations. The model’s novel design and training methodology have sparked active discussions across tech forums about its application potential and the broader impact on AI industry practices.
Cost Efficiency Comparison: DeepSeek-R1 vs OpenAI's o1
In the rapidly evolving world of artificial intelligence, cost efficiency has emerged as a critical factor influencing the deployment and accessibility of AI models. The recent introduction of DeepSeek-R1, an open-source language model from China, marks a significant advancement in this domain. It challenges the proprietary o1 model developed by OpenAI, offering comparable performance while drastically reducing operational costs by approximately 95%. This stark financial contrast highlights the potential of open-source models to democratize advanced AI capabilities and to make them accessible to a wider audience, including smaller businesses and organizations that were previously unable to afford such technology.
DeepSeek-R1's cost-effectiveness can be largely attributed to its innovative approach to training. It employs a three-stage pipeline that integrates cold-start data, reinforcement learning through Gradient-based Reinforcement Policy Optimization (GRPO), and supervised fine-tuning. This strategy focuses heavily on improving reasoning capabilities, setting it apart from traditional supervised learning approaches that often prioritize computational power. This method not only contributes to the model's lower costs but also enhances its performance in specific tasks, such as mathematics and coding, proven by its high benchmark scores in MATH-500 and Codeforces competitions.
The comparison between DeepSeek-R1 and OpenAI's o1 also underscores important trade-offs. While DeepSeek-R1 is celebrated for its affordability and specialized task performance, o1 retains certain advantages, notably in its robust safety protocols and broader general capabilities. DeepSeek-R1's transparency, being open-source, allows for greater scrutiny and community-driven improvement, yet OpenAI's proprietary o1 model can leverage its comprehensive testing and safety measures to maintain high standards in deployment. These differences highlight the complex decisions organizations must make when selecting AI models, balancing cost with capability and security.
Learn to use AI like a Pro
Get the latest AI workflows to boost your productivity and business performance, delivered weekly by expert consultants. Enjoy step-by-step guides, weekly Q&A sessions, and full access to our AI workflow archive.
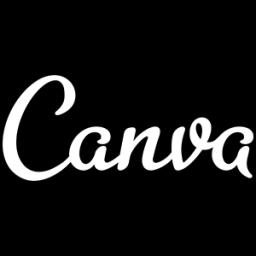
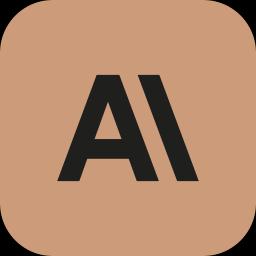
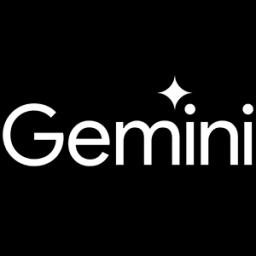
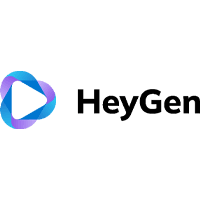
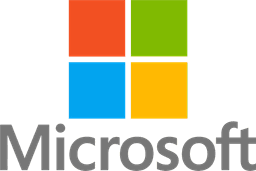
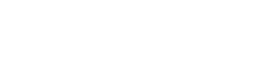
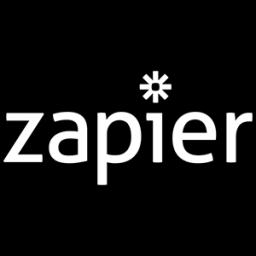
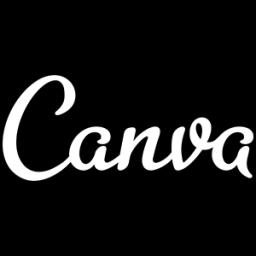
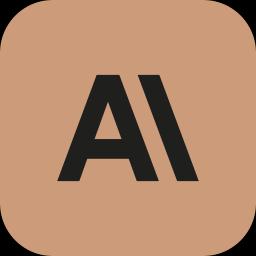
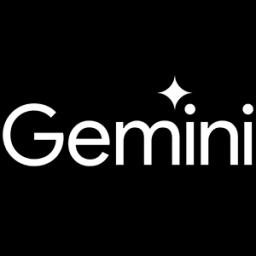
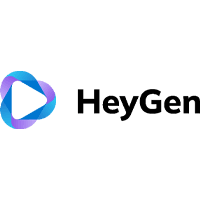
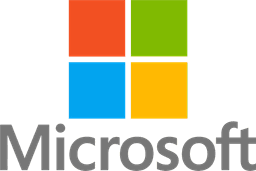
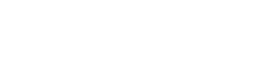
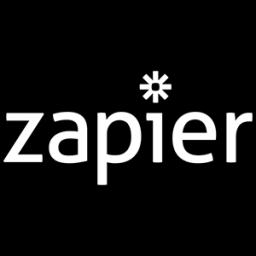
Furthermore, the emergence of DeepSeek-R1 embodies a broader movement towards open-source AI and its implications on future development. This model signifies a pivotal moment where open-source platforms are not only competing with but potentially outpacing proprietary models in cost-efficiency. Such advancements raise pivotal questions about the future of AI innovation, accessibility, and safety, prompting industry players to reconsider existing cost structures and value propositions to stay competitive.
The impact of DeepSeek-R1 extends beyond just cost comparison; it is indicative of potential shifts in AI development paradigms. The success of this open-source model could accelerate the transformation of industry practices, encouraging hybrid development models that blend proprietary and open-source elements. This would foster more collaborative environments for AI research and development, potentially leading to faster innovation cycles and a more diversified AI landscape. As both regulatory and market conditions evolve, DeepSeek-R1's introduction prompts a reevaluation of how AI technologies are created, distributed, and governed across global platforms.
Performance Benchmarks of DeepSeek-R1
DeepSeek-R1, an emergent open-source language model from China, represents a pivotal challenge to the established proprietary AI systems. This innovative model has been crafted using a unique three-stage training pipeline that combines cold-start data collection, reinforcement learning with GRPO techniques, and supervised fine-tuning. This approach has honed DeepSeek-R1's reasoning capabilities to a level competitive with top-tier proprietary models, all while operating at a mere fraction of their cost. This positions DeepSeek-R1 not just as an affordable alternative, but as a substantial contender in advanced AI performance.
Among DeepSeek-R1's most notable attributes is its stellar cost efficiency. When juxtaposed to OpenAI’s proprietary o1 model, DeepSeek-R1 offers comparable performance metrics in benchmarks like MATH-500 and Codeforces, but at approximately 5% of the cost. Without sacrificing quality, this dramatic cost reduction transforms DeepSeek-R1 into an extremely attractive solution for diverse AI applications, especially when budget constraints are paramount.
DeepSeek-R1's open-source nature provides an unparalleled level of transparency, especially relevant in AI's rapidly evolving landscape. Users and researchers benefit from its accessible weights under an MIT license, enabling detailed scrutiny and fostering collaborative enhancements. This shift could trigger a democratization wave in AI research, where insights and advancements are not hampered by proprietary restrictions, but rather propelled by collective knowledge.
Despite its many strengths, DeepSeek-R1 is not without challenges. Concerns persist regarding security and compliance, particularly amidst rigorous Chinese censorship regulations. Although its capabilities in mathematical and software engineering tasks are commendable, the model's general knowledge and accuracy in factual reasoning are areas where it doesn't completely outshine its proprietary counterparts. Further, there's an ongoing debate about the originality embedded within its architecture and training methodologies.
Learn to use AI like a Pro
Get the latest AI workflows to boost your productivity and business performance, delivered weekly by expert consultants. Enjoy step-by-step guides, weekly Q&A sessions, and full access to our AI workflow archive.
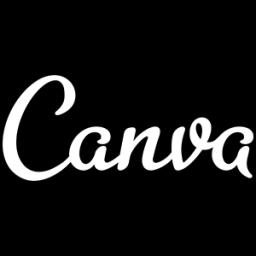
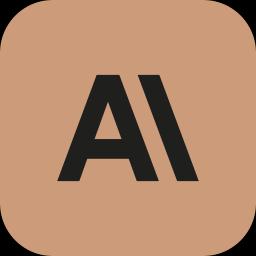
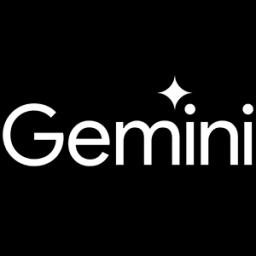
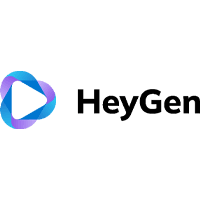
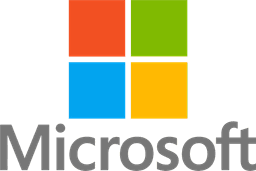
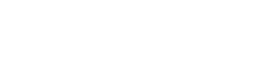
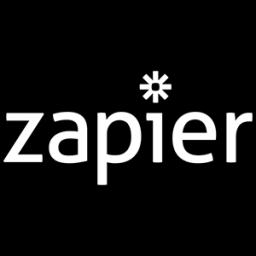
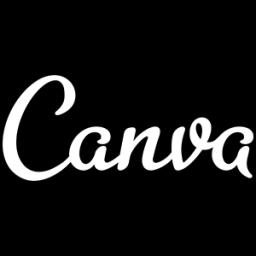
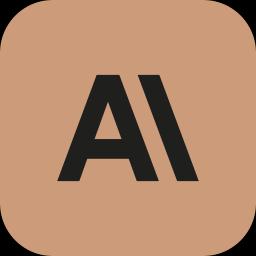
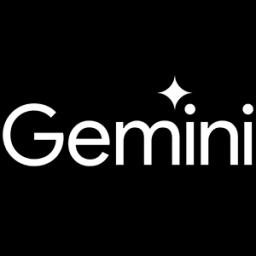
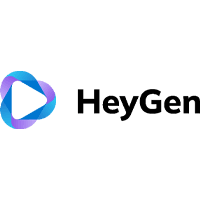
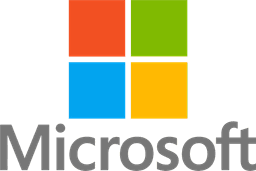
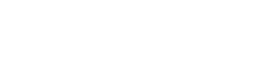
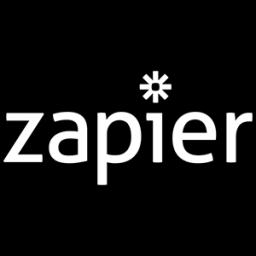
The emergence of DeepSeek-R1 stands as a testament to the potential of open-source models to rival proprietary incumbents. This breakthrough has implications for the entire field of AI, contesting the prevalent narrative that superior AI capabilities necessitate exorbitant costs. While the model encourages enthusiasm and optimism for cost-effective AI solutions, it concurrently invites scrutiny on regulatory and ethical fronts, ensuring its deployment is aligned with safety and fairness.
Analysis of Model Trade-offs: Open-Source vs Proprietary
The landscape of AI model development is seeing significant shifts as open-source models like DeepSeek-R1 challenge proprietary models such as OpenAI's o1. DeepSeek-R1, emerging from China, offers a comparable performance at a fraction of the cost of traditional models, showcasing the potential to democratize access to advanced AI technology. The key differentiator is its innovative use of reinforcement learning, specifically GRPO, which enhances reasoning capabilities and positions it as a more cost-effective alternative in the rapidly evolving domain of AI.
DeepSeek-R1's training methodology underscores its uniqueness. It adopts a three-stage pipeline consisting of cold-start data, reinforcement learning via GRPO, and supervised fine-tuning. This approach emphasizes reasoning abilities which are crucial for complex problem-solving, distinguishing it from traditional supervised learning models. These capabilities are reflected in its impressive performance on benchmarks such as MATH-500 and Codeforces, making it a strong contender in the open-source AI landscape.
Economic considerations further highlight DeepSeek-R1's advantages. Its operation cost is significantly lower than proprietary models like o1, attributed to its efficient architecture and the benefits inherent to open-source frameworks. This has profound implications for the AI industry, where cost efficiency can directly influence the adoption rate across various sectors. By lowering the cost of AI deployment, smaller enterprises can now harness cutting-edge AI capabilities that were previously unattainable due to financial limitations.
The trade-offs between DeepSeek-R1 and its proprietary counterparts like o1 revolve around transparency and safety. While DeepSeek-R1 is celebrated for its open-source nature offering transparency and fostering innovation, o1 retains an edge with stronger safety protocols and broader general capabilities. This contrast highlights the ongoing debate between open-source accessibility and the comprehensive testing and safety benefits often associated with proprietary models.
Public and expert opinions on DeepSeek-R1 are mixed but largely positive. Enthusiasts from tech communities commend its cost-effectiveness and benchmark performances, while some experts emphasize the pivotal role it could play in AI democratization. Conversely, concerns about the model's security and compliance with regulatory standards persist, reflecting the broader challenges faced by open-source AI initiatives.
Learn to use AI like a Pro
Get the latest AI workflows to boost your productivity and business performance, delivered weekly by expert consultants. Enjoy step-by-step guides, weekly Q&A sessions, and full access to our AI workflow archive.
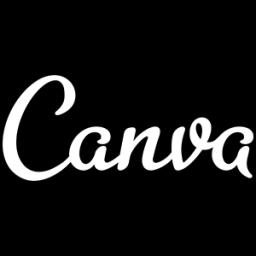
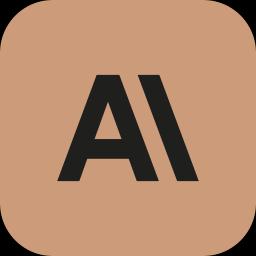
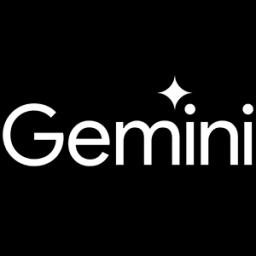
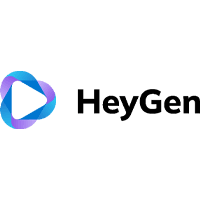
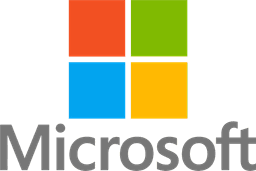
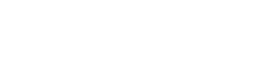
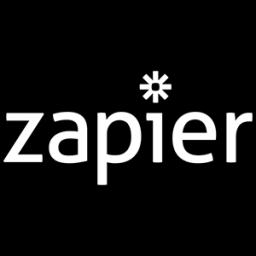
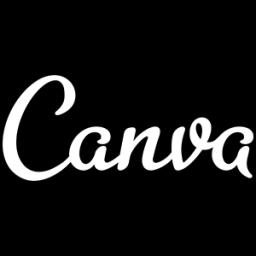
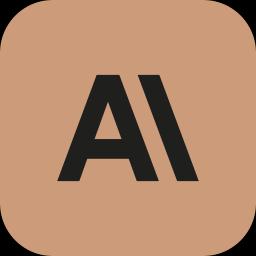
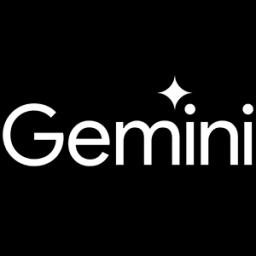
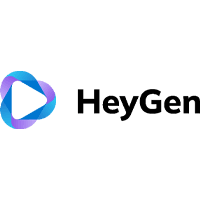
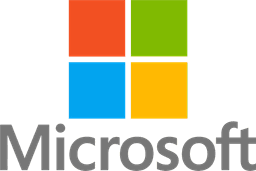
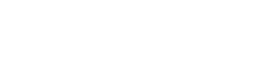
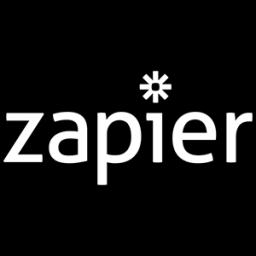
Looking forward, the emergence of models like DeepSeek-R1 suggests a potential shift towards hybrid development paradigms that balance the benefits of open-source and proprietary approaches. This trend could accelerate AI innovation, reshape industry practices, and influence regulatory frameworks globally, fostering a more inclusive and competitive AI ecosystem. The impact of such developments extends beyond the technological realm, potentially transforming socio-economic opportunities, particularly in regions poised to leverage accessible AI tools for growth.
Implications for AI Development and Democratization
The rise of models like DeepSeek-R1 introduces significant implications for the development and democratization of AI technology. One of the most immediate impacts is the potential for open-source AI models to rival their proprietary counterparts. DeepSeek-R1, for instance, matches the performance of OpenAI's o1 at a fraction of the cost, highlighting the ability of resource-efficient models to deliver high performance without the heavy financial burden associated with proprietary systems. This shift could democratize AI access, making advanced capabilities available to a wider array of users and developers globally.
Moreover, DeepSeek-R1's innovative use of reinforcement learning (GRPO) demonstrates that alternative training methodologies can enhance AI capabilities, specifically in areas such as reasoning and problem-solving. This methodological diversity enriches the AI landscape, paving the way for models that prioritize efficient learning strategies over sheer computational power. The emphasis on open-source frameworks also promotes greater transparency and collaborative innovation, enabling researchers and developers to build upon existing models and accelerate technological advancements.
However, the democratization of AI also brings forth challenges regarding safety, regulation, and ethical considerations. The EU's AI Act, for example, underscores the importance of mandatory safety testing and bias assessments, which must be integrated into both open-source and proprietary models. This regulatory focus ensures that the proliferation of AI technologies does not compromise user safety or ethical standards, thus balancing accessibility with responsibility.
Furthermore, the competition between Chinese and Western AI models, such as DeepSeek-R1 and OpenAI's o1, could enhance innovation through a more dynamic and competitive environment. This cross-cultural technological rivalry might lead to faster innovation cycles, creating diverse and powerful AI solutions tailored to a broad range of applications. However, it also raises international governance questions, as the balance between accessibility, innovation, and security becomes increasingly delicate in a globally interconnected technology landscape.
Recent Developments in the LLM Landscape
In early 2025, the launch of DeepSeek-R1, a new open-source large language model (LLM) from China, has stirred the AI industry by challenging OpenAI's proprietary o1 model with a performance that matches 95% of o1’s capabilities while operating at a mere 5% of the cost. This model, developed by DeepSeek AI, leverages an innovative training methodology that involves a three-stage pipeline: cold-start data acquisition, reinforcement learning (specifically through Guaranteed Relevance Policy Optimization or GRPO), and supervised fine-tuning. These strategies collectively aim to enhance reasoning abilities over traditional supervised learning methods.
Learn to use AI like a Pro
Get the latest AI workflows to boost your productivity and business performance, delivered weekly by expert consultants. Enjoy step-by-step guides, weekly Q&A sessions, and full access to our AI workflow archive.
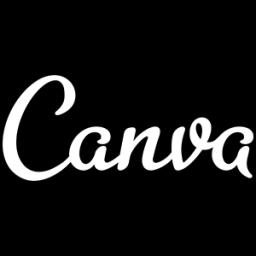
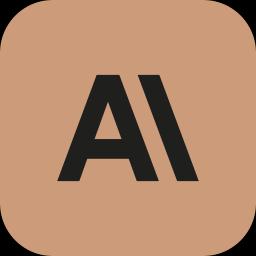
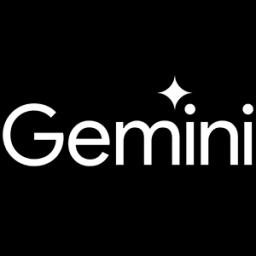
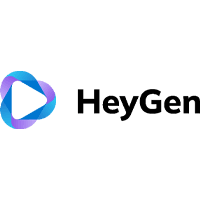
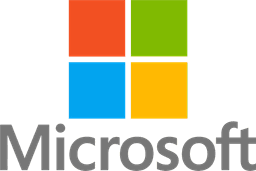
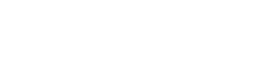
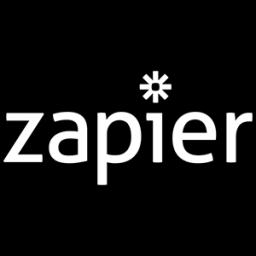
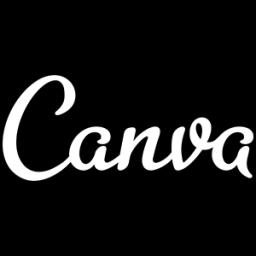
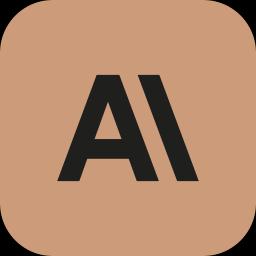
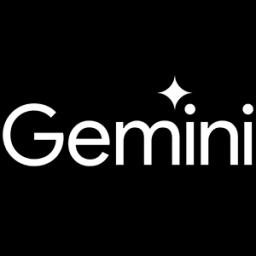
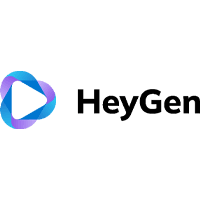
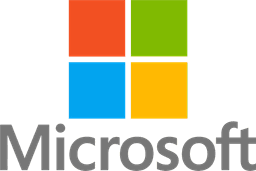
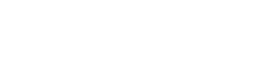
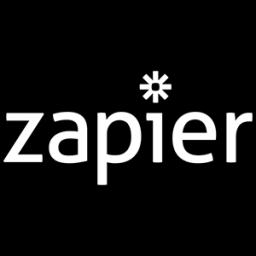
The introduction of DeepSeek-R1 signifies a pivotal moment in the AI landscape, highlighting the potential of open-source models to rival proprietary giants not just in performance but in cost-effectiveness as well. While cost is a major advantage, DeepSeek-R1’s architecture also plays a role in widening the accessibility of advanced AI technologies, making it feasible for smaller enterprises to exploit these technologies without bearing the traditionally high costs. However, this low-cost advantage does not come without trade-offs.
Comparatively, the o1 model by OpenAI remains a strong contender due to its comprehensive safety protocols and broad general capabilities, factors where DeepSeek-R1 currently needs improvement. Furthermore, the proprietary nature of the o1 model allows OpenAI to maintain a stronghold in extensive testing and deploying rigorous safety measures, aspects that are sometimes challenging for open-source projects to fully embody due to resource constraints. Despite the trade-offs, DeepSeek-R1 brings transparency to the table with its open-weight model under the MIT license, thereby enabling researchers worldwide to access, study, and further innovate with it.
This open-access approach could potentially democratize AI development, setting a precedent for future projects aimed at balancing efficiency, safety, and innovation. Enthusiasm around DeepSeek-R1’s potential for democratization is further fueled by the model’s stellar performances in math and coding benchmarks like MATH-500 and Codeforces, underscoring its specialized capabilities.
However, as DeepSeek-R1 makes waves, it also raises critical questions about security and originality. There are concerns regarding the model's susceptibility to security vulnerabilities, given its open-source nature, and debates about the originality of its architecture and training methods persist among tech communities. Nonetheless, the active discussions and interest in the model indicate its significant impact on the LLM landscape's evolution.
Expert Opinions on DeepSeek-R1's Impact
Dr. Sarah Chen, AI Research Director at Stanford, emphasizes that DeepSeek-R1's performance at a fraction of the cost typically associated with models like OpenAI's o1 is a significant development towards democratizing advanced AI technology. This efficiency underscores the potential for optimized resource allocation to make impactful strides in AI development without relying solely on substantial computational power. Her viewpoint highlights the broader implications for the industry as open-source models like DeepSeek-R1 challenge proprietary systems not just in capability, but in accessibility.
Prof. James Miller of MIT commends the technical prowess demonstrated by DeepSeek-R1, particularly its advanced search functionality. This feature, he notes, ranks closely with leading technologies and signifies a possible shift in training methodologies within the AI industry. Miller's insights suggest that such innovations could influence future AI development strategies, potentially creating a ripple effect that redefines standard practices across various applications.
Learn to use AI like a Pro
Get the latest AI workflows to boost your productivity and business performance, delivered weekly by expert consultants. Enjoy step-by-step guides, weekly Q&A sessions, and full access to our AI workflow archive.
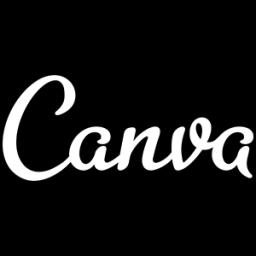
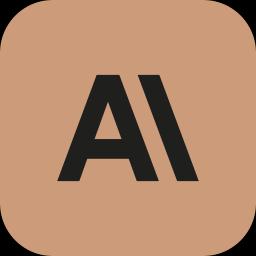
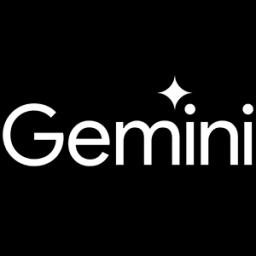
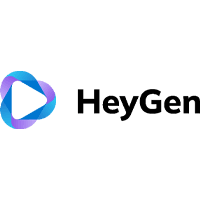
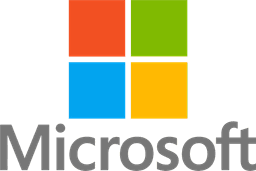
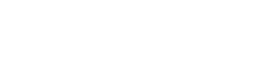
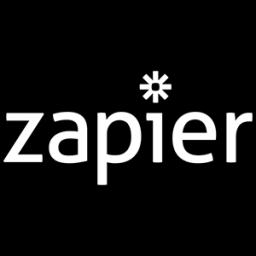
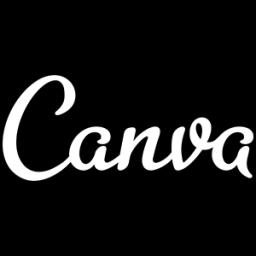
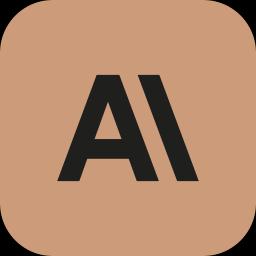
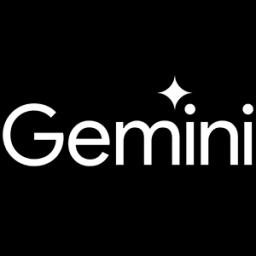
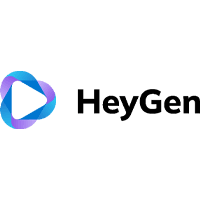
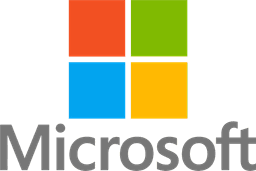
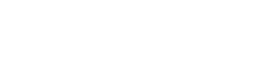
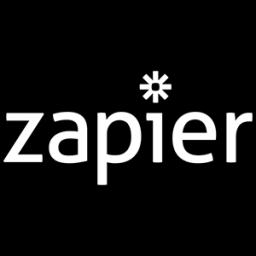
Dr. Elena Rodriguez from Berkeley appreciates the model's open-weight nature under a flexible MIT license, which affords a level of transparency rare in AI research circles. This transparency facilitates opportunities for collaborative advancements and deeper academic inquiry into artificial intelligence. Her observations point towards an era of increased openness and shared progress in AI capabilities as researchers can study and innovate upon the foundations laid by DeepSeek-R1.
Despite the optimism, Dr. Michael Chang of Harvard advises caution, emphasizing the importance of comprehensive evaluation. He notes that while DeepSeek-R1 excels in specific tasks, like mathematical computations and software engineering, it still trails behind in more generalized knowledge domains when compared to other models such as OpenAI's o1. Chang's perspective serves as a reminder of the nuanced balance necessary in evaluating AI effectiveness across diverse performance metrics.
Public Reactions and Community Feedback
The public response to DeepSeek-R1 has been notably divided across social media platforms and tech forums. On one hand, tech communities have shown enthusiastic support for the model’s cost-effectiveness, with many praising its ability to achieve 90-95% of OpenAI's performance at a fraction of the cost. Reddit discussions highlight strong interest in its open-source nature, which many believe has the potential to democratize AI access. Developers, in particular, have applauded DeepSeek-R1's impressive performance in math and coding benchmarks, viewing it as a significant leap in making advanced AI capabilities more accessible and affordable.
However, the model has also faced skepticism and concerns. Users on various platforms have expressed wariness regarding potential security vulnerabilities associated with its open-source design. Significant debate exists about the model's compliance with Chinese censorship regulations, raising questions about its global applicability and safety. Additionally, some community members have questioned the originality of DeepSeek-R1's architecture and training approach, suggesting it may not be as groundbreaking as its proponents claim.
The developer community has had a mixed response to DeepSeek-R1. While there are active discussions on tech forums about implementing the model for various applications, feedback is mixed regarding its performance on simple logical puzzles. Despite excelling in complex reasoning tasks, some developers find its capabilities lacking in more straightforward scenarios. Nonetheless, there is growing interest in its potential for mobile-first applications due to the model's cost advantages, which is seen as a promising opportunity for expanding AI usage in new areas.
Future Economic and Industry Implications
The emergence of DeepSeek-R1, an open-source language learning model from China, signals a pivotal shift in the economic landscape concerning artificial intelligence technologies. By offering comparable performance to OpenAI's proprietary o1 model at a fraction of the cost—95% less as reported—DeepSeek-R1 is poised to democratize AI accessibility significantly. This cost reduction in AI deployment could level the playing field, allowing small to medium enterprises that previously couldn't afford high-end AI solutions to now leverage these advanced capabilities. Such democratization might not only stimulate innovation across varied sectors but could also drive new advances in AI-enabled products and services, all while challenging existing AI pricing models and forcing proprietary platforms to reassess their pricing strategies and value propositions.
Learn to use AI like a Pro
Get the latest AI workflows to boost your productivity and business performance, delivered weekly by expert consultants. Enjoy step-by-step guides, weekly Q&A sessions, and full access to our AI workflow archive.
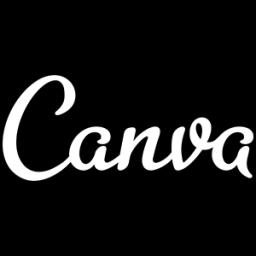
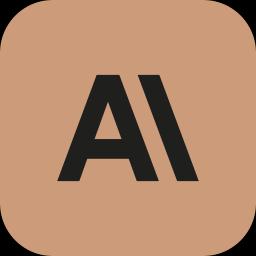
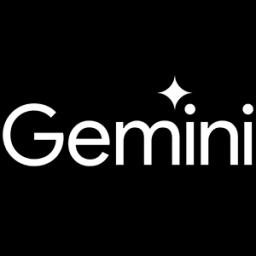
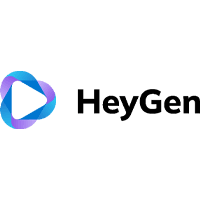
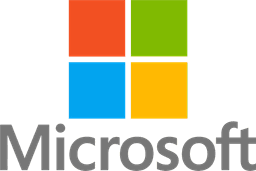
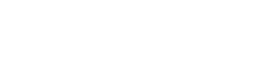
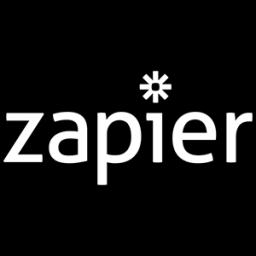
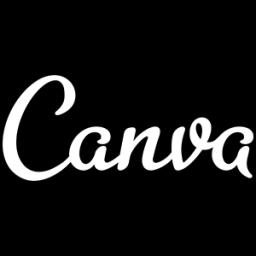
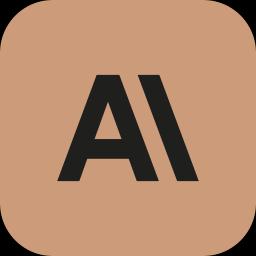
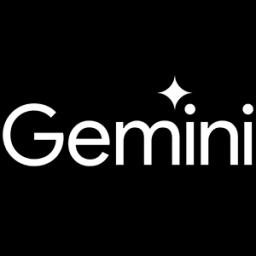
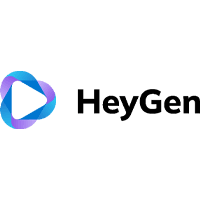
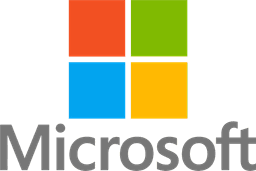
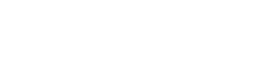
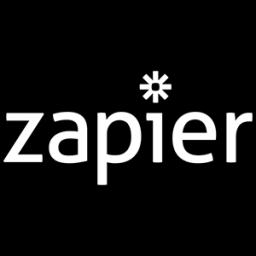
The development of DeepSeek-R1 reflects broader trends of industry transformation. The paradigm shift towards hybrid opensource models, bolstered by the transparency and accessibility offered by open-weight access, quickens collaborative innovation and fosters an environment for rapid development in AI. This transformative approach allows for a confluence of global research talents, catalyzing advancements that could significantly accelerate AI technology development cycles. With Chinese companies like DeepSeek entering direct competition with Western AI titans, the industry may encounter faster innovation cycles, driven by the strategic utilization of cost-effective, open-source technologies.
However, as AI technologies become more widespread, the need for regulatory considerations becomes even more pressing. The rapid proliferation of open-source AI models such as DeepSeek-R1 necessitates international discourse on AI governance, focusing on oversight to ensure security, bias assessment, and adherence to ethical norms. Existing regulatory frameworks might be insufficient to address the unique challenges posed by these cost-effective AI systems, possibly leading to the creation of new guidelines. Balancing the rapid innovation spurred by open-source AI with necessary safety regulations will be pivotal in ensuring that AI technologies are both accessible and safe.
The societal implications of democratized AI technologies are profound. As AI capabilities become more accessible, sectors across the globe stand to benefit from enhanced automation, which could lead to increased operational efficiencies and innovations. There is also a potential for democratizing AI education, allowing developing regions to build knowledge bases and contribute to the global AI discourse. However, alongside these positive changes, there lies the risk of exacerbating the technological divide. Organizations with the infrastructure to safely implement AI solutions may advance significantly, while those rushing to deploy cost-effective solutions without adequate safeguards may face challenges related to technological integration and safety concerns. Thus, ensuring equitability in the adoption of these technologies remains a crucial issue.
Regulatory and Social Impact Considerations
The rise of open-source large language models (LLMs) like DeepSeek-R1 represents both an opportunity and a challenge from a regulatory perspective. One of the main advantages of open-source models is their potential to democratize access to advanced AI technologies, allowing smaller entities to leverage these tools without the exorbitant costs typically associated with proprietary models. This shift could prompt regulatory bodies to develop new frameworks that ensure open-source models are safe and reliable without stifling innovation.
Moreover, the international AI governance landscape might need to adapt to accommodate these cost-efficient, widely accessible systems. This includes addressing potential security vulnerabilities, particularly in models hailing from regions with strict government regulations, such as China. The global nature of AI also raises questions regarding compliance with diverse regulatory standards, necessitating a more unified approach to AI governance.
On the social front, broader access to advanced AI capabilities could accelerate automation and innovation across various sectors, from healthcare to education. However, it also risks exacerbating the technological divide between entities capable of safely implementing these technologies and those that may engage in rushed deployments to gain competitive edges. Managing this balance between innovation, accessibility, and safety will be crucial in ensuring that AI's benefits are distributed equitably.
Learn to use AI like a Pro
Get the latest AI workflows to boost your productivity and business performance, delivered weekly by expert consultants. Enjoy step-by-step guides, weekly Q&A sessions, and full access to our AI workflow archive.
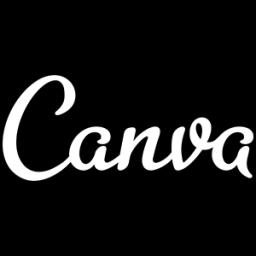
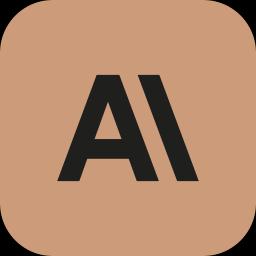
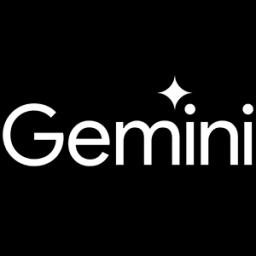
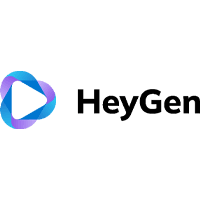
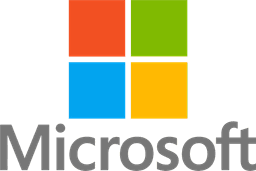
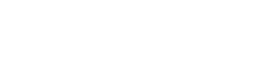
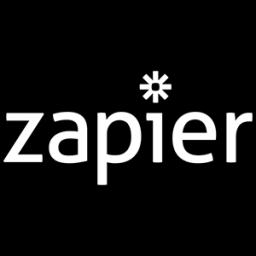
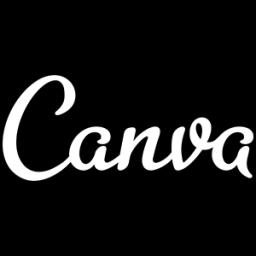
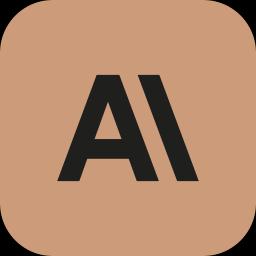
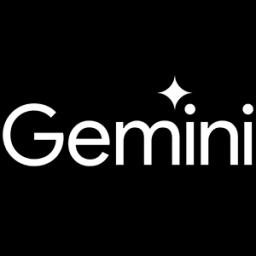
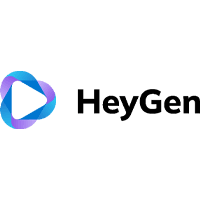
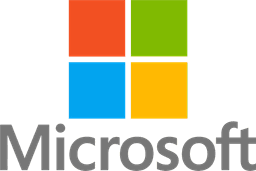
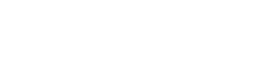
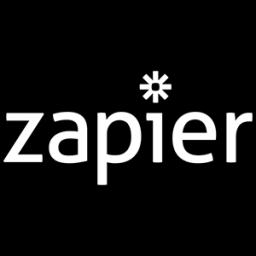
In response to these developments, ongoing discussions about AI regulation are likely to intensify. Policymakers will face the challenge of fostering an environment where AI can flourish while mitigating risks associated with its proliferation. This includes addressing ethical concerns, particularly around bias and fairness, that may arise from AI systems that become increasingly integrated into everyday life. By crafting transparent and adaptable regulatory measures, societies can aim to harness the full potential of AI, maximizing its positive impacts while minimizing potential drawbacks.