Elon's AI Drama!
Grok 3: xAI's Latest AI Ambition Hits Delay - What's the Stall About?
Last updated:

Edited By
Mackenzie Ferguson
AI Tools Researcher & Implementation Consultant
xAI's highly awaited Grok 3 artificial intelligence model has missed its 2024 release date, adding to the growing trend of AI industry delays. Amid industry-wide challenges, hints of an interim release, Grok 2.5, spark curiosity. What does this mean for AI's future?
Introduction to Grok 3's Delay
The world of artificial intelligence is rife with surprises, and the recent delay of xAI's highly anticipated Grok 3 model is no exception. Initially slated for a grand release in 2024, the AI community was abuzz with expectations, largely fueled by Elon Musk's ambitious promises about the revolutionary advancements Grok 3 would bring. Hailed as the next leap forward in AI technology, Grok 3 was expected to be trained on an unprecedented scale of GPU clusters, promising to redefine capabilities in image analysis, question-answering, and integration with platforms like Twitter, or 'X' as it is now branded.
However, as the curtain fell on 2024 without the model's release, a cascade of industry-wide concerns and critiques followed. This setback is not an isolated case but part of a broader trend of high-profile delays in the AI sector. It's a vivid reminder of the unpredictabilities that come with pushing the boundaries of technology amidst scaling law constraints. Previous instances include setbacks like Anthropic's delayed Claude 3.5 Opus, which similarly faced challenges that impede progress despite expansive data use and computational efforts.
Learn to use AI like a Pro
Get the latest AI workflows to boost your productivity and business performance, delivered weekly by expert consultants. Enjoy step-by-step guides, weekly Q&A sessions, and full access to our AI workflow archive.
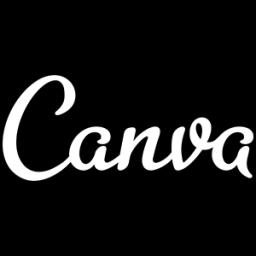
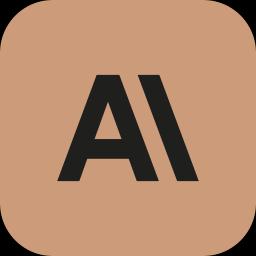
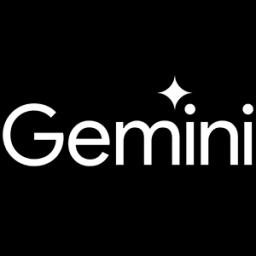
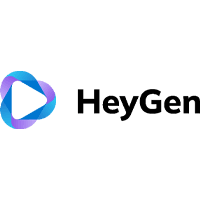
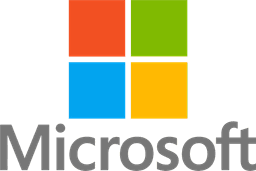
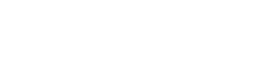
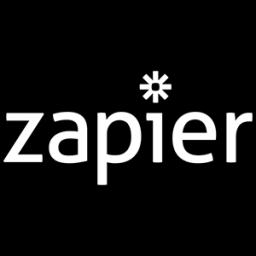
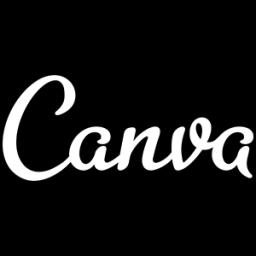
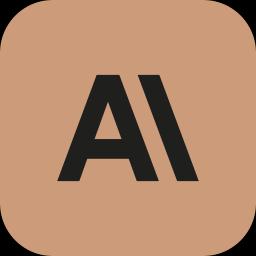
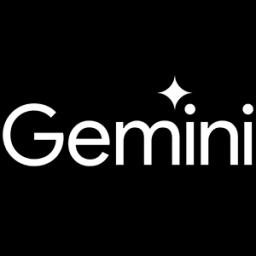
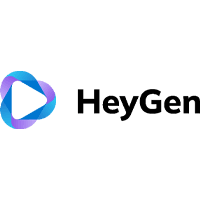
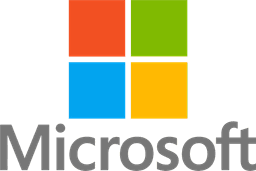
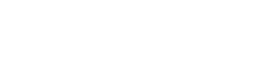
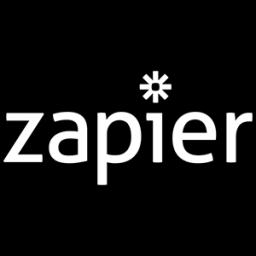
This ongoing trend of delayed launches stirs compelling discussions on the future of AI scalability. Researchers and developers acknowledge the constraints of current scaling laws, questioning whether further enlargement in model size yields proportionate performance gains. As Dr. Timnit Gebru, a notable AI ethics researcher, pointed out, a critical issue arises from the smaller team dynamics at xAI compared to their larger, more resource-rich competitors. These issues point not only to logistical constraints but also highlight a need for innovative strategies in AI development as met by competitor domains.
Hints of an interim release of Grok 2.5 emerged, a potential pivot point for xAI amidst public anticipation and industry skepticism. Described as 'Our most intelligent model' to date, Grok 2.5 could serve as a strategic intermediary, potentially smoothing xAI's path to releasing Grok 3 while grappling with developmental and scalability hurdles. However, this raises crucial questions on how xAI plans to realize its ambitious goals under such conditions, especially when contending with performance benchmarks set by titans like OpenAI's GPT-4 and Google's Gemini.
The ripple effects of Grok 3's delay extend beyond technical aspects, potentially reshaping economic and strategic landscapes in AI. As Professor Stuart Russell from UC Berkeley emphasized, the road to artificial general intelligence (AGI) is proving to be non-linear, prompting stakeholders to recalibrate their expectations towards qualitative, not just quantitative, improvements. Thus, this push for novel architectural designs and refined training methodologies may not just redefine AI's horizon but is seen as imperative for continued progress amidst growing pains in the industry.
Historical Context of AI Delays
Artificial Intelligence (AI) has been at the forefront of technological advancement, promising transformative impacts across industries. However, the journey towards realizing these promises has often been fraught with delays and unmet expectations, particularly concerning the development and deployment of next-generation AI models. The recent announcement that xAI's Grok 3 model has missed its anticipated 2024 release date is a case in point, reflecting a broader industry trend where companies consistently struggle to meet their ambitious timelines.
Learn to use AI like a Pro
Get the latest AI workflows to boost your productivity and business performance, delivered weekly by expert consultants. Enjoy step-by-step guides, weekly Q&A sessions, and full access to our AI workflow archive.
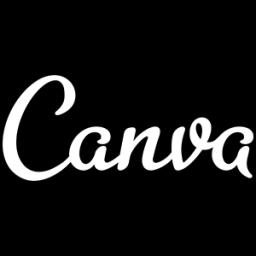
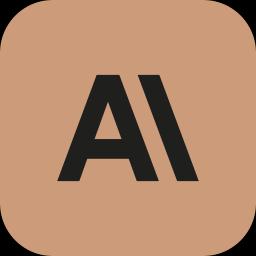
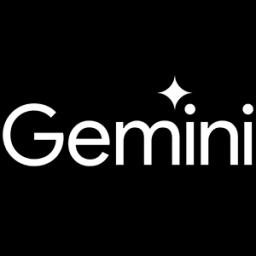
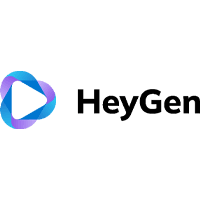
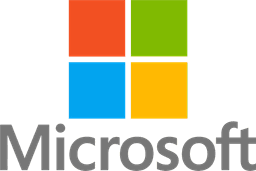
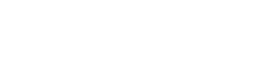
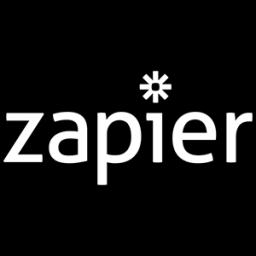
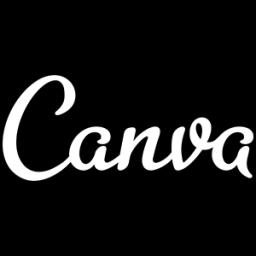
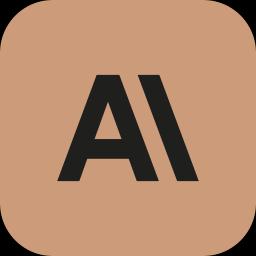
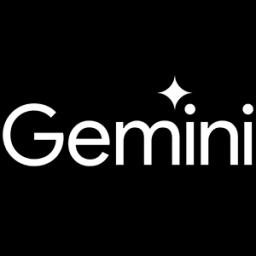
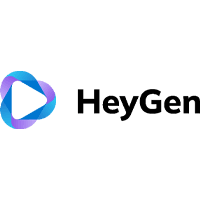
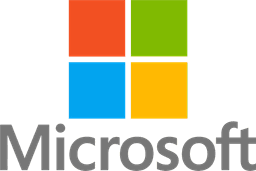
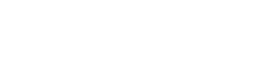
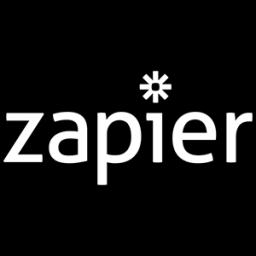
The failure of Grok 3 to debut on schedule adds to a list of AI models facing similar setbacks, including those from competitors like Anthropic with its Claude 3.5 Opus. These delays raise critical questions about the challenges inherent in scaling AI technologies, which were once believed to be remedied simply by increasing computational resources and model size. However, it appears that such scaling laws are exhibiting diminishing returns, prompting a reevaluation of AI development methodologies.
Elon Musk, who has been an advocate for the forward momentum of AI, had previously praised Grok 3 for its advanced capabilities and the massive GPU clusters used in its training. This makes its delay particularly notable and concerning. There are hints of an interim release of Grok 2.5, which xAI has described as 'Our most intelligent model,' but no firm dates have been set, leaving stakeholders and the public in anticipation yet unsure of the exact timeline.
The delay not only impacts the perception of xAI and its ability to deliver groundbreaking AI technologies but also reflects broader challenges within the field. These include questions regarding the scalability of training AI models, the effectiveness of current technological approaches, and the resource limitations faced by smaller firms compared to industry giants, which can exacerbate disparities in AI innovation.
Going forward, the implications of Grok 3's delay are multifaceted, affecting economic projections, public expectations, and strategic directions within the AI sector. There is an increased call for innovative solutions to overcome current limitations posed by existing scaling laws. Moreover, as public skepticism grows over the reliability of launch timelines, the industry faces heightened pressure to align promises with capabilities, marking a pivotal moment for AI development and deployment strategies.
Challenges Facing xAI in Developing Grok 3
Developing Grok 3 by xAI has been riddled with several challenges, contributing to its missed 2024 launch target. Firstly, the trend of AI models missing deadlines is not isolated to Grok 3 but is evident across the industry, as seen with Anthropic's Claude 3.5 Opus. The ambitious timelines set for Grok 3 were ambitious, with its launch previously emphasized by Elon Musk as a leap in advancement, trained on a massive GPU cluster. However, this ambition seems to be contrasted by the realities of scaling AI technology, especially with xAI's relatively smaller team compared to its competitors like OpenAI and Google. These organizations possess more resources to tackle the intensive demands of AI model development.
The delay of Grok 3 raises pertinent questions about the scalability of AI training infrastructure. Emerging theories suggest diminishing returns despite traditional reliance on increasing model size, data, and computational power. The delay illustrates the possible limitations of current scaling laws and brings attention to the fact that achieving higher performance through mere scaling might not be sustainable. This has necessitated a reevaluation of growth targets against what is achievable under existing technological constraints, reflecting a need for innovative solutions beyond size and compute capabilities.
Learn to use AI like a Pro
Get the latest AI workflows to boost your productivity and business performance, delivered weekly by expert consultants. Enjoy step-by-step guides, weekly Q&A sessions, and full access to our AI workflow archive.
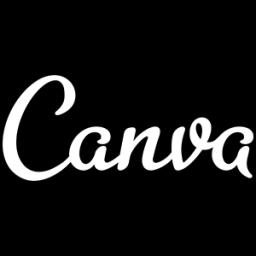
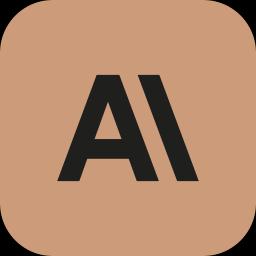
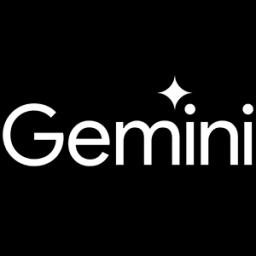
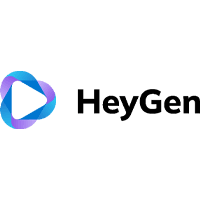
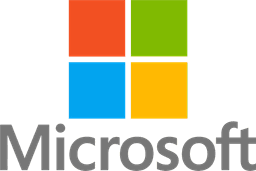
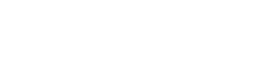
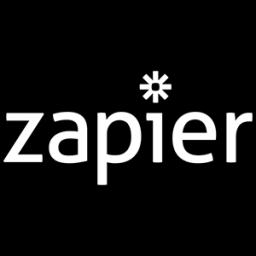
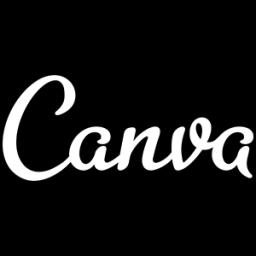
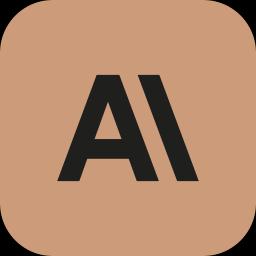
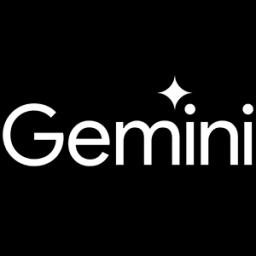
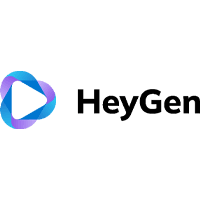
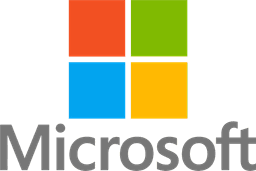
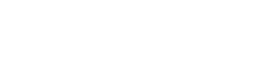
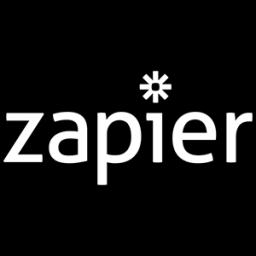
Amidst the Grok 3 delay, there’s a potential release of Grok 2.5, highlighted as an interim solution and described as "Our most intelligent model". This step might be seen as pragmatic, preparing the field for an eventual Grok 3 launch. Nonetheless, the absence of confirmed release dates for either version underscores uncertainties regarding the schedule of advancements, echoing a wider issue of reliability in delivery timelines within the AI sector.
Another significant challenge for xAI is related to broader industry practices, where optimization for specialized, task-centric AI models is becoming more prevalent. For instance, Meta’s approach of developing smaller, more efficient models for specific tasks represents a significant shift from the erstwhile "bigger is better" belief, also seen in Google's PaLM and DeepMind's initiatives focusing on AI safety. These strategies suggest an industry pivot towards more sustainable developments, balancing capability with ethical safety frameworks.
Furthermore, expert opinions underline the broader implications of Grok 3's delay. The growing complexity of AI development and its scalability challenges hint at a potential plateau phase for traditional AI scaling laws. Industry thought leaders like Dr. Dario Amodei and Dr. Timnit Gebru reiterate the need for novel architectures and the importance of addressing resource disparities within AI entities compared to tech giants. This highlights the requirement for inclusive strategies that democratize AI capabilities, ensuring smaller entities are not outpaced by resource-intensive demands.
Comparative Analysis: Grok vs Other AI Models
The emergence of Grok 3, a model developed by xAI, has generated significant discussion, especially given its delayed release that was initially announced for 2024. As the AI industry repeatedly experiences setbacks with model release schedules, Grok 3's situation sheds light on several deeper challenges. The delay mirrors xAI's acknowledged constraints: the ambitious goals set forth were perhaps underestimated in terms of complexity and resources needed. Comparatively, xAI operates with a smaller team than its prominent competitors, underscoring the difficulties smaller players face in a domain dominated by tech giants. Notably, Grok 3 had been anticipated to bring substantial upgrades over earlier variants, targeting advancements in image analysis, question answering, and integration with platforms like X (formerly Twitter). Elon Musk highlighted its potential, yet the postponement has raised concerns about the feasibility of current AI scaling methodologies, hinting that for Grok 3 and other models to meet their projected capabilities, innovative strategies beyond traditional scaling might be essential.
Grok 3 is directly competing with other leading models such as OpenAI's GPT-4 and Google's Gemini. While these models have already established their importance, Grok 3 was hailed as a contender that could potentially set new standards in AI functionality. Designed for sophisticated tasks such as image analysis and interactive communication, it also promised enhanced compatibility with digital platforms, particularly benefiting social media analytics and user engagement. Despite this, the focus now shifts to ensuring the release of an interim model, Grok 2.5, which is branded as xAI's smartest model to date. This could serve as a litmus test for the promised capabilities of Grok 3, potentially alleviating some skepticism associated with its delay.
The delay of Grok 3 is not an isolated incident but part of a broader trend reflecting the challenges associated with contemporary AI scaling laws. These scaling laws, which once promised direct improvements in AI performance through increased parameters and data, are showing signs of diminishing returns. This shift has spurred discussions within the AI community about the need for innovative architectural solutions and training models that don't rely purely on size increase. Experts like Dr. Dario Amodei and Professor Stuart Russell articulate a growing consensus: the field needs a paradigm shift from quantitative to qualitative growth, focusing on refining compositions and strategies that maximize performance without depending solely on scale. As AI companies recalibrate their expectations, there's an acknowledgement that traditional scaling routes could reach an impasse.
Learn to use AI like a Pro
Get the latest AI workflows to boost your productivity and business performance, delivered weekly by expert consultants. Enjoy step-by-step guides, weekly Q&A sessions, and full access to our AI workflow archive.
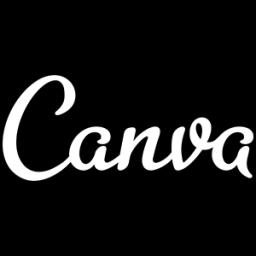
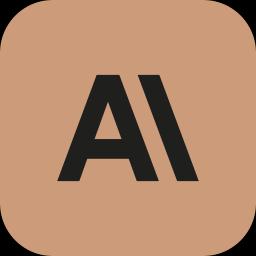
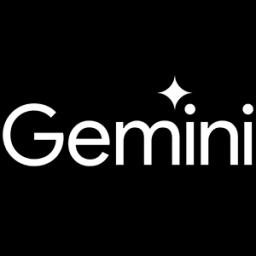
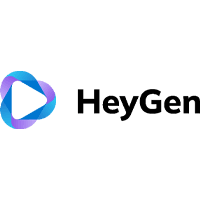
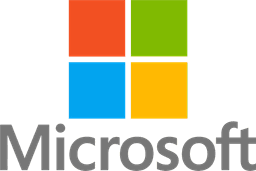
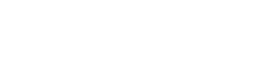
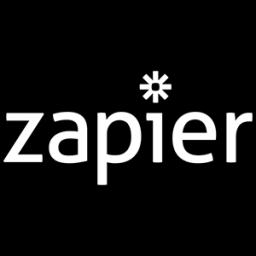
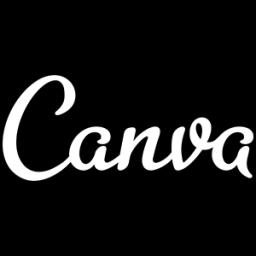
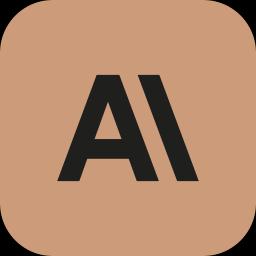
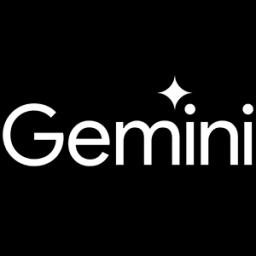
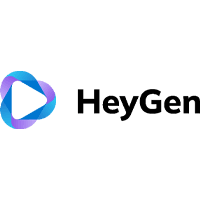
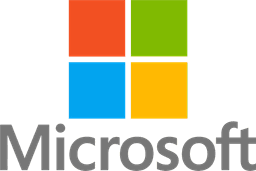
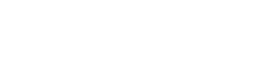
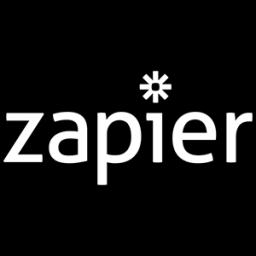
The AI industry's current trajectory poses vital implications not only for technological development but also for economic, social, and political arenas. The economic landscape could see a pivot with investments leaning towards techniques boosting AI efficiency rather than raw computational power, which historically has been the main method for breakthrough achievements. Socially, there is a growing recalibration of public expectations concerning AI advancements, and an emphasis on embedding AI ethics and safety into developmental models gains momentum. Politically, the status quo of AI development calls into question the role of government regulation, highlighting the need for policies to ensure equitable competition and prevent technological monopolization. Future AI advancements are expected to emphasize specialized, agile models rather than larger, cumbersome systems, thereby enabling more refined deployments across varying applications.
Critically, the Grok 3 delay prompts a reassessment of long-term AI developmental goals. The path to artificial general intelligence (AGI) is increasingly acknowledged as non-linear, demanding a focus on qualitative enhancement over sheer quantitative expansion. As AI evolves, the need to reconsider foundational objectives under evolving technological boundaries instead of pursuing a mechanical scalability race is paramount. Hence, Grok 3's delay could serve as a pivotal learning point for AI developers to prioritize cohesive and adaptable development processes, fostering an ecosystem where continuous learning and innovation are ingrained in the pursuit of advanced AI capabilities.
Understanding AI Scaling Laws
AI scaling laws describe how the performance of AI models improves as the resources allocated for their training and deployment, such as the number of parameters, dataset size, and computational power, are increased. In earlier stages of AI development, increasing these resources often yielded substantial gains in model performance. For example, deepening the layers in neural networks or using more extensive datasets typically led to improved accuracy and broader capabilities.
However, recent advancements and research into AI scaling laws suggest that this approach of merely scaling resources might not indefinitely result in proportional performance improvements. There's growing evidence that AI systems may face diminishing returns when expanded beyond a certain point. This challenges the traditional belief that larger models, given enough data and computation, will continue to outperform their predecessors effectively.
The delays faced by cutting-edge AI models like Grok 3 by xAI point to the limitations of scaling laws. Despite ambitious goals and substantial resources, these models sometimes fail to meet performance expectations within projected timeframes. Factors such as team size, resource allocation, and overly ambitious timelines contribute to these setbacks, but core among them is the inherent challenge posed by scaling laws as we currently understand them.
Notably, these challenges are not unique to xAI. They reflect a broader trend within the AI industry, where companies like OpenAI and Google also encounter obstacles to producing new, more powerful iterations of their AI systems. The focus is gradually shifting from simply enlarging models to exploring innovative architectures and methodologies that promise more efficient and effective performance without being constrained by the scaling laws.
Learn to use AI like a Pro
Get the latest AI workflows to boost your productivity and business performance, delivered weekly by expert consultants. Enjoy step-by-step guides, weekly Q&A sessions, and full access to our AI workflow archive.
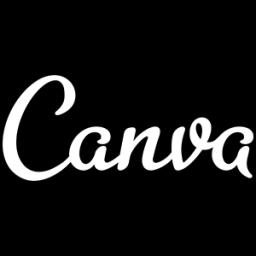
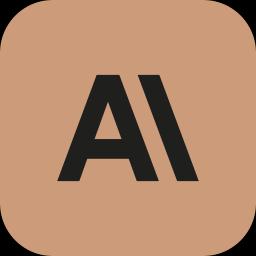
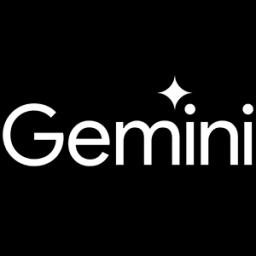
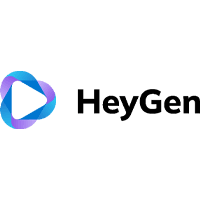
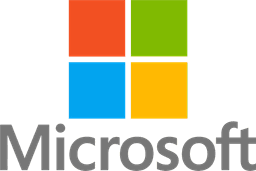
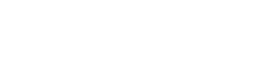
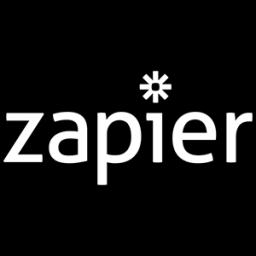
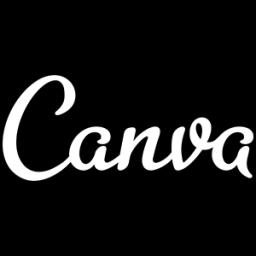
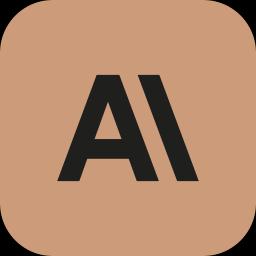
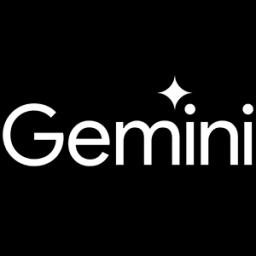
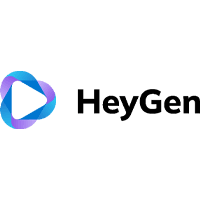
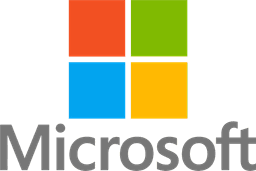
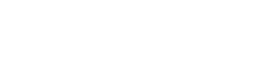
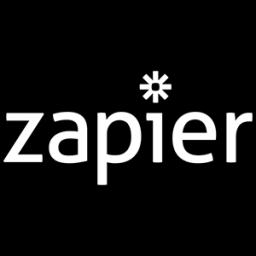
The implications of stretching the limits of AI scaling laws resonate deeply across technological and academic communities. They underline a need for a paradigm shift toward qualitative improvements in AI research and development. By prioritizing novel training techniques and architectures, the AI community aims to overcome current limitations, steering towards more sustainable and revolutionary advancements in the field.
In the context of Grok 3's delay and similar events, the industry is beginning to recognize the importance of recalibrating expectations concerning AI progress. This recalibration involves acknowledging that growth along traditional scaling lines is proving difficult, and innovation will likely stem from rethinking approaches and overcoming the bottlenecks identified in existing scaling laws. New breakthroughs in AI may not only depend on more or better resources but could hinge on how intelligently these resources are structured and applied.
Projected Release Timelines for Grok 2.5 and 3
The much-anticipated Grok 3, xAI's flagship next-generation AI model, has experienced a significant delay in its release, which was initially slated for 2024. xAI, founded by Elon Musk, is facing similar challenges to those encountered by other industry players, leading to the missed timeline. Such delays are becoming increasingly common in the field, exemplified by other instances such as Anthropic's postponed release of its Claude 3.5 Opus model.
The delay of Grok 3 isn't just a reflection of scheduling issues but poses critical questions regarding the scalability of AI training. These setbacks bring to light the limitations of current scaling laws, which dictate the relationship between the size of AI models—defined by parameters, data, and computational power—and their performance. While growing these aspects traditionally led to performance enhancements, emerging evidence suggests we're approaching a point where the returns are diminishing.
Hints from xAI's communication channels suggest the possibility of an interim release named Grok 2.5, portrayed as their most intelligent model yet. However, there are still no definite release dates for Grok 2.5 or Grok 3, leaving stakeholders and potential users in a state of anticipation.
The broader implications of such delays are far-reaching for the AI industry. On one front, these challenges emphasize a paradigm shift within the industry that favors qualitative architectural advancements over mere quantitative scaling. Moreover, the persistence of these issues could lead to increased investment in more efficient AI models focused specifically on accomplishing designated tasks rather than broader, more generalized improvements.
Learn to use AI like a Pro
Get the latest AI workflows to boost your productivity and business performance, delivered weekly by expert consultants. Enjoy step-by-step guides, weekly Q&A sessions, and full access to our AI workflow archive.
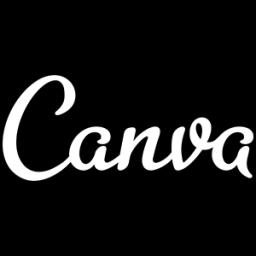
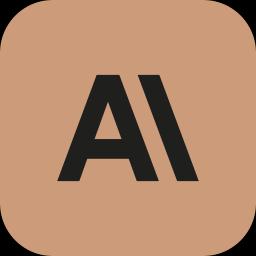
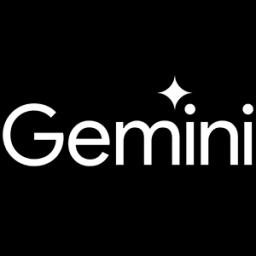
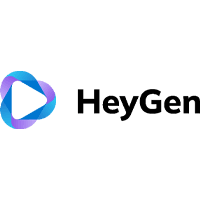
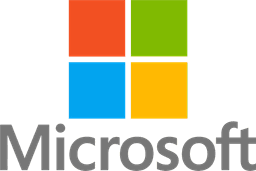
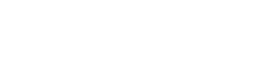
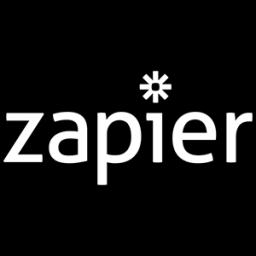
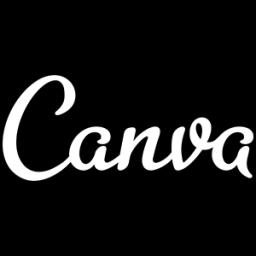
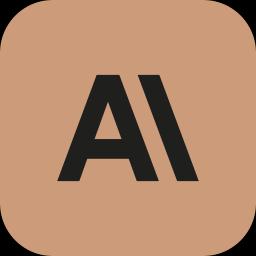
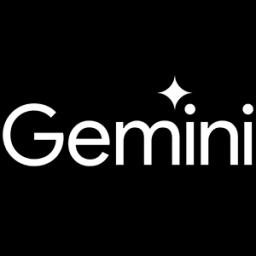
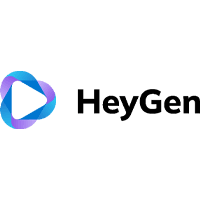
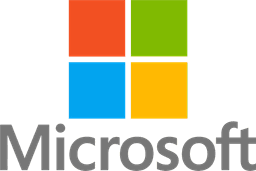
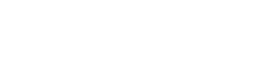
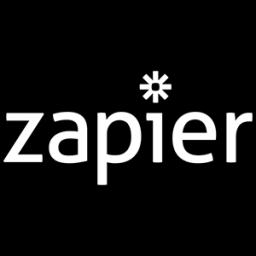
AI experts like Dr. Dario Amodei and Dr. Timnit Gebru have voiced concerns regarding the scalability challenges and the disparities in resource allocation between tech giants and smaller AI firms. This disparity is becoming a focal point of discussion in the AI community, underlining issues of equitable development and innovation within the field.
Public reactions to the news of Grok 3's delay span a spectrum of disappointment, skepticism, and pragmatic acceptance. While some express frustration over the unmet expectations, others take the delay as a reminder of the industry-wide challenges in AI scalability and performance optimization. This has sparked widespread discourse about the reliability and transparency of AI development timelines.
Broader Implications for AI Development
The delay in the release of xAI's Grok 3 model holds significant implications for the broader field of AI development. One of the primary issues highlighted by this delay is the challenge of scaling AI models. Many in the industry have relied heavily on the principle that increasing model size and data input will continue to lead to enhanced performance. However, Grok 3's delay shines a spotlight on the diminishing returns of this approach, indicating that the field may have reached a plateau in terms of what can be achieved by simply scaling existing technologies.
This situation puts a spotlight on the need for the AI industry to innovate beyond traditional scaling laws. As companies like OpenAI and Anthropic also experience delays with their respective models, the pressure mounts for new methodologies and architectures that can break the current deadlock. The industry must prioritize research and development into more efficient models that do not solely depend on computational power and extensive datasets. This could involve exploring alternative model architectures or novel training techniques that can achieve intended breakthroughs without the necessity of exponential scaling.
Moreover, the delay of Grok 3 raises questions about resource allocation and the competitive landscape in the AI industry. Smaller AI companies, such as xAI, often operate with fewer resources compared to tech giants like Google and OpenAI. This resource disparity suggests a potential power concentration that could inhibit wider innovation and competition. Indeed, those companies with more extensive resources can afford to dominate the market, leading to ethical concerns regarding the democratization of AI technology and potential monopolistic practices.
Finally, this trend of delays and re-evaluation could prompt a shift in public and industry expectations. The continued postponements force both developers and the general public to reassess the timelines and promises associated with AI advancements. Public discourse may increasingly focus on ethical considerations and the balance between rapid AI advancements and safety. This could lead to a more cautious approach to AI development, prioritizing robustness and ethical usage over mere capability expansion.
Learn to use AI like a Pro
Get the latest AI workflows to boost your productivity and business performance, delivered weekly by expert consultants. Enjoy step-by-step guides, weekly Q&A sessions, and full access to our AI workflow archive.
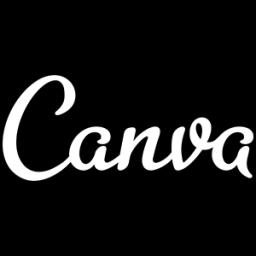
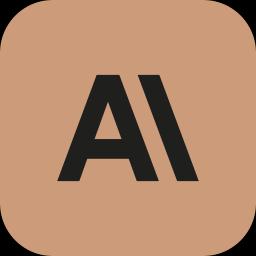
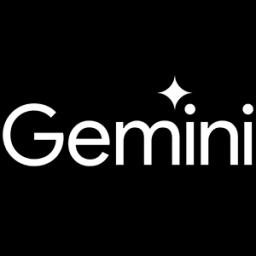
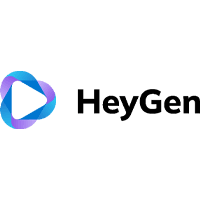
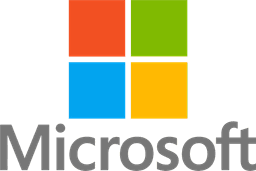
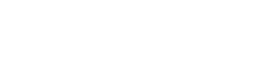
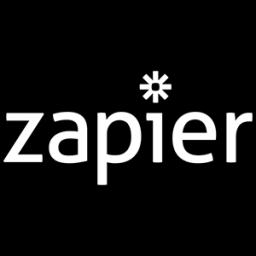
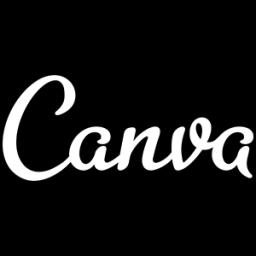
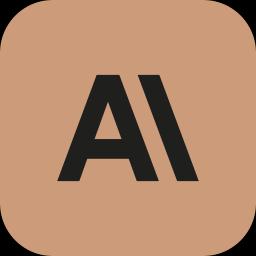
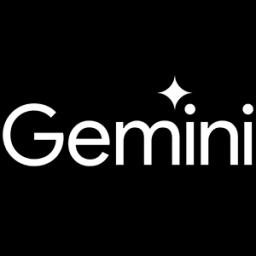
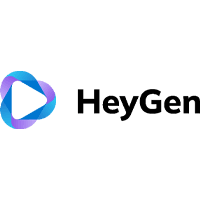
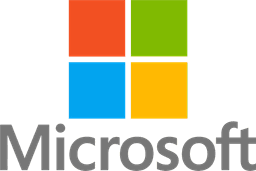
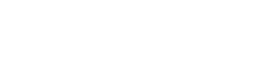
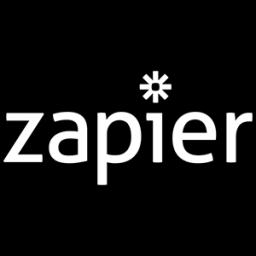
Expert Opinions on AI Trends
The recent delay of xAI's much-anticipated Grok 3 model underscores a broader trend of missed deadlines that have become all too common in the AI industry. This delay is not an isolated instance but rather part of a pattern that includes setbacks for other hyped models like Anthropic's Claude 3.5 Opus. xAI, led by Elon Musk, had positioned Grok 3 as a groundbreaking advancement, trained on an enormous GPU cluster. However, as the tech world awaited its release, the delay has left many questioning the scalability and feasibility of current AI training methods and the challenges in achieving the promised performance gains.
The delay in Grok 3's launch can be attributed to several critical factors that have become evident across the AI landscape. One prominent issue is the overarching trend of AI models missing their proposed timelines, largely due to the inherent challenges of scaling AI technologies effectively with current methodologies. These challenges are exacerbated for companies like xAI that operate with smaller teams and resources compared to giants such as Google and OpenAI. The ambitious goals set forth by Musk and his team have, in this instance, proven to be overly optimistic.
When evaluating Grok 3 against other prevailing AI models, it becomes apparent that xAI aimed to position this model as a serious contender against the likes of OpenAI's GPT-4 and Google's Gemini. Grok 3 is designed for a multitude of applications, from image analysis to question-answering and integration with platforms like X (formerly Twitter). The model is expected to outstrip its predecessors significantly, contributing to the competitive AI market landscape.
The concept of AI scaling laws plays a pivotal role in understanding the current challenges faced by companies like xAI. These laws articulate the relationship between model size—encompassing parameters, data, and compute power—and the subsequent performance improvements that can be expected. Historically, enlarging models has led to clear gains, but recent patterns suggest diminishing returns, creating obstacles in AI development and contributing to delays such as Grok 3's.
Despite the challenges and delays, xAI has hinted at the potential release of an interim model, Grok 2.5, which is suggested to be "Our most intelligent model" to date. This intermediate step reflects a strategic pivot by xAI to maintain momentum amidst uncertain development timelines for Grok 3. While no official release dates have been confirmed for either model, the anticipation for Grok 2.5 provides a glimmer of progress in the interim.
The postponement of major AI model releases, including Grok 3, indicates a potential deceleration in AI advancements using traditional development paths. This apparent slowdown punctuates the need for innovative techniques to circumvent current scaling law limitations and underscores the complexity of perpetually advancing AI capabilities. The AI field must now focus on revolutionary architectural designs and training methodologies to continue driving forward progress in the long-term.
Learn to use AI like a Pro
Get the latest AI workflows to boost your productivity and business performance, delivered weekly by expert consultants. Enjoy step-by-step guides, weekly Q&A sessions, and full access to our AI workflow archive.
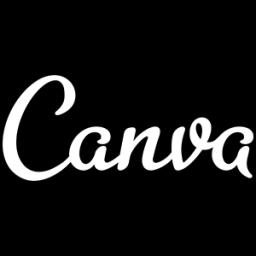
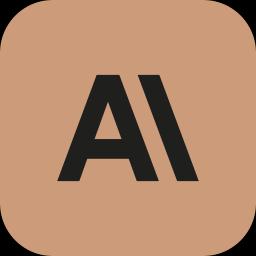
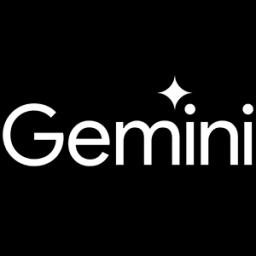
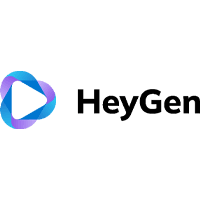
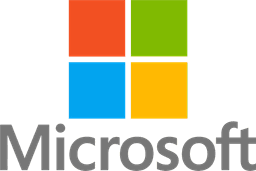
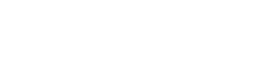
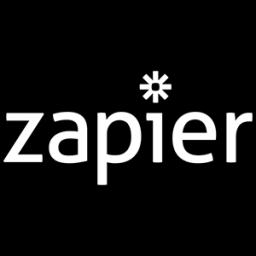
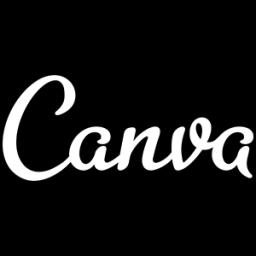
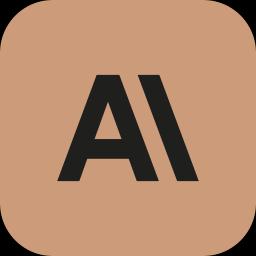
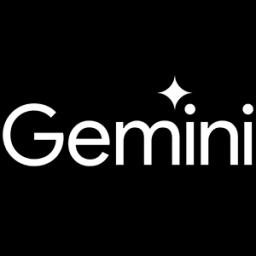
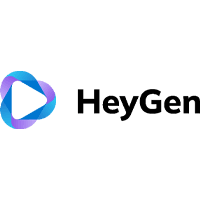
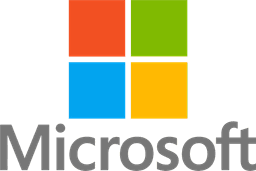
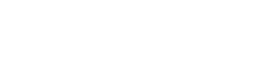
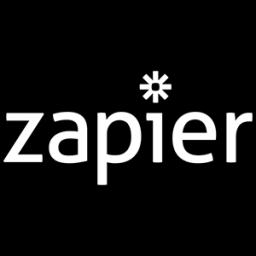
Public Reaction to the Grok 3 Delay
The delay in the release of xAI's Grok 3 model has sparked a significant public reaction, highlighting widespread disappointment and frustration in the tech community. Many observers see this delay as part of a broader pattern of missed deadlines within the AI industry, contributing to growing skepticism about the ambitious timelines often set by such companies, particularly those associated with high-profile figures like Elon Musk.
Public discourse has increasingly focused on the limitations of current AI scaling laws, which may have played a role in delaying Grok 3. These discussions are bringing attention to the various challenges AI developers face in enhancing performance while managing resource constraints.
The idea of an interim release, Grok 2.5, has been met with mixed reactions. While some view this as a practical solution, providing a stopgap until Grok 3 is ready, others see it as an insufficient measure that may not address underlying technological hurdles. The skepticism extends to broader concerns about the reliability of AI companies' promises and a push for more realistic expectations regarding future advancements.
Future Directions for AI Research and Development
The delay of xAI's Grok 3 model, which was highly anticipated and promoted as a breakthrough in AI technology, marks a significant turning point in the AI research and development landscape. Such delays are not isolated incidents but reflect a broader trend within the industry, marked by high-profile projects from other major AI labs such as Anthropic's Claude 3.5. This indicates deeper challenges inherent in scaling AI models according to traditional expectations. As confirmed by experts, the scaling laws that once led to predictable and straightforward performance improvements are now showing signs of diminishing returns, necessitating innovative approaches and methods to push the capabilities of AI further.
Historically, AI advancements have closely followed a strategy of scaling up – increasing the size of models, using more data, and leveraging more computational power to enhance capabilities. However, the Grok 3 delay underscores the realization that simply making models larger may no longer result in proportionate improvements in performance. This realization has spurred significant reflection within the AI community about future directions, emphasizing quality over quantity, and seeking novel architectures and training methodologies distinct from the current norm.
A critical implication of these delays is the shift in focus from sheer model size towards efficiency and specialization. For instance, Meta's recent pivot towards developing smaller, task-specific models illustrates a growing recognition of the need for more targeted solutions. Meanwhile, Google's success with PaLM 2, where a smaller model has outperformed larger predecessors, reinforces this shift in strategic thinking. Such industry shifts indicate a potential move away from general-purpose monolithic models to more nuanced, application-specific AI applications tailored to meet specific needs effectively.
Learn to use AI like a Pro
Get the latest AI workflows to boost your productivity and business performance, delivered weekly by expert consultants. Enjoy step-by-step guides, weekly Q&A sessions, and full access to our AI workflow archive.
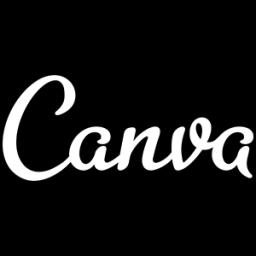
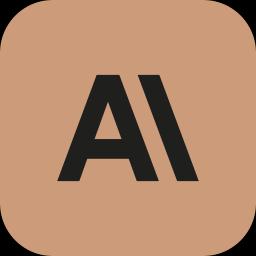
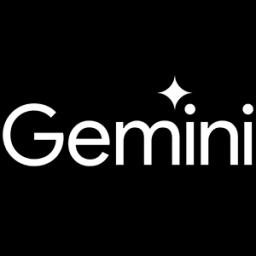
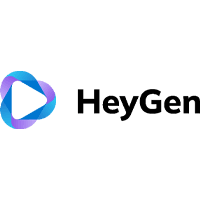
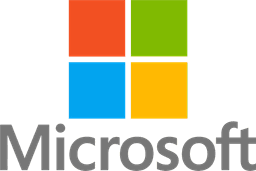
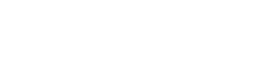
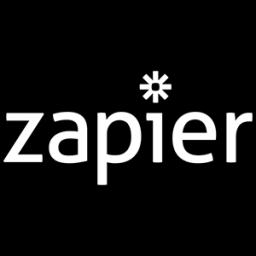
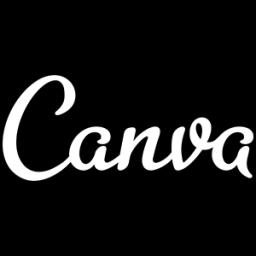
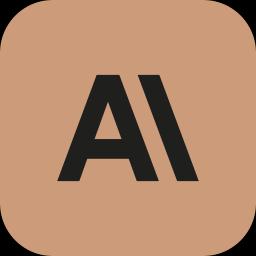
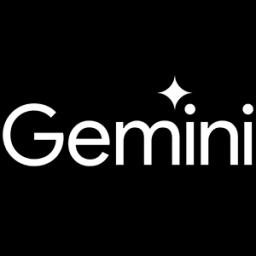
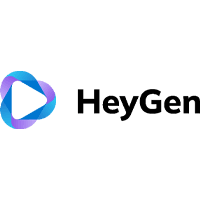
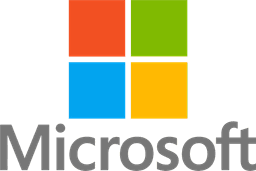
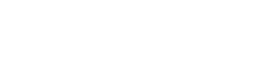
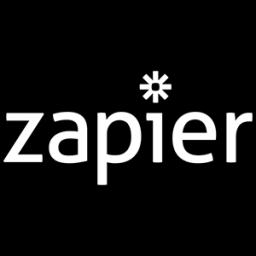
The delay also accentuates resource disparities within the industry. Smaller firms like xAI, despite having promising technologies, struggle to compete against giants with more extensive resources. According to ethics researcher Dr. Timnit Gebru, this imbalance raises concerns about democratization and innovation within AI, as fewer entities control more advanced AI capabilities. This trend could potentially stifle innovation and centralize AI technology development, which highlights a critical area for regulatory and policy intervention to ensure fair competition and innovation.
AI research's future direction seems poised to focus more intensely on overcoming the limitations observed in scaling laws. This includes a concerted push towards developing more economically feasible models that do not rely on sheer size to achieve performance gains. Additionally, the heightened emphasis on AI safety and ethics, as advocated by leaders like DeepMind and industry experts, points towards a more responsible and secure approach to AI development that balances capability expansion with safety and ethical considerations.