Safety Concerns in AI
Does RAG Make LLMs Less Safe? Bloomberg's Alarming Findings
Last updated:

Edited By
Mackenzie Ferguson
AI Tools Researcher & Implementation Consultant
Discover how Retrieval Augmented Generation (RAG) might be compromising the safety of Large Language Models (LLMs), according to Bloomberg's latest research. Learn about the hidden dangers, the significant rise in unsafe responses, and the call for domain-specific safety measures.
Introduction to Retrieval Augmented Generation (RAG)
Retrieval Augmented Generation (RAG) represents a novel approach in the realm of artificial intelligence that seeks to enhance the capabilities of Large Language Models (LLMs) by integrating external knowledge into their functioning. This method involves augmenting the model's existing datasets with additional data retrieved from various sources, thereby providing more contextually rich responses. The process essentially grounds the model’s outputs in factual information, significantly improving response accuracy and minimizing hallucinatory outputs. By providing models access to a vast pool of external knowledge, RAG effectively increases the reliability of AI outputs, which is particularly beneficial in complex decision-making scenarios [0](https://venturebeat.com/ai/does-rag-make-llms-less-safe-bloomberg-research-reveals-hidden-dangers/).
However, despite its promising approach to improving LLM accuracy, recent studies, such as the one conducted by Bloomberg, have raised concerns regarding the safety implications of RAG implementations. The research indicates that while RAG enhances factual grounding, it can inadvertently weaken the safety frameworks of LLMs. Specifically, the augmented data inputs may bypass existing safety protocols, leading to unintended and potentially harmful responses. This finding highlights a critical challenge in AI development where synchronization between advanced data augmentation techniques and robust safety mechanisms is essential to prevent such vulnerabilities [0](https://venturebeat.com/ai/does-rag-make-llms-less-safe-bloomberg-research-reveals-hidden-dangers/).
Learn to use AI like a Pro
Get the latest AI workflows to boost your productivity and business performance, delivered weekly by expert consultants. Enjoy step-by-step guides, weekly Q&A sessions, and full access to our AI workflow archive.
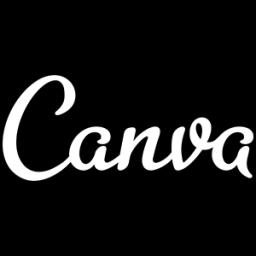
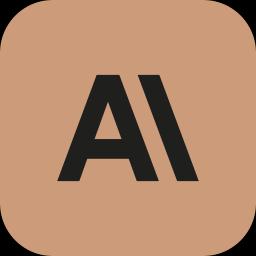
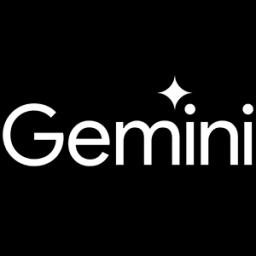
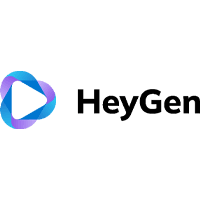
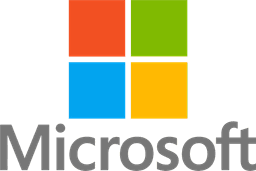
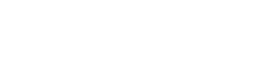
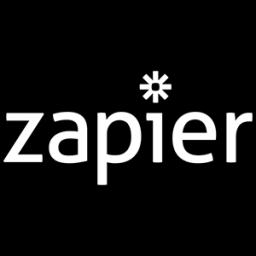
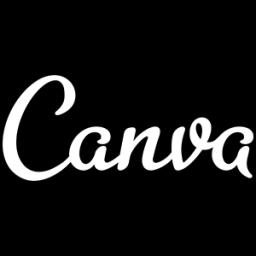
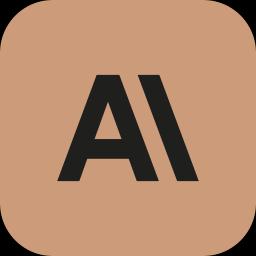
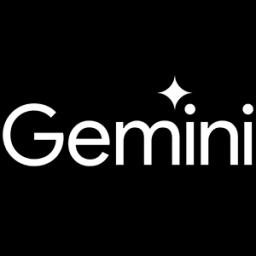
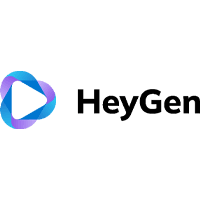
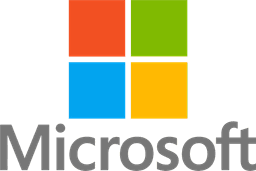
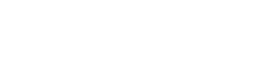
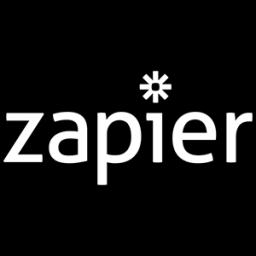
Furthermore, the reported increase in unsafe responses has underscored the necessity for domain-specific safety measures in RAG implementations. Safety considerations must be tailored to align with the unique requirements and risk profiles of different industries. In the financial sector, for instance, integrating bespoke safety systems into the RAG framework could mitigate the risks highlighted by Bloomberg’s research, thereby securing a safer passage for AI-driven decision-making in high-stakes environments. Lessons from these findings emphasize the importance of continuous innovation in AI safety protocols to address emerging challenges as AI technologies evolve [0](https://venturebeat.com/ai/does-rag-make-llms-less-safe-bloomberg-research-reveals-hidden-dangers/).
Bloomberg's Research Findings on RAG and LLM Safety
Bloomberg's recent research has sparked considerable discussion regarding the safety of Large Language Models (LLMs) when augmented by Retrieval Augmented Generation (RAG). The findings presented by Bloomberg indicate a potential compromise in safety, which is particularly concerning given the increasing reliance on AI across various sectors. The research reveals that by integrating external context, RAG inadvertently bypasses pre-existing safety mechanisms, thus triggering unsafe responses even to harmful queries. This reflects a larger problem since LLMs are not inherently designed to manage long, complex inputs safely, especially in the absence of strong internal safeguards.
The study's implications are particularly significant in domains like finance, where even minor inaccuracies can lead to substantial consequences. Bloomberg underscores the need for domain-specific safety measures, advocating for specialized AI content risk taxonomies tailored to unique industry needs. This is crucial to address sector-specific risks that generic safety protocols might overlook. By integrating these custom safety systems directly into RAG implementations, organizations can mitigate potential dangers while maintaining the benefits of enhanced LLM accuracy and contextual relevance.
Bloomberg’s nuanced approach to AI safety aligns with recent developments in global AI regulation. The shift towards domain-specific safety frameworks is not only a reflection of regulatory trends but also an acknowledgment of the complex, multifaceted nature of AI deployment across industries. As governments like the EU move forward with initiatives such as the AI Act, the importance of creating AI systems that are not only effective but also secure is becoming increasingly evident. Key measures include red teaming for identifying vulnerabilities and ensuring that AI operates transparently and accountably.
Learn to use AI like a Pro
Get the latest AI workflows to boost your productivity and business performance, delivered weekly by expert consultants. Enjoy step-by-step guides, weekly Q&A sessions, and full access to our AI workflow archive.
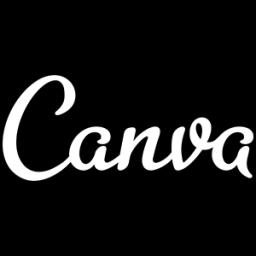
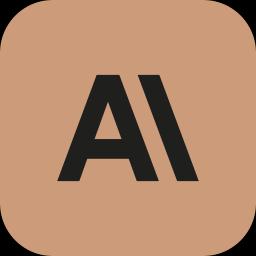
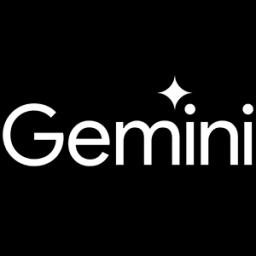
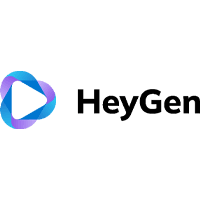
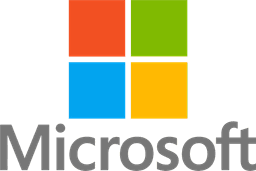
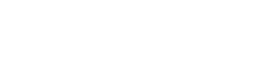
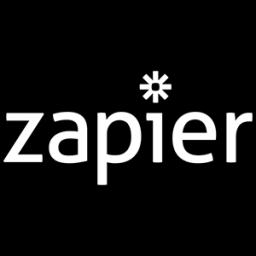
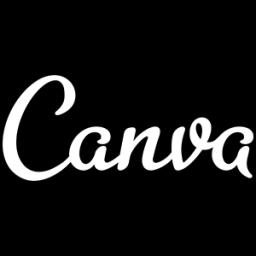
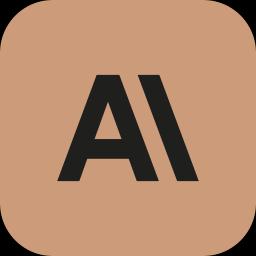
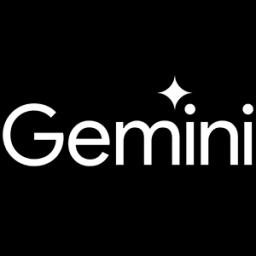
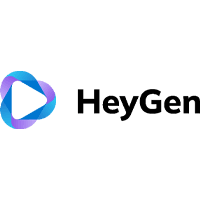
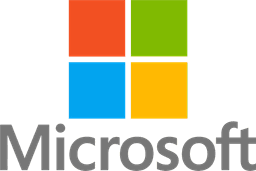
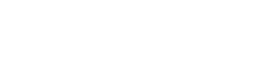
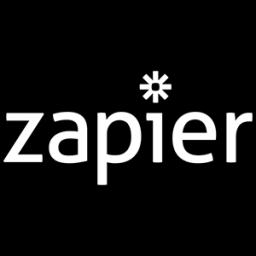
Despite these efforts, public reactions have been mixed, reflecting a spectrum of emotions from surprise to skepticism. The inherent contradiction presented by Bloomberg's findings — that augmenting safety-focused AI systems with additional context could paradoxically reduce their safety — has sparked debate about the future trajectory of RAG in AI development. For businesses, this signifies the importance of adopting a holistic approach to RAG implementation, carefully balancing the benefits of enhanced capabilities and the risks associated with potential safety breaches. In doing so, they must ensure compliance with evolving safety regulations while maintaining competitive advantages.
Given Bloomberg’s own stake in the financial data market, there are questions about potential conflicts of interest in their research. While some may argue that their focus on AI transparency and reliability serves to safeguard their existing market position, others view it as a legitimate effort to advance industry standards for responsible AI usage. Regardless of their motivations, Bloomberg's contributions underscore the critical need for ongoing dialogue and development in the AI domain to address complex safety challenges. Their research stands as a call to action for industries to innovate responsibly, ensuring that AI enhances rather than undermines societal and economic well-being.
Understanding RAG's Mechanisms and Potential Risks
Retrieval Augmented Generation (RAG) represents a significant advancement in the realm of artificial intelligence, specifically in the way it enhances the functionality of Large Language Models (LLMs). By integrating external, relevant data sources into the AI's processing pretext, RAG enables more accurate and contextually grounded responses. This integration helps reduce the incidences of AI "hallucinations"—the generation of incorrect or nonsensical information by a model. The objective is to create responses that are not only factually accurate but also reliable, aligning closely with the information provided by credible external sources. While these enhancements call for applauding RAG's potential, it is essential to also consider the accompanying risks emerging from its use, as highlighted in recent research studies [VentureBeat].
Bloomberg's research underscores a paradox where the same mechanisms that enhance LLMs through RAG can also erode their safety. This occurs when safety protocols built into LLMs are insufficient or bypassed during the retrieval and augmentation process. The study reveals that RAG might inadvertently present the potential for unsafe responses even when handling queries assumed to be benign [VentureBeat]. This unexpected vulnerability arises because traditional LLM safety mechanisms are not always equipped to handle the scope of content introduced through RAG. As a result, the need for integrating domain-specific safety measures into RAG frameworks becomes crucial, ensuring that inputs, no matter how extended or varied, do not compromise the overall safety of the system.
From a regulatory perspective, the implications of RAG's vulnerabilities cannot be overstated. As governments and regulatory bodies focus on AI safety and regulation, tools like RAG, which can affect crucial areas of governance like data privacy, misinformation, and security, must be closely examined. Laws similar to the EU's AI Act, which seeks to regulate AI through a thorough assessment of associated risk levels, need to adapt and consider the unique challenges posed by RAG-enhanced models [VentureBeat]. Additionally, AI practitioners are urged to remain vigilant, implementing business logic checks and developing safety taxonomies tailored to specific domains of application, to avoid the unintended spread of unsafe outputs.
In light of these discoveries, the future trajectory of RAG's integration into mainstream applications is uncertain. The findings not only stir a debate on the practical utility of RAG but also raise considerable concern about its role in sectors where high-stakes decisions are made, such as finance and healthcare. The realization that widely trusted LLMs might operate less safely due to RAG demands immediate action and redress through comprehensive research and strategic planning [VentureBeat]. AI developers and stakeholders need to collaborate to ensure these systems are responsibly harnessed to augment human decision-making processes without compromising their inherent safety.
Learn to use AI like a Pro
Get the latest AI workflows to boost your productivity and business performance, delivered weekly by expert consultants. Enjoy step-by-step guides, weekly Q&A sessions, and full access to our AI workflow archive.
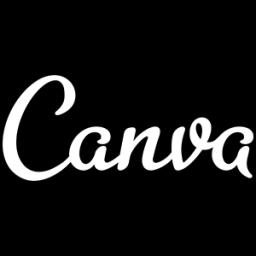
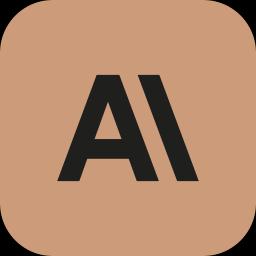
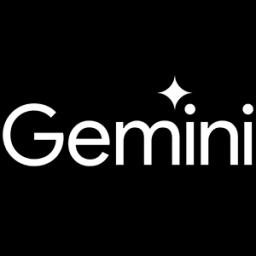
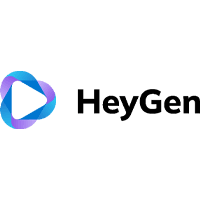
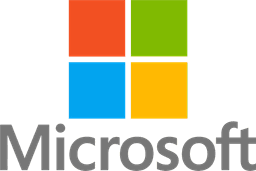
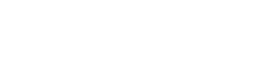
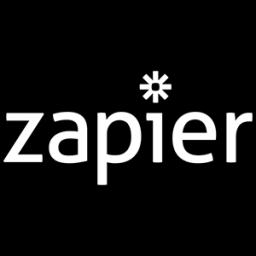
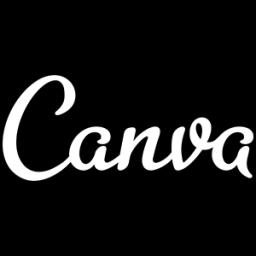
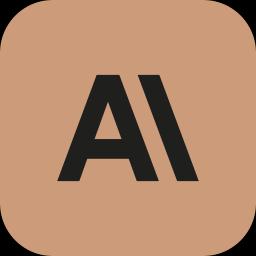
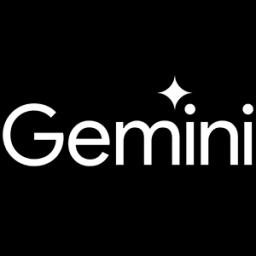
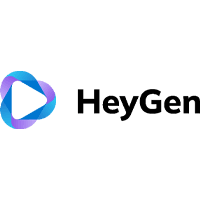
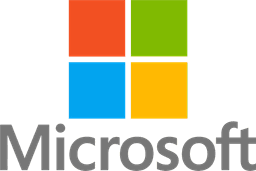
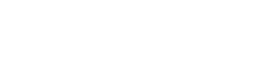
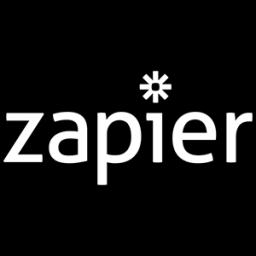
The Role of Domain-Specific Safety Measures
The ever-evolving landscape of artificial intelligence (AI) continues to present both opportunities and challenges, particularly when it comes to ensuring the safety of its applications. Domain-specific safety measures have emerged as a pivotal approach to mitigating risks associated with AI technologies like Retrieval Augmented Generation (RAG). According to Bloomberg's research, integrating safety systems directly into RAG implementations is crucial as it has been noted that RAG can inadvertently bypass established safety guardrails when applied to Large Language Models (LLMs), introducing unexpected vulnerabilities in various domains. Developing these tailored safety measures ensures that each industry can address its unique vulnerabilities, safeguarding against potential harmful outputs in sensitive areas like finance and healthcare .
One of the primary insights from the recent findings is the pressing need for AI systems to be evaluated within the contexts they are deployed, transcending general safety claims. As emphasized by experts such as Sebastian Gehrmann from Bloomberg, domain-specific AI safety taxonomies align safety protocols with the intricate needs of different sectors. For example, in the financial industry, such measures can address specific risks like fraud and data misconduct that generic safety systems might overlook. By moving beyond blanket safety frameworks and creating specialized taxonomies, businesses can better anticipate how retrieved content interacts with a model's safety mechanisms, ensuring more reliable and responsible AI applications .
The significance of domain-specific safety approaches is underscored by the various challenges that arise from LLMs in sectors reliant on precise and secure information exchange. The research led by Bloomberg shows that without these tailored safety measures, sectors like finance—which heavily depend on accurate data interpretation—face increased risks from inaccurate or unsafe outputs generated by AI systems. By implementing robust safeguards specific to each domain, companies can mitigate these risks, enhancing trust and stability within industries notorious for high stakes in data integrity and confidentiality .
The broader implications of this research indicate a move towards collaborative efforts between AI developers, industry specialists, and policymakers to create AI systems that do not just comply with generic safety standards but are specifically refined for the unique challenges and demands of each field. This underscores the necessity for ongoing dialogue and partnership in the AI community to develop and regularly update these safety measures. The involvement of committed stakeholders across different sectors in this collaborative endeavor is vital to navigate the complexities of AI application and to ensure its safe and ethical deployment across various domains .
Integrating Safety Systems in RAG Implementations
As the implementation of Retrieval Augmented Generation (RAG) becomes more prevalent, integrating safety systems into its framework is paramount. RAG is designed to enhance the performance of Large Language Models (LLMs) by utilizing external data to provide more contextually accurate and reliable responses. However, according to Bloomberg's recent research, this approach can inadvertently undermine safety protocols, leading to potentially harmful outputs. Implementing robust safety systems within RAG can mitigate these risks by ensuring that both the retrieval process and the integration of external data do not bypass existing safeguards, thereby maintaining the integrity and safety of LLMs .
The study by Bloomberg underscores the necessity for domain-specific safety measures when integrating RAG into LLM platforms. By tailoring safety protocols to the unique requirements of different fields—such as finance, where the implications of unsafe outputs can be particularly severe—businesses can better guard against the potential pitfalls of RAG augmentation. This involves developing comprehensive taxonomies that address sector-specific risks, such as data confidentiality and financial misconduct, which generic safety measures might overlook. With the integration of specialized safety systems, companies can harness the benefits of RAG while minimizing its risks .
Learn to use AI like a Pro
Get the latest AI workflows to boost your productivity and business performance, delivered weekly by expert consultants. Enjoy step-by-step guides, weekly Q&A sessions, and full access to our AI workflow archive.
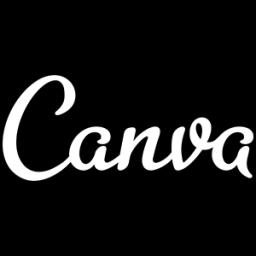
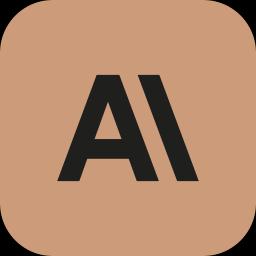
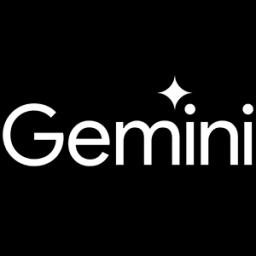
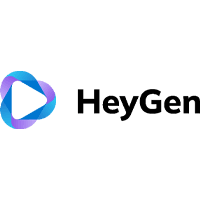
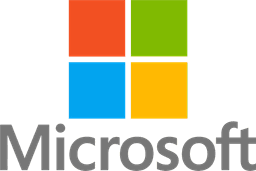
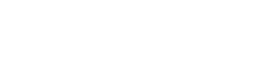
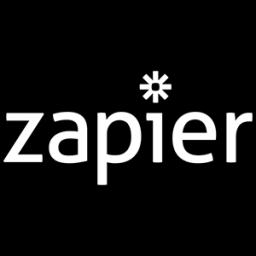
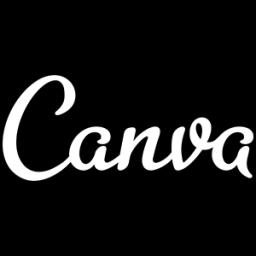
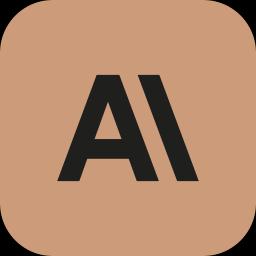
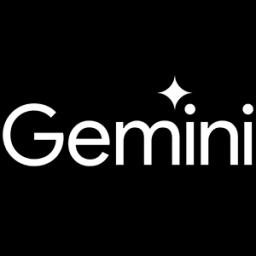
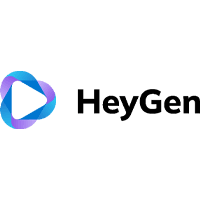
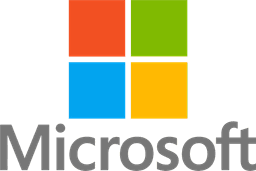
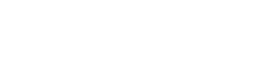
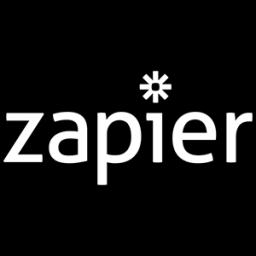
Integrating safety within RAG implementations not only involves refining technical protocols but also requires a coordinated effort across technological, ethical, and regulatory spheres. Experts, such as Sebastian Gehrmann from Bloomberg, emphasize evaluating AI systems within their specific deployment contexts to move beyond blanket safety claims. A framework that involves regular assessments of how retrieved content interacts with model safeguards is critical. Industries must create collaborative environments where technological innovation is matched with ethical guidelines and regulatory standards to ensure safe AI deployment .
Furthermore, the integration of safety systems into RAG models also highlights the importance of explainability and transparency within AI processes. As Amanda Stent from Bloomberg points out, ensuring that AI practitioners are mindful of the implications of RAG can lead to safer and more reliable outcomes in applications like customer support and automated reporting. Increasing transparency in these systems will not only aid in understanding and predicting model behavior but also build public trust. It’s essential that businesses leverage explainable AI tools as part of their RAG implementation to demystify AI decision-making processes and enhance accountability .
Bloomberg's AI Content Risk Taxonomy for Financial Services
Bloomberg's AI content risk taxonomy for the financial services sector has gained significant attention in recent times due to its strategic focus on addressing unique risks associated with financial data. As highlighted in VentureBeat's article, this taxonomy is pivotal in managing sector-specific challenges, such as financial misconduct and the inadvertent disclosure of sensitive information, which are frequently overlooked by more generic AI safety frameworks. The significance of adapting AI safety measures to the nuances of specific industries cannot be overstated, and Bloomberg is at the forefront of this initiative, ensuring that AI implementations within the finance sector are not only efficient but also secure against potential risks.
The development of Bloomberg's AI content risk taxonomy comes in the wake of research revealing the potential risks posed by Retrieval Augmented Generation (RAG) when integrated with Large Language Models (LLMs). Such risks include the inadvertent circumvention of existing safety measures governing LLMs, thereby potentially leading to harmful or unsafe responses. In finance, where data precision and confidentiality are paramount, the adoption of a specialized taxonomy helps in delineating the boundaries within which RAG technologies, and indeed all AI implementations, must operate. This focused approach is further essential because it recognizes the intricacies specific to financial services where regulatory demands and the nature of data differ widely from other sectors.
The potential dangers posed by RAG, such as those discovered in Bloomberg's study, underscore the need for industry-specific safety measures. Bloomberg’s AI content risk taxonomy is a direct response to these challenges, addressing vulnerabilities that might not be covered under broad, generic AI safeguards. As explained in the study, by enforcing these tailored measures, Bloomberg aims to mitigate the risk of AI models producing unsafe output, thereby enhancing the reliability and safety of AI technologies within firms that are highly dependent on them for critical decision-making processes.
In implementing this taxonomy, Bloomberg is forging a path for other sectors to follow, advocating for a deep understanding of domain-specific challenges when developing AI safety mechanisms. The focus on creating these detailed safety frameworks reflects a growing acknowledgment that one-size-fits-all approaches to AI safety are insufficient, particularly in domains as sensitive and high-stakes as financial services. By leveraging their extensive experience within the industry, Bloomberg can offer a well-rounded and practically applicable risk taxonomy that not only aligns with but also anticipates future regulatory and operational needs within the sector. This positions the company as a leader in the evolving landscape of AI safety in finance.
Learn to use AI like a Pro
Get the latest AI workflows to boost your productivity and business performance, delivered weekly by expert consultants. Enjoy step-by-step guides, weekly Q&A sessions, and full access to our AI workflow archive.
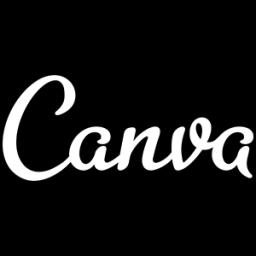
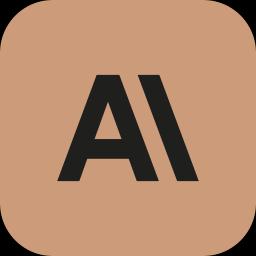
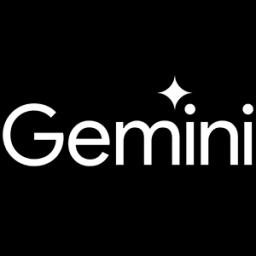
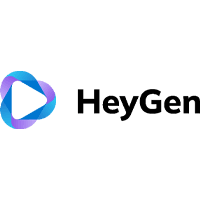
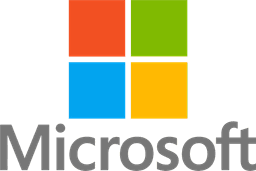
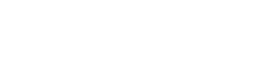
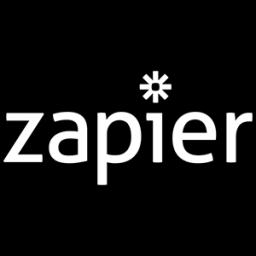
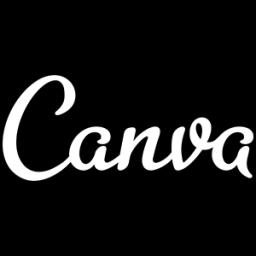
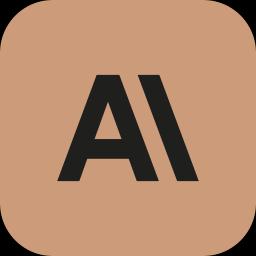
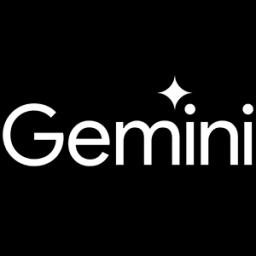
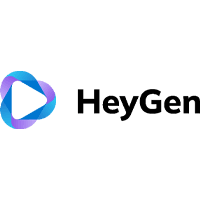
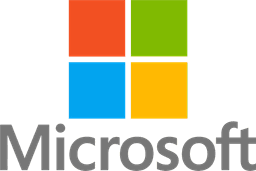
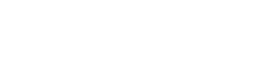
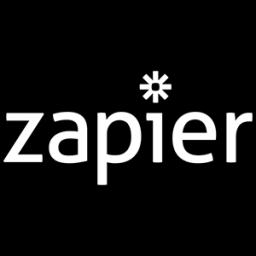
Practical Implications for Businesses Using RAG
The research on Retrieval Augmented Generation (RAG) presents several crucial practical implications for businesses that rely on large language models (LLMs). As highlighted by Bloomberg's findings, one of the primary concerns for companies is integrating robust safety systems within RAG frameworks to prevent these technologies from bypassing traditional safety guardrails. This is particularly significant in sectors like finance, where misinformation or unsafe outputs can have devastating effects. Businesses must therefore consider investing in developing domain-specific safety measures that tie back to their operational contexts, ensuring AI deployments are not only effective but responsibly managed. Amanda Stent from Bloomberg underscores the necessity for AI practitioners to be vigilant and fortify their implementations with appropriate safeguards to mitigate potential risks .
Moreover, the economic implications cannot be overlooked. Companies operating in high-stakes environments like finance need to navigate the balance between leveraging sophisticated AI capabilities and managing the associated risks. Implementing domain-specific risk taxonomies, as advocated by Bloomberg researchers, can help these businesses ensure that their AI systems are tailored to meet their unique risk profiles. Achieving this balance could lead to substantial competitive advantages, allowing businesses not only to safeguard their reputation but also to gain market confidence by demonstrating a commitment to responsible AI use . This means an increased need for companies to allocate resources effectively towards crafting risk management frameworks that are not only predictive but also adaptive to the fast-changing landscape of AI technologies.
For businesses utilizing RAG in customer-facing applications, the implications are equally profound. The potential for generating unsafe content makes it imperative for companies to implement red teaming practices and adversarial testing to identify and mitigate vulnerabilities within their AI systems. As noted by David Rabinowitz at Bloomberg, while there is an intensive focus on AI safety within broader consumer applications, industry-specific concerns necessitate tailored tools and resources to address these challenges . This proactive approach can help maintain customer trust and uphold brand integrity in an increasingly AI-driven market.
Finally, fostering transparency and accountability through explainable AI frameworks is essential. The public's trust in AI systems heavily relies on their perceived transparency and fairness, which makes it necessary for businesses to provide clear justifications for AI-driven decisions. Companies need to focus on embedding explainability as a core component of their AI strategies, ensuring that not only are they compliant with current regulations, but that they are also positioned to adapt to future legislative changes. This can be seen in initiatives like the EU's AI Act, which emphasizes risk-based regulation of AI systems .
Addressing Conflict of Interest Concerns
Addressing conflict of interest concerns in the context of Bloomberg's research on Retrieval Augmented Generation (RAG) and large language models (LLMs) is crucial for maintaining trust and transparency in AI technologies. Bloomberg, a leading figure in the financial data market, is also pioneering research in AI, particularly focusing on the safety implications of RAG implementations. This dual role could potentially lead to skepticism about the objectivity and motivation behind their findings. Some industry experts might question whether the research, highlighting the hazards of RAG, is truly an unbiased effort to enhance AI safety, or whether it serves to fortify Bloomberg's market advantage by discouraging competitors from utilizing potentially unsafe AI methodologies. For more insights into this dynamic, a detailed examination of Bloomberg's strategies is necessary, as seen in their joint efforts to build domain-specific AI safety measures alongside their financial services. Read more here.
An inherent conflict of interest arises when a company like Bloomberg, deeply embedded in the financial sector, ventures into AI safety research that directly impacts its core business operations. The findings that RAG can compromise LLM safety might be perceived as serving dual purposes: advancing universal AI safety standards and protecting Bloomberg's entrenched interests in data integrity and reliability. This dual motive scenario necessitates a transparent revelation of intentions and methodologies used in their research. As stakeholders evaluate these findings, assurances of independent verification and peer reviews become invaluable to substantiate claims made in highly specialized fields. This approach not only disperses doubts about conflicts of interest but also reinforces the credibility and integrity of Bloomberg's AI initiatives. Industry observers can further explore the implications of these findings by accessing comprehensive detailed discussions, further scrutinizing the motivations behind Bloomberg's research. Explore the full article.
Learn to use AI like a Pro
Get the latest AI workflows to boost your productivity and business performance, delivered weekly by expert consultants. Enjoy step-by-step guides, weekly Q&A sessions, and full access to our AI workflow archive.
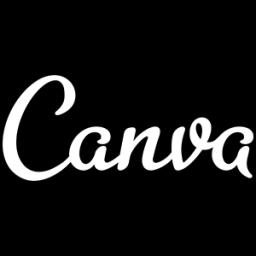
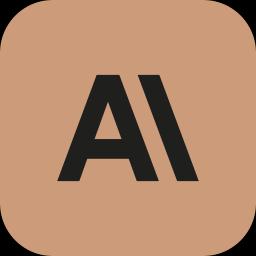
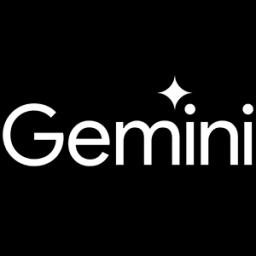
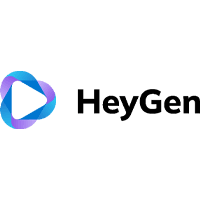
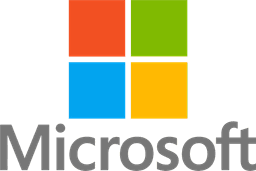
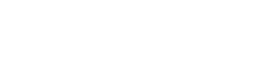
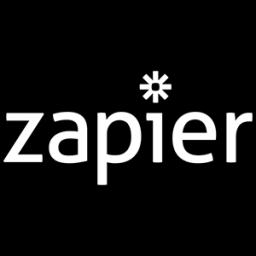
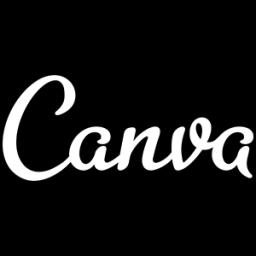
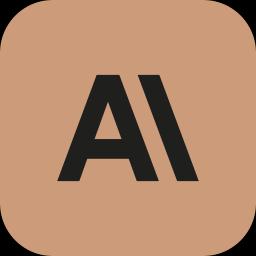
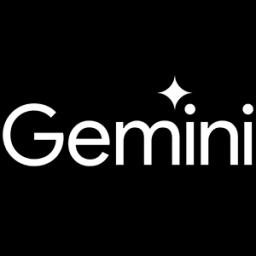
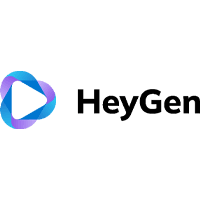
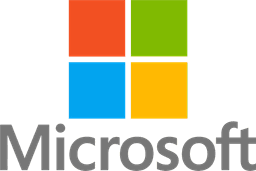
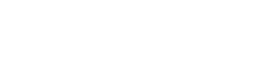
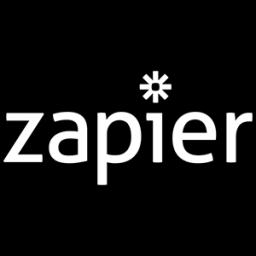
The debate over potential conflicts of interest in Bloomberg's RAG research underscores broader concerns about AI ethics and corporate influence in shaping technology narratives. Given the rapidly evolving role of AI in finance, questions about transparency arise, questioning whether proprietary interests could skew the research agenda toward self-serving outcomes. Bloomberg's emphasis on financial sector-specific taxonomy of risks does suggest a commitment to addressing unique contextual challenges faced by their clientele, yet some critics argue that it may subtly prioritize their own business frameworks over broader industry needs. The commitment to mitigate GenAI risks by tailoring these safety measures uniquely applicable to financial services is indeed crucial; however, it simultaneously poses questions about the adaptability of these solutions to other domains. Such discussions are vital in maintaining an accountable narrative in AI research and development, with findings that could potentially influence broader regulatory perspectives. Further elaboration on these topics can be gleaned from the original research reports by Bloomberg. Read detailed analyses.
Related Developments in AI Safety and Regulation
Recent developments in artificial intelligence (AI) safety and regulation highlight a growing global emphasis on creating responsible and secure AI systems. A significant event in this domain is the European Union's introduction of the AI Act, which categorizes AI systems based on their risk levels. By doing so, the EU aims to implement a comprehensive framework ensuring that as AI technologies advance, they adhere to rigorous safety and ethical standards, minimizing potential misuse or harm. These efforts reflect a broader global movement towards not only maximizing the beneficial potential of AI but also curbing its risks through thoughtful and adaptive legislation.
Moreover, the debate over AI bias and discrimination continues to gain momentum. Researchers are increasingly focused on understanding and mitigating the biases inherent in AI data and algorithms. This is crucial for ensuring fairness and equity as AI technologies become more integrated into decision-making processes across various sectors. The push towards creating unbiased AI systems is not only a technical challenge but also a social imperative, requiring multidisciplinary collaboration among technologists, ethicists, and policymakers.
Transparency and explainability are also at the forefront of AI safety discussions. The concept of explainable AI (XAI) is gaining traction, driven by the need for systems to offer comprehensible insights into their decision-making processes. This transparency is vital for users' trust and confidence in AI technologies, particularly in sensitive or high-stakes environments where AI-driven conclusions must be readily understood and justified.
Red teaming and adversarial attacks are becoming key components in assessing AI safety. These methods allow developers to identify vulnerabilities within AI systems by simulating potential attack scenarios. As AI systems become more sophisticated, so do the strategies for testing them, ensuring they are resilient under threats. This defensive approach is pivotal for cultivating robust AI systems capable of withstanding real-world adversities.
There's a noticeable shift towards crafting domain-specific AI safety taxonomies, an initiative prominently led by organizations like Bloomberg. By tailoring safety measures to particular industry contexts, such as financial services, these taxonomies aim to address unique risks that generic safety protocols might overlook. This trend underscores the importance of not only developing generalized AI safety standards but also recognizing the intricacies and specificities of different sectors in the AI landscape.
Learn to use AI like a Pro
Get the latest AI workflows to boost your productivity and business performance, delivered weekly by expert consultants. Enjoy step-by-step guides, weekly Q&A sessions, and full access to our AI workflow archive.
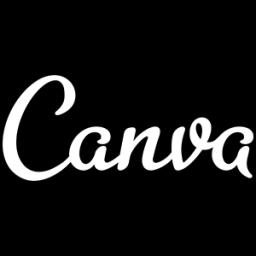
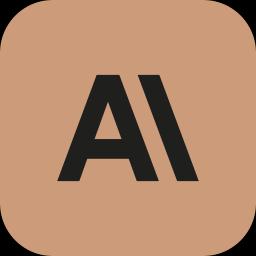
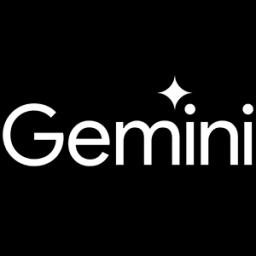
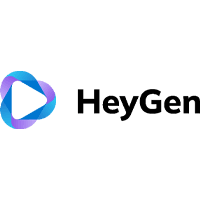
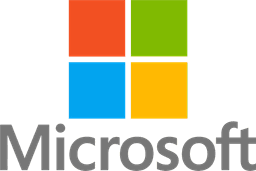
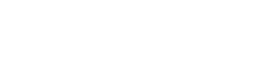
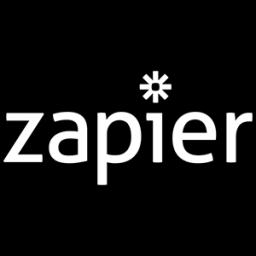
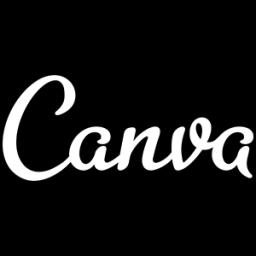
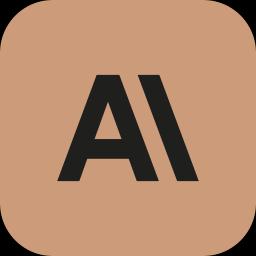
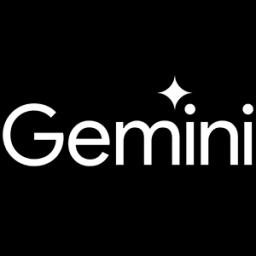
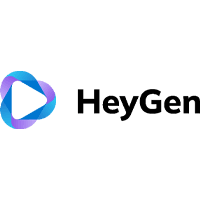
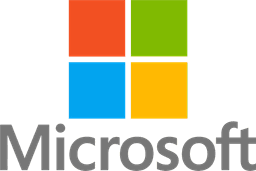
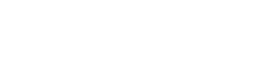
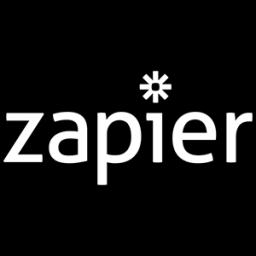
Expert Opinions on RAG and AI Safety
In the rapidly evolving field of artificial intelligence, understanding the safety implications of various technologies is crucial. Recently, Bloomberg's research has turned a spotlight on Retrieval Augmented Generation (RAG), raising concerns about its potential to undermine safety in Large Language Models (LLMs). This narrative has been front and center in discussions around AI safety, as experts like Sebastian Gehrmann, Head of Responsible AI at Bloomberg, emphasize the need for domain-specific safety taxonomies. Gehrmann argues that evaluating AI systems within their deployment contexts is indispensable for mitigating any hidden dangers. This approach is not just about identifying problems but ensuring that RAG implementations can predict and control how external content might interact with AI models, thereby reinforcing the guardrails rather than sidestepping them. For more details, including whether RAG truly makes LLMs less safe, see [Bloomberg's insights](https://venturebeat.com/ai/does-rag-make-llms-less-safe-bloomberg-research-reveals-hidden-dangers/).
Amanda Stent, Head of AI Strategy and Research at Bloomberg, has commented on the widespread applications of RAG in systems like customer support and QA environments. She points out that while RAG's potential for enhancing accuracy through added contextual information is promising, its implications for safety cannot be overlooked. Given the unexpected risks associated with RAG, Stent underscores the urgency for AI practitioners to embed comprehensive safeguards that prevent unsafe outputs. This is especially crucial as the utilization of RAG becomes more prevalent across industries. For more specific examples of how Bloomberg is addressing these issues, refer to [Bloomberg's press release](https://www.bloomberg.com/company/press/bloomberg-ai-researchers-mitigate-risks-of-unsafe-rag-llms-and-genai-in-finance/).
The debate on the safety of RAG in AI models extends beyond purely technical discussions to encompass ethical and industry-specific considerations. David Rabinowitz, Bloomberg's Technical Product Manager for AI Guardrails, highlights a gap in industry-specific safety tools, especially in the finance sector, where inaccurate information could lead to significant financial repercussions. He stresses that while general consumer applications have received considerable attention in terms of safety research, there's an urgent need to tailor these insights to high-stakes domains like finance, where the risk of unsafe AI decisions is highest. For an in-depth explanation of Bloomberg's approach to these challenges, [read more here](https://www.bloomberg.com/company/stories/bloomberg-responsible-ai-research-mitigating-risky-rags-genai-in-finance/).
Public reactions to Bloomberg's research reveal a spectrum of emotions—from surprise to skepticism. Many find it counterintuitive that a technology designed to increase accuracy could also increase risk. This paradox fuels public demand for more transparency and accountability in AI systems, an area where Bloomberg is keenly focused. They advocate for robust domain-specific measures that align with their business focus, like the content risk taxonomy specifically developed for financial services, to ensure AI applications within these areas are both innovative and safe. For a detailed dive into the public sentiment and potential conflicts of interest, see [Bloomberg's detailed report](https://globalfintechseries.com/finance/bloomberg-ai-researchers-mitigate-risks-of-unsafe-rag-llms-and-genai-in-finance/).
Public Reactions to Bloomberg's RAG Findings
The public's reaction to Bloomberg's findings on Retrieval Augmented Generation (RAG) has been both intense and varied. Many were caught off guard by the revelations, as RAG was previously hailed for enhancing the performance of Large Language Models (LLMs) by grounding responses in factual contexts. The notion that RAG could potentially diminish safety by sidestepping established guardrails has sparked both disbelief and concern among AI enthusiasts and tech critics alike. This unexpected contradiction has led to a broader discussion about the trusted roles of AI technologies in sensitive sectors. As reported in VentureBeat, Bloomberg's research accentuates the need for domain-specific safety measures, particularly shedding light on vulnerabilities that could impact sectors dependent on high-stakes decision-making scenarios, like finance. (source)
Calls for greater transparency and accountability have also been widespread, with many commentators urging that AI developers take proactive steps in ensuring the safety of LLMs, especially when augmented by RAG mechanisms. The central concern revolves around the possibility of 'safe' models generating unsafe outputs after RAG implementation. This has led to heightened demands for heightened traceability and the development of integrated safety systems that can predict and mitigate potential risks. The Bloomberg piece highlights that in the absence of such measures, the potential for misuse and harmful outputs could undermine public trust in AI technologies. (source)
Learn to use AI like a Pro
Get the latest AI workflows to boost your productivity and business performance, delivered weekly by expert consultants. Enjoy step-by-step guides, weekly Q&A sessions, and full access to our AI workflow archive.
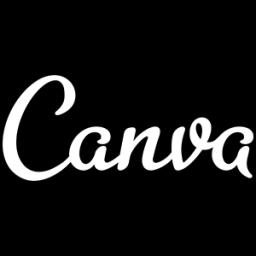
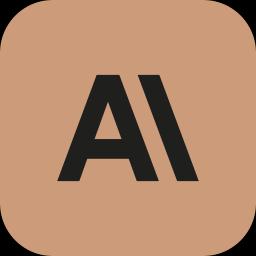
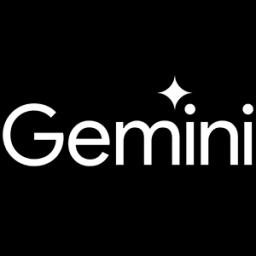
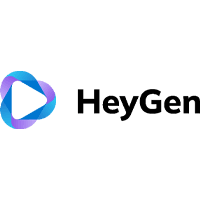
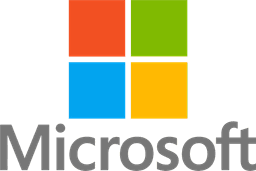
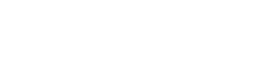
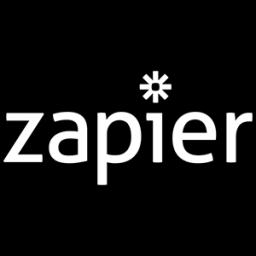
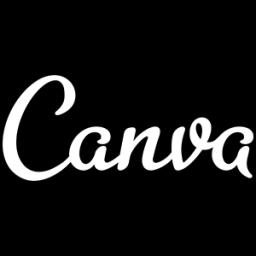
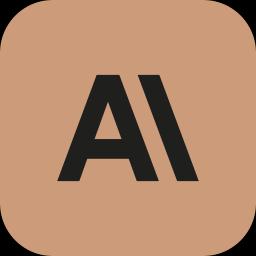
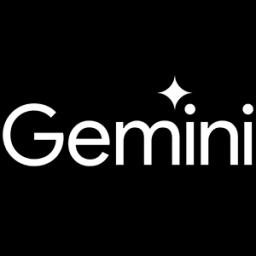
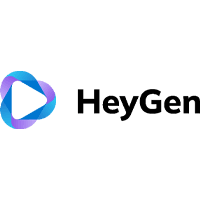
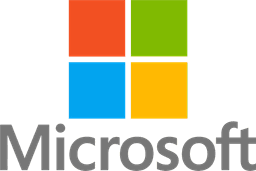
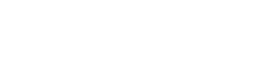
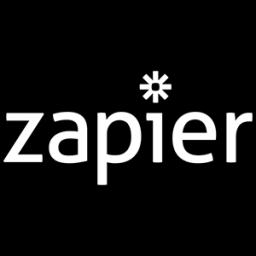
Moreover, professionals in regulated industries such as finance have resonated with Bloomberg's emphasis on the necessity of domain-specific safety approaches. For stakeholders invested in these areas, the findings serve as a catalyst to explore more tailored AI strategies that encompass industry-specific risks, including bias, misconduct, and confidentiality breaches. This aspect of Bloomberg's research sparks a crucial debate about the long-term viability and usage of RAG, pushing for an industry-wide reevaluation of the integration of ambient AI technologies into business operations. (source)
The broader implications of Bloomberg's findings extend beyond immediate industry reactions, potentially fueling debates about the future trajectory of AI technologies. While some argue that the findings may stifle innovation due to increased regulatory scrutiny and the need for thorough safety checks, others believe this moment represents an opportunity to refine and advance AI integrations, ensuring that they align with ethical guidelines and promote trustworthy outputs. The discourse touches on deeper societal and political layers, indicating a shift towards more conscientious AI development strategies. (source)
Future Economic Implications of RAG in AI
The future economic implications of Retrieval Augmented Generation (RAG) in AI are vast and multifaceted, with potential benefits and risks that could shape various industries and economies worldwide. RAG's ability to enhance the accuracy and reliability of Large Language Models (LLMs) by grounding them in external sources is significant, but Bloomberg's research highlights the possibility of unintended vulnerabilities that might lead to serious economic repercussions. If safety measures aren't robust, businesses utilizing RAG-augmented AI could face increased financial losses, especially in high-stakes sectors like finance, where inaccurate content can have costly consequences .
Moreover, the costs associated with implementing and maintaining domain-specific safety measures may slow AI adoption in industries that haven't yet resolved these challenges. Companies that can effectively manage these vulnerabilities and innovate in creating trustworthy AI solutions may stand to gain significant competitive advantage, positioning themselves as leaders in AI integration .
While the revelations of Bloomberg's research emphasize the importance of domain-specific approaches to AI safety, they also point to a rising necessity for investment in AI safety solutions. As a result, there might be an economic shift towards industries that specialize in developing these safety measures, potentially opening new markets and driving economic growth. Additionally, international cooperation in establishing standardized regulations and frameworks could foster a more technologically secure and economically stable global environment .
Social Challenges and Risks Associated with RAG
The integration of Retrieval Augmented Generation (RAG) with Large Language Models (LLMs) introduces several social challenges that stakeholders need to address proactively. One of the primary concerns is the potential amplification of misinformation and harmful content, stemming from the model's enhanced ability to retrieve and generate data that might not always be reliable or safe. This capability can inadvertently serve those looking to spread false narratives or engage in malicious activities. The situation becomes more complex in regulated industries like finance, where misleading information can have catastrophic consequences. Bloomberg's recent research underscores this concern, prompting industry leaders to advocate for stricter implementation of domain-specific safety measures. This strategic move aims to mitigate the proliferation of inaccurate or harmful outputs in critical sectors like finance [source].
Learn to use AI like a Pro
Get the latest AI workflows to boost your productivity and business performance, delivered weekly by expert consultants. Enjoy step-by-step guides, weekly Q&A sessions, and full access to our AI workflow archive.
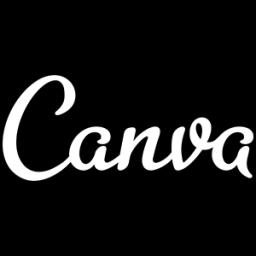
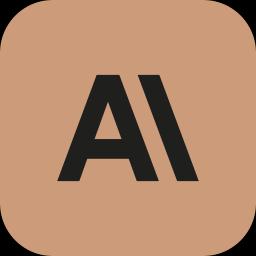
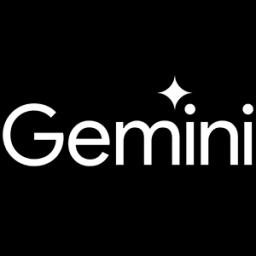
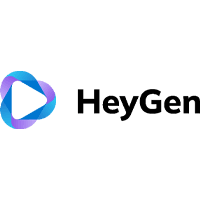
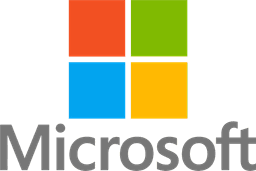
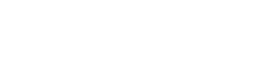
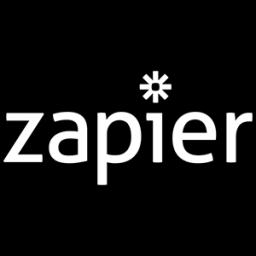
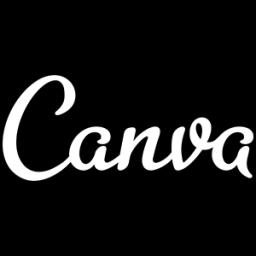
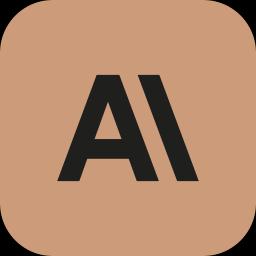
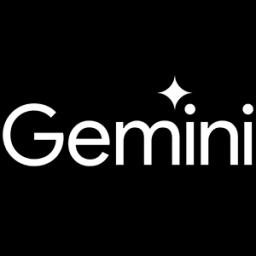
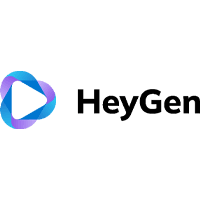
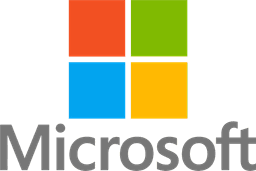
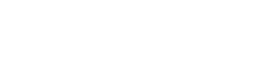
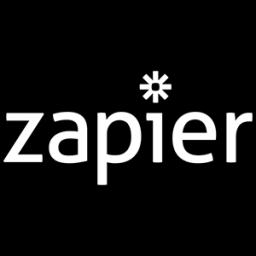
Another significant risk associated with RAG in social contexts is the undermining of public trust in technological institutions and outputs. As models become capable of sophisticated information retrieval and generation, the boundary between fact and fabrication can blur, impacting the trustworthiness of digital interfaces. Users might begin to question the validity of their interactions with these systems, leading to a widespread skepticism of AI-driven outputs. This issue is compounded in environments where AI lacks transparency and explainability, both of which are crucial for fostering trust. The call for more transparent AI, as highlighted by experts like Sebastian Gehrmann from Bloomberg, points to a shift towards creating accountable systems that can offer clearer insights into AI decision-making processes, ensuring these technologies are used responsibly [source].
Privacy concerns also loom large with the adoption of RAG. The enhanced data retrieval capabilities of RAG models heighten the risk of privacy violations, particularly if sensitive information is unintentionally exposed through AI interactions. This potential breach of privacy necessitates the development of robust data protection frameworks to safeguard personal information. The lack of comprehensive privacy standards could lead to public backlash and a demand for stricter regulations. Therefore, integrating ethical guidelines and vigilant monitoring into the design of RAG systems is critical to maintaining an equilibrium between technological advancement and individual privacy rights [source].
As society grapples with these challenges, the role of AI developers and policymakers becomes pivotal. Developing tools and resources tailored to specific industries could mitigate the risks associated with RAG, particularly in high-stakes domains. By doing so, developers can ensure that the deployment of AI technologies does not inadvertently compromise society’s moral and ethical values. Paragraphs from Bloomberg's findings echo the sentiment that more targeted safety taxonomies are essential, alongside integrated safety systems that are sensitive to the interaction between retrieved content and model safeguards. This alignment necessitates a close collaboration between technologists and regulatory bodies to build a resilient AI framework [source].
Political Ramifications and the Need for Regulation
The political ramifications of Bloomberg's findings on Retrieval Augmented Generation (RAG) and its influence on Large Language Models (LLMs) underscore the pressing need for stringent regulation and accountability in AI development. In light of the research, governments worldwide might be compelled to revisit their existing AI policies and introduce more robust frameworks to govern the use of RAG-enhanced LLMs. The EU's AI Act is a prime example of such regulation, focusing on categorizing AI systems based on risk levels, illustrating a proactive approach that could serve as a benchmark for other countries [2](https://www.bloomberg.com/company/press/bloomberg-ai-researchers-mitigate-risks-of-unsafe-rag-llms-and-genai-in-finance/).
Striking a balance between fostering innovation and ensuring public safety will be a key challenge for policymakers. The need for regulations arises not only from the safety risks posed by RAG but also from the potential use of AI technologies to manipulate democratic processes and influence elections. In this context, international cooperation is paramount to create a unified front that can mitigate the risks posed by disparate national policies [3](https://www.bloomberg.com/company/press/bloomberg-ai-researchers-mitigate-risks-of-unsafe-rag-llms-and-genai-in-finance/). Such collaboration is essential to prevent a mix of regulations that could stifle innovation while not adequately shielding citizens from AI's unintended consequences.
The potential for misuse of AI technologies, such as spreading propaganda or conducting targeted misinformation campaigns, highlights the urgency for political bodies to establish transparent standards. These standards should be aimed at creating AI systems that are both accountable and explainable. This focus on explainability echoes related concerns in AI ethics, where understanding and interpreting AI decision-making processes are crucial for maintaining trust across all levels of society [2](https://www.bloomberg.com/company/press/bloomberg-ai-researchers-mitigate-risks-of-unsafe-rag-llms-and-genai-in-finance/).
Learn to use AI like a Pro
Get the latest AI workflows to boost your productivity and business performance, delivered weekly by expert consultants. Enjoy step-by-step guides, weekly Q&A sessions, and full access to our AI workflow archive.
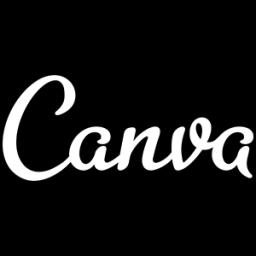
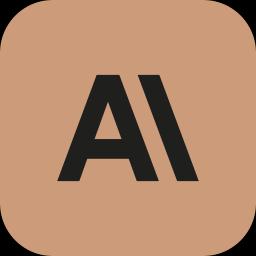
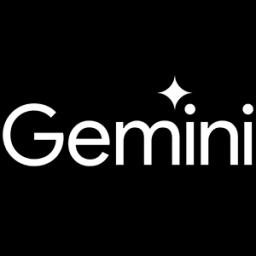
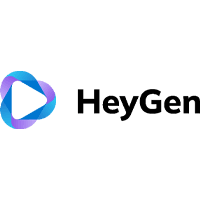
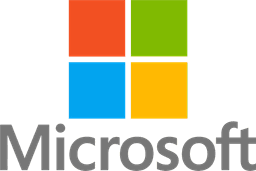
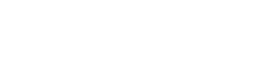
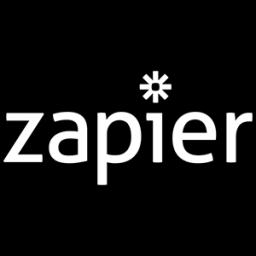
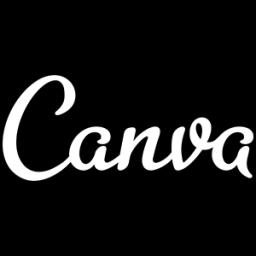
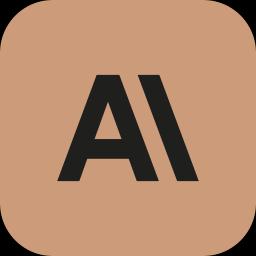
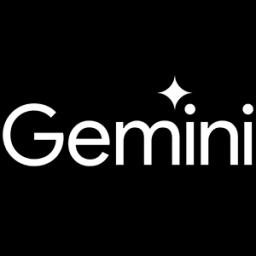
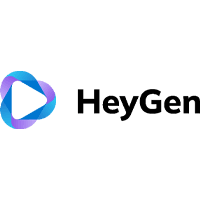
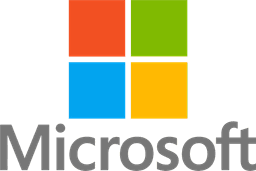
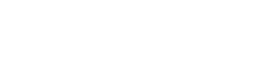
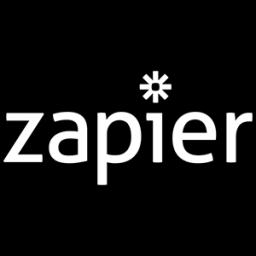
Furthermore, the political discourse around the safety of RAG and LLMs indicates the necessity for domain-specific safety measures. Domain-specific taxonomies, such as those emphasized by Bloomberg, provide tailored safety strategies to address unique challenges within contexts like the financial sector [1](https://venturebeat.com/ai/does-rag-make-llms-less-safe-bloomberg-research-reveals-hidden-dangers/). Such targeted approaches are likely to gain traction, potentially influencing legislative agendas focused on AI governance.
Finally, the scrutiny of Bloomberg's stance on AI safety may lead to broader discussions on industry self-regulation versus government intervention. While Bloomberg asserts the integration of AI in augmenting its financial services, the potential conflict of interest highlights the dual nature of industry-led research, which can either advance market interests or contribute to responsible and ethical AI development [1](https://venturebeat.com/ai/does-rag-make-llms-less-safe-bloomberg-research-reveals-hidden-dangers/). This duality invites political debate over the most effective pathways to achieving safe and equitable AI innovation and application.
Bloomberg's Perspective on Transparency and Bias
Bloomberg's research into the effects of Retrieval Augmented Generation (RAG) on Large Language Models (LLMs) has sparked significant discussion around transparency and bias. According to a VentureBeat article, Bloomberg has revealed critical insights that challenge the perceived safety of these AI systems. While RAG is supposed to enhance accuracy by grounding outputs in factual data, Bloomberg's findings suggest that it might actually bypass safety protocols, leading to unexpected, unsafe outputs. This has raised questions about transparency in AI systems, as many stakeholders believe that the lack of clarity in AI decision processes is a fundamental issue that needs to be addressed. Indeed, Bloomberg's focus on creating domain-specific safety measures, especially within their own financial services sector, underscores the importance of tailored approaches to mitigate risks inherent in advanced AI applications.
A crucial aspect of Bloomberg's findings is the potential for RAG to introduce new biases or amplify existing ones within AI models. While RAG is designed to inject external knowledge into AI outputs, the sources and contexts selected for retrieval may inadvertently reflect biases that persist in data. As acknowledged by Amanda Stent, Head of AI Strategy and Research at Bloomberg, this has significant implications across various applications, from customer support to financial advisories. The company emphasizes the need for rigorous, scenario-specific testing and validation to minimize these biases. According to Bloomberg's press release, the challenge is compounded by the black-box nature of many AI systems, which obscures the pathways through which biases might influence model behavior. To address this, Bloomberg advocates for explainable AI to ensure that users can understand and trust the decisions generated by these systems.
Bloomberg’s commitment to transparency goes beyond theoretical discussions; it is reflected in their operational strategies and research directives. By developing a specialized AI content risk taxonomy for the financial sector, Bloomberg is actively working to identify and mitigate risks that generic frameworks might overlook. This reflects a broader industry trend where domain-specific issues demand tailored solutions. Furthermore, as highlighted by David Rabinowitz, Technical Product Manager for AI Guardrails at Bloomberg, there is an urgency in emphasizing context-driven evaluations over generalized safety claims. Rabinowitz's insights, shared in recent communications, argue for the necessity of contextual understanding in AI system evaluations—in part a response to the complex compliance landscape of financial services where undeclared risks can have far-reaching consequences.
In light of these findings, Bloomberg stresses the importance of integrating safety measures directly into RAG implementations, rather than relying solely on external safety frameworks. This approach not only addresses potential biases but also aligns with increasing demands for accountability in AI development. As regulators and industry leaders push for transparency, Bloomberg sees its proactive stance as fundamental to setting the standard for responsible AI usage in the financial sector. Ensuring traceability within AI systems—an explicit goal outlined in their recent studies—aims to provide users with confidence in the systems they deploy, ultimately fortifying public trust in AI innovations. Yet, amidst these efforts, Bloomberg remains cognizant of its dual role as both a researcher and industry player, acknowledging potential conflicts of interest due to its substantial stakes in the finance industry—thus aiming to separate business incentives from ethical AI stewardship.
Learn to use AI like a Pro
Get the latest AI workflows to boost your productivity and business performance, delivered weekly by expert consultants. Enjoy step-by-step guides, weekly Q&A sessions, and full access to our AI workflow archive.
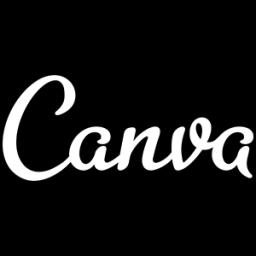
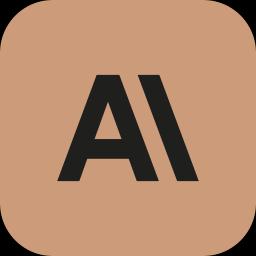
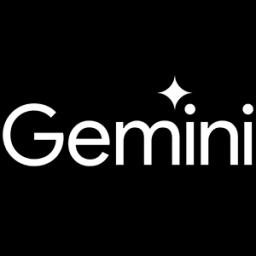
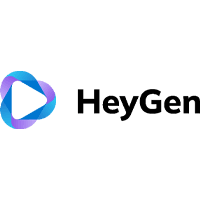
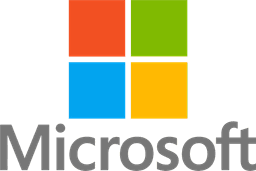
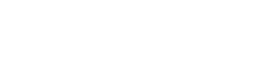
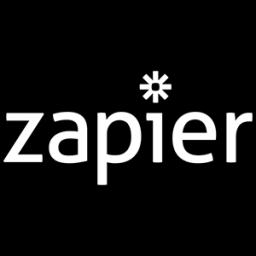
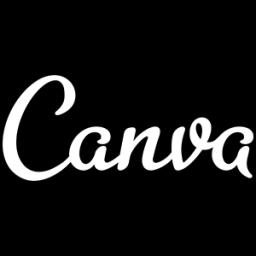
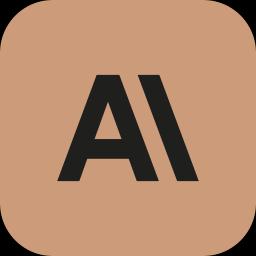
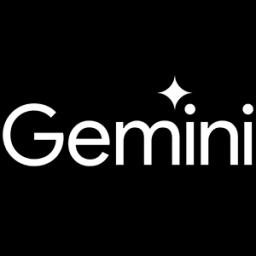
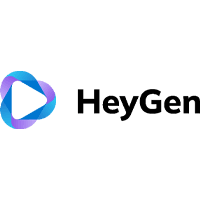
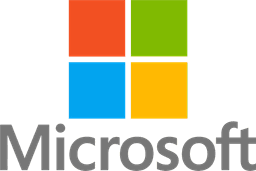
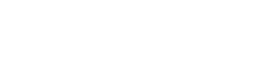
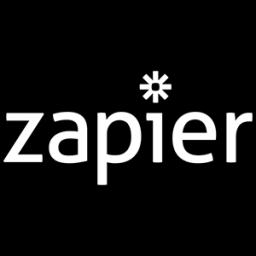
The broader implications of Bloomberg's research suggest a pivotal moment for industries reliant on AI technologies. The findings call for a reevaluation of current AI transparency and bias mitigation strategies, especially within critical sectors like finance. The revelations that even "safe" AI systems can generate harmful outputs underscore the need for continuous scrutiny and improvement of AI frameworks. As Sebastian Gehrmann, Head of Responsible AI at Bloomberg, notes, establishing clear guidelines and robust safety measures is crucial for the evolution of secure AI practices. Such measures not only enhance operational transparency but also assure ethical integrity in AI-assisted decision-making processes. As the global discourse around AI accountability intensifies, Bloomberg's perspective offers a valuable blueprint for fostering a more transparent and unbiased AI landscape.