AI Innovation on a Budget
DeepSeek's Cost-Crunching AI Revolution: Training for Less!
Last updated:

Edited By
Mackenzie Ferguson
AI Tools Researcher & Implementation Consultant
DeepSeek, a Chinese AI company, stunned the tech world with its groundbreaking process that slashed AI training costs to just $6 million, a tenth of Meta's expenses. By innovating with specialized neural networks and optimizing hardware usage, they've set a new benchmark in affordable AI development. Dive into the features propelling this cost-effective revolution and their potential global impact.
Introduction to DeepSeek's Approach
DeepSeek, a visionary Chinese AI startup, has revolutionized the landscape of artificial intelligence development with its groundbreaking efficiency strategies. In an era where AI training costs are escalating rapidly, DeepSeek offers an innovative contrast by managing to train a sophisticated AI model at a mere fraction of the typical cost. Their approach involves a fascinating integration of cutting-edge methodologies that put them on par with tech giants like Meta, but at significantly reduced expenses. In a detailed evaluation by The Straits Times, it's highlighted that while companies like Meta invest a staggering $60 million for AI training, DeepSeek manages with just $6 million for similar capabilities, underscoring their efficient use of resources (source).
One of the key aspects of DeepSeek's strategy is the implementation of a Mixture of Experts (MoE) architecture. This architecture effectively allocates tasks among specialized neural networks, orchestrated by a coordinating generalist network. Such an arrangement not only heightens performance but also cuts down on the costly inter-GPU communication, traditionally seen as a major expense in AI training (source). Additionally, DeepSeek has innovatively employed 8-bit precision calculations, normally perceived to compromise quality, but they have mitigated this by storing final results in 32-bit precision, ensuring that there is no loss in output accuracy (source).
Learn to use AI like a Pro
Get the latest AI workflows to boost your productivity and business performance, delivered weekly by expert consultants. Enjoy step-by-step guides, weekly Q&A sessions, and full access to our AI workflow archive.
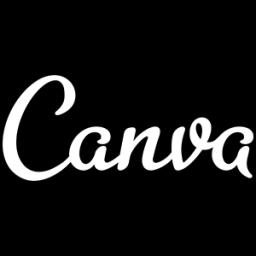
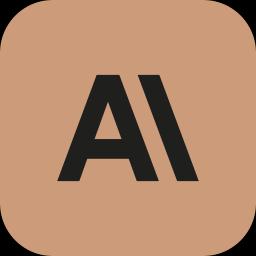
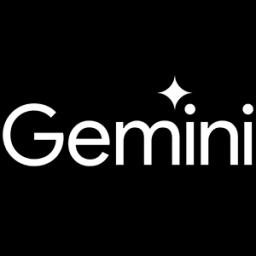
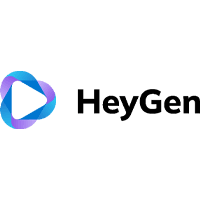
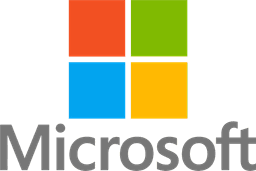
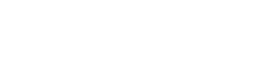
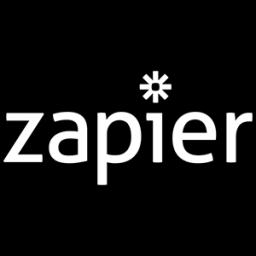
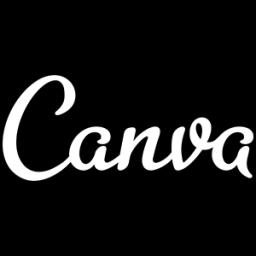
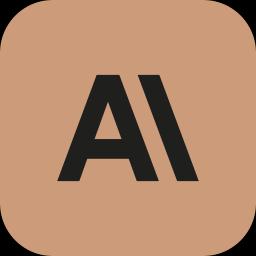
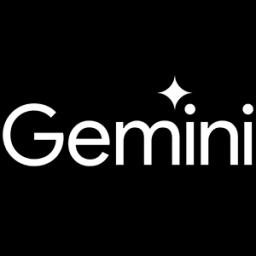
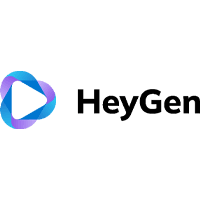
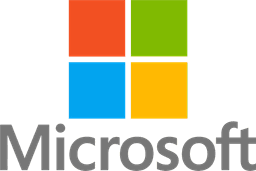
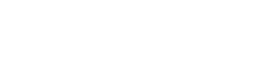
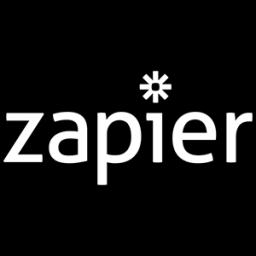
The firm's ability to achieve these efficiencies has had a ripple effect across the AI industry. Not only has it set a precedent for cost-effective AI training, but it has also prompted responses from other notable organizations. For example, French startup Mistral AI has adopted a similar MoE framework, reportedly achieving powerful outputs at a fraction of the cost compared to traditional methods (source). Meanwhile, major players like IBM have similarly started optimizing their AI training processes, resulting in significant reductions in associated costs (source).
Despite the excitement and the industry buzz that DeepSeek's announcements have generated, there are mixed feelings about the real impact of their cost-saving measures. While the immediate reduction in training costs is undeniable, skeptics point out that this $6 million figure only accounts for the final phase of training. Many industry experts, therefore, call for a more comprehensive view of the R&D expenses involved. Debut Infotech suggests that hardware and long-term development costs could be significantly higher, pushing total investments into the hundreds of millions, a view that finds some resonance among critical observers (source).
Innovations Behind the Cost Efficiency
DeepSeek, a Chinese AI startup, has revolutionized cost efficiency in AI development through its pioneering innovations. A standout factor is their Mixture of Experts (MoE) architecture, which intelligently distributes AI tasks among specialized neural networks under the coordination of a generalist system. This approach significantly minimizes the expensive inter-GPU communication that typically escalates costs in traditional AI systems. By effectively aligning the operational tasks, DeepSeek has managed to cut the costs drastically, enabling them to achieve competitive results at a fraction of the expected price [source](https://www.straitstimes.com/business/how-did-deepseek-build-its-ai-with-less-money).
Another critical component in DeepSeek's cost efficiency strategy is their implementation of 8-bit precision calculations, where operations are executed using 8-bit precision and the results are stored in 32-bit precision. This strategic decision ensures that while computational expenses are reduced, the accuracy of the final outcomes remains uncompromised. By prioritizing resource efficiency without sacrificing precision, DeepSeek has set a precedent in achieving high-quality AI model training on a budget that was previously deemed unachievable [source](https://www.straitstimes.com/business/how-did-deepseek-build-its-ai-with-less-money).
Learn to use AI like a Pro
Get the latest AI workflows to boost your productivity and business performance, delivered weekly by expert consultants. Enjoy step-by-step guides, weekly Q&A sessions, and full access to our AI workflow archive.
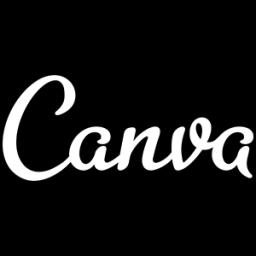
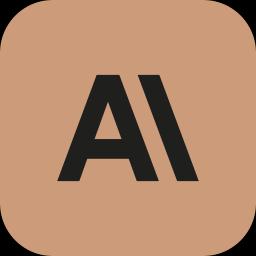
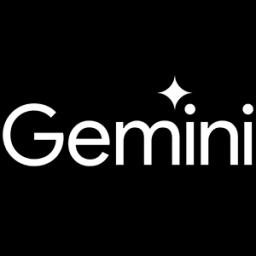
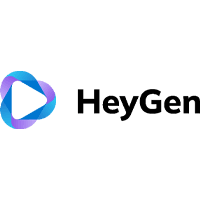
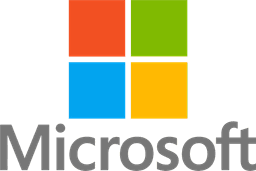
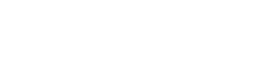
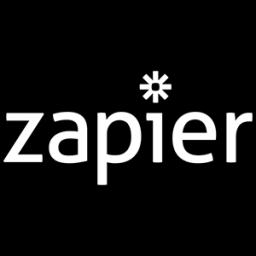
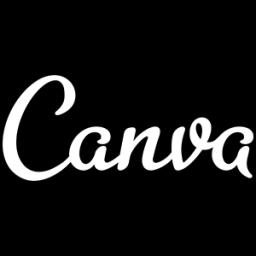
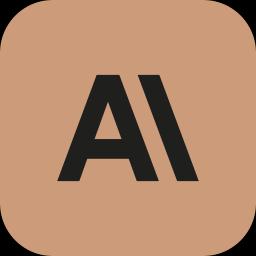
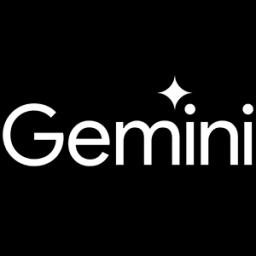
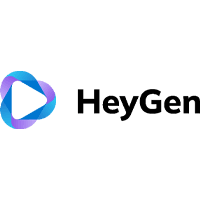
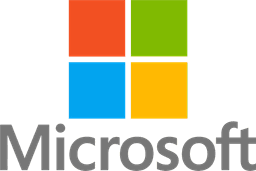
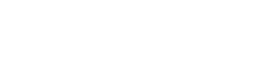
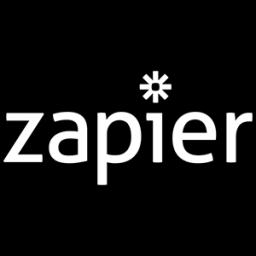
The optimization of GPU code within DeepSeek's systems further contributes to their cost-efficiency. By fine-tuning GPU operations, they have been able to maximize performance while minimizing costs. This optimization is a reflection of their comprehensive approach in harnessing available computational resources to their fullest potential, demonstrating that with the right innovations, significant financial savings are achievable in the high-stakes field of AI development [source](https://www.straitstimes.com/business/how-did-deepseek-build-its-ai-with-less-money).
Moreover, the financial implications of DeepSeek’s $6 million AI training are profound, especially when juxtaposed against the industry norm, such as Meta's $60 million budget for similar capabilities. The reduction of training costs without compromising the system’s capabilities has sparked discussions about the democratization of AI development. It signifies a shift towards more accessible and affordable AI solutions, potentially paving the way for more companies to enter the AI development space without the need for massive capital [source](https://www.straitstimes.com/business/how-did-deepseek-build-its-ai-with-less-money).
Understanding the Mixture of Experts Architecture
Mixture of Experts (MoE) architecture represents a significant evolution in the design of artificial intelligence systems, aiming to optimize efficiency and resource allocation in model training. At its core, MoE leverages a diverse ensemble of specialized neural networks, each dedicated to excelling in particular tasks. These specialized networks are orchestrated by a generalist supervision layer, which strategically assigns tasks to the most suitable expert networks. This configuration reduces computational overhead by minimizing inter-GPU communication, thus cutting down on costs and energy consumption, as demonstrated in DeepSeek's innovative approach to AI development. For instance, DeepSeek has successfully managed to train AI with expenditures amounting to just $6 million, a fraction of the expected costs seen in other major tech firms like Meta, which reportedly invests around $60 million for similar capabilities ().
The concept of using a Mixture of Experts architecture is not only a cost-saving innovation but also a technological advancement that addresses common limitations in traditional AI models. By utilizing a combination of specialized models, the system can handle various tasks with increased precision and reduced latency. Furthermore, DeepSeek's model employs 8-bit precision calculations during the initial processing stages, which substantially reduces resource demands. These calculations are stored with a higher 32-bit precision, ensuring that the integrity and quality of the outcomes are preserved, while also allowing efficient data handling and storage (). This approach showcases a balancing act between efficiency and performance, offering an optimized pathway for AI deployment across diverse applications.
The Mixture of Experts architecture also opens new avenues for scalability and customization in AI systems. By compartmentalizing tasks across specialized models, it offers flexibility in adjusting and upgrading individual components without disrupting the overall system. This adaptability is particularly crucial for companies looking to expand their AI capabilities without incurring substantial additional costs. DeepSeek's utilization of MoE is backed by highly optimized GPU code, which complements these efficiency gains, demonstrating an interplay between advanced coding practices and architectural ingenuity (). The strategic integration of these elements positions the MoE architecture as a forward-thinking solution that could reshape current paradigms in AI technology.
The Role and Impact of 8-bit Precision Calculations
In recent years, the field of artificial intelligence has seen significant advancements, particularly in the efficiency and cost-effectiveness of model training processes. A key development in this area is the use of 8-bit precision calculations, which DeepSeek has expertly implemented to optimize their AI systems. Unlike traditional 32-bit computations, 8-bit precision allows models to perform calculations utilizing less computational power without compromising the quality of results. In DeepSeek's approach, calculations are executed using 8-bit precision, but the final results are stored in 32-bit precision. This method balances efficiency with accuracy, ensuring that while computational resources are conserved, the precision of the final output remains unaffected. This innovative technique is one of several that have enabled DeepSeek to achieve a remarkable reduction in training costs, positioning them as a leader in cost-effective AI development.
Learn to use AI like a Pro
Get the latest AI workflows to boost your productivity and business performance, delivered weekly by expert consultants. Enjoy step-by-step guides, weekly Q&A sessions, and full access to our AI workflow archive.
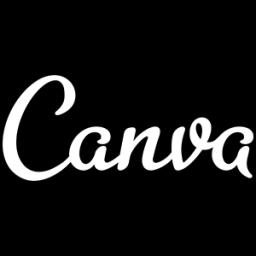
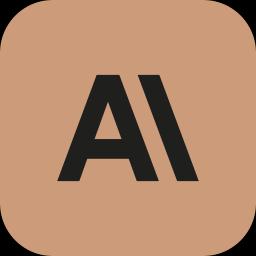
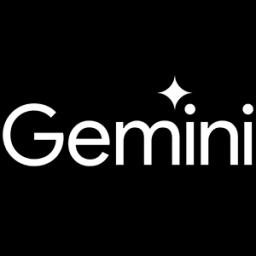
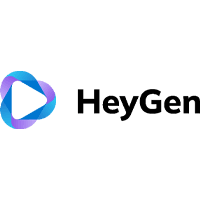
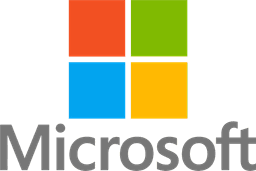
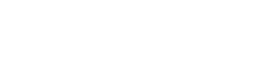
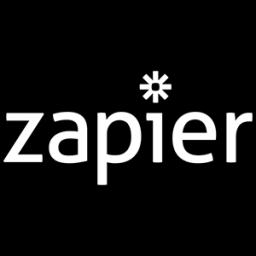
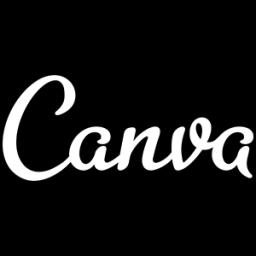
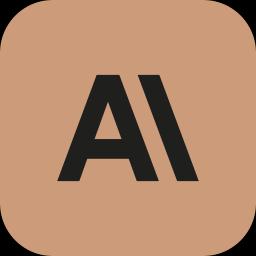
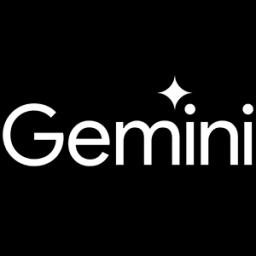
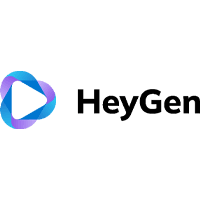
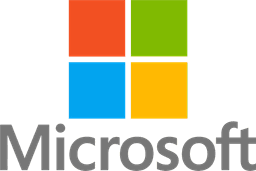
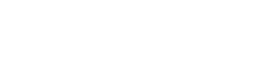
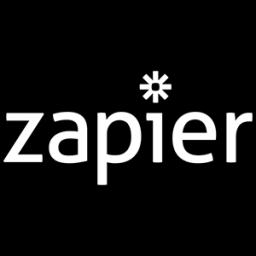
The impact of 8-bit precision calculations extends beyond just cost savings. It represents a transformative change in how AI models can be trained. The use of 8-bit precision calculations, as demonstrated by DeepSeek, not only reduces hardware costs but also decreases energy consumption during the training phase. As AI models grow increasingly complex and large-scale, the need for such efficient computational techniques becomes more critical. DeepSeek's system, which cost approximately $6 million to train, showcases the potential for significant savings in energy and resource usage while maintaining high performance standards [DeepSeek's approach](https://www.straitstimes.com/business/how-did-deepseek-build-its-ai-with-less-money).
Furthermore, the adoption of 8-bit precision methods could catalyze broader industry changes. Other companies may begin to follow suit, adopting this approach to reduce their own development costs and energy use. Given the competitive nature of the AI industry and the substantial financial and environmental savings offered by 8-bit precision calculations, it's likely that this technique will become more prevalent. DeepSeek’s success signals a potential paradigm shift, where efficiency-driven innovations become a primary focus for AI development [Insights on industry impacts](https://www.straitstimes.com/business/how-did-deepseek-build-its-ai-with-less-money).
Comparative Analysis with Other AI Developers
While many companies have capitalized on the potential of AI, DeepSeek has remarkably distinguished itself by achieving unprecedented cost-efficiency in AI training through innovation. The heart of their strategy lies in the Mixture of Experts (MoE) architecture, where specific neural networks are designated for specialized tasks, coordinated by a central generalist system. This approach substantially reduces the costly overhead associated with inter-GPU communication, enabling them to train their system for just $6 million, a stark contrast to Meta's expenditure of $60 million for similar capabilities .
DeepSeek's implementation of 8-bit precision calculations further exemplifies their pioneering efficiency. Despite the reduced bit usage during computation, the final results maintain 32-bit precision, ensuring the integrity of high-quality outcomes . Additionally, their meticulously optimized GPU code cuts down processing times and energy consumption, setting a new standard in AI development. These innovations not only represent significant cost savings but also pose a challenge to industry leaders like Nvidia, whose stock fell amid concerns over DeepSeek's disruptive methods .
The rise of efficient AI models, as seen with Mistral AI's and IBM's advancements, reflects a burgeoning movement toward cost-effectiveness in AI development. Mistral, utilizing similar MoE structures to DeepSeek, managed to drop development costs by two-thirds, while IBM's integration of 8-bit calculations facilitated a groundbreaking 70% reduction in training expenses . These companies illustrate how DeepSeek's methodologies are reshaping competitive dynamics across the AI landscape, urging traditional players to reassess their strategies.
Public and Expert Reactions
Public reaction to DeepSeek's recent AI training achievement has been largely positive, with many seeing it as a potential game-changer in the field of artificial intelligence. Enthusiasts are particularly excited about the democratization of AI development, as DeepSeek's cost-efficient methods could enable smaller companies and individual developers to compete in a space traditionally dominated by tech giants. This shift could foster innovation and accelerate advancements across various industries, bringing a new wave of technological solutions to complex problems. However, skepticism remains among industry watchers, who question the exclusion of substantial R&D costs from the reported $6 million final training cost. They argue that without these figures, it's challenging to fully assess the economic impact and viability of DeepSeek's approach [source].
Learn to use AI like a Pro
Get the latest AI workflows to boost your productivity and business performance, delivered weekly by expert consultants. Enjoy step-by-step guides, weekly Q&A sessions, and full access to our AI workflow archive.
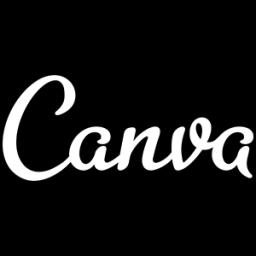
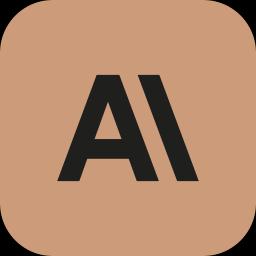
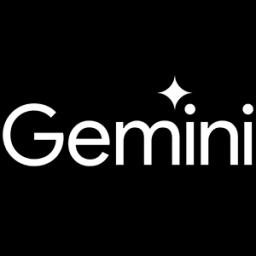
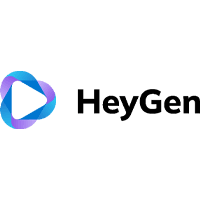
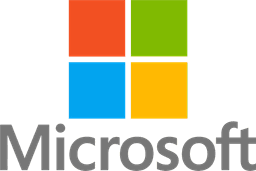
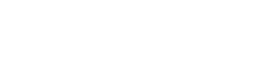
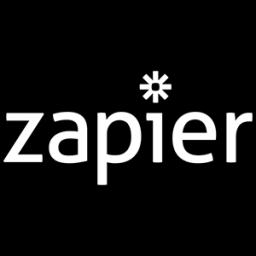
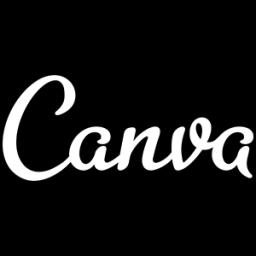
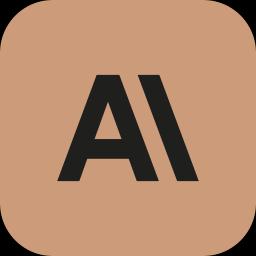
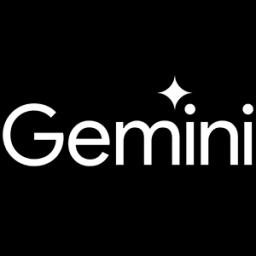
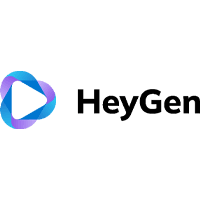
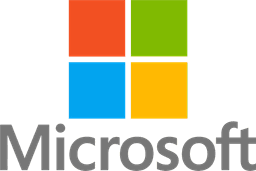
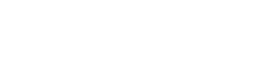
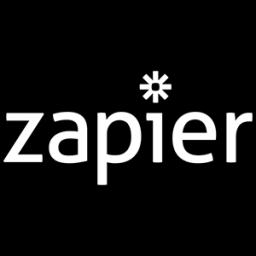
Expert opinions highlight the innovative nature of DeepSeek's AI training methodology, particularly their use of Mixture of Experts (MoE) architecture and advanced optimization techniques. Analysts suggest that these methods, while cost-effective, could introduce performance trade-offs that need to be clearly understood and validated. The IDC has noted DeepSeek's strategic application of 8-bit precision calculations stored in 32-bit format as a cornerstone of their efficiency drive. There is a generalized sense of optimism in the expert community about the potential for such techniques to reduce overall AI development costs, but detailed evaluations and time will be key to confirming these expectations [source].
The public's response on various discussion forums reflects a blend of intrigue and caution. While some participants are eager to explore how these cost-saving techniques could be adopted elsewhere, there's an underlying curiosity about why more companies haven't implemented similar methods. Forum members speculate whether the hesitation is due to high upfront costs, perceived risks, or the potential need for complex overhauls of existing systems. There is strong interest in the community to see if DeepSeek's model will inspire broader changes within the industry, or if it will remain a unique case study in cost-efficient AI development [source].
Future Implications and Potential Impact
The revelations from DeepSeek have sparked a wave of anticipation about the potential future implications on the landscape of artificial intelligence (AI). The fundamental reshaping of AI development through cost-efficient models may democratize access to AI technologies, enabling smaller companies to compete in a field previously dominated by tech giants. By lowering the barriers to entry, DeepSeek's innovative approaches could initiate a new era of technological entrepreneurship and accelerate innovation cycles across diverse sectors. Such democratization is expected to lead to significant market disruption, as smaller startups challenge established players through agility and cost competitiveness. Analysts believe that potential price wars and shifts in valuation metrics in the tech industry could arise from this paradigm shift [source](https://foreignpolicy.com/2025/02/05/deep-seek-china-us-artificial-intelligence-ai-arms-race).
Socially, these advancements in AI could enhance global access to sophisticated AI tools for researchers and developers, who can apply them across various disciplines, fostering innovation in areas previously limited by high costs. However, the rise of accessible AI also raises concerns about potential misuse and necessitates the implementation of new regulations to safeguard against ethical and security risks [source](https://www.sciencedirect.com/science/article/pii/S2949697724000055). While improved access to AI can lead to increased productivity and efficiency, it may simultaneously disrupt existing job markets, prompting the need for proactive retraining programs to prepare the workforce for the new AI-driven economy [source](https://www.sciencedirect.com/science/article/pii/S2949697724000055).
On the geopolitical stage, DeepSeek's breakthroughs might alter global power structures in AI, challenging the tech hegemony traditionally held by the United States. This shift could catalyze a reevaluation of American export controls on critical AI technologies, as nations worldwide strive to bolster their own AI capacities in response to China's burgeoning advancements [source](https://foreignpolicy.com/2025/02/05/deep-seek-china-us-artificial-intelligence-ai-arms-race). Furthermore, these developments are likely to accelerate national AI development programs as countries recognize the strategic importance of advancing AI technologies in maintaining global competitive advantages [source](https://www.bruegel.org/first-glance/geopolitics-artificial-intelligence-after-deepseek). Despite these promising potential impacts, there remains notable uncertainty surrounding DeepSeek's claims, necessitating ongoing scrutiny and validation of their methods and results [source](https://cyber.fsi.stanford.edu/publication/taking-stock-deepseek-shock).