AI Weather Predictions Just Got a Major Upgrade!
Google DeepMind Reveals WeatherNext: AI's Forecasting Revolution Begins!
Last updated:

Edited By
Mackenzie Ferguson
AI Tools Researcher & Implementation Consultant
Google DeepMind, in collaboration with Google Research, has launched WeatherNext, an innovative AI-driven weather forecasting model that promises greater accuracy and efficiency than traditional forecasting methods. Key features include single deterministic forecasts and ensemble scenarios, available through Google Cloud. Select components are open-sourced for widespread access and use in disaster preparedness, grid reliability, and more.
Introduction
In a new era of weather forecasting, WeatherNext by Google DeepMind and Google Research represents a monumental leap forward. This innovative system, available through the synergy of Google Cloud platforms like BigQuery and Earth Engine, introduces AI-based models that redefine accuracy and efficiency in weather prediction. These advancements are not just incremental; they challenge the very foundations of traditional physics-based forecasting models, offering faster and more reliable forecasts to meet the diverse needs of users globally [1](https://deepmind.google/technologies/weathernext/).
WeatherNext's capabilities are impressive: its Graph model provides deterministic forecasts up to 10 days with updates every six hours, while the Gen model supports ensemble forecasting across 50 scenarios, extending up to 15 days [1](https://deepmind.google/technologies/weathernext/). These enhanced features make WeatherNext an invaluable tool for applications in fields such as disaster preparedness, electric grid management, and agricultural planning, ensuring that critical decisions are informed by the most accurate data available [1](https://deepmind.google/technologies/weathernext/).
Learn to use AI like a Pro
Get the latest AI workflows to boost your productivity and business performance, delivered weekly by expert consultants. Enjoy step-by-step guides, weekly Q&A sessions, and full access to our AI workflow archive.
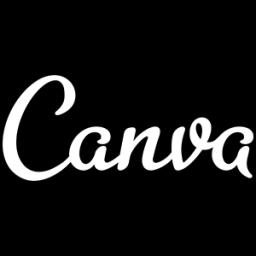
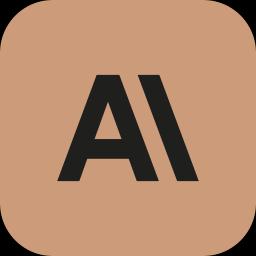
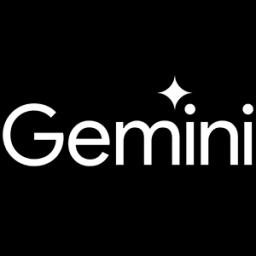
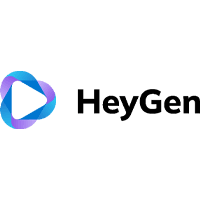
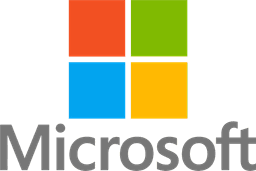
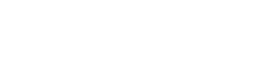
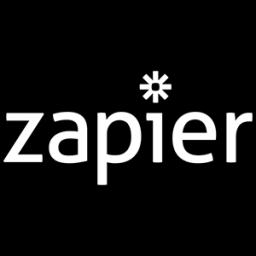
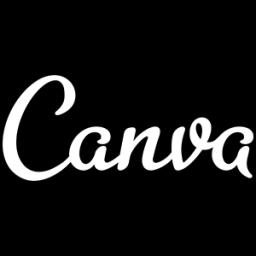
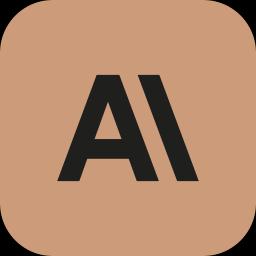
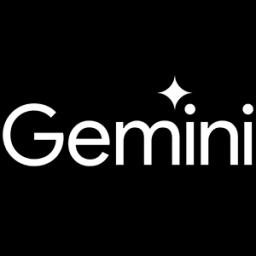
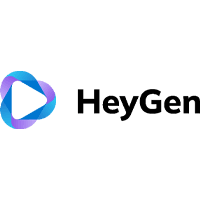
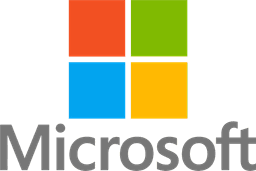
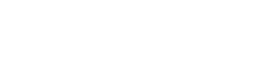
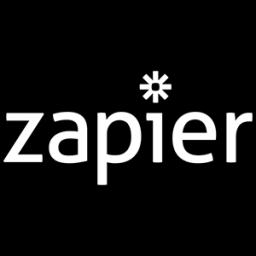
This transformative technology is set to impact not just the meteorological community, but also industries and governments that depend heavily on accurate weather information. The integration of select WeatherNext components into GitHub for open-source collaboration highlights Google’s commitment to community-driven development while ensuring that the core models remain robust and continually improved [1](https://deepmind.google/technologies/weathernext/).
As the world grapples with the implications of climate change, the need for accurate and reliable weather forecasting becomes even more urgent. WeatherNext's sophisticated AI models capitalize on extensive data processing capabilities to deliver quicker and more precise forecasts, a skill increasingly critical in navigating the unpredictable weather changes of our time [1](https://deepmind.google/technologies/weathernext/).
Public and expert reactions have been overwhelmingly positive, with WeatherNext being praised for technological advances that promise to enhance public safety and economic stability. However, it also sparks discussions about accessibility and the potential monopolization of weather data by large tech companies, prompting calls for open access and collaborative models to ensure equitable use of such transformative technology [1](https://deepmind.google/technologies/weathernext/).
Key Features of WeatherNext
WeatherNext, a pioneering development by Google DeepMind in collaboration with Google Research, embodies a sophisticated leap in weather forecasting technology. At the heart of WeatherNext are two distinct AI-based models: the WeatherNext Graph and WeatherNext Gen. The former offers deterministic forecasts with a robust 10-day lead time and updates every six hours, ensuring users receive timely, accurate single-scenario forecasts. Meanwhile, WeatherNext Gen expands on this by producing ensemble forecasts, delivering up to 50 different future weather scenarios with a commendable 15-day lead time, updating twice a day. This blend of deterministic and ensemble forecasting arms policymakers, businesses, and the general public with comprehensive, adaptable insights crucial for planning and decision-making. Such capabilities mark a significant enhancement over traditional forecasting methods, reinforcing WeatherNext's pivotal role in modern meteorological practices.
Learn to use AI like a Pro
Get the latest AI workflows to boost your productivity and business performance, delivered weekly by expert consultants. Enjoy step-by-step guides, weekly Q&A sessions, and full access to our AI workflow archive.
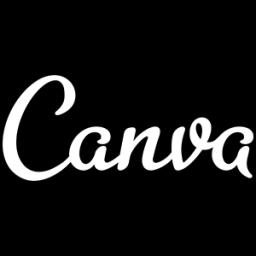
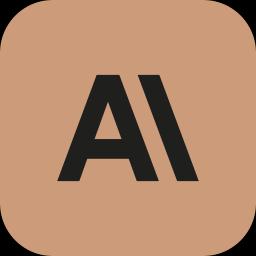
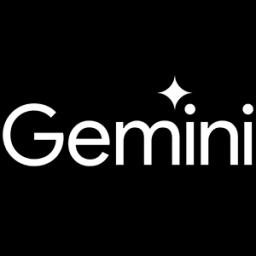
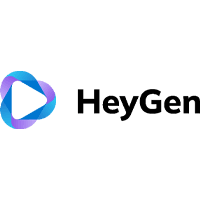
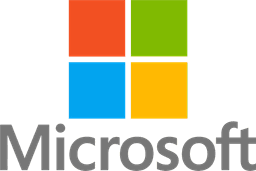
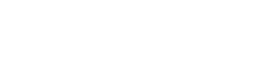
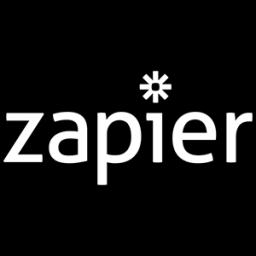
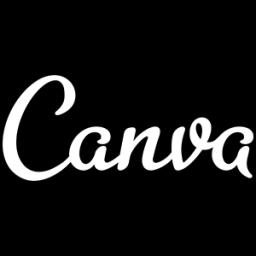
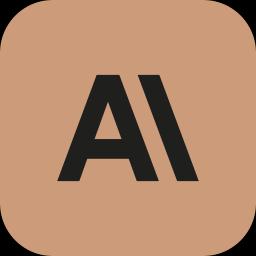
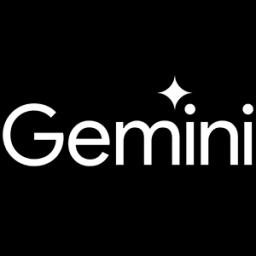
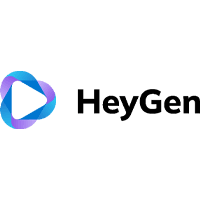
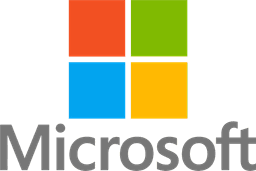
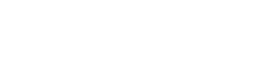
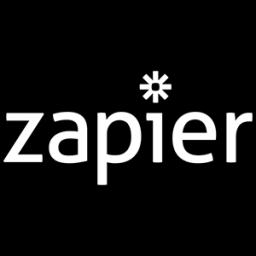
Accessible through leading Google Cloud services, namely Google Cloud BigQuery and Earth Engine, WeatherNext leverages the global reach of cloud computing to offer its state-of-the-art weather prediction capabilities. A notable aspect of this initiative is its commitment to transparency and collaboration, as evidenced by certain components of WeatherNext being open-sourced on GitHub. This open-source strategy invites developers and researchers worldwide to contribute to and refine the system, fostering a collaborative approach to technological advancements in meteorology. Such access empowers a wide range of users to harness these forecasts, potentially transforming areas such as disaster management and agricultural planning, thereby contributing significantly to global food security and safety initiatives.
One of the primary revolutionary aspects of WeatherNext is how it processes data. Unlike traditional physics-based models that rely heavily on complex equations and massive computational resources, WeatherNext uses advanced AI algorithms to quickly process vast amounts of meteorological data. This enhances not only the speed of delivery but also the reliability of forecasts produced, setting a new benchmark in weather prediction technology. Furthermore, its introduction comes at a pivotal moment, as the world grapples with increasingly frequent and severe weather events due to climate change. With its ability to provide more timely and accurate predictions, WeatherNext holds potential to play a crucial role in disaster preparedness and mitigation strategies. As noted on Google's platform, the model shows notable promise in improving the precision of forecasting extreme weather scenarios, a critical component in safeguarding communities against climate-related disruptions.
Despite its groundbreaking capabilities, WeatherNext does face some challenges. Experts such as Dr. Claire Monteleoni have highlighted limitations inherent in the model's reliance on historical data for training. This reliance could potentially impact the model's accuracy as climate change continues to alter established weather patterns. However, the system's developers are addressing these limitations by continually updating the data inputs and algorithm refinements, aiming to minimize prediction inaccuracies. Moreover, the partial open-source nature of WeatherNext allows for external expertise to contribute to overcoming these hurdles, ensuring the model evolves in response to environmental changes. This dynamic adaptability is critical to maintaining forecast reliability as climatic conditions continue to evolve rapidly, thereby ensuring that WeatherNext remains at the forefront of weather forecasting technology.
WeatherNext Graph vs Gen: Differences and Uses
WeatherNext, a groundbreaking AI-driven weather forecasting tool, is an impressive innovation by Google DeepMind and Google Research. It aims to transform the way weather predictions are made, enhancing both the accuracy and efficiency of forecasts . WeatherNext is available in two models: WeatherNext Graph and WeatherNext Gen, each designed to cater to different forecasting needs and scenarios.
The key difference between WeatherNext Graph and WeatherNext Gen lies in their approach and output. WeatherNext Graph prioritizes precision, offering deterministic forecasts with a 10-day lead time and updating every six hours. This model is ideal for scenarios requiring highly precise and reliable short-term forecasts, such as planning outdoor events or optimizing short-term logistics . It provides single forecasts, which can be critical for users needing clarity and direct action plans.
WeatherNext Gen, in contrast, is built to handle uncertainty more effectively. It delivers ensemble forecasts with up to 50 different scenarios over a 15-day lead time and a 12-hour update cycle . This model is exceptionally useful for long-term planning and risk assessment, allowing users to prepare for a range of potential weather outcomes. Such capabilities are particularly valuable in fields like agriculture, disaster management, and strategic business planning, where understanding a spectrum of possibilities can significantly impact decision-making.
Learn to use AI like a Pro
Get the latest AI workflows to boost your productivity and business performance, delivered weekly by expert consultants. Enjoy step-by-step guides, weekly Q&A sessions, and full access to our AI workflow archive.
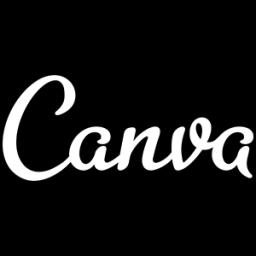
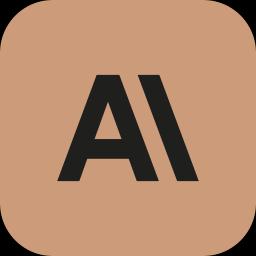
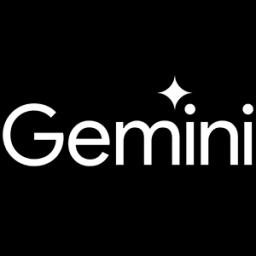
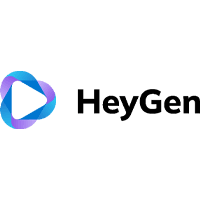
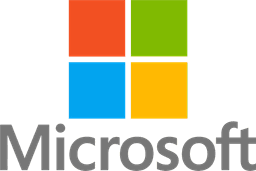
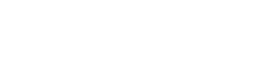
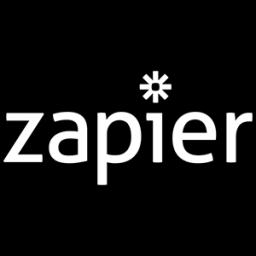
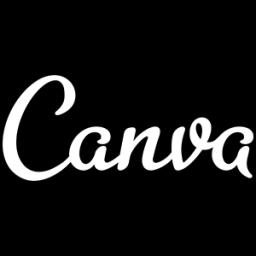
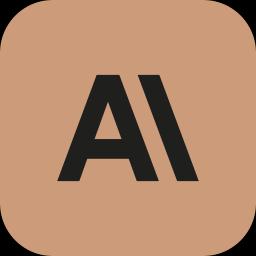
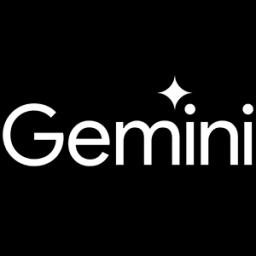
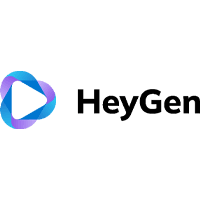
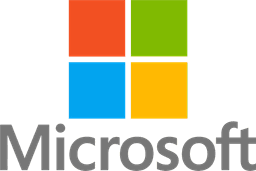
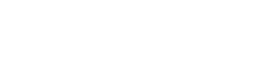
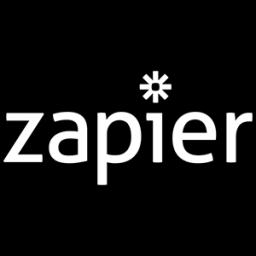
Both models of WeatherNext are made accessible through platforms such as Google Cloud's BigQuery and Earth Engine, helping developers and researchers integrate advanced forecasting capabilities into their projects . Furthermore, the partial open-sourcing on platforms like GitHub ensures that a variety of collaborators can participate in expanding and refining these tools, keeping them adaptable and evolutionary.
While WeatherNext Graph and Gen provide significant technological advancements in weather prediction, their development raises essential considerations about data usage and prediction accuracy, especially as climate change introduces atypical weather patterns not accounted for in historical data . As noted by experts, ongoing adaptation and updates to these models will be crucial in maintaining their reliability and utility.
Accessing WeatherNext: Tools for Developers and Researchers
WeatherNext, introduced by Google DeepMind and Google Research, is revolutionizing the landscape of weather forecasting with its innovative AI models. These models are designed to significantly enhance the accuracy and efficiency of predictions compared to traditional methods, making them invaluable tools for both developers and researchers. By leveraging advanced algorithms, WeatherNext processes vast amounts of data more efficiently, delivering faster and more reliable predictions, a breakthrough highlighted in numerous discussions on platforms like [Reddit](https://www.reddit.com/r/google/comments/1fraogo/how_is_this_possible_google_weather_forecast/).
The WeatherNext suite includes two main model types: WeatherNext Graph and WeatherNext Gen. WeatherNext Graph focuses on delivering precise, deterministic forecasts with a ten-day lead time and a six-hour resolution window, ideal for applications needing high accuracy in a shorter timeframe. In contrast, WeatherNext Gen is tailored for situations requiring assessment of uncertainty; it generates ensemble forecasts of up to 50 different scenarios over a 15-day lead time with a 12-hour resolution. This capability is particularly useful for predicting extreme weather events, as noted by Google's Dr. Demis Hassabis on [LinkedIn](https://www.linkedin.com/posts/demishassabis_accurate-weather-prediction-is-one-of-the-activity-7291135811582844928-DvnU).
Access to WeatherNext is designed to be straightforward for developers and researchers, thanks to its integration with Google Cloud services. Users can utilize BigQuery and Earth Engine to tap into the system's capabilities, making it a versatile tool for a wide range of applications from electric grid reliability to food security management. Additionally, select components of WeatherNext are open-sourced, available on platforms like [GitHub](https://deepmind.google/technologies/weathernext/), providing developers the opportunity to contribute to and enhance its functionalities.
The practical applications of WeatherNext extend far beyond straightforward weather predictions. For instance, its ability to improve disaster preparedness and response can lead to significant economic and social benefits, potentially reducing losses from extreme weather events. Furthermore, enhanced forecasting accuracy plays a crucial role in optimizing electric grid management and ensuring food security, as highlighted by public reactions seen on [Product Hunt](https://www.producthunt.com/posts/weathernext-by-google?ref=upstract.com).
Learn to use AI like a Pro
Get the latest AI workflows to boost your productivity and business performance, delivered weekly by expert consultants. Enjoy step-by-step guides, weekly Q&A sessions, and full access to our AI workflow archive.
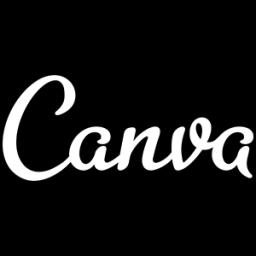
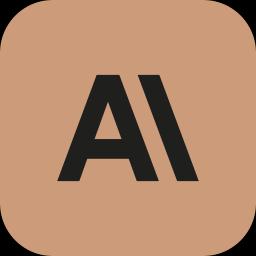
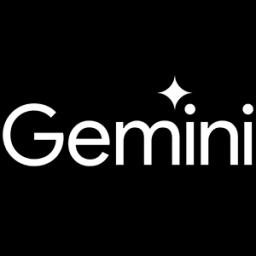
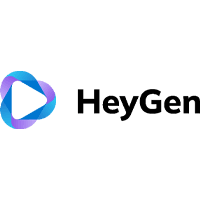
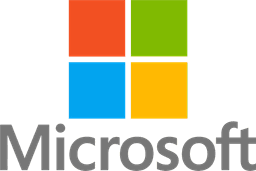
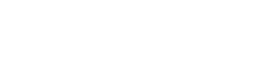
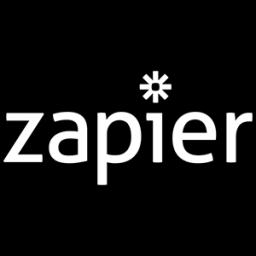
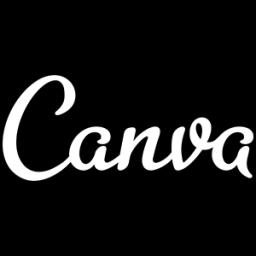
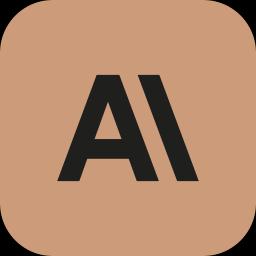
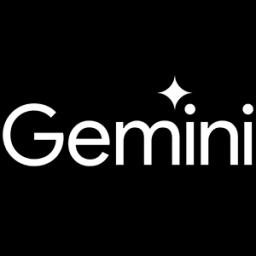
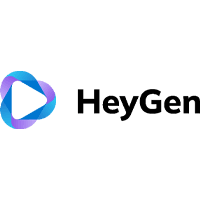
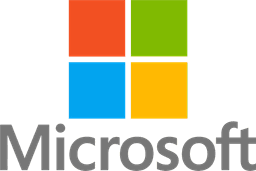
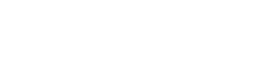
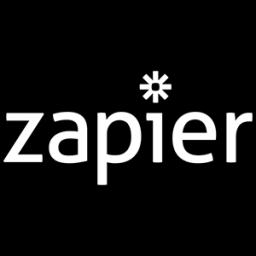
Despite the remarkable advancements brought by WeatherNext, there are ongoing discussions about its implications and potential limitations. Experts, such as Dr. Peter Dueben, point out that while the system shows impressive accuracy, its reliance on historical training data might pose challenges as climate change alters weather patterns far beyond the models' baseline training data. These insights suggest a need for adaptive strategies in AI model training to maintain WeatherNext's accuracy as highlighted by [MIT Technology Review](https://www.technologyreview.com/2024/12/04/1107892/google-deepminds-new-ai-model-is-the-best-yet-at-weather-forecasting/).
Forecast Accuracy: A New Benchmark
The unveiling of WeatherNext by Google DeepMind sets a new benchmark in the realm of weather forecasting, showcasing significant advancements in accuracy and efficiency over traditional models. With the ability to generate both deterministic and ensemble forecasts, WeatherNext offers unprecedented insights into weather patterns. This technological leap enables a 10-day forecasting capability with a resolution of six hours using WeatherNext Graph, while WeatherNext Gen extends this to 15 days with up to 50 different scenarios. These robust features are poised to transform how we predict and respond to weather events, providing organizations, government bodies, and individuals with the tools necessary to enhance disaster preparedness, improve electric grid reliability, and bolster food security. As part of its rollout, Google DeepMind has made select components available through open-source platforms like GitHub, furthering innovation in the field.
Unlike conventional weather forecasting systems that heavily rely on physics-based models to interpret atmospheric conditions, WeatherNext leverages the power of artificial intelligence to process vast amounts of historical data and generate high-precision forecasts. This methodology allows for faster computations, which are crucial during evolving weather situations. Such efficiency is not merely academic but has tangible benefits in real-world applications – from preemptively mitigating the impacts of hurricanes to guiding agricultural decisions to ensure optimal crop yield. The integration into Google's Cloud services like BigQuery and Earth Engine underscores WeatherNext's accessibility to developers and researchers aiming to leverage these insights into practical solutions for complex climate challenges.
The strategic importance of WeatherNext cannot be overstated, particularly in the context of global climate change. As traditional models struggle with the rapid pace of changing weather patterns, AI-driven models like WeatherNext offer a more adaptable and accurate tool for forecasters. However, experts like Dr. Peter Dueben caution about the model's dependence on historical data, which may become less indicative as climatic anomalies become the norm. Nevertheless, WeatherNext's innovative approach marks a pivotal shift in the forecasting landscape, enhancing not just our understanding but our proactive capabilities in addressing the climatic challenges of today and tomorrow.
Public reaction to WeatherNext has largely been positive, with many viewing it as a transformative leap forward in meteorological science. Enthusiasts on platforms like Product Hunt have eagerly discussed its potential, though some have expressed concerns regarding hyper-local forecasting capabilities and the integration of climate change data into its predictions. On Reddit, debates continue around the accessibility of such advanced tools to the general public and the possibility of such technologies being monopolized by tech giants. This dialogue reflects a broader conversation about the balance between innovation and equitable access, highlighting the need for continued dialogue about the role of AI in public sector utilities.
The future implications of technologies like WeatherNext extend beyond scientific curiosity, impacting economic and social structures worldwide. As AI-powered weather forecasting becomes more prevalent, it offers potential reductions in the economic losses associated with extreme weather while also lowering energy consumption through optimized grid management. In agriculture, more accurate forecasts can significantly enhance food production, addressing global food security challenges. However, this reliance on AI may lead to concentrations of power within tech industries, prompting calls for fair access to such critical infrastructure and the establishment of collaborative international standards for AI meteorological data sharing and utilization.
Learn to use AI like a Pro
Get the latest AI workflows to boost your productivity and business performance, delivered weekly by expert consultants. Enjoy step-by-step guides, weekly Q&A sessions, and full access to our AI workflow archive.
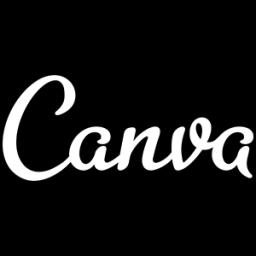
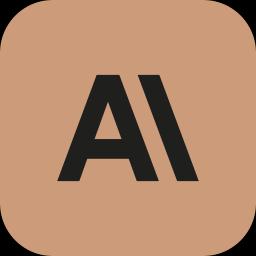
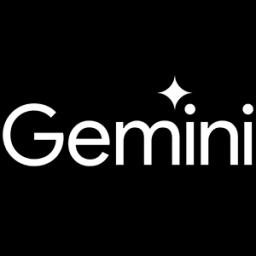
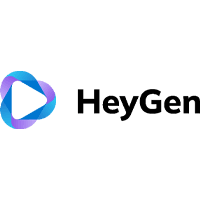
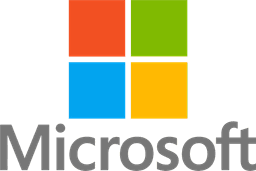
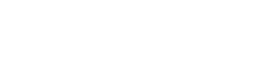
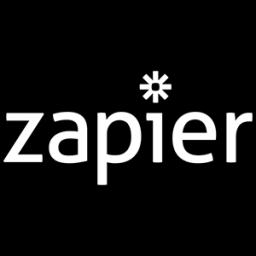
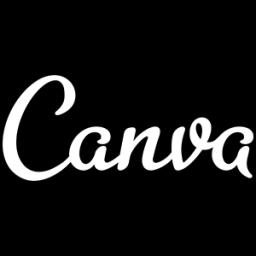
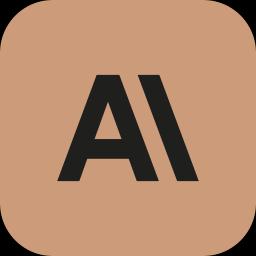
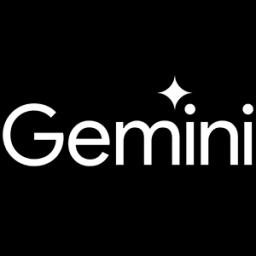
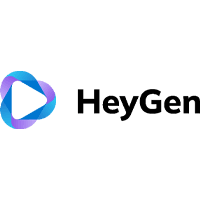
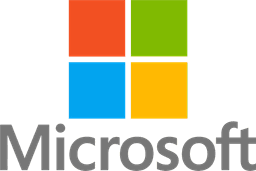
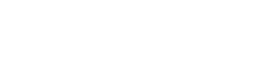
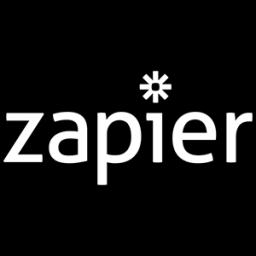
Related Industry Developments
The weather forecasting industry is undergoing significant transformation with the advent of AI technologies. One of the most significant developments is the introduction of WeatherNext by Google DeepMind and Google Research. This groundbreaking model leverages advanced AI techniques to enhance the accuracy and efficiency of weather predictions. WeatherNext's capabilities, such as the WeatherNext Graph and WeatherNext Gen, provide precise forecasts with considerable lead times, making it a valuable tool for sectors that rely on accurate weather data. The model's integration through Google Cloud has made it accessible for developers and researchers, potentially wide-reaching impacts across industries such as agriculture, energy, and public safety [1](https://deepmind.google/technologies/weathernext/).
Parallel to this, other major developments in AI weather forecasting are taking place globally. Microsoft, for instance, has partnered with NOAA to develop AI-driven hurricane tracking systems. This collaboration enhances real-time processing of satellite data, aiming to improve storm prediction accuracy significantly [1](https://www.microsoft.com/press/2024/01/15/microsoft-noaa-partnership). Similarly, the European Space Agency's new MeteoSat Fourth Generation satellite system incorporates AI algorithms to strengthen weather monitoring across Europe and Africa, signifying a shift towards more reliable and advanced weather tracking technologies [2](https://www.esa.int/Applications/Observing_Earth/MeteoSat/2024/01/new-weather-satellite-launch).
China's advancements in the field are also noteworthy, particularly with the introduction of the FengYun-5 weather satellite by the Chinese Meteorological Administration. This AI-enhanced system demonstrated significant improvements in typhoon tracking accuracy during its initial trials, a crucial capability given the increasing intensity of such events due to climate change [3](https://news.cgtn.com/news/2024-01-20/China-launches-FengYun-5-weather-satellite). Moreover, the World Meteorological Organization has initiated a global AI Weather Data Sharing Protocol to standardize international collaboration in AI-powered weather forecasting, which is vital for addressing the dynamic and interconnected nature of global weather systems [4](https://public.wmo.int/en/media/press-release/2024-01-25/global-ai-weather-data-sharing).
Further advancements include Amazon Web Services' Climate Intelligence Platform, which combines machine learning with climate data to anticipate and mitigate weather-related supply chain disruptions. This platform underscores the growing trend of integrating AI into climate risk management and underscores the increasing relevance of AI-driven solutions in addressing complex global challenges [5](https://aws.amazon.com/blogs/industries/2024-01-30/climate-intelligence-platform-launch).
These developments indicate a broader trend of leveraging AI to enhance weather forecasting capabilities. As these technologies mature, they promise to significantly improve the accuracy, reliability, and accessibility of weather forecasts. However, this rapid evolution also raises questions about the equitable distribution of these technologies and the importance of maintaining open access to critical data to ensure that benefits are widespread and inclusive. In this context, international cooperation and the establishment of global standards are crucial for the responsible advancement of AI in weather forecasting [5](https://www.technologyreview.com/2024/12/04/1107892/google-deepminds-new-ai-model-is-the-best-yet-at-weather-forecasting/).
Expert Opinions on WeatherNext's New Models
Experts have voiced varied opinions on the introduction of Google's WeatherNext AI weather forecasting models, reflecting both optimism and caution. Dr. Peter Dueben, a computational scientist at ECMWF, expressed concerns over the model's reliance on historical data, especially in the context of rapidly changing climate patterns. He suggests that "the challenge is that the weather patterns we're seeing now are increasingly different from what these models were trained on," highlighting a potential limitation of the system. Meanwhile, the CEO of Google DeepMind, Dr. Demis Hassabis, underscored the models' transformative potential, stating that WeatherNext represents "a significant step forward in weather prediction accuracy, particularly for extreme weather events that can have devastating impacts on communities." This underscores a crucial benefit, considering the increasing frequency of such events due to climate change. More on this development can be found here.
Learn to use AI like a Pro
Get the latest AI workflows to boost your productivity and business performance, delivered weekly by expert consultants. Enjoy step-by-step guides, weekly Q&A sessions, and full access to our AI workflow archive.
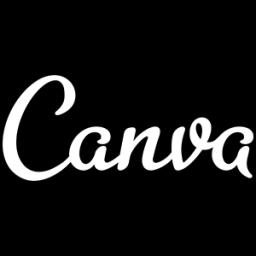
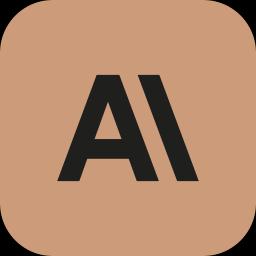
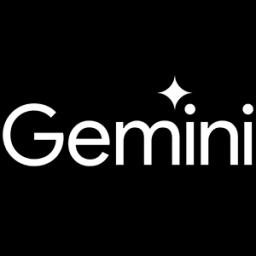
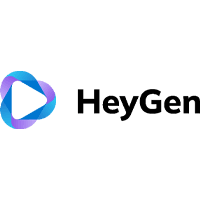
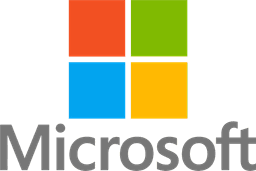
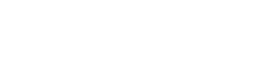
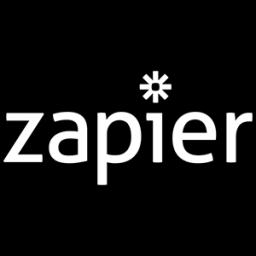
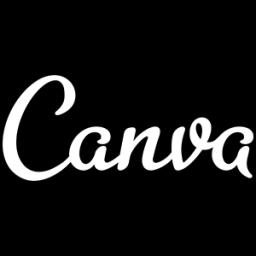
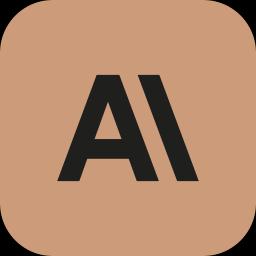
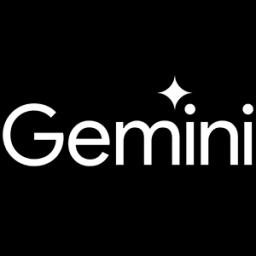
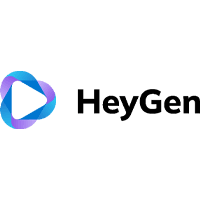
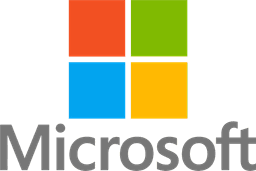
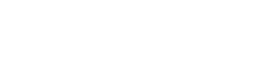
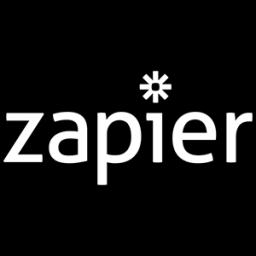
Adding to the discourse, Dr. Claire Monteleoni, a climate informatics expert, points out specific limitations of WeatherNext, noting it "shows particular weakness in predicting conditions in the upper troposphere and cyclone intensities." Despite these challenges, she acknowledges that the models "excel in other areas compared to traditional forecasting methods." This balance of strengths and weaknesses paints a nuanced picture of WeatherNext's capabilities. The models are particularly noted for enhanced accuracy over traditional systems as discussed here.
The broader implications of these expert opinions reveal a narrative of cautious optimism. The enthusiasm about WeatherNext's potential to revolutionize weather forecasting is tangible, yet tempered by the realistic challenges posed by evolving climate conditions. Experts agree that while the present capabilities of AI-powered models like WeatherNext are impressive, ongoing adaptation and integration of real-time climate data will be pivotal in maintaining accuracy and relevance in forecasting. For more insights into the expert perspectives on WeatherNext, visit Google DeepMind's overview.
Public Reactions to WeatherNext
The unveiling of Google's WeatherNext has stirred a diverse array of responses from the public, largely characterized by approval for its innovative capabilities and improved accuracy over traditional methods. On platforms such as Reddit and Product Hunt, users have shown significant interest, particularly highlighting WeatherNext's enhanced forecasting potential. Despite the excitement, some users have expressed reservations concerning the system's hyper-local accuracy and its adaptability to climate change.
Simultaneously, some public skepticism revolves around WeatherNext's accessibility and the potential for privatization of weather technology. While the integration with Google Cloud has been widely welcomed, the limited public access to the full capabilities of the technology is a topic of contention. The initiative to open-source some components on GitHub has received a mixed reception. On Product Hunt, users debate the sufficiency of this transparency for broader public benefit.
Conversations have also engaged with wider implications of Google retaining proprietary controls over core weather forecasting tools. Public forums often reflect on the implications of such advancements remaining within private domains, with discussions focusing on ensuring these technologies evolve as public assets rather than purely commercial ventures. These concerns amplify the debate about how technological advancements like WeatherNext can be leveraged to maximize public good without exacerbating control within powerful tech entities.
Overall, the dialogue surrounding WeatherNext is one of cautious optimism, as users weigh the transformative potential of AI in weather forecasting against the challenges posed by its implementation within existing societal and economic frameworks. This dynamic interplay of enthusiasm and critical analysis underscores a growing public awareness of WeatherNext's journey within the ever-evolving landscape of weather technology.
Learn to use AI like a Pro
Get the latest AI workflows to boost your productivity and business performance, delivered weekly by expert consultants. Enjoy step-by-step guides, weekly Q&A sessions, and full access to our AI workflow archive.
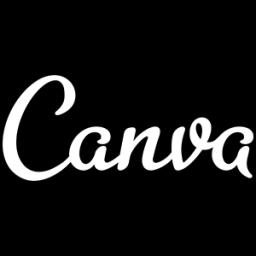
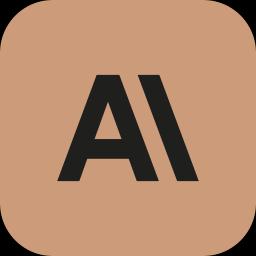
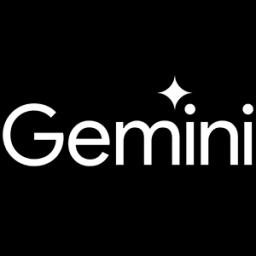
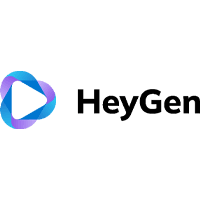
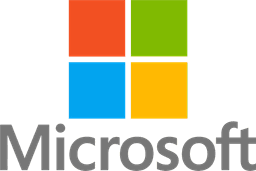
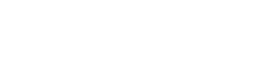
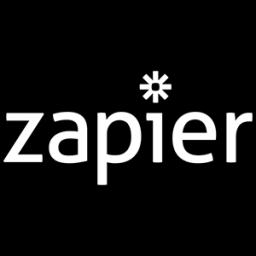
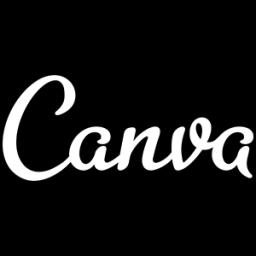
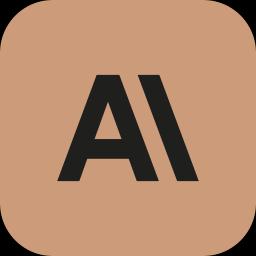
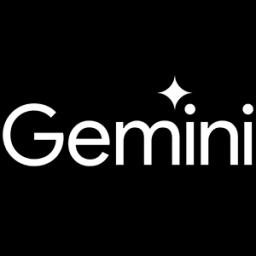
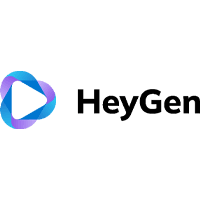
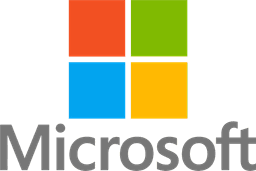
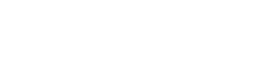
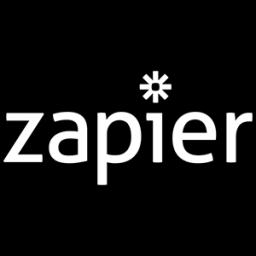
Future Implications and Challenges
In the face of accelerating climate change, WeatherNext stands at a pivotal intersection of technological innovation and environmental necessity. The AI-driven models developed by Google DeepMind harness vast datasets to produce more accurate weather forecasts, but their reliance on historical data does invite concerns. As the climate becomes increasingly unpredictable, the foundational datasets on which these models are trained might pose challenges in forecasting future weather patterns. This gap underscores the need for continuous refinement and integration of real-time climate data, ensuring that forecasting models like WeatherNext remain robust against evolving climatic conditions [2](https://www.climateforesight.eu/articles/reacting-to-the-ai-revolution-in-weather-forecasting/).
The implementation of AI in weather prediction systems, such as WeatherNext, brings with it profound economic and social implications. By providing more reliable and timely forecasts, sectors ranging from agriculture to public energy management stand to benefit considerably. For instance, being able to anticipate weather conditions with greater accuracy could substantially reduce the economic impact of agricultural losses due to weather variability, enhancing crop security and stability. Moreover, optimized predictions can lead to efficient energy distribution, cutting down unnecessary costs and reducing the carbon footprint of power generation [2](https://www.climateforesight.eu/articles/reacting-to-the-ai-revolution-in-weather-forecasting/).
Despite these promising advancements, there is significant discourse about the centralization of AI weather technologies among giant tech corporations like Google. This centralization raises issues around accessibility and equitable technology dissemination, especially when essential tools that serve public interest are under the purview of private entities. The partial open-sourcing of some components of WeatherNext, while a step towards more transparency, may be perceived as insufficient to ensure that this becomes a publicly beneficial resource. This scenario calls for robust international collaboration to establish standards and protocols that govern AI weather forecasting, so the benefits are universally accessible rather than solely driven by commercial interests [2](https://www.producthunt.com/posts/weathernext-by-google?ref=upstract.com).
Globally, accurate weather forecasts have strategic importance, especially as nations compete technologically. Advances in AI weather models could spur competitive development among countries, potentially shifting geopolitical landscapes. The pursuit of such technologies highlights the necessity for international partnerships and agreements to facilitate data sharing and collaborative development. These endeavors, guided by fairness and accessibility, can enhance global resilience against extreme weather events and ensure public safety without exacerbating technology-driven divides between nations [8](https://medium.com/@hassantaher-blog/the-revolutionary-impact-of-deepmind-on-weather-forecasting-8f1f0d4191e3).
The transformative potential of WeatherNext is not confined within meteorological boundaries; it extends to policy-making and infrastructure planning, especially in disaster management and risk mitigation. By improving the predictive capacity of extreme weather events, policymakers can make more informed decisions to safeguard communities. However, these advancements also emphasize the importance of international cooperation to maintain data transparency and address ethical concerns surrounding AI in public domains. As these technologies evolve, societal trust and investment in AI-driven models will be crucial determinants of their successful integration and effectiveness in real-world applications [5](https://www.technologyreview.com/2024/12/04/1107892/google-deepminds-new-ai-model-is-the-best-yet-at-weather-forecasting/).
Conclusion
In conclusion, WeatherNext by Google DeepMind epitomizes a remarkable advancement in weather forecasting technology, setting a new standard in prediction accuracy and efficiency. By utilizing advanced AI models, WeatherNext surpasses traditional physics-based methods, offering both deterministic and ensemble forecasts with varying lead times. This flexibility allows for a more comprehensive understanding of potential weather scenarios, ranging from immediate forecasts to fifteen-day outlooks [1](https://deepmind.google/technologies/weathernext/).
Learn to use AI like a Pro
Get the latest AI workflows to boost your productivity and business performance, delivered weekly by expert consultants. Enjoy step-by-step guides, weekly Q&A sessions, and full access to our AI workflow archive.
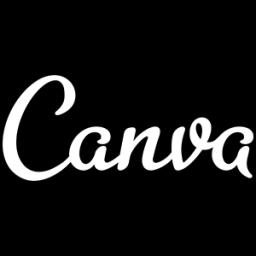
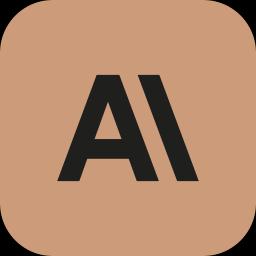
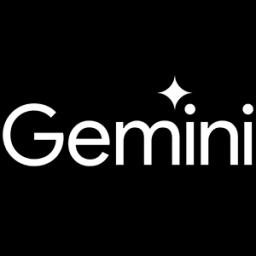
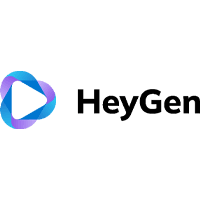
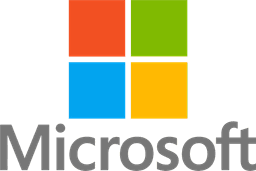
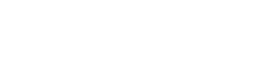
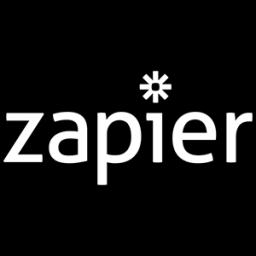
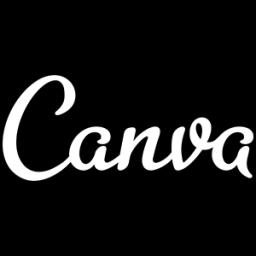
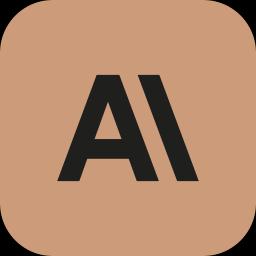
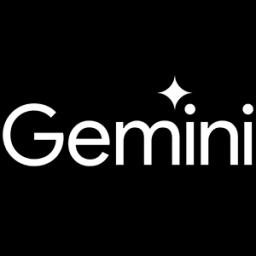
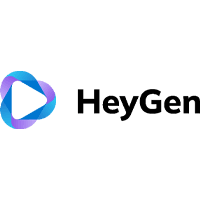
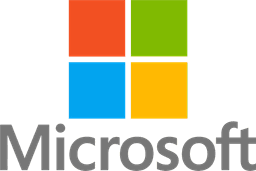
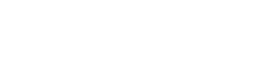
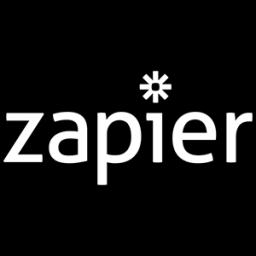
The public reception of WeatherNext underscores its transformational potential, yet it also highlights broader discussions about accessibility and the ethical implications of private control over crucial forecasting technologies. While feedback has been generally positive, concerns have been raised about the potential exclusivity of access and the partial open-sourcing of the models. Thus, ensuring that these advances are shared equitably and not solely as commercial assets remains a critical challenge [2](https://www.reddit.com/r/google/comments/1fraogo/how_is_this_possible_google_weather_forecast/).
Looking to the future, WeatherNext's implications for both society and the economy are profound. From enhancing disaster preparedness to optimizing energy management and agricultural productivity, the potential applications are vast. However, given the reliance on historical data, there's an essential need to adapt these models in the face of changing climate patterns. This challenge calls for ongoing innovation and collaboration to sustain its promise of improved weather intelligence [11](https://artsci.tamu.edu/news/2024/12/five-questions-how-ai-is-shaping-the-future-of-weather-forecasting.html).
As AI-driven weather forecasting like WeatherNext becomes more prevalent, its role could significantly influence geopolitical landscapes, shaping the future of technological competition and collaboration among nations. The strategic importance of accurate forecasts may lead to a new era of international partnerships or rivalries, highlighting the necessity for comprehensive and inclusive data-sharing protocols that balance innovation with equitable access [8](https://medium.com/@hassantaher-blog/the-revolutionary-impact-of-deepmind-on-weather-forecasting-8f1f0d4191e3).