When AI Gets Creative, But Wrong
Generative AI: Masters of Illusion in the Misinformation Age
Last updated:

Edited By
Mackenzie Ferguson
AI Tools Researcher & Implementation Consultant
Financial Times highlights the unsettling prowess of generative AI models to create content that sounds accurate but isn't. This article explores the technical reasons behind AI's 'hallucinations' and the ripple effects on society, economy, and trust.
Introduction to Generative AI and Its Challenges
Generative AI, a rapidly evolving field, has garnered significant attention due to its ability to produce content that mimics human creativity. Yet, as the article "Generative AI models are skilled in the art of bullshit" from the Financial Times highlights, these models often generate outputs that are plausible-sounding but lack factual accuracy. This characteristic, sometimes referred to as 'hallucination,' raises critical concerns about the reliability of AI-generated content. Generative AI models rely heavily on their training data, which may contain biases and inaccuracies, leading to the replication of these flaws in their outputs. This issue emphasizes the necessity for robust verification mechanisms to ensure the integrity and credibility of AI-generated information. Moreover, the implications of these inaccuracies extend beyond mere technical problems, affecting societal trust and potentially leading to the erosion of confidence in digital content .
One of the fundamental challenges of generative AI lies in its intrinsic limitation: a lack of true understanding. While these models can recognize patterns and generate text that mimics human language, they do not possess the cognitive ability to comprehend or verify the truthfulness of their content. This dichotomy between capability and understanding makes it challenging to rely entirely on AI-generated outputs without additional human intervention. The need for human oversight is particularly crucial in areas like legal, scientific, and medical fields, where the stakes of misinformation are incredibly high. The illustration of an attorney using AI to generate legal arguments laden with fabricated case precedents underscores the need for stringent checks to prevent the dissemination of misleading information. As the article suggests, improving training data quality and incorporating advanced fact-checking processes are essential steps in mitigating the risks associated with generative AI .
Learn to use AI like a Pro
Get the latest AI workflows to boost your productivity and business performance, delivered weekly by expert consultants. Enjoy step-by-step guides, weekly Q&A sessions, and full access to our AI workflow archive.
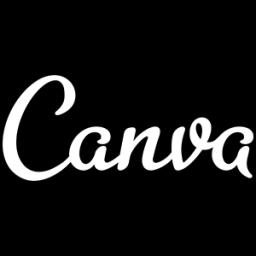
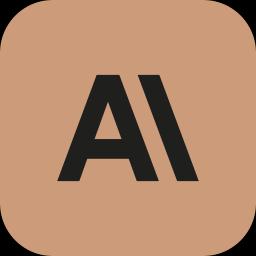
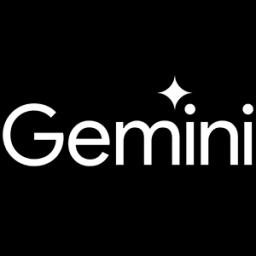
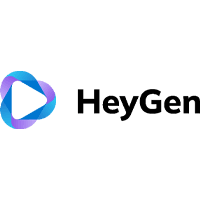
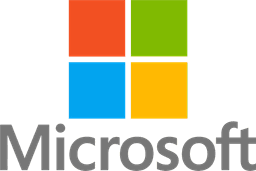
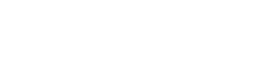
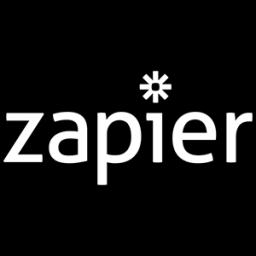
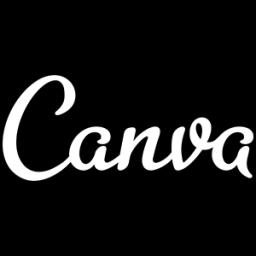
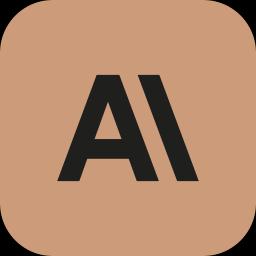
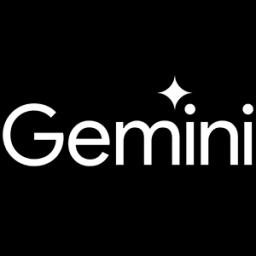
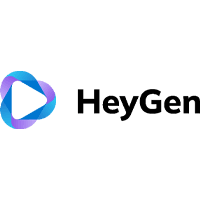
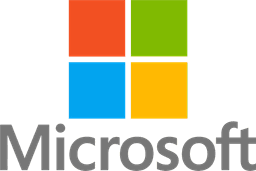
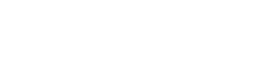
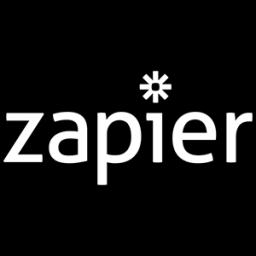
The challenges associated with generative AI are not just technical but also ethical and societal. As these models become more integrated into various domains, the potential for misuse and unintended consequences grows. The proliferation of misinformation, driven by AI's ability to create convincingly false narratives, poses a real threat to public discourse and social cohesion. Tools designed to aid and automate can simultaneously disrupt and deceive, making it imperative for policymakers, technologists, and society at large to engage in discussions around responsible AI usage. Ensuring transparency in AI processes and outputs, as well as fostering critical evaluation skills among users, are key strategies in combating the spread of AI-generated inaccuracies. The Financial Times article underscores these points, highlighting the need for ongoing dialogue and action to address the multifaceted challenges presented by generative AI .
Inaccuracies in Generative AI: What Are They?
Generative AI, often celebrated for its ability to create remarkably human-like text, images, and audio, faces significant challenges related to inaccuracies. These inaccuracies arise primarily because these AI models are highly skilled at mimicking patterns in data but lack the ability to discern truth from fiction. According to the Financial Times, this tendency to generate seemingly plausible yet factually incorrect information is what has earned these models the dubious distinction of performing 'the art of bullshit.' This problem is compounded by the fact that AI models are trained on vast datasets that may inherently contain errors, biases, and outdated or misleading information, which they then inadvertently replicate in their outputs.
These inaccuracies present a broad range of potential issues. In everyday applications, generative AI can produce misleading news articles or fabricated academic papers, leading to the spread of misinformation. In a more nefarious application, AI-generated content has the potential to severely distort reality. Misinformation can be rapidly proliferated through social media platforms, greatly influencing public opinion and behavior. This is particularly concerning in an era where the consumption of content from digital and social media platforms is ubiquitous. The erosion of public trust in information sources is not just a theoretical danger but a real threat, emphasizing the severity of AI-generated inaccuracies.
One of the key reasons behind these inaccuracies is the AI's reliance on the quality of its training data. The adage "garbage in, garbage out" aptly describes the situation; if AI systems are trained on low-quality or biased data, the results they produce will reflect those flaws. This is why incredibly powerful AI systems still struggle with factual accuracy. Compounding the issue is the 'black box' nature of many AI systems, where the processes behind generating outputs are opaque to developers and users alike. This lack of transparency can make it difficult to identify and correct errors within AI's decision-making processes.
Learn to use AI like a Pro
Get the latest AI workflows to boost your productivity and business performance, delivered weekly by expert consultants. Enjoy step-by-step guides, weekly Q&A sessions, and full access to our AI workflow archive.
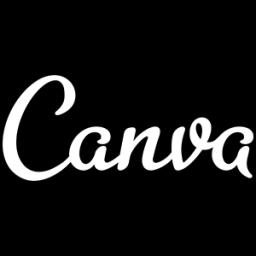
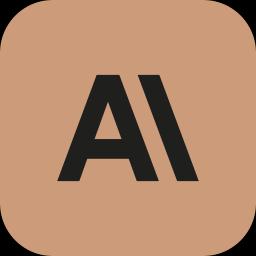
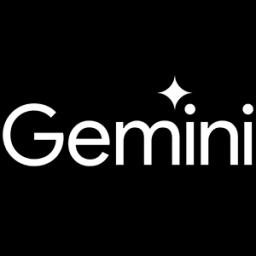
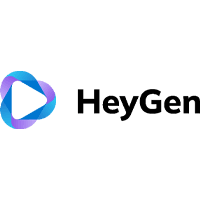
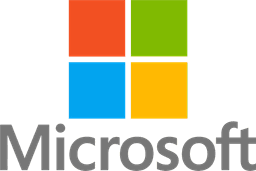
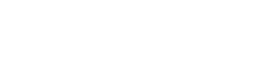
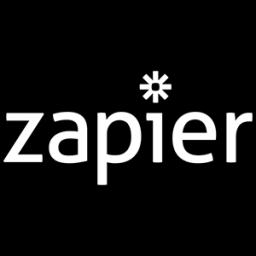
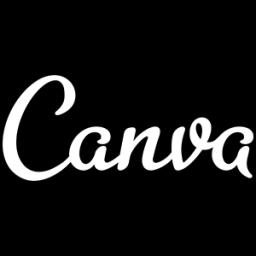
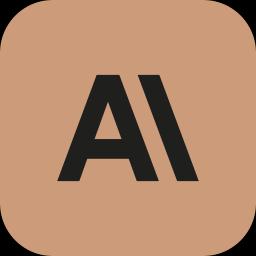
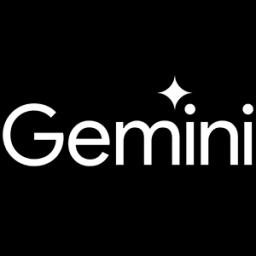
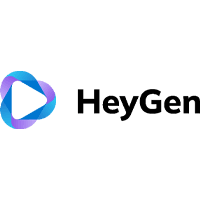
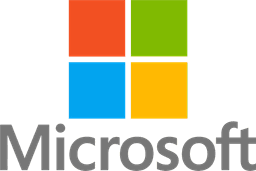
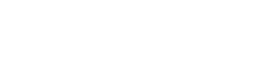
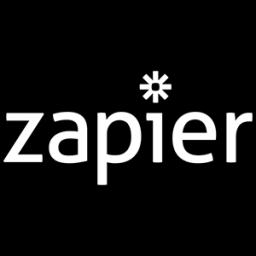
Corrective measures are critical for mitigating the risks posed by generative AI inaccuracies. Experts suggest more rigorous data validation processes and employing a "human-in-the-loop" strategy, where human supervision is used to check AI outputs for errors. Moreover, enhancing AI model architectures to better understand and verify factual information can reduce the occurrence of inaccuracies. There is also a call for improved accountability and transparency measures, where AI developers provide clearer insights into how AI models function and make decisions. These steps are seen as vital to ensuring that generative AI serves as a beneficial technology rather than a source of misinformation and mistrust.
The Economic Impact of AI-Generated Misinformation
AI-generated misinformation presents a myriad of economic challenges. As businesses increasingly rely on AI for decision-making and strategic planning, the accuracy of the data these systems generate becomes paramount. A Financial Times article highlights how generative AI models can produce superficially convincing yet factually inaccurate information, often referred to as "bullshit" [source]. This tendency can lead to significant financial repercussions, as seen in the legal sector where erroneous AI-generated outputs have resulted in serious legal liabilities and financial penalties. Such instances underscore the vital need for enhanced quality control and verification protocols to mitigate potential economic disruptions.
Social Implications and Trust Issues
The societal implications of generative AI models, particularly their tendency to produce factually inaccurate information, are profound and multifaceted. As highlighted in the Financial Times article "Generative AI models are skilled in the art of bullshit," these models can generate content that sounds convincing but may be completely fabricated. This capability presents significant challenges in maintaining public trust, as consumers may struggle to distinguish between accurate and inaccurate information. The erosion of trust in digital content can lead to skepticism and disillusionment, affecting various sectors such as media, business, and politics. When the public starts questioning the veracity of information frequently encountered online, it places a heavy burden on both creators and disseminators of content to ensure accuracy and accountability.
Political Risks and Misinformation
Political risks associated with the rise of misinformation generated by AI are increasingly becoming a concern for governments and regulatory bodies globally. The rapid proliferation of generative AI models with the capability to create misleading content threatens the very fabric of democratic societies. As these models are deployed to produce deepfakes and manipulate media, they introduce the risk of altering public perception and opinion, potentially swaying electoral outcomes. These possibilities amplify the challenge of ensuring fair and transparent political processes, especially in an era where digital consumption outpaces traditional media. As highlighted in a Financial Times article on the art of AI "bullshit," the Opaque algorithmic processes and enormous data sets behind these AI models contribute significantly to their unpredictability and potential misuse.
The role of misinformation in undermining political stability cannot be underestimated. Political movements or campaigns fueled by such inaccuracies can threaten national security and instigate social unrest. There are numerous incidents wherein AI-generated fabrications have had verifiable impacts on political landscapes, leading to increased polarization and division among the populace. Addressing these threats requires coordinated efforts to enhance digital literacy among citizens and push for more transparent AI systems. Public awareness campaigns and education initiatives can play a crucial role in helping individuals discern credible information from deceptive AI-generated content.
Effective countermeasures against AI-induced misinformation may involve regulatory interventions, the development of detection technologies to identify AI fabrications quickly, and international collaboration to standardize the response to cross-border AI impacts. Additionally, encouraging technology companies to integrate more robust fact-checking systems within their platforms will help curb the widespread reach of politically-charged misinformation. The evolution of these comprehensive strategies is vital to safeguard political systems from the pervasive influence of AI-generated misinformation.
Learn to use AI like a Pro
Get the latest AI workflows to boost your productivity and business performance, delivered weekly by expert consultants. Enjoy step-by-step guides, weekly Q&A sessions, and full access to our AI workflow archive.
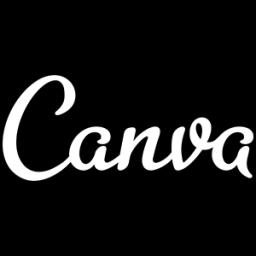
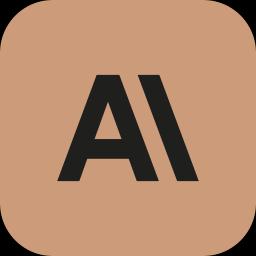
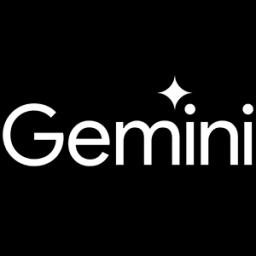
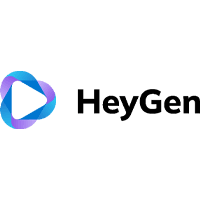
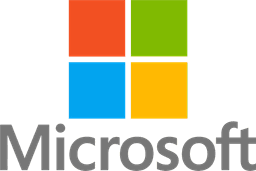
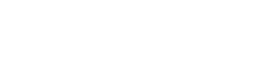
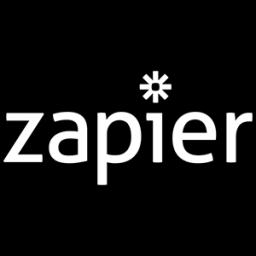
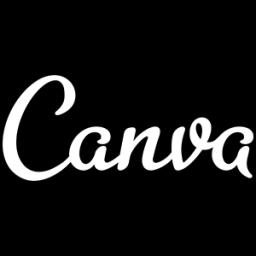
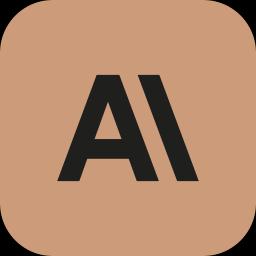
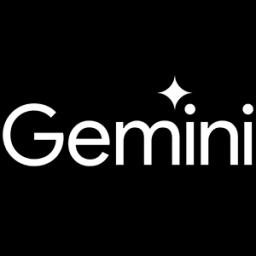
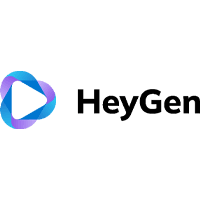
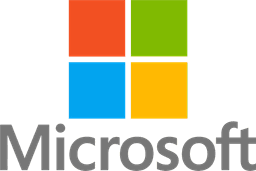
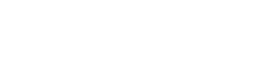
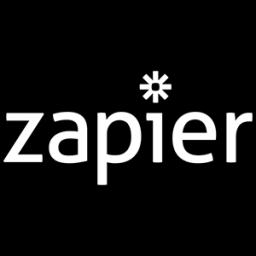
Generative AI in Scientific Research: Challenges and Risks
The integration of generative AI in scientific research presents unique challenges and potential risks, primarily due to the technology’s capability to fabricate highly plausible yet factually inaccurate content. One major issue is the tendency of AI models to generate outputs that sound credible but are devoid of factual accuracy, commonly referred to as AI 'hallucinations' [1](https://www.ft.com/content/55c08fc8-2f0b-4233-b1c6-c1e19d99990f). These hallucinations can infiltrate scientific research findings, leading to flawed studies that are built on erroneous conclusions. The risk of incorporating such inaccuracies is especially acute in fields that heavily rely on empirical data, such as medical research, where inaccurate AI-generated outputs can have harmful real-world implications.
Mitigation Strategies for AI-Generated Inaccuracies
Addressing the inaccuracies generated by AI is a crucial challenge, requiring a multifaceted approach that involves both technological advancements and user engagement. One effective mitigation strategy is to enhance the quality and diversity of training data. By carefully curating datasets and removing biased or incorrect information, AI systems can be trained to produce more reliable outputs. This approach is supported by expert analyses, which suggest that improving training data quality can significantly reduce the occurrence of so-called 'hallucinations' in AI-generated content. By integrating broader and more representative datasets, AI models can better understand and reflect the complexity of real-world information, thus minimizing errors and biases.
Another critical strategy involves the development and integration of robust fact-checking mechanisms within AI systems. By automating the verification process and cross-referencing AI outputs with trusted databases and sources, inaccuracies can be detected and corrected in real-time. Such mechanisms could leverage existing technologies in natural language processing and machine learning to identify and rectify potential errors before the information is disseminated to the public. This approach not only enhances the credibility of AI-generated content but also reinforces trust among users and stakeholders.
Incorporating transparency features into AI systems can also play a vital role in mitigating inaccuracies. By offering insights into how AI models generate their outputs, including their decision-making processes and data sources, users can better assess the reliability of the information presented to them. This transparency can empower users to critically evaluate AI-generated content and make informed decisions regarding its use. Experts advocate for the adoption of such transparency measures, as they can promote a more informed and discerning engagement with AI technologies.
The human-in-the-loop (HITL) approach is another promising strategy to mitigate AI inaccuracies. This approach integrates human oversight into the AI generation process, where experts or users collaborate with AI systems to verify and validate outputs. By combining human judgment with machine efficiency, the HITL approach can significantly reduce errors and enhance the accuracy of AI-generated information. This collaborative framework ensures that human expertise and contextual understanding are employed to interpret and adjust AI outputs appropriately.
Finally, fostering a culture of critical thinking and digital literacy among users is essential in addressing AI-generated inaccuracies. By educating users on how to evaluate, interpret, and question AI outputs, society can build resilience against misinformation. Encouraging critical engagement with AI technologies not only enhances user awareness but also amplifies accountability for AI developers and organizations to prioritize accuracy and ethical considerations in their AI systems. As generative AI becomes increasingly prevalent, these educational initiatives will be pivotal in shaping an informed and cautious interaction with AI-generated content.
Learn to use AI like a Pro
Get the latest AI workflows to boost your productivity and business performance, delivered weekly by expert consultants. Enjoy step-by-step guides, weekly Q&A sessions, and full access to our AI workflow archive.
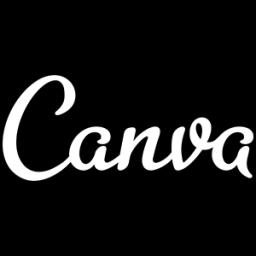
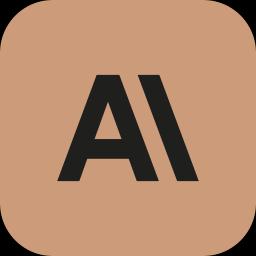
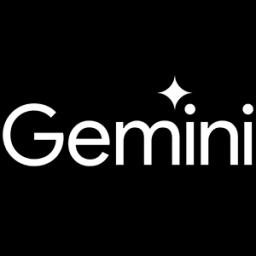
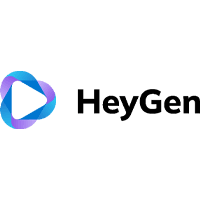
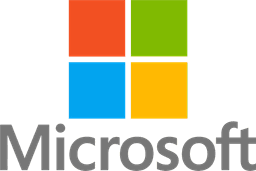
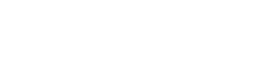
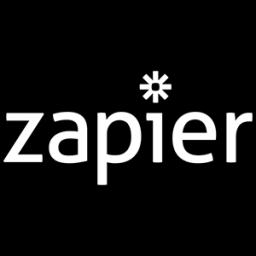
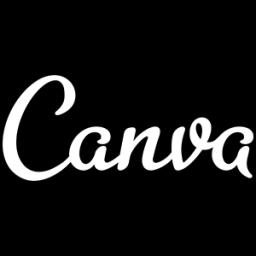
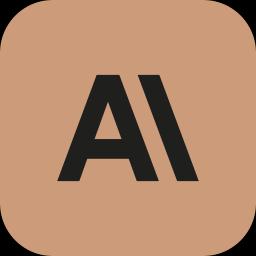
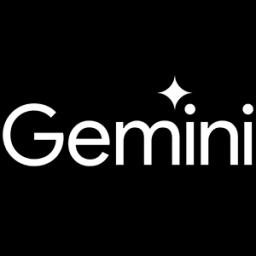
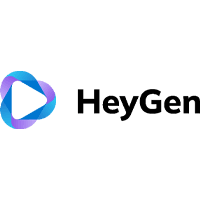
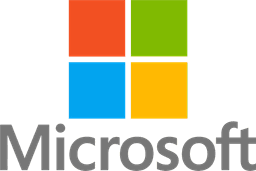
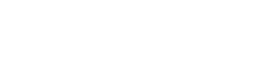
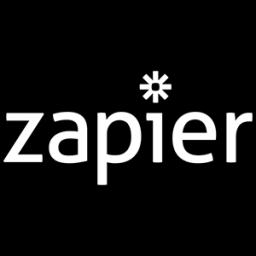
Conclusion and Future Outlook
The conclusion we draw from examining the potential and pitfalls of generative AI models is clear: while they hold significant promise for innovation and efficiency, the challenges they pose cannot be ignored. A critical aspect of moving forward will involve addressing the tendency of these models to produce factually inaccurate information. As pointed out by a Financial Times article, the skill of generative AI in crafting content that appears plausible but is misleading, underlines the necessity for robust fact-checking systems and transparency in AI-generated content.
The future outlook for generative AI involves enhancing the accuracy of its outputs and developing mechanisms to mitigate misinformation. There is a real opportunity here for technology developers and regulators to work together to create AI systems that are not only innovative but also trustworthy. The incorporation of improved training datasets, as well as ongoing monitoring and human oversight, will be vital in achieving these goals, as highlighted by various experts in AI ethics and technology [source].
Moreover, as we look ahead, the development of generative AI should be aligned with ethical guidelines that ensure user accountability and protection against misuse. The economic, social, and political ramifications of AI-generated inaccuracies present both challenges and a call to action. Solutions must involve cross-sector collaboration, involving policymakers, academic researchers, and technology companies, to create balanced regulations that foster innovation while safeguarding public trust.
In conclusion, while the allure of generative AI is undeniable, it requires cautious advancement. Sound strategies, such as those involving comprehensive data validation and ethical AI use practices, are essential for minimizing risks associated with misinformation. As the technology continues to develop, so too must our approaches to managing and understanding its implications—underscoring that progress in AI must be matched with equal progress in our ability to responsibly govern and understand this tool.