Claude May Cost Enterprises More Than They Bargained For
Hidden Costs of Anthropic's Claude AI Models Uncovered
Last updated:

Edited By
Mackenzie Ferguson
AI Tools Researcher & Implementation Consultant
Discover how Anthropic's Claude AI models could be 20-30% more expensive than OpenAI's GPT models for businesses, despite having a lower per-token cost. The unexpected cost hike is due to Claude generating significantly more tokens per input, impacting enterprises' budgets and AI deployment strategies.
Introduction to AI Deployment Costs
The deployment cost of Artificial Intelligence (AI) solutions is a critical consideration for enterprises looking to leverage advanced technologies for improved operational efficiency and innovation. In recent discussions, the comparison between Anthropic's Claude AI models and OpenAI's GPT models has highlighted significant financial implications, with Claude models appearing 20-30% more expensive despite their lower per-token cost. This counterintuitive pricing dynamic largely results from Claude's tokenizer, which generates more tokens per input than GPT, thus consuming more resources and increasing costs. This inefficiency is particularly evident in certain content types, such as programming code and structured data, where Claude's token count can be substantially higher than GPT's, as per the analysis shared by VentureBeat.
Understanding these deployment costs is essential for decision-makers. The perceived advantages of lower token prices with AI models like Claude can be misleading if the overall cost multiplies due to inefficiencies in token generation. This highlights the importance of evaluating both cost structures and technical performance when selecting AI solutions for enterprise needs. Enterprises must weigh whether the costs associated with additional tokens outweigh the benefits these models offer. Additionally, the broader implications for the AI market, such as potential shifts in competitive dynamics and technology adoption rates, are influenced by how these costs are managed, as underscored by industry observations like those on VentureBeat.
Learn to use AI like a Pro
Get the latest AI workflows to boost your productivity and business performance, delivered weekly by expert consultants. Enjoy step-by-step guides, weekly Q&A sessions, and full access to our AI workflow archive.
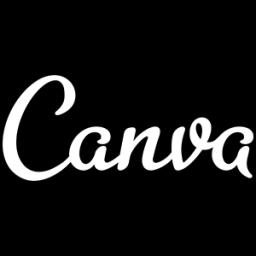
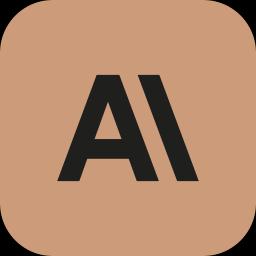
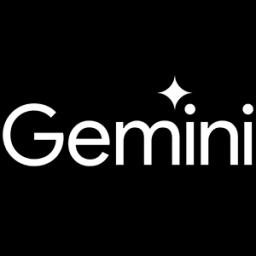
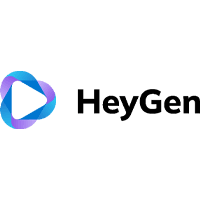
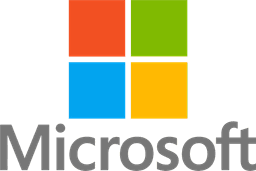
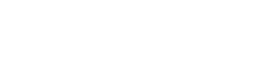
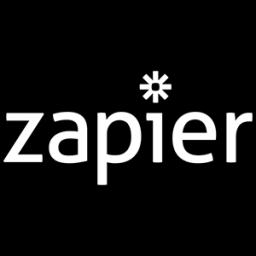
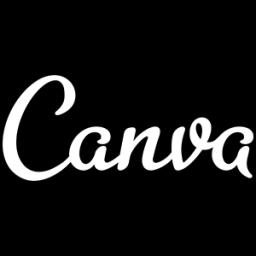
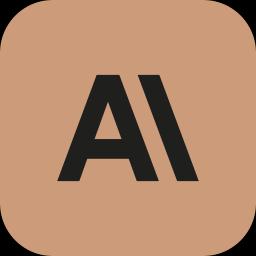
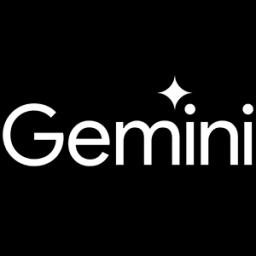
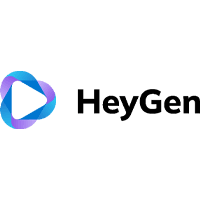
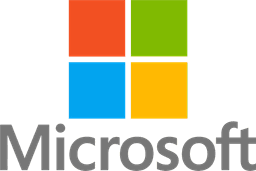
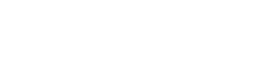
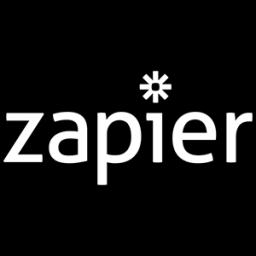
Comparative Analysis of Claude and GPT Models
When it comes to evaluating the relative costs of Claude and GPT models, a deeper dive into their tokenizer efficiency unveils significant differences. Although Claude models initially appear more economical due to their lower per-token price, a hidden cost emerges from their tokenizer's inefficiency. This tokenizer, as reported by VentureBeat, generates a higher number of tokens than OpenAI's GPT for the same input. As a result, enterprises can witness an overall cost increase of 20-30% when using Claude, depending on the data type. This increased expenditure is particularly pronounced for structured content like Python code, where Claude's tokenizer adds around 30% more tokens compared to GPT, a critical factor for businesses aiming to manage AI operational costs efficiently.
The discussion surrounding model performance offers a nuanced view of the Claude versus GPT debate. According to AppyPie Automate, Claude 3.5 Sonnet excels in specific areas such as knowledge and reasoning, potentially offering significant advantages in tasks like handling graduate-level problem sets and mathematical challenges. Nonetheless, GPT-4 remains a formidable competitor, especially in the domain of coding. This juxtaposition illustrates a trade-off scenario for users and enterprises who must weigh Claude's superior performance in certain areas against the cost-effectiveness of GPT models.
Pricing and accessibility are key considerations for businesses choosing between Claude and GPT models. Both models offer competitive pricing structures with free and premium plans that include access to more sophisticated features for a monthly fee of roughly $20. However, as Writesonic notes, Claude's API pricing could be misleadingly attractive, should organizations overlook the impact of its higher token generation on overall costs. Therefore, for large-scale applications, while Claude's nominal per-token cost is lower, enterprises need to factor in potential upcharges linked to token counts, especially when processing diverse and complex datasets.
The public's response to the comparison of Claude and GPT models is diverse, as highlighted in various discussions online, including platforms like Reddit. While many users appreciate Claude's human-like writing style and accuracy, concerns about its higher tokenization costs cannot be ignored, particularly for substantial deployments within corporate settings. As articulated in VentureBeat, this inefficiency where Claude's models generate significantly more tokens can make them 20-30% more expensive compared to GPT models, sparking debate over their cost-effectiveness.
Learn to use AI like a Pro
Get the latest AI workflows to boost your productivity and business performance, delivered weekly by expert consultants. Enjoy step-by-step guides, weekly Q&A sessions, and full access to our AI workflow archive.
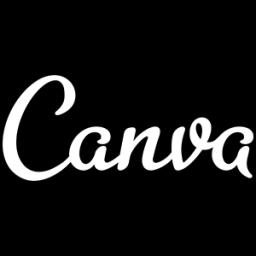
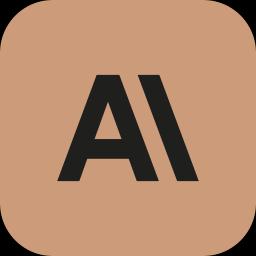
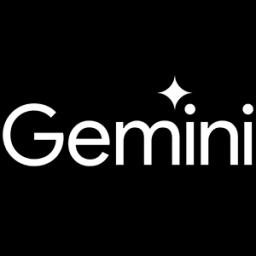
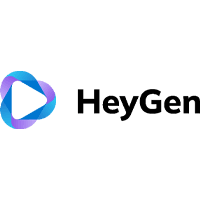
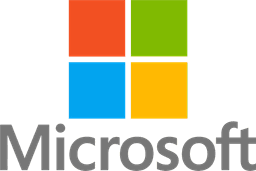
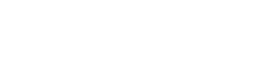
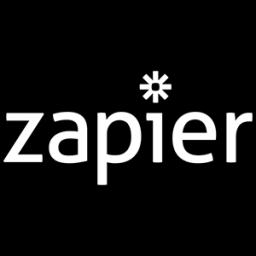
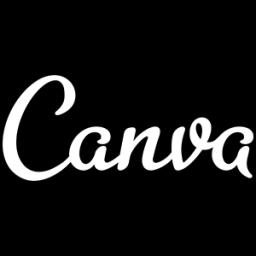
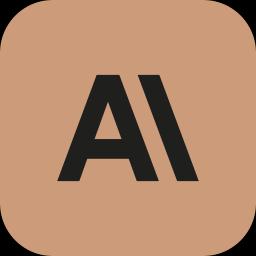
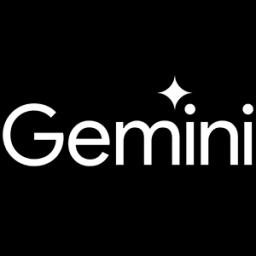
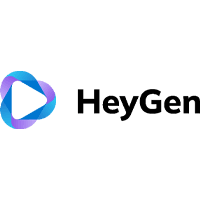
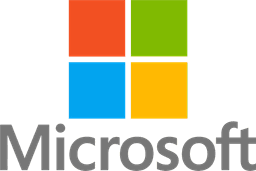
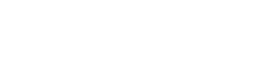
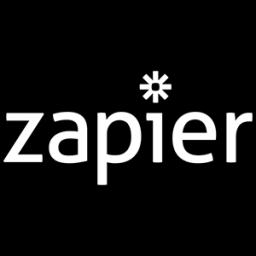
Looking ahead, the economic and social implications of choosing between Claude and GPT models are vast. If Claude continues to incur higher costs, it could potentially lose its competitive edge, paving the way for GPT models to gain a larger market share, as noted in various analyses like those by Vantage. Such a shift might affect investor decisions, lead to pricing reevaluation, and encourage technological innovations at Anthropic to improve tokenizer efficiency. Socially, the preference for more cost-effective AI could democratize access to AI capabilities, fostering a broader deployment across smaller enterprises and individual users, albeit it may also necessitate initiatives to address potential job displacements from increased automation.
Understanding Tokenizer Inefficiency
Tokenizer inefficiency is a critical consideration when evaluating the cost-effectiveness of AI models, such as Anthropic's Claude and OpenAI's GPT. While Claude AI models boast a lower per-token cost, this advantage is overshadowed by the inefficiency of its tokenizer. Unlike OpenAI's tokenizer, which utilizes Byte Pair Encoding (BPE) for a balanced approach to token generation, Claude's tokenizer produces significantly more tokens for the same input. This results in Claude models being 20-30% more expensive overall in enterprise settings, as detailed in a recent analysis from VentureBeat [].
This tokenizer inefficiency has ramifications for how Claude and GPT models utilize their context windows. Despite Claude’s larger advertised context window, which can handle up to 200K tokens, its verbosity in token generation quickly fills up the available space. In comparison, GPT's more efficient tokenization process ensures that its smaller context window remains effectively utilized. This situation could lead enterprises to question the real-world advantages of Claude’s purportedly larger context window if it can't be fully leveraged [].
Content type further exacerbates Claude's token generation issues. Structured content, like programming code, amplifies the inefficiency, with Python code processing resulting in 30% more tokens generated by Claude than GPT. In less structured text, such as English articles, Claude still trails, producing roughly 16% more tokens than its counterpart. Such variations underline the necessity for potential users to consider the specific applications and content types before deciding on an AI model, as costs and efficiencies can differ notably between Claude and GPT [].
In a competitive AI landscape, the inefficiency seen in Claude's tokenizer might not only increase direct deployment costs but also subtly influence strategic decisions within enterprises. Constructively, this could spur innovations in tokenizer technology and a push towards more efficient processing methods. However, if unresolved, the inability to capitalize on lower per-token costs due to higher overall expenses could see enterprises gravitate towards GPT models, influencing market share dynamics and potentially shaping future development priorities for both Anthropic and OpenAI [].
Cost Implications for Enterprises
The cost implications of deploying AI in enterprise settings have become increasingly critical as companies strive to harness the power of advanced technologies while maintaining fiscal responsibility. When evaluating AI models like Anthropic's Claude and OpenAI's GPT, enterprises must consider not only the sticker price but also the hidden costs associated with their use. According to a detailed analysis, even though Claude's per-token cost appears lower, its practical cost rises by 20-30% compared to GPT when deployed at scale. This is largely due to Claude's tokenizer, which significantly increases the total number of tokens processed for the same input, thereby inflating the overall expenditure (source).
Learn to use AI like a Pro
Get the latest AI workflows to boost your productivity and business performance, delivered weekly by expert consultants. Enjoy step-by-step guides, weekly Q&A sessions, and full access to our AI workflow archive.
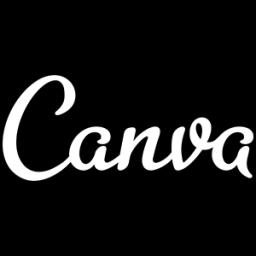
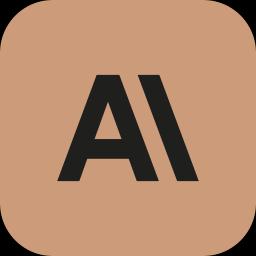
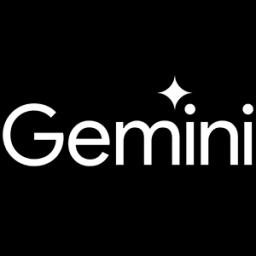
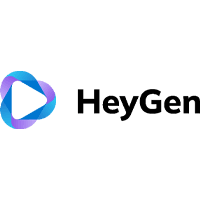
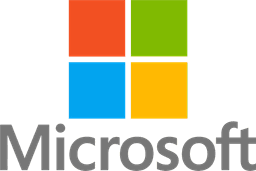
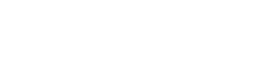
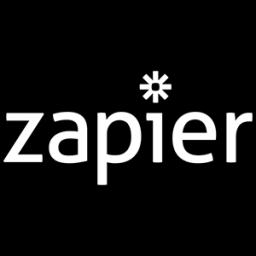
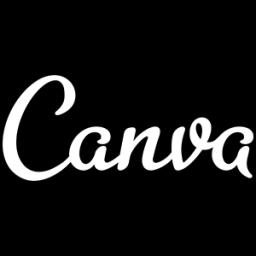
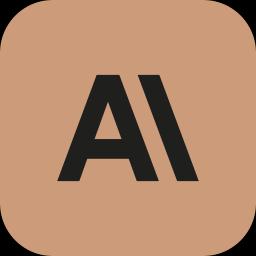
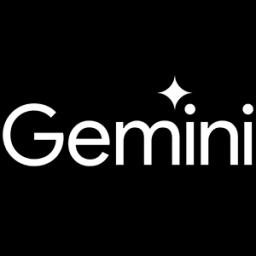
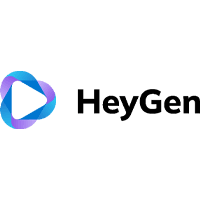
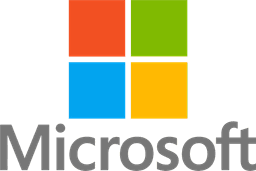
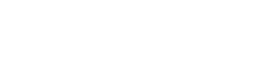
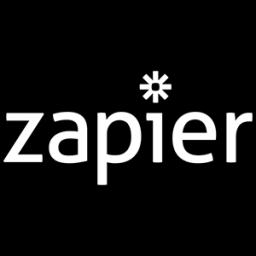
The tokenizer inefficiency inherent in Claude's design can have profound effects on a company's budget, particularly in fields requiring extensive data processing, such as tech industries or firms dealing with large volumes of programming code. For instance, when handling Python scripts, Claude's token overhead can be 30% greater than that of GPT, leading to unexpected budgetary overruns. In contrast, for natural language processing tasks like English text, the increase is about 16%, highlighting how varying content types influence costs (source). This variability necessitates a detailed cost-benefit analysis when selecting an AI model, to ensure that the chosen solution aligns with specific financial and operational goals.
Additionally, beyond just the tokenization costs, enterprises must consider other financial impacts such as the reduced usable context window when using Claude models. Though Claude advertises a large context window, its verbose tokenizer effectively reduces the practical size available for operations, potentially necessitating more frequent input restarts and increasing computation times and associated costs. Such hidden expenses can erode the apparent price advantage of Claude's lower per-token algorithm. Enterprises need to weigh these factors carefully against other advantages that Claude might offer, such as enhanced reasoning capabilities in certain tasks (source).
In conclusion, while Claude presents certain benefits in specific areas, the economic implications of its deployment can potentially outweigh its technical advantages in enterprises focused on cost-efficiency. As the AI landscape continues to evolve, companies will need to remain agile, updating their AI strategies to control costs while maximizing utility. This dynamic might eventually drive Anthropic to refine its models further, aiming to minimize token overhead and enhance cost-effectiveness, or perhaps adopt an alternative pricing strategy to remain competitive against robust alternatives like GPT (source).
Examining Context Window Utilization
In exploring how context window utilization plays a crucial role in AI deployment, especially when comparing models like Anthropic's Claude and OpenAI's GPT, it becomes evident that even small inefficiencies can lead to substantial financial impacts. The use of a context window refers to the maximum limit of tokens that can be input into a model at any given time. Claude's models, while advertised as having a larger context window of up to 200K tokens, suffer from a drawback due to its verbose tokenizer. This results in more tokens being generated for the same input, ultimately diminishing the effective space available within the context window, as opposed to GPT's more economical tokenization. Consequently, such inefficiencies directly affect the user experience and cost-effectiveness, making it imperative for enterprises to choose models that offer streamlined token usage .
Evaluating context window utilization's implications provides insights into the strategic deployment of AI models in enterprise settings. Despite Claude's lower per-token cost, the inefficiency in its token generation means enterprises could end up paying 20-30% more overall because more tokens are consumed rapidly within any given task. This rapid consumption can restrict the amount of useful data that can be processed at once, thereby nullifying the potential benefits of a larger context window. Efficient use of a context window is crucial, as it dictates how well a model can manage complex tasks that require understanding large blocks of text or data .
The impact of tokenizer inefficiency highlights the importance of understanding both the advertised and practical capabilities of AI models. While Claude may initially appear to offer more value with its larger context window and lower per-token cost, the necessity to process additional tokens quickly offsets these benefits. This means that while enterprises might be drawn to the appealing specifications on paper, real-world application could reveal cost increases and less efficient performance. Thus, understanding context window utilization can inform better decision-making, ensuring that businesses align their choice of AI models with their operational needs and fiscal parameters .
Learn to use AI like a Pro
Get the latest AI workflows to boost your productivity and business performance, delivered weekly by expert consultants. Enjoy step-by-step guides, weekly Q&A sessions, and full access to our AI workflow archive.
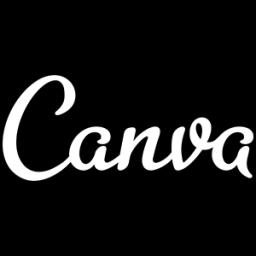
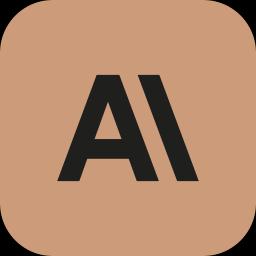
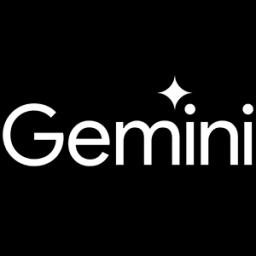
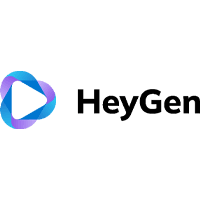
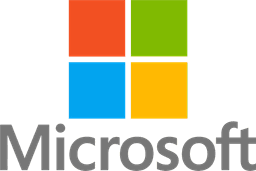
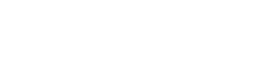
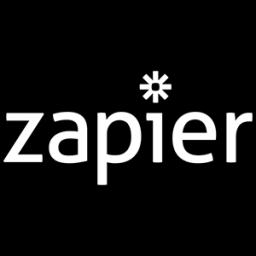
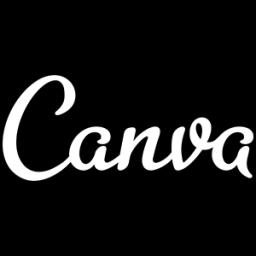
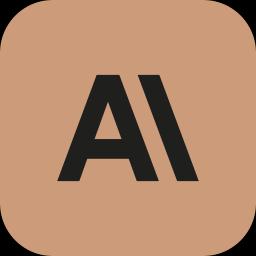
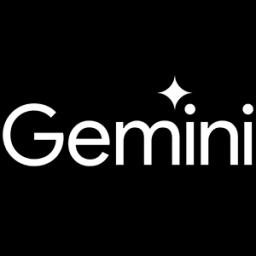
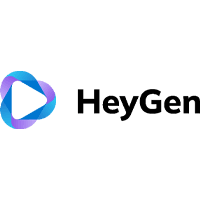
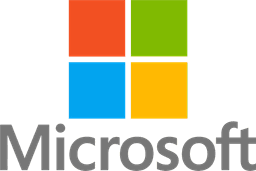
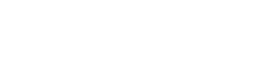
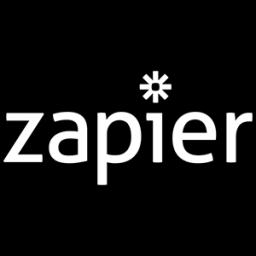
Expert Opinions on AI Model Pricing
In the ever-evolving landscape of artificial intelligence, pricing models of AI systems have become a focal point of discussion among experts. A notable dialogue has emerged around the cost-effectiveness of different AI models, particularly between Anthropic's Claude and OpenAI's GPT models. Experts have pointed out that, despite Claude boasting a 40% lower per-token cost than GPT, its overall expenses can be 20-30% higher due to inefficiencies in tokenization. These inefficiencies cause Claude to generate more tokens per input, effectively increasing the cost despite the lower price per token. This characteristic has prompted experts to explore the broader implications of tokenization on AI deployment costs, particularly in enterprise settings where efficiency and cost management are critical. VentureBeat highlights this concern, emphasizing the need for a balance between tokenization efficiency and pricing strategy in future AI model deployments.
A distinct expert perspective that adds to the discourse on AI model pricing comes from a Vantage analysis, which presents seemingly contradictory findings. While some analyses spell out Claude's tendency towards higher costs due to its verbose tokenizer, Vantage offers a different outlook. They assert Claude as being cheaper per input token than GPT-4, quoting an impressive 95% less expensive rate. However, the analysis does not tackle the token inefficiency issue raised by other reports. This discrepancy underscores the complexity and variability in pricing models for AI deployments and the necessity for comprehensive evaluations, taking into account differences in model versions and methodologies as reasons for cost variation. Experts agree that while Claude's model presents competitive token pricing, a more nuanced understanding of tokenization and content type influence is crucial to truly grasping its economic impact. Vantage provides a different context to contemplate these financial dynamics.
Moreover, experts contend that the efficiency of token utilization plays a pivotal role in determining the practical cost of using AI models. While Anthropic claims a larger context window of 200K tokens, this perceived advantage is mitigated by the inefficient use of those tokens due to a tendency towards verbosity. This can neutralize the benefits of having a larger context window, effectively equating or reducing it in operational contexts compared to OpenAI's 128K tokens. Considering the divergent findings and perspectives, it is evident that experts encourage both users and developers to prioritize strategic analysis of token efficiency when adopting AI models to ensure cost-effectiveness and optimal performance across different use case scenarios. As the AI technology continues to advance, maintaining transparency in pricing strategies and tokenization techniques will remain fundamental to fostering informed decisions and trust in AI applications.
Public Reactions to AI Cost Analysis
Public reactions to the cost analysis of AI models like Anthropic's Claude and OpenAI's GPT have been mixed, reflecting varying perspectives on value versus cost-efficiency. Many users express concerns over Claude's higher overall cost despite its lower per-token pricing. These concerns are highlighted by the tokenizer inefficiency noted in the VentureBeat article, which discusses how Claude's tendency to generate more tokens can increase costs by 20-30% more than GPT. This revelation has raised alarm, particularly in enterprise settings where budget constraints are critical, prompting some users to reconsider the cost-effectiveness of deploying Claude over less expensive alternatives.
In online forums such as Reddit and the Effective Altruism Forum, there are debates about the efficiency of Claude compared to GPT. While Claude receives praise for its accuracy and human-like writing style, there is significant discussion regarding whether these benefits justify the higher cost reported in the VentureBeat article. This dialogue underscores a broader public sentiment that more transparency is needed concerning how Claude's tokenizer works. Transparency could enable more informed decision-making for businesses analyzing the overall value of investing in Claude for specific use cases.
Users who prioritize cost-saving measures in their operations have expressed that the higher token count generated by Claude's tokenizer—leading to a faster filling of its context window—can negate the apparent benefits of its lower per-token cost. This factor could potentially narrow the choice towards GPT models, which might offer more predictable and manageable expenses. These discussions are particularly relevant in fields demanding large-scale text processing, where cost calculations significantly influence AI model adoption.
Learn to use AI like a Pro
Get the latest AI workflows to boost your productivity and business performance, delivered weekly by expert consultants. Enjoy step-by-step guides, weekly Q&A sessions, and full access to our AI workflow archive.
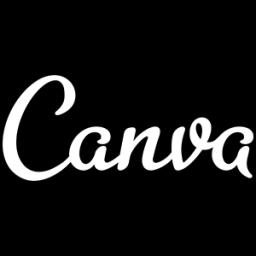
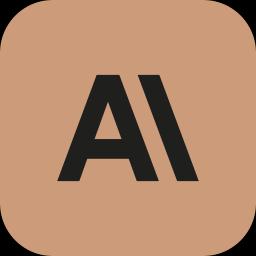
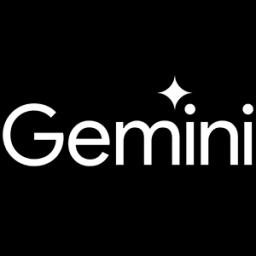
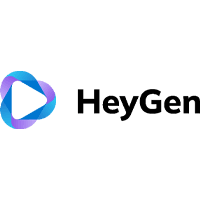
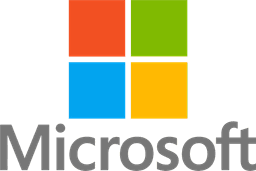
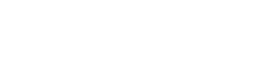
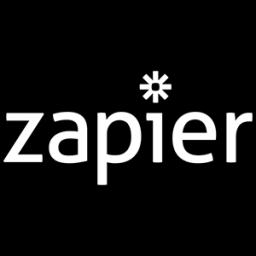
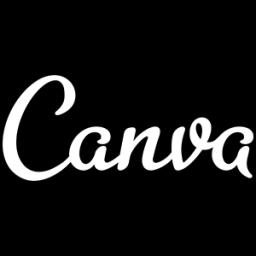
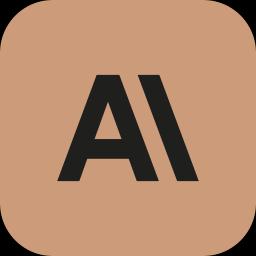
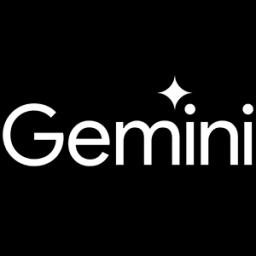
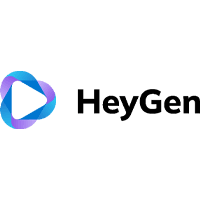
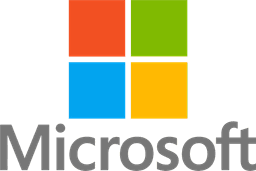
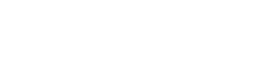
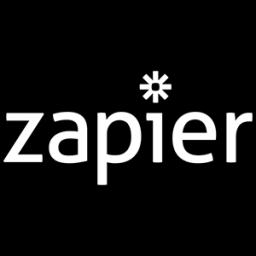
The discourse surrounding AI cost analysis is not merely about the financial implications but also involves considerations of performance and contextual application. Many in the AI community argue that enterprises must weigh these costs against potential gains in computational efficiency or product output. As noted in the VentureBeat discussion, some users are willing to pay a premium for Claude if it results in superior outcomes in areas like content generation and creative tasks, where Claude's strengths are more pronounced.
Future Implications of AI Cost Structures
As artificial intelligence continues to evolve, the cost structures associated with its deployment will significantly shape its future implications across various sectors. One crucial aspect influencing these costs is the efficiency of tokenization processes used by different AI models. VentureBeat highlights that although Anthropic's Claude models advertise a lower per-token price compared to OpenAI's GPT, the overall costs can be deceptively higher due to what is termed "tokenizer inefficiency". This inefficiency, wherein Claude generates more tokens per input than GPT, not only affects the economic feasibility of its enterprise deployment but also the overall effectiveness of its application in real-world scenarios.
From an economic perspective, should Claude models remain more costly, OpenAI's GPT might gain an upper hand in the AI marketplace. This dominance could potentially freeze the competitive landscape, slowing innovation and reducing incentives for investing in alternative technologies like Claude. Enterprises evaluating AI solutions might gravitate towards the more cost-effective GPT models, even at the expense of certain performance benefits that Claude might offer. This cost discrepancy could necessitate Anthropic to either enhance the efficiency of its tokenizers or to reconfigure its pricing models to maintain market relevance.
Socially, the broader accessibility of more affordable AI models like GPT can democratize technology use, allowing smaller enterprises and individuals to harness AI capabilities previously exclusive to larger corporations with ample budgets. This shift can potentially mitigate economic disparities by opening new avenues for innovation and entrepreneurship. However, the same affordability that enables wider access to AI could also expedite automation, leading to job displacement, thus requiring proactive policies to retrain the workforce and integrate these technologies into society constructively.
Politically, notable cost differences between AI models could invite regulatory attention to ensure a balanced competitive environment. Policymakers might steer focus towards funding the development and deployment of cost-effective AI technologies to enhance technological sovereignty and boost national competitiveness. The interplay of pricing dynamics and technological efficiency will likely be pivotal in shaping not just market trends but also in determining which countries emerge as leaders in AI innovation.