Revolutionizing Efficiency in AI Models
Google's Gemini 2.5: A Game Changer in AI with Implicit Caching
Last updated:

Edited By
Mackenzie Ferguson
AI Tools Researcher & Implementation Consultant
Google has introduced its Gemini 2.5 models, featuring a groundbreaking implicit caching capability that aims to enhance AI efficiency. This development is sparking discussions in the AI community about its potential impact and advantages over traditional methods. The new feature is set to optimize performance and reduce latency, making AI applications faster and more reliable.
Introduction to Gemini 2.5 Models
The Gemini 2.5 models represent a significant advancement in artificial intelligence, providing enhanced capabilities and performance improvements over their predecessors. One of the most notable features of these models is the incorporation of implicit caching, which streamlines processes and boosts computational efficiency. This new capability means that processes requiring iterative calculations can be completed more swiftly, enhancing the overall responsiveness of systems utilizing these models. For a deeper dive into how implicit caching functions within the Gemini 2.5 models, you can refer to the official announcement on the Google Developers Blog, where the technology is explored in greater detail.
Enhancements in Implicit Caching
Implicit caching has become a cornerstone in optimizing data retrieval and enhancing computational efficiency. As highlighted in the recent updates from Google's Gemini v2.5 models, implicit caching allows systems to store and retrieve data seamlessly without explicit commands from the user. This means that frequently accessed data is readily available, reducing retrieval times and enhancing overall system performance. By allowing automatic management of cache storage, implicit caching minimizes the latency traditionally associated with data retrieval, thereby improving the responsiveness of applications.
Learn to use AI like a Pro
Get the latest AI workflows to boost your productivity and business performance, delivered weekly by expert consultants. Enjoy step-by-step guides, weekly Q&A sessions, and full access to our AI workflow archive.
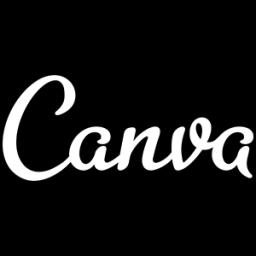
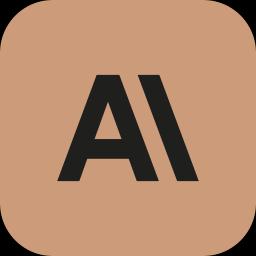
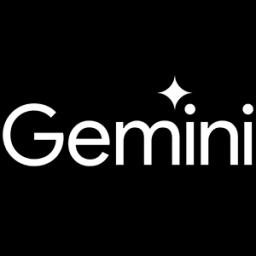
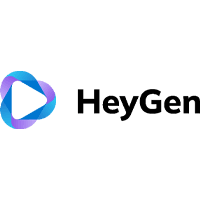
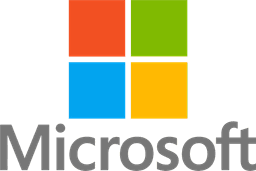
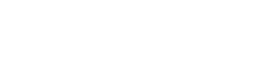
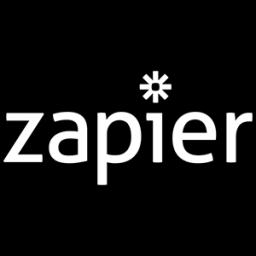
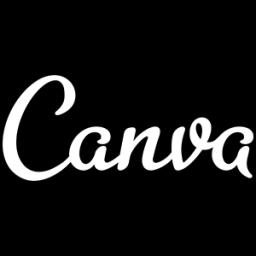
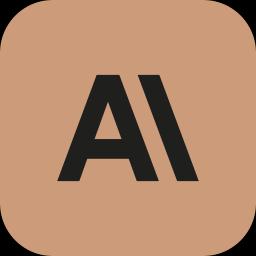
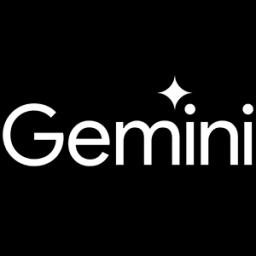
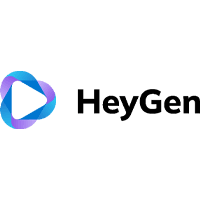
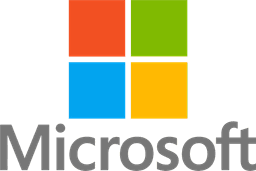
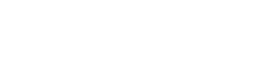
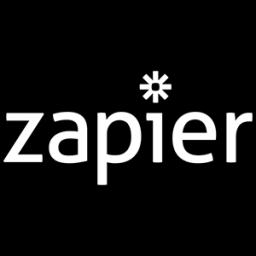
The move to integrate implicit caching into more systems offers a significant advantage in handling large volumes of data. With the availability of implicit caching in the Gemini v2.5 models, developers can now build more robust applications that handle data more efficiently. This is particularly beneficial in real-time applications where rapid data access is paramount. As the demand for faster and more efficient data processing grows, the role of implicit caching will become even more crucial, leading to innovations and improvements in various technological domains.
Technical Specifications and Improvements
The latest model enhancements introduce significant improvements in efficiency and performance. Leveraging *Gemini 2.5*, these updates provide implicit caching support, which drastically optimizes processing times by reducing the need for repeated data fetching. This improvement marks a considerable advancement in computational operations, enhancing both speed and accuracy without additional configuration required from the user. For those keen on the technical intricacies, more detailed insights can be found on the official announcement here.
The integration of implicit caching within the Gemini models signifies a strategic shift towards developing systems that are not just faster but also more intuitive in handling data. This feature allows for intelligent data management by preloading data that the system predicts will be used, thus ensuring it is readily available without delays. This cutting-edge approach facilitates seamless operations, particularly in data-intensive scenarios where real-time processing is crucial. Insights from developers indicate that this enhancement may reduce operational costs and improve resource allocation, further establishing the model's efficiency.
Beyond the immediate technical upgrades, the introduction of implicit caching is expected to influence future AI model developments significantly. By emphasizing the importance of speed and efficiency, it sets a new benchmark for forthcoming models, encouraging further innovation in data management strategies. Stakeholders have responded positively, showing enthusiasm for the potential reduction in latency and enhanced system responsiveness. As industries continue to demand faster data processing capabilities, these improvements underscore a pivotal move towards more agile and robust AI models. The broader implications of these advancements can be explored in the detailed coverage on their development blog here.
Learn to use AI like a Pro
Get the latest AI workflows to boost your productivity and business performance, delivered weekly by expert consultants. Enjoy step-by-step guides, weekly Q&A sessions, and full access to our AI workflow archive.
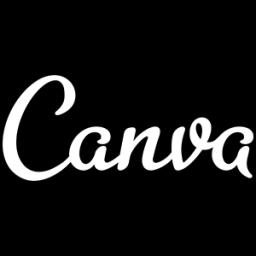
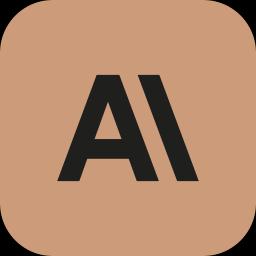
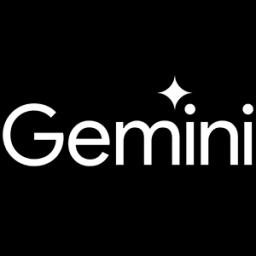
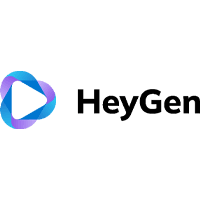
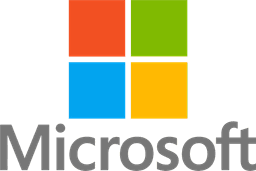
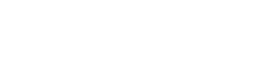
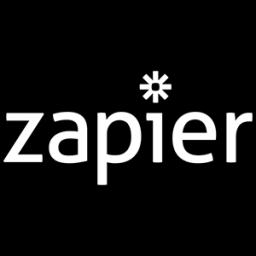
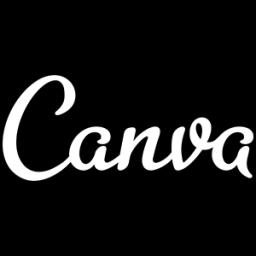
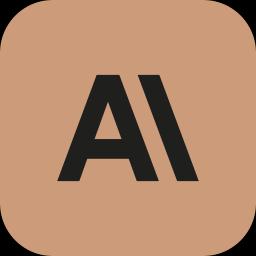
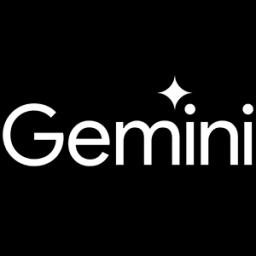
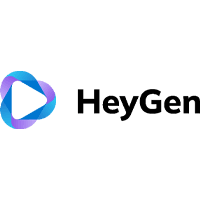
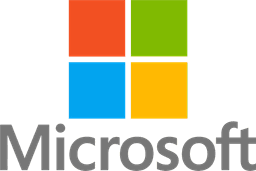
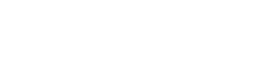
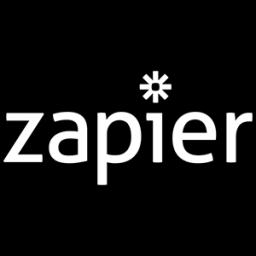
Reactions from the Developer Community
The introduction of the Gemini 2.5 models, as detailed on the Google Developers Blog, has sparked significant discussions within the developer community. Many developers have embraced the update for its potential to streamline processes through implicit caching, thereby enhancing efficiency. The ability to cache content automatically could potentially reduce latency, a feature particularly prized by developers focused on performance-oriented applications.
According to feedback gathered from various online forums and social media platforms, a segment of the developer community has expressed eagerness to explore the new functionalities provided by the Gemini 2.5 models. However, some developers have also voiced concerns over the integration complexities of these new models into existing systems. Despite these concerns, the overall sentiment remains optimistic, largely because the update promises improvements in operational efficiency without requiring extensive manual configurations.
Expert developers have weighed in with their views on how the implicit caching feature could redefine coding practices in web development. By alleviating the manual labor associated with cache management, it is anticipated that this new capability will allow developers to refocus their efforts on creating innovative solutions and features. As noted on the official blog, this development is likely to inspire further advancements and discussions within the technology community.
Looking at the future implications, the enhancements brought by the Gemini 2.5 models could set a new standard for other technology companies striving to optimize their own platforms. The developer community, continuously seeking ways to advance technological capabilities, could find new pathways for innovation as a result. The ongoing discourse in tech circles highlights a shared interest in leveraging these advancements to foster more groundbreaking developments that push the boundaries of what current technology can achieve.
Implications for Future Development
The recent developments in AI and machine learning, exemplified by the advancements in Google's Gemini 2.5 models, have opened new avenues for innovation. These models now supporting implicit caching introduce a paradigm shift in how machine learning operations can be streamlined for enhanced efficiency and performance in data-intensive environments. The ability to cache implicitly means that operations requiring frequent data fetching can now be executed with reduced latency, thereby increasing the throughput of AI applications. As Google elaborates in their blog, these improvements could potentially lead the way for more resilient and intelligent systems capable of operating seamlessly in complex digital ecosystems. Read more here.
In the realm of future technology development, the enhancements brought by models like Gemini 2.5 signify a transition towards more adaptive and autonomously functioning AI models. The implicit caching feature not only enhances data retrieval speeds but also signifies a step towards reducing computational resources, which is crucial for sustainable tech development. Such efficiency gains are pivotal for future applications in areas such as autonomous driving, real-time data processing in IoT devices, and sophisticated user interaction platforms, where speed and responsiveness are critical. As these advancements continue to unfold, they are expected to pave the way for more robust AI solutions that align with the industry's push towards green computing. Explore further details.
Learn to use AI like a Pro
Get the latest AI workflows to boost your productivity and business performance, delivered weekly by expert consultants. Enjoy step-by-step guides, weekly Q&A sessions, and full access to our AI workflow archive.
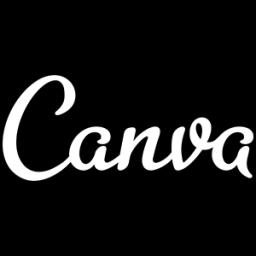
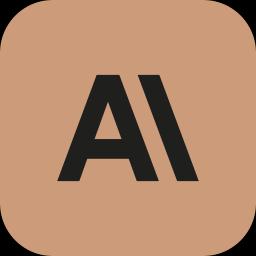
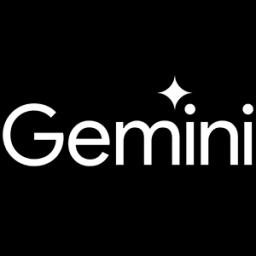
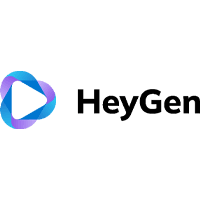
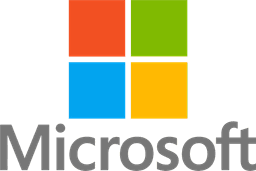
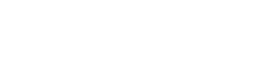
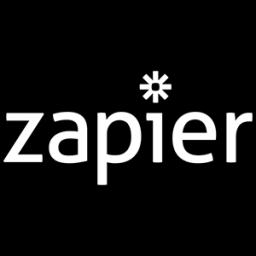
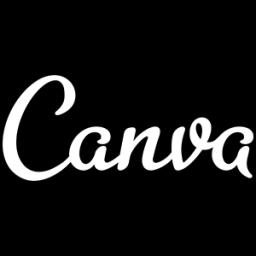
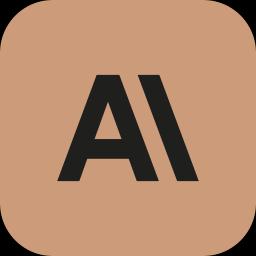
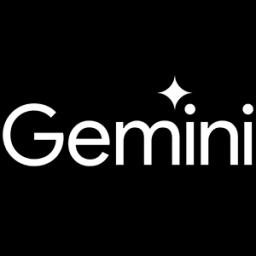
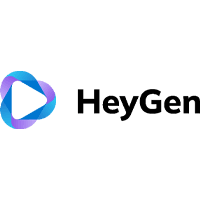
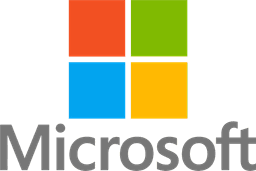
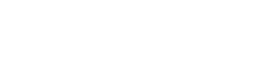
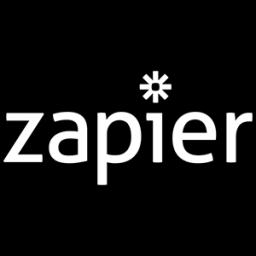
Furthermore, the integration of implicit caching in machine learning models like Gemini 2.5 suggests an exciting trajectory for AI development. Given the increasing demand for real-time data processing across various sectors, the implications are profound in terms of computational efficiency and cost reduction. This feature could lead to more dynamic pricing models for AI services, where reduced computational overheads translate to cost savings for businesses and developers. Additionally, by leveraging this technology, developers could innovate more sophisticated models that offer enhanced predictive analytics capabilities, giving businesses a competitive edge. For an in-depth understanding of these developments, you can visit Google's detailed blog post here.
Conclusion
In conclusion, the advancements introduced with Gemini 2.5 models have sparked intrigue and anticipation in the tech community. These models are now capable of supporting implicit caching, a feature that significantly optimizes computational efficiency and data handling. The news about this feature was shared on the Google Developers Blog, highlighting a critical leap forward in the development of AI models both in terms of performance and scalability. This aligns with Google’s ongoing commitment to innovation in AI technology, paving the way for more sophisticated and seamless user experiences.
While experts are excited about this development, they are also considering the broader implications of such advancements. As AI models become more efficient, there is potential for enhanced applications in various fields, ranging from improving search algorithms to advancing machine learning capabilities. This enhanced efficiency might also stimulate competition among leading tech firms, driving further innovation in AI and related technologies.
The public reaction has been notably positive, with many users eager to see how these improvements will translate into daily applications. The introduction of implicit caching is seen not just as a technical upgrade but also as a forward-thinking strategy that sets a new benchmark in the AI industry. Moving forward, it is expected that such innovations will substantially influence future AI model developments, encouraging a shift towards more sustainable and efficient computational processes.