Forecasting the Future, Faster
GenCast Revolutionizes Weather Forecasting with AI Precision
Last updated:

Edited By
Mackenzie Ferguson
AI Tools Researcher & Implementation Consultant
Google DeepMind's GenCast AI system has taken weather forecasting by storm, outperforming leading systems with 20% more accuracy. This AI marvel harnesses 40 years of data to predict storms and severe weather in just 8 minutes, a dramatic improvement over traditional methods. However, experts caution that while GenCast impresses, it may have limitations in replicating the complex dynamics of atmospheric phenomena like the butterfly effect.
Introduction to GenCast: The Innovative AI Weather Forecasting System
Google DeepMind recently released GenCast, an innovative AI system for weather forecasting. In groundbreaking developments, GenCast has significantly surpassed the existing European Centre for Medium-Range Weather Forecasts' (ECMWF) ENS system. Harnessing machine learning and analyzing over 40 years of weather data, GenCast improves prediction accuracy and speed for various weather events, including hurricanes. Remarkably, GenCast generates forecasts in just 8 minutes, a stark contrast to the hours required by conventional physics-based methods utilizing supercomputers. However, some experts question GenCast's ability to replicate real-world atmospheric phenomena, such as the butterfly effect, and its potential to completely replace traditional physics-based forecasting methods.
For many years, scientists and meteorologists have relied on complex physical models and supercomputers to predict weather patterns. These traditional models, though advanced, have their limits in terms of computational expense and reaction time. GenCast, by leveraging a monumental dataset, offers superior accuracy with a 20% improvement over ECMWF systems, particularly in short-term weather predictions and severe weather events. This AI-driven methodology presents a paradigm shift in how we approach meteorological forecasting.
Learn to use AI like a Pro
Get the latest AI workflows to boost your productivity and business performance, delivered weekly by expert consultants. Enjoy step-by-step guides, weekly Q&A sessions, and full access to our AI workflow archive.
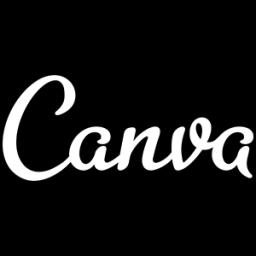
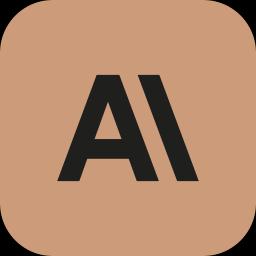
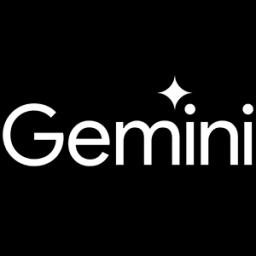
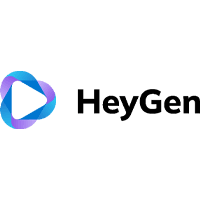
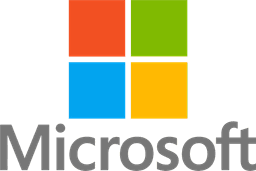
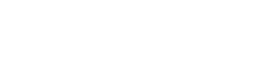
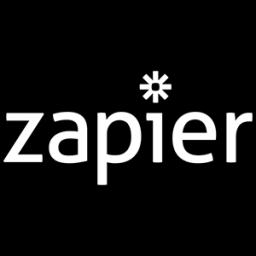
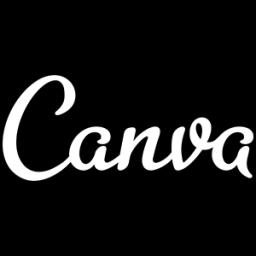
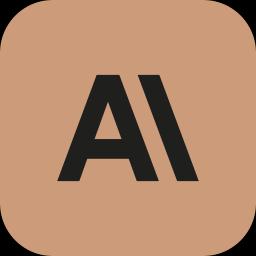
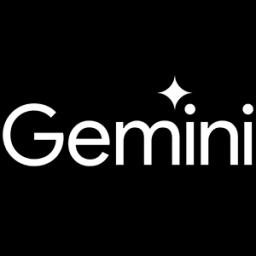
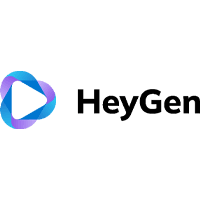
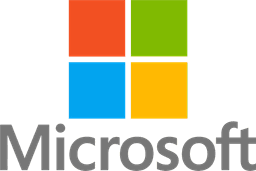
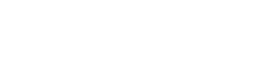
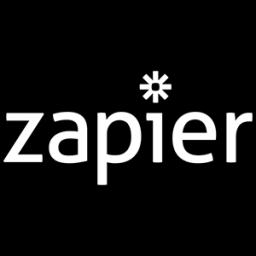
GenCast's method involves creating numerous forecast scenarios through rigorous data analysis. Using Google's Cloud Tensor Processing Units (TPUs), the system simulates multiple outcomes by drawing from decades of accumulated weather data, offering predictions in as little as 8 minutes. This technological leap not only outpaces traditional methods but also holds the potential for developing larger ensemble forecasts by national meteorological services, contributing to better reliability and preparedness for extreme weather conditions.
Despite GenCast's impressive advancements, the adoption of AI-based forecasting is not without challenges. Questions arise about the model's extrapolation from historical data, especially in contexts heavily influenced by climate change. Furthermore, certain meteorologists express concerns regarding the system’s handling of chaotic weather dynamics, such as the butterfly effect in long-term forecasts. Thus, experts argue for GenCast to work alongside traditional models to maximize forecasting gains, reflecting a hybrid approach as opposed to replacement.
Public reaction to GenCast has been a mix of optimism and caution. On one hand, many celebrate the system's potential to advance weather prediction to unprecedented levels, impacting sectors like disaster preparedness and renewable energy planning. On the other hand, there is hesitance; stakeholders advocate for extensive testing and validation to confirm GenCast's reliability in capturing complex meteorological phenomena. There’s a consensus on the need for ongoing scrutiny and research to ensure that AI does not overreach, but rather complements, established forecasting tools.
Moving forward, GenCast could influence numerous aspects of society and the global economy. By delivering accurate, rapid forecasts, GenCast could revolutionize industries reliant on weather determinations, ranging from agriculture to transportation and energy. These capabilities may enhance disaster response effectiveness, ultimately saving lives and resources. Additionally, as countries explore GenCast's applications, its adoption could spark collaboration and innovation across international meteorological and AI communities. Nonetheless, transparency and maintaining an equilibrium between AI advancements and traditional methodologies remain vital to addressing climate challenges effectively.
Learn to use AI like a Pro
Get the latest AI workflows to boost your productivity and business performance, delivered weekly by expert consultants. Enjoy step-by-step guides, weekly Q&A sessions, and full access to our AI workflow archive.
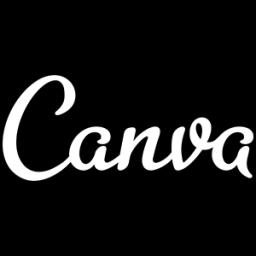
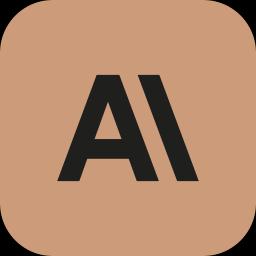
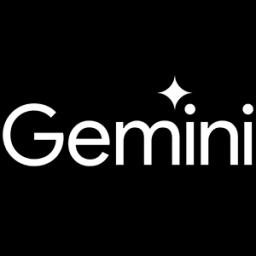
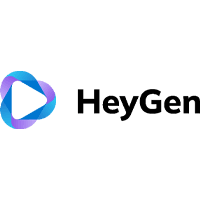
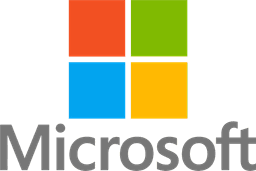
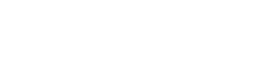
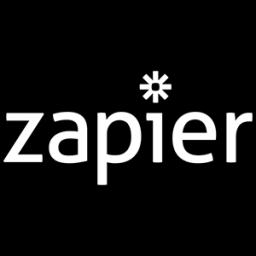
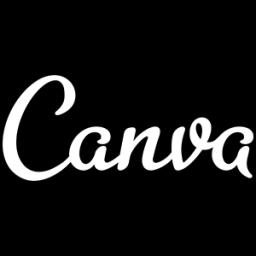
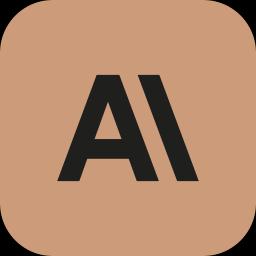
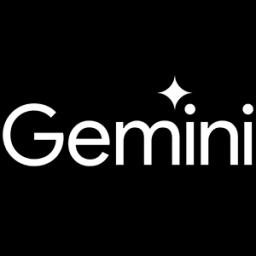
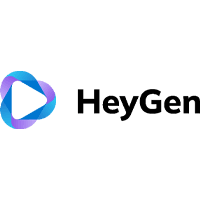
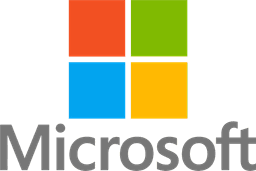
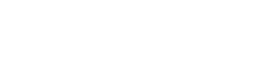
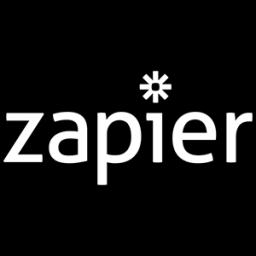
Comparative Accuracy: GenCast vs Traditional Forecasting Models
Google DeepMind's latest innovation, GenCast, has emerged as a frontrunner in the field of weather forecasting, boasting impressive advances that outshine traditional models. The recent comparison with the renowned ENS system from the European Centre for Medium-Range Weather Forecasts (ECMWF) reveals GenCast's ability to deliver forecasts that are a full 20% more accurate. This level of precision not only marks a significant leap forward in meteorology but also highlights the evolving capabilities of AI-powered models in handling complex predictive tasks that were once the sole purview of physics-based systems.
GenCast's strengths lie in its ability to harness the power of machine learning, building upon 40 years of historical weather data. This approach allows the model to accurately predict a range of weather events, ranging from the everyday nuances of day-to-day changes to the volatile paths of hurricanes and tropical cyclones. Such capabilities are critical, especially in regions prone to severe weather patterns where timely and precise forecasts can mean the difference between safety and disaster.
The speed at which GenCast operates further sets it apart from traditional forecasting models. It uses the power of Google Cloud TPU to generate a comprehensive set of forecasts in mere minutes – a task that typically requires several hours for traditional physics-based models that rely heavily on supercomputing resources. This not only illustrates the efficiency of AI-driven models but also underscores the potential for timely decision-making in sectors reliant on accurate weather data.
Despite its impressive performance, GenCast does not come without its challenges and criticisms. Questions linger regarding its ability to fully replicate atmospheric complexities, like the "butterfly effect," which can significantly alter weather predictions. Critics also voice concerns about potential limitations of using solely historical data, especially in an era where climate change is rapidly altering weather patterns and introducing unprecedented variables that might not be captured by past data.
The deployment of GenCast represents a pivotal moment in weather forecasting, with implications that extend beyond the scientific community. Economically, its accuracy and swift processing can revolutionize industries such as agriculture and energy, which heavily depend on reliable weather predictions. Socially, the model holds potential for dramatically improving disaster preparedness and response, potentially saving lives by providing accurate advance warnings. As the world increasingly faces extreme weather events, GenCast's introduction could mark a turning point in the integration of AI in vital societal applications.
Looking ahead, while the benefits of AI models like GenCast are promising, it is recommended that they complement rather than replace existing forecasting methods. A hybrid approach that integrates the robustness of traditional physics-based models with the speed and agility of AI could offer the most comprehensive weather forecasting solution. This dual-approach might ensure accuracy and reliability across diverse meteorological scenarios, providing a more robust defense against the unpredictable forces of nature.
Learn to use AI like a Pro
Get the latest AI workflows to boost your productivity and business performance, delivered weekly by expert consultants. Enjoy step-by-step guides, weekly Q&A sessions, and full access to our AI workflow archive.
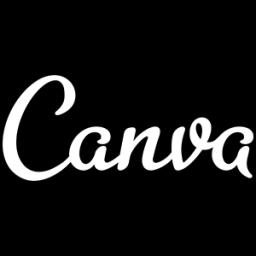
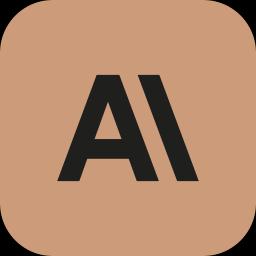
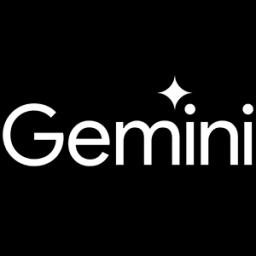
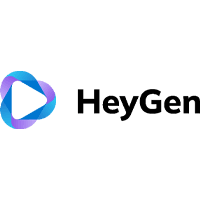
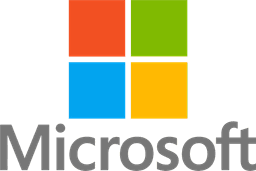
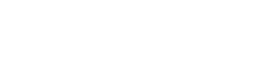
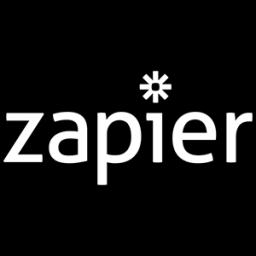
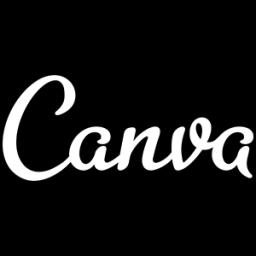
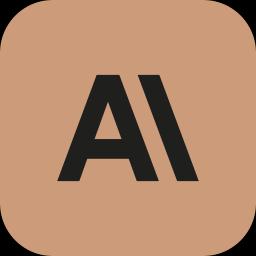
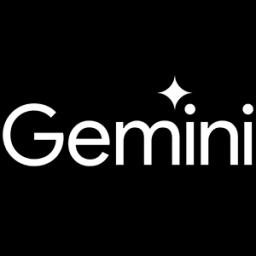
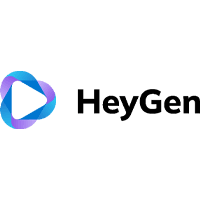
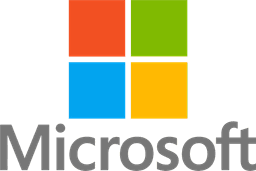
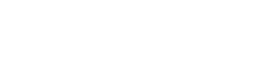
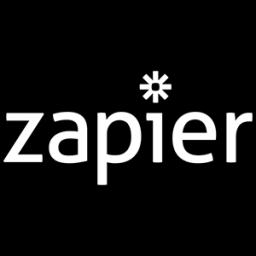
Unveiling Weather Events: GenCast's Predictive Capabilities
Google DeepMind's latest advancement in weather forecasting, GenCast, showcases the remarkable capabilities of artificial intelligence in predicting complex atmospheric phenomena. Unlike traditional systems that rely on physics-based models and extensive computation time, GenCast leverages machine learning techniques coupled with 40 years of historical weather data to enhance both the speed and accuracy of weather forecasts. It outstrips the European Centre for Medium-Range Weather Forecasts (ECMWF) by delivering forecasts in under 10 minutes, offering quicker insights into weather events like hurricanes and cyclones, which are crucial for timely disaster response and management.
One of the key strengths of GenCast lies in its ability to predict extreme weather events with enhanced precision. By simulating over 50 different forecast scenarios, it provides a probabilistic ensemble that can more accurately pinpoint the trajectories of storms and the fluctuations of daily weather conditions. This represents a significant improvement over traditional methods, which often cannot match the rapid computation speed without sacrificing reliability. As a result, GenCast has become an invaluable tool for weather services aiming to provide more robust and timely warnings to mitigate the impacts of severe weather conditions.
However, the rise of AI-driven weather forecasting methods like GenCast also introduces challenges and prompts important questions within the scientific community. Concerns about the physical realism of AI models, particularly in capturing the full complexities of atmospheric dynamics such as the 'butterfly effect', remain persistent. This refers to the sensitivity of systems where small changes can lead to vastly different outcomes. Additionally, the heavy reliance on historical data raises questions about GenCast’s ability to adapt to future shifts influenced by climate change, potentially limiting its forecasting accuracy in novel conditions.
GenCast's development also highlights a broader trend among tech giants in harnessing AI to solve complex meteorological challenges. Similar advancements, such as Nvidia's StormCast and IBM's collaborative project with NASA, indicate growing recognition of AI's potential to revolutionize weather forecasting. These systems hold promise not only for improving emergency response but also for optimizing sectors like agriculture and energy, where weather plays a critical role in operational planning and risk management. As these technologies evolve, they underscore the importance of interdisciplinary collaboration and innovation in addressing global weather and climate-related challenges.
Public reception to GenCast is cautiously optimistic, with many recognizing its substantial potential benefits alongside certain hesitations. On one hand, the AI model's accelerated processing time and improved accuracy are celebrated as transformative for industries and emergency preparedness. On the other, skeptics urge for continued evaluation to ensure these enhancements translate comprehensively from AI simulations to real-world conditions. Concerns about dependency on AI over proven methods persist, yet many agree that GenCast represents a valuable addition to existing forecasting tools, advocating a hybrid approach that integrates both AI and traditional methods for the most comprehensive weather predictions.
Accelerated Forecasting: Speed and Efficiency of GenCast
GenCast, Google DeepMind's AI weather forecasting system, marks a significant leap forward in the realm of meteorological predictions by blending cutting-edge machine learning techniques with extensive historical weather data. Its name, GenCast, indicates its generative capabilities, enabling it to produce multiple forecast scenarios rapidly. Equipped with the ability to analyze 40 years of global weather data, GenCast is not only faster but also consistently outperforms traditional systems such as those used by the European Centre for Medium-Range Weather Forecasts (ECMWF).
Learn to use AI like a Pro
Get the latest AI workflows to boost your productivity and business performance, delivered weekly by expert consultants. Enjoy step-by-step guides, weekly Q&A sessions, and full access to our AI workflow archive.
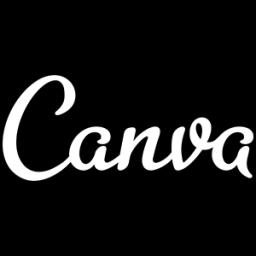
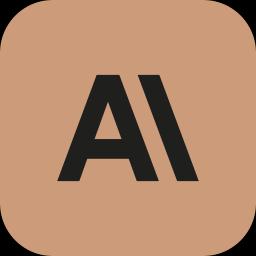
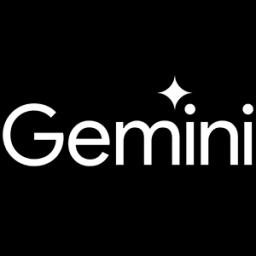
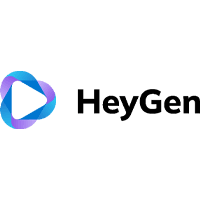
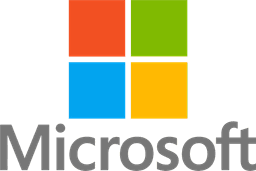
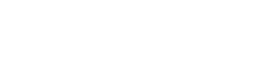
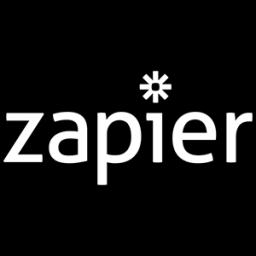
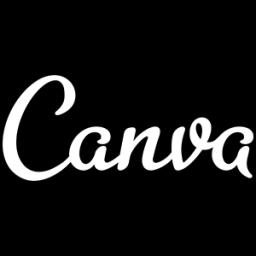
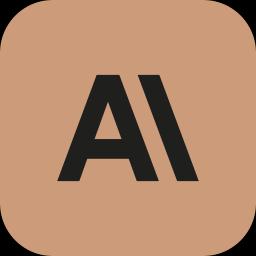
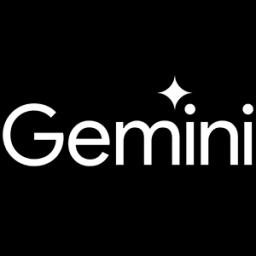
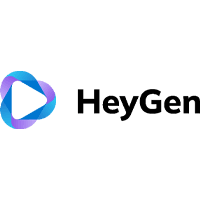
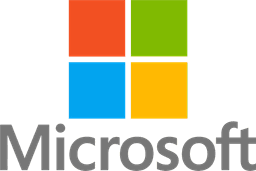
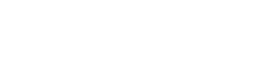
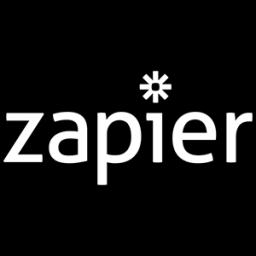
In stark contrast to conventional methods, which depend heavily on physics-based models run on supercomputers for several hours, GenCast can generate forecasts in approximately 8 minutes using Google Cloud's TPUs. This significant decrease in processing time does not sacrifice accuracy, as GenCast is touted to be about 20% more precise, particularly in predicting the trajectories of hurricanes and the day-to-day variations in weather patterns. Such improvements could redefine standard practices in weather forecasting, offering timely insights critical for sectors reliant on weather data, ranging from agriculture to disaster response.
Despite the high expectations surrounding GenCast, there remains a spectrum of challenges particularly related to the underlying complexities and uncertainties inherent in atmospheric dynamics. The 'butterfly effect,' a term popularized in chaos theory, exemplifies these challenges by suggesting how small changes in initial conditions can result in vastly different outcomes, posing a significant hurdle for any forecasting model, especially those dependent on machine learning algorithms. Critics emphasize the importance of GenCast's ability to realistically replicate this complexity to foster trust and enhance predictive accuracy.
The emergence of AI forecasting models like GenCast and its counterparts, NeuralGCM and GraphCast, heralds a broader transformative shift within meteorological sciences and beyond. By integrating AI with traditional physics-based forecasting, these models not only optimize computational demands but also improve the accuracy of predictions. This hybrid approach, as demonstrated by NeuralGCM, which blends both methodologies, is an indication of future directions in weather forecasting: harnessing the strengths of AI while maintaining the foundational principles of traditional meteorology.
The Advantages of AI-Enhanced Weather Forecasting
Artificial intelligence (AI) is revolutionizing the field of weather forecasting, as demonstrated by systems like Google DeepMind's GenCast. This advanced AI-generated forecasting model has been shown to outperform the traditional European Centre for Medium-Range Weather Forecasts (ECMWF) model by a significant margin. GenCast achieves this by employing machine learning techniques, which are trained on an extensive dataset of 40 years of historical weather data. Such a comprehensive training regime allows GenCast to craft more accurate and efficient predictive models, especially for complex weather events like hurricanes and day-to-day weather variations. This improvement in accuracy is crucial for areas reliant on precise weather predictions, such as agriculture, disaster preparedness, and energy management.
The speed at which GenCast produces forecasts is another major advantage over traditional systems. While conventional weather forecasting methods can take several hours to generate predictions, GenCast operates with remarkable efficiency, delivering forecasts in just about 8 minutes using Google Cloud's TPU. This reduction in time not only makes GenCast a faster option but also allows for the creation of larger ensemble forecast sets. The size of these ensembles substantially increases the reliability and robustness of predictions, particularly for extreme weather events. This speed and scalability position AI systems like GenCast as critical tools for meteorology, where timely and accurate information can have significant impacts on safety and economic planning.
Despite these advantages, AI-driven forecasting models such as GenCast also come with their share of challenges. One primary concern is the physical realism of these models, especially in capturing the entire breadth of atmospheric phenomena. Issues such as the 'butterfly effect,' which describes how small changes can lead to vastly different outcomes, pose significant obstacles in modeling the full complexity of the atmosphere. Moreover, while GenCast provides superior short-term forecasts, its reliance on historical data might limit its efficacy in scenarios that are significantly influenced by the ongoing shifts in climate patterns. Researchers advocate for a hybrid approach, complementing AI model strengths with traditional physics-based systems to overcome these limitations.
Learn to use AI like a Pro
Get the latest AI workflows to boost your productivity and business performance, delivered weekly by expert consultants. Enjoy step-by-step guides, weekly Q&A sessions, and full access to our AI workflow archive.
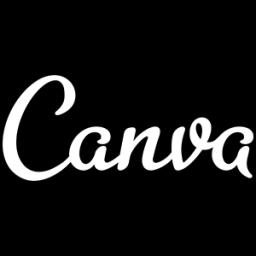
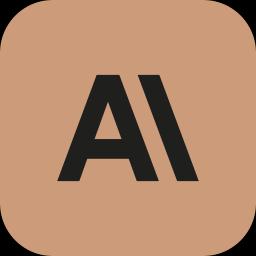
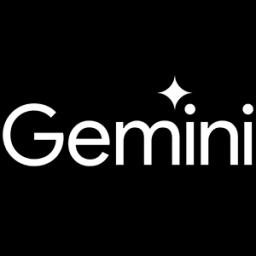
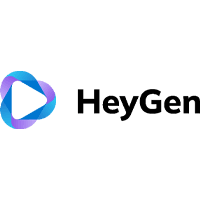
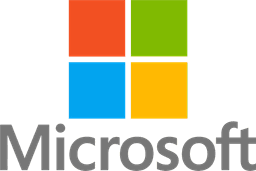
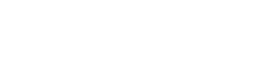
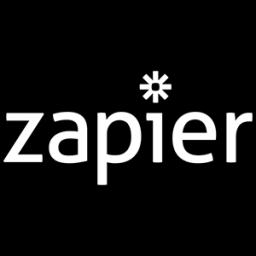
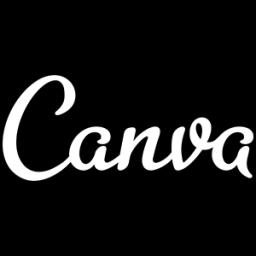
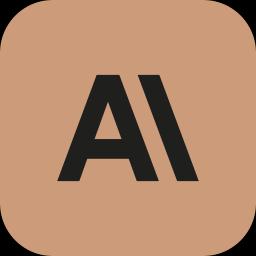
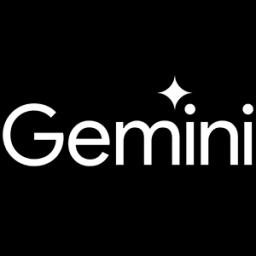
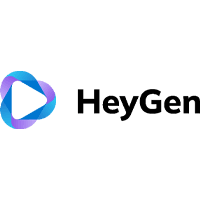
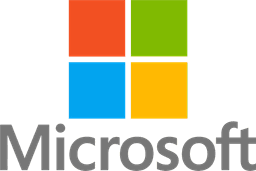
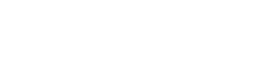
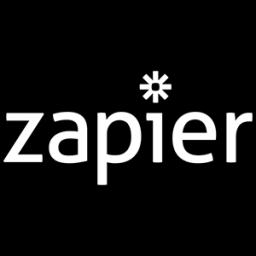
Challenges and Concerns: AI vs Physics-Based Forecasting
The advent of AI-based weather forecasting systems, such as Google DeepMind's GenCast, presents a significant departure from traditional physics-based methods. While these AI models promise improved accuracy and faster generation of weather forecasts, they face several challenges and concerns that need examination. GenCast, for example, has been trained on 40 years of historical weather data, which raises questions about its ability to account for unprecedented weather patterns driven by climate change.
The "butterfly effect," a concept that describes how small changes in one part of a system can lead to significant variations in another, is a point of contention in AI weather forecasting. Critics argue that AI models, including GenCast, may struggle to fully encapsulate the chaotic nature of atmospheric dynamics. This complexity, often managed through sophisticated computational physics in traditional models, presents a hurdle for AI systems making long-range weather predictions.
Another concern is the physical realism that AI models can provide. While AI can simulate multiple forecast scenarios quickly, there is a debate about how accurately these represent real-world atmospheric processes. Unlike physics-based models, which rely on fundamental equations to depict the atmosphere's behavior, AI models hinge on pattern recognition from historical data, potentially limiting their applicability in novel or extreme situations.
Despite these challenges, the potential benefits of AI in weather forecasting cannot be overstated. By drastically reducing the time required to produce forecasts, AI systems like GenCast can offer more timely insights during critical weather events, aiding disaster preparedness and response. Furthermore, these models enable the generation of larger forecast ensembles, thus enhancing the reliability of predictions.
There is also an underlying concern about the role of AI in weather forecasting overshadowing the need for continued use of traditional methods. The consensus among experts suggests that a hybrid approach that combines AI with physics-based methodologies could yield the most reliable and comprehensive forecasting systems. This integration aims to leverage the strengths of both AI's speed and data processing capabilities with the proven accuracy of physics-based modeling.
In summary, while AI weather forecasting systems like GenCast are proving to be groundbreaking, they are not without their set of challenges. Addressing these concerns, particularly around physical realism and data limitations, is crucial for these systems to gain broader acceptance and reliability in the meteorological community. As technology continues to evolve, a collaborative approach that includes AI and traditional methods will likely be the path forward.
Learn to use AI like a Pro
Get the latest AI workflows to boost your productivity and business performance, delivered weekly by expert consultants. Enjoy step-by-step guides, weekly Q&A sessions, and full access to our AI workflow archive.
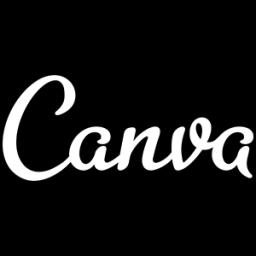
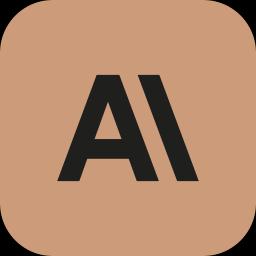
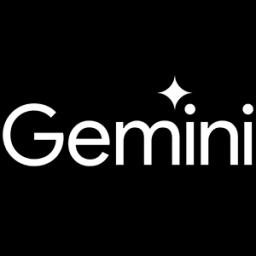
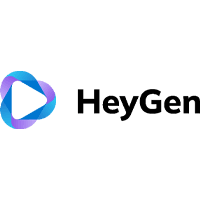
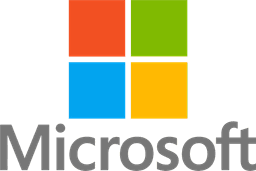
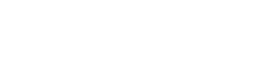
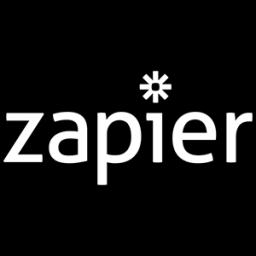
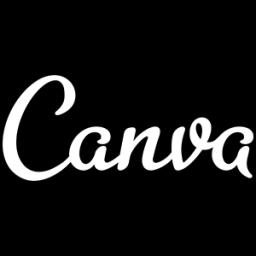
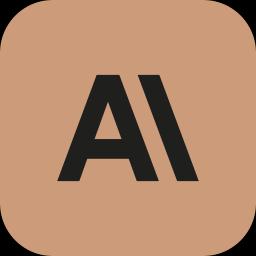
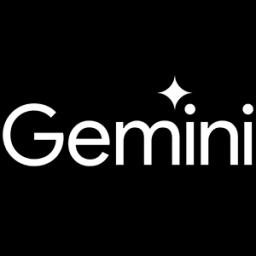
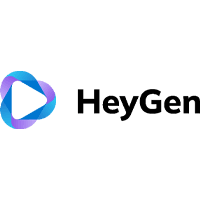
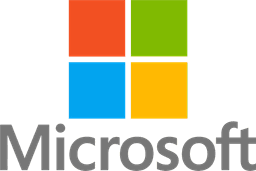
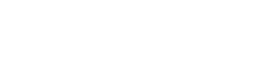
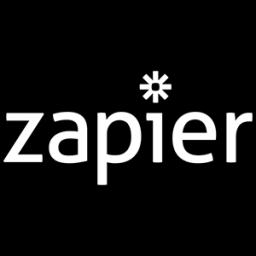
Experts Weigh In: Opinions on GenCast's Impact
Google DeepMind's GenCast has disrupted the weather forecasting industry by improving prediction accuracy by 20% over traditional methods. Experts in meteorology express both amazement and caution regarding its capabilities. On one hand, the AI's proficiency in analyzing 40 years of weather data rapidly and efficiently is a game changer. However, there are concerns about its ability to capture the full complexity of atmospheric phenomena. The model's rapid processing speed promises faster preparedness for extreme weather conditions, which is increasingly vital in our changing climate.
Professor Sarah Dance from the University of Reading highlights potential issues with AI models like GenCast when dealing with phenomena such as the "butterfly effect," which impacts long-term predictions. Her insights stress the need for caution in relying solely on historical data, as climate change can introduce unprecedented variables not accounted for in the model's training. Her concerns echo throughout the scientific community, emphasizing the need for a hybrid approach combining AI with proven physics-based methodologies for optimal results.
The enthusiasm from industry leaders like Steven Ramsdale at the Met Office underscores GenCast’s potential to transform disaster response frameworks and energy management systems. The advancement presented by GenCast illustrates the potential for AI to revolutionize traditional fields and improve public safety measures significantly. Yet, the consensus among experts is that while GenCast sets a new standard in weather technology, it complements rather than replaces the depth offered by traditional forecast models.
Public reaction to GenCast reflects a blend of optimism and caution. On platforms like Reddit and various online forums, users express excitement, anticipating the capabilities of GenCast to enhance weather-related safety and planning. Nonetheless, there remains a cautious edge, with discussions revolving around AI's reliability compared to physics-based systems regarding long-term and potentially life-altering forecasts. Critics call for a balanced approach, advocating for GenCast’s use as part of a larger collaborative forecasting framework.
Looking ahead, the potential influences of GenCast extend beyond meteorology into economic and social spheres. Its forecast accuracy can influence agricultural outputs and aid transportation logistics by offering reliable weather data proactively. Additionally, the use of AI in weather prediction stands to bolster public confidence in technological tools, encouraging integrations across various sectors where such precision can drive efficiency and safety. However, political discourse around data privacy and the ethics of AI deployment continues, warranting cautious optimism as GenCast paves new pathways in forecasting.
Public Reception: Excitement and Skepticism
Public reactions to Google DeepMind's GenCast weather forecasting system have been varied, encompassing both excitement and skepticism. Enthusiasts on social media platforms and various forums celebrate GenCast's ability to enhance the precision and speed of weather predictions. The system's reported 20% improvement in accuracy compared to existing systems like the ECMWF, particularly in forecasting daily weather and extreme events, is lauded as potentially game-changing. This development is seen as a breakthrough in weather forecasting, akin to achieving decades of progress within a single year. Many express optimism about its applications in areas such as renewable energy planning and disaster preparedness, foreseeing considerable advancements in these fields.
Learn to use AI like a Pro
Get the latest AI workflows to boost your productivity and business performance, delivered weekly by expert consultants. Enjoy step-by-step guides, weekly Q&A sessions, and full access to our AI workflow archive.
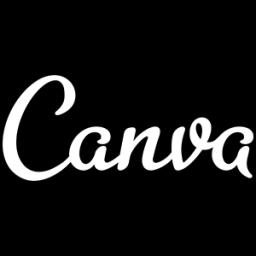
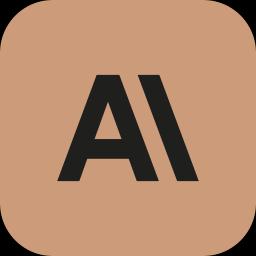
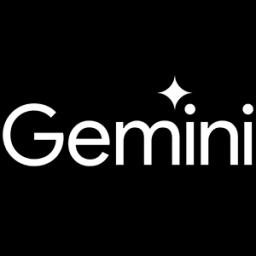
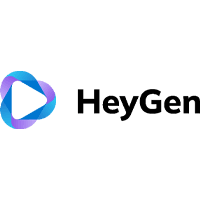
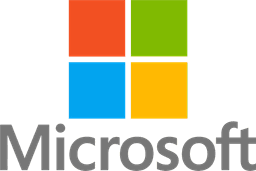
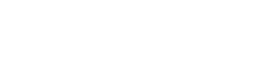
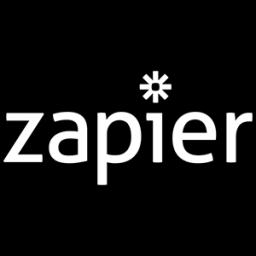
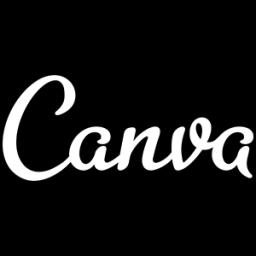
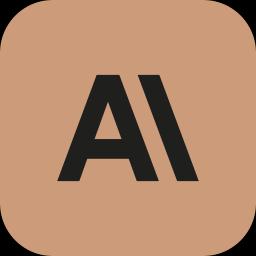
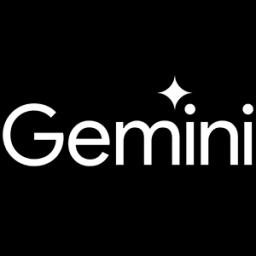
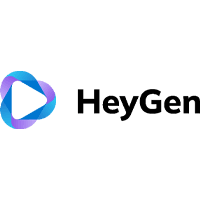
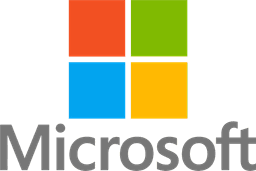
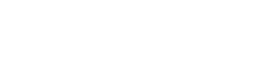
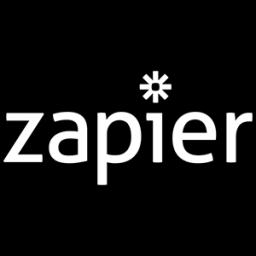
However, alongside the excitement exists a cautious optimism, as critics emphasize the need for further evaluation of GenCast’s capabilities. Concerns are raised regarding the system's proficiency in managing the complex dynamics of weather phenomena, including its ability to account for the "butterfly effect" and its performance in long-range forecasting. Doubts persist about whether AI-based models like GenCast can genuinely replace the reliability of traditional physics-based forecasting methods. Skeptics call for continuous research and validation to confirm that GenCast can meet the high reliability standards demanded for widespread adoption, especially given that even state-of-the-art AI systems can occasionally falter.
Generally, the public appreciates the potential GenCast represents but acknowledges the necessity of verifying its robustness and accuracy over a more extended period before it can be entrusted with crucial weather forecasting tasks. The system's open-source nature is welcomed, as it allows for greater scrutiny and collaborative improvements from the global research community. The prevailing sentiment is one of cautious optimism: while there is a recognition of GenCast's impressive capabilities, there remains a collective understanding that traditional methods should not be entirely forsaken, advocating for a future where AI augments rather than replaces existing forecasting frameworks.
Future Implications: Economic, Social, and Political Impact of GenCast
The emergence of GenCast as a leading AI weather forecasting system presents a myriad of potential future implications across economic, social, and political spheres. Economically, industries that are heavily dependent on accurate weather forecasting, such as agriculture, energy, and transportation, are likely to experience significant transformations. The ability to accurately predict weather with greater precision can optimize operations, reduce costs, and prevent losses resulting from unforeseen weather conditions. In particular, renewable energy sectors stand to benefit from improved grid management and cost efficiency, as GenCast's enhanced forecast accuracy facilitates better planning for energy production and distribution.
Socially, GenCast holds the promise of drastically enhancing disaster preparedness and response strategies. Accurate and timely warnings about extreme weather events such as hurricanes and cyclones can save lives, prevent property damage, and reduce economic disruption. By providing reliable forecasts, GenCast can foster greater public trust in AI technologies, encouraging additional integration of AI solutions in other areas of society. Moreover, as households and communities become more reliant on advanced weather forecasting, there is potential for increased civic engagement and proactive planning in response to climate-related challenges.
On the political front, the deployment of GenCast could stimulate both international cooperation and competition. Countries may engage collaboratively to share data and improve forecasting accuracy globally, or they might strive competitively to leverage advanced meteorological technologies within national infrastructures. These dynamics could become particularly relevant in discussions around climate policy and international agreements aimed at addressing climate change. However, the growing reliance on AI-based forecasting systems like GenCast may also spark debates about the need for algorithmic transparency, the handling of sensitive data, and the pertinence of maintaining traditional physics-based methods alongside AI innovations to ensure comprehensive and balanced weather forecasting capabilities.
In conclusion, the advancement of GenCast as a superior AI-driven weather forecasting tool heralds considerable opportunities and challenges across different societal dimensions. As countries and industries adapt to these technologies, the ongoing discourse around ethical AI application, data governance, and collaborative international frameworks will be vital in maximizing GenCast's potential benefits while mitigating its risks. This continuous dialogue will ensure that AI advancements in weather forecasting contribute constructively to global efforts to cope with and adapt to the pressing realities of climate change.
Learn to use AI like a Pro
Get the latest AI workflows to boost your productivity and business performance, delivered weekly by expert consultants. Enjoy step-by-step guides, weekly Q&A sessions, and full access to our AI workflow archive.
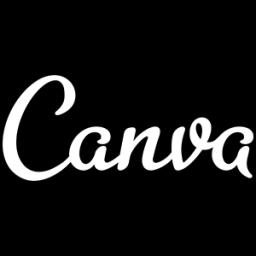
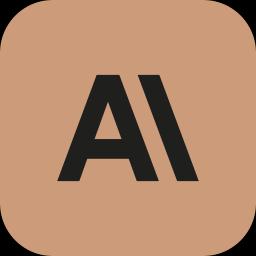
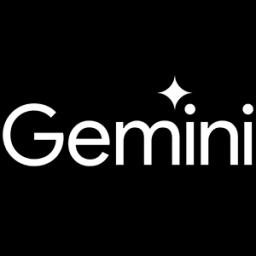
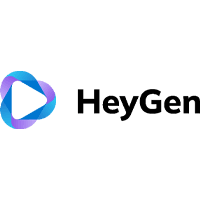
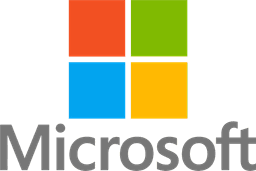
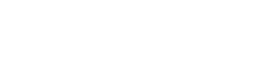
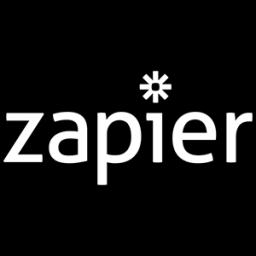
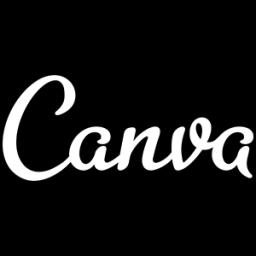
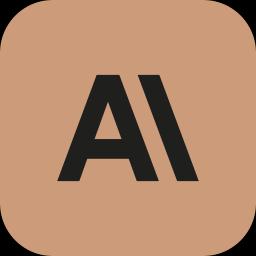
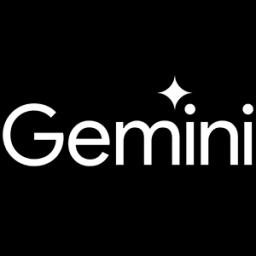
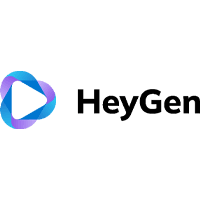
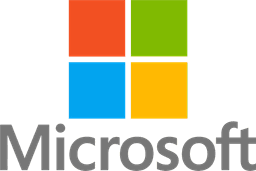
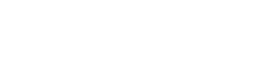
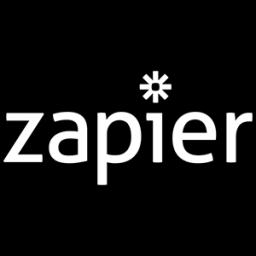