A New Era or Just a Quick Fix?
Inference-Time Search: A Game-Changer or Temporary Hack in AI Scaling?
Last updated:

Edited By
Mackenzie Ferguson
AI Tools Researcher & Implementation Consultant
Researchers have developed 'inference-time search,' a novel method purported to scale AI models by creating multiple potential answers and selecting the best one. It promises enhanced performance for AI models like Google's Gemini 1.5 Pro, yet experts view it as a temporary fix for current model limitations instead of a genuine advancement.
Introduction to Inference-Time Search
The anticipated implications of inference-time search are extensive, impacting various sectors. Economically, more efficient AI models could lead to productivity boosts and cost reduction across industries. Socially, increased AI efficiency might democratize technology access, though it could also necessitate reskilling as certain job roles evolve or become obsolete. Moreover, such advancements are likely to heighten global competition, prompting new government regulations and policies focused on managing AI's multifaceted impacts, from economic to ethical considerations .
How Inference-Time Search Works
Inference-time search represents an innovative approach to enhancing the capabilities of AI models by addressing one of their core challenges: the generation of reliable outputs. Originating from recent research, this method involves AI models generating a multitude of potential answers to a single query and subsequently selecting the one deemed most accurate or 'best.' This process not only capitalizes on the AI's existing capabilities but also introduces an element of self-verification, where the model evaluates its own outputs. The adaptability of inference-time search becomes apparent as models scale; with increased size and complexity, the efficacy of this self-verification process seems to improve, suggesting a scalable solution for existing AI challenges. You can explore more about this method in a detailed article on TechCrunch.
Learn to use AI like a Pro
Get the latest AI workflows to boost your productivity and business performance, delivered weekly by expert consultants. Enjoy step-by-step guides, weekly Q&A sessions, and full access to our AI workflow archive.
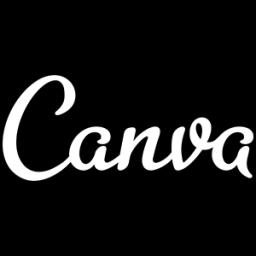
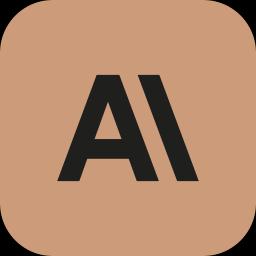
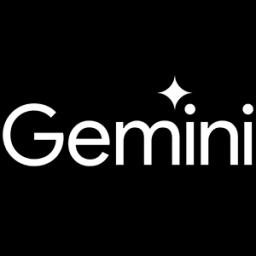
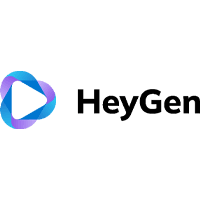
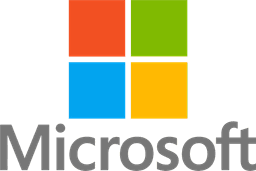
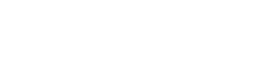
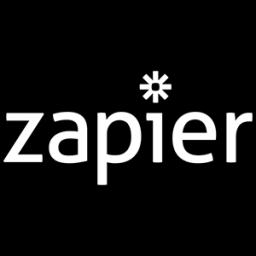
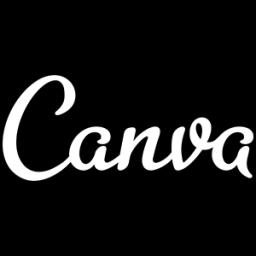
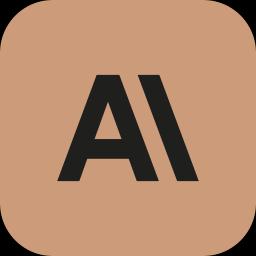
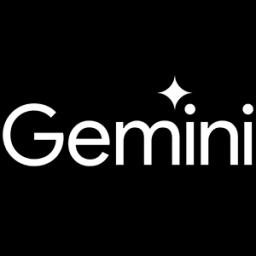
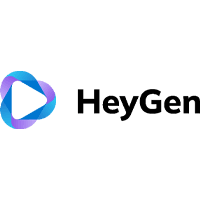
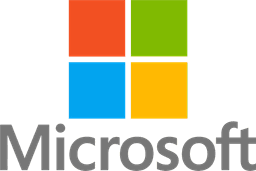
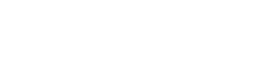
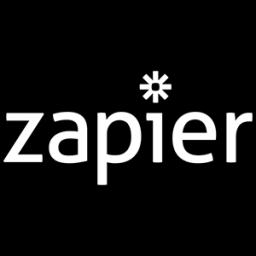
While inference-time search holds promise, it is met with a degree of skepticism among experts. Some consider it more of a stopgap or workaround than a genuine leap forward in advancing AI capabilities. The primary concern lies in its efficacy: the method presupposes the existence of a robust mechanism to determine the 'best' answer, which isn't always present in real-world scenarios. This limitation has led to the perception that while it does improve the reliability of outputs in controlled environments, it does not fundamentally enhance the reasoning abilities of the AI itself. These nuances invite ongoing debate within the AI community, as discussed further in this article on TechCrunch.
The introduction of inference-time search is significant for the AI industry, particularly concerning the compute-efficient scaling of reasoning models. Traditional AI scaling has heavily relied on increasing data and computational resources, but this method offers a potentially more cost-effective strategy. The ongoing discourse reflects the balance AI researchers are trying to strike between achieving improved performance metrics and confronting the inherent limitations of current AI models. As AI strives for greater integration into diverse industry sectors, methods like inference-time search could pave the way for more versatile and adaptable applications, despite their current constraints. More details on these implications can be found in a detailed review on TechCrunch.
Expert Skepticism and Concerns
Despite the promise of a new AI scaling method dubbed "inference-time search," the approach has been met with caution and skepticism by experts in the field. While the technique, which allows an AI model to generate multiple potential answers and choose the best one, reportedly improves performance in systems such as Google’s Gemini 1.5 Pro, experts warn that this method might not represent a genuine improvement in AI's reasoning abilities. Instead, they view it as a temporary workaround to circumvent the current limitations of AI models, rather than addressing fundamental issues in reasoning capabilities (TechCrunch).
The skepticism surrounding "inference-time search" is largely due to its reliance on predefined evaluation criteria to select the best answer. Researchers and experts argue that many real-world scenarios lack such clear-cut criteria, rendering this method less effective in practical applications. Matthew Guzdial, an AI researcher at the University of Alberta, highlights that while the potential for self-verification becomes more efficient at scale, the success of this scaling method is contingent upon the availability of reliable evaluation metrics, which may not always be feasible (TechCrunch).
Learn to use AI like a Pro
Get the latest AI workflows to boost your productivity and business performance, delivered weekly by expert consultants. Enjoy step-by-step guides, weekly Q&A sessions, and full access to our AI workflow archive.
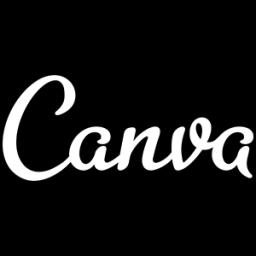
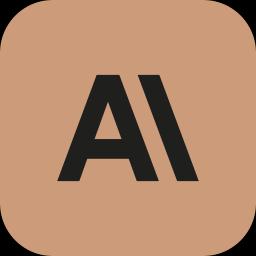
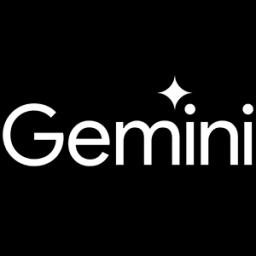
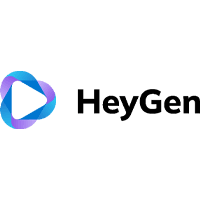
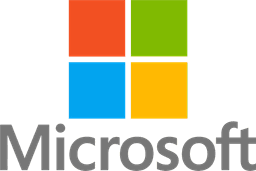
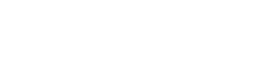
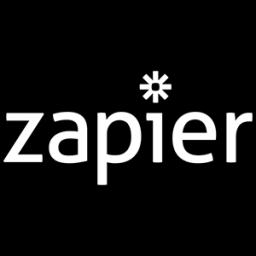
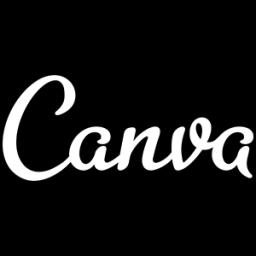
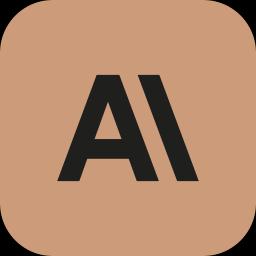
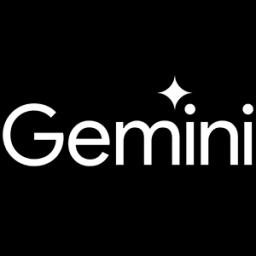
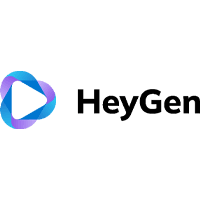
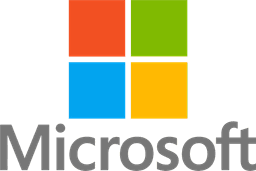
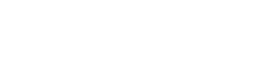
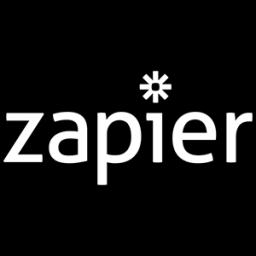
Additionally, experts like Mike Cook from King’s College London point out that "inference-time search" may not fundamentally advance an AI model's reasoning skills. Instead, it serves more as a patch to improve reliability by compensating for errors inherent in existing models. This perception poses a significant concern for the AI industry, which is eager to find more compute-efficient ways to scale up models that require robust reasoning capabilities. Consequently, the search for genuine advancements continues, as the industry grapples with ensuring computational efficiency and innovation in large AI systems (TechCrunch).
Understanding AI Scaling Laws
The concept of AI scaling laws plays a crucial role in understanding the development and optimization of artificial intelligence models. These laws essentially describe how AI model performance tends to improve with the increase in training data and computational resources. Traditionally, pre-training scaling was considered the primary approach to enhancing AI capabilities. This involved increasing the size of the datasets used to initially train the models, thereby improving their understanding and generalization abilities.
However, as AI research has progressed, diverse scaling methodologies have emerged. Two significant recent developments are post-training scaling and test-time scaling. Post-training scaling refers to adjustments made to the AI models after they have been trained, aiming to enhance their performance without the need for extensive retraining. Test-time scaling involves adapting models in real-time as they process information, allowing for dynamic adjustments that could improve decision-making and execution efficiency.
One intriguing approach discussed in recent research is the "inference-time search." As detailed in a [TechCrunch article](https://techcrunch.com/2025/03/19/researchers-say-theyve-discovered-a-new-method-of-scaling-up-ai-but-theres-reason-to-be-skeptical/), this method involves AI models generating a variety of possible answers to a query and then selecting the most suitable one among them. While it has shown promise in enhancing the performance of models like Google's Gemini 1.5 Pro, experts warn that this should not be mistaken for a genuine improvement in AI's reasoning capabilities. Many see it as a workaround to bypass current model limitations, rather than a fundamental improvement.
The practical implications of AI scaling laws are vast and multidimensional. On one hand, effective scaling could reduce computational costs and improve AI model accessibility, making it feasible for broader application across various industries. On the other hand, scaling up models without addressing underlying limitations could lead to increased skepticism among stakeholders about AI's true capabilities, as noted in discussions from [tech experts](https://techcrunch.com/2025/03/19/researchers-say-theyve-discovered-a-new-method-of-scaling-up-ai-but-theres-reason-to-be-skeptical/) who view recent developments with caution.
Implications for the AI Industry
The introduction of the 'inference-time search' method in AI scaling signals a complex wave of implications for the AI industry. This technique, which facilitates an AI model's ability to generate and evaluate multiple answers before selecting the most suitable one, points towards a new direction in efficiency and performance, albeit met with skepticism. The method could potentially enhance AI model capabilities, as demonstrated by Google's Gemini 1.5 Pro, which reportedly benefitted in performance through this scaling approach. However, the industry's challenge lies in the perceived limitations of inference-time search as a true evolvement in reasoning abilities, rather than just a temporary fix. Experts are voicing the concern that while the method might yield performance gains, it does not necessarily transform the reasoning architecture essential for real-world application scenarios. This nuanced view is crucial for stakeholders across the AI landscape who aim to scale their reasoning models without succumbing to the high computational costs involved. Read more about it.
Learn to use AI like a Pro
Get the latest AI workflows to boost your productivity and business performance, delivered weekly by expert consultants. Enjoy step-by-step guides, weekly Q&A sessions, and full access to our AI workflow archive.
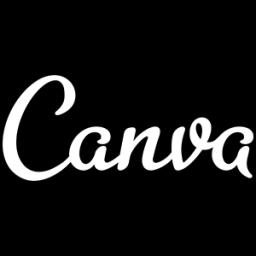
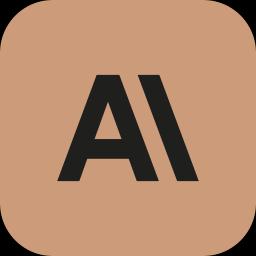
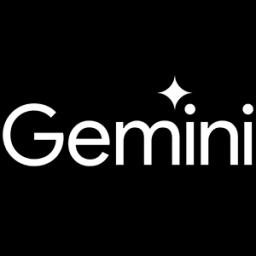
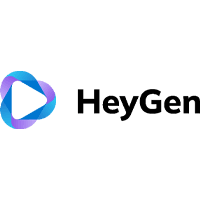
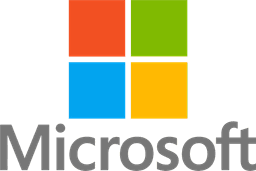
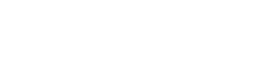
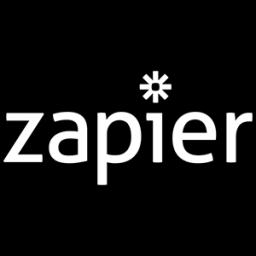
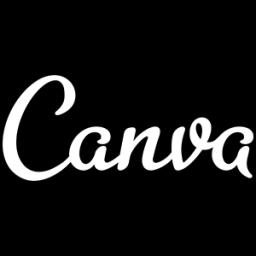
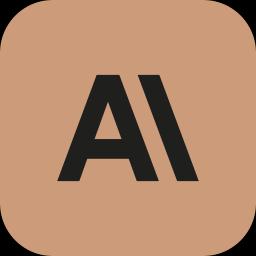
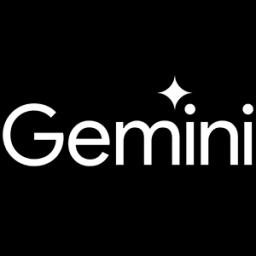
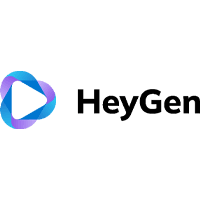
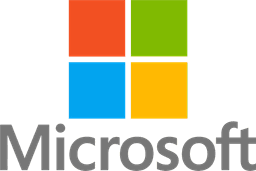
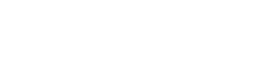
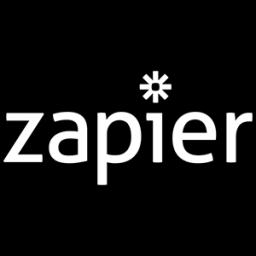
In the broader context, the AI industry's search for more refined and computationally efficient models is gaining momentum. The advent of faster models like GroQ, noted for its high-speed inference capabilities, further illustrates the direction in which the field is moving towards reducing the immense compute costs associated with large AI models. These advancements could potentially offset some of the computational drawbacks of inference-time search, offering complimentary pathways for balanced model expansion. At the same time, the ongoing exploration of quantization techniques to cut down memory and computational demands shows the AI industry's willingness to innovate rapidly to meet both performance and sustainability goals. In this dynamic environment, ensuring that technological breakthroughs like inference-time search are thoroughly vetted to deliver not just short-term gains but strategic, long-term value through genuine reasoning augmentation remains a pivotal consideration. Discover how GroQ is changing the game.
Despite the skepticism, the economic, social, and political implications of such advancements cannot be ignored. Economically, if inference-time search proves successful, it can lead to improved productivity and cost-efficiency, reshaping market dynamics and fostering new employment opportunities within AI development spheres. Socially, the more efficient deployment of AI models can help bridge the digital divide, although it will inevitably call for substantial reskilling efforts to mitigate the displacement of certain job categories. Politically, as AI models become more central to technological competition, issues surrounding regulation, ethical considerations, and international rivalry will demand attention from policymakers. Public trust in AI hinges on transparent and accountable AI practices, making it essential for the industry to align breakthroughs such as inference-time search with broader societal expectations to avoid backlash and promote inclusive technological growth. Learn more about the potential impacts.
Related Advancements in AI Technology
In recent years, the landscape of AI technology has been ever-evolving, with newfound advancements shaping the industry's future. One notable development is the concept of "inference-time search," described as a method where AI models generate various possible answers to a query and select the most appropriate one. This approach, although promising, has faced scrutiny for being more of a circumvention than genuine progress in reasoning capabilities. Research by TechCrunch highlights that Google’s Gemini 1.5 Pro has seen performance improvements through this method, yet experts remain cautious, emphasizing the need for clear evaluative criteria to ensure effectiveness.
Further advancements include the development of high-speed models like GroQ, which boasts significant efficiency in AI inference time, potentially outpacing models such as GPT-4. This not only reduces computational costs but also addresses time constraints in AI deployment. According to TomsHardware, GroQ's innovations could play a pivotal role in alleviating the burdens associated with current AI systems, making high-speed processing more accessible across various applications.
Amidst these innovations, the problem of AI's growing energy consumption cannot be overlooked. Large AI models demand substantial power, raising environmental and economic concerns. Studies, such as those reported by IEEE Spectrum, continually emphasize research into more energy-efficient algorithms and hardware to mitigate the environmental impact while fulfilling technological demand.
With the rise of quantization techniques and small language models (SLMs), the AI field is witnessing a shift towards optimized performance with minimal resource usage. These techniques involve reducing a model’s memory footprint, enabling faster and more efficient operations on less powerful devices. As detailed in NVIDIA's blog, such advancements make AI technologies more attainable, promoting a more sustainable approach to AI development without sacrificing performance excellence.
Learn to use AI like a Pro
Get the latest AI workflows to boost your productivity and business performance, delivered weekly by expert consultants. Enjoy step-by-step guides, weekly Q&A sessions, and full access to our AI workflow archive.
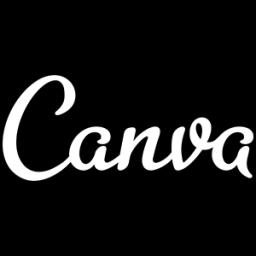
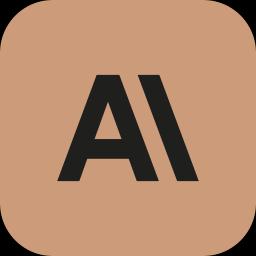
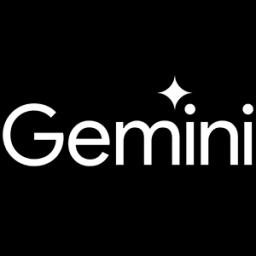
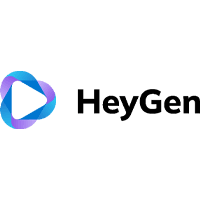
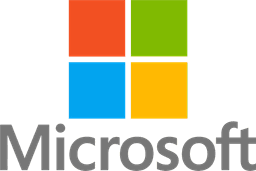
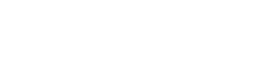
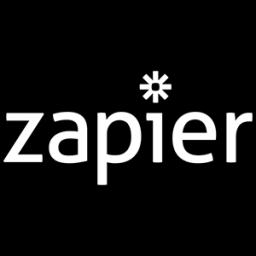
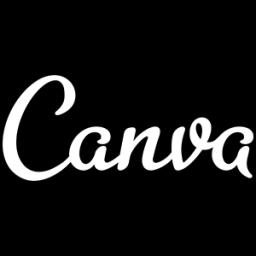
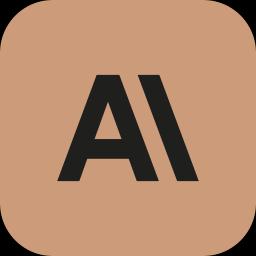
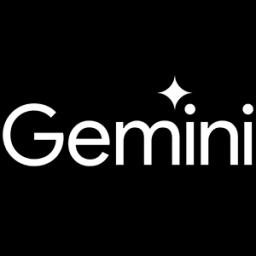
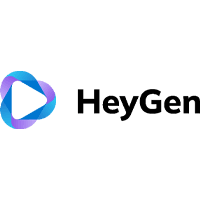
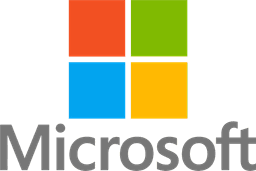
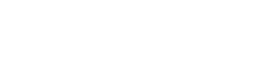
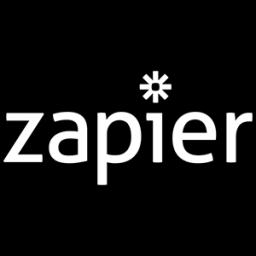
Expert Opinions on Inference-Time Search
On the other hand, proponents of inference-time search, like Google research fellow Eric Zhao, argue that its potential for self-verification at scale could contribute significantly to AI's evolution. Despite the method's controversial reception, it has found some favorable critiques in specialized cases where ground-truth verifiers are non-existent. As outlined by TechCrunch, Zhao contends that when executed at scale, the iterative nature of self-verification might pave the way for more reliable AI models. Nonetheless, the broader applicability and impact of these findings remain a point of contention within the AI research community.
Public Reactions and Perceptions
Public reactions to the newly proposed AI scaling method known as 'inference-time search' demonstrate a mixed landscape of enthusiasm and skepticism. Initially, the AI community and social media platforms buzzed with excitement over claims by researchers like Eric Zhao from Google, who highlighted significant performance improvements with models such as Gemini 1.5 Pro . However, this optimism was quickly met with caution from experts who viewed the technique as a stopgap measure rather than a breakthrough in AI's reasoning abilities .
The general public, keen on AI developments, found the notion that AI can now generate and choose from multiple potential answers intriguing. Yet, there was an underlying concern about the real-world applicability of such choices, especially in complex scenarios that lack clear-cut answers . Amidst these discussions, experts like Matthew Guzdial from the University of Alberta and Mike Cook from King’s College London have emphasized that the absence of definitive evaluation functions in many queries might undermine the utility of inference-time search in everyday tasks .
In summary, while the method holds promise for enhancing current AI models' performance—especially under constraints—there remains a prevailing consensus that it does not signify a paradigm shift in AI technology. Instead, it is often seen as a practical yet limited technique for circumventing specific challenges faced by current scalable reasoning models . This nuanced view indicates that, while innovation in AI continues to captivate and inspire, it also encourages critical discussions about the parameters of true technological advancement in artificial intelligence .
Future Implications of Inference-Time Search
The advancement of inference-time search methodologies in AI signals a transformative period for numerous sectors, though it brings both opportunities and challenges. This method, which enables AI models to generate and evaluate multiple responses to queries, promises enhanced efficiency and accuracy. If effectively harnessed, it has the potential to revolutionize industries by drastically cutting costs and boosting productivity. A notable example is the reported performance boost in Google's Gemini 1.5 Pro, illustrating the tangible improvements that can be achieved through this approach (). However, skepticism remains about its capability to truly advance AI reasoning rather than merely serving as a temporary solution to existing limitations ().
On an economic level, the potential benefits of inference-time search are profound. By improving AI accuracy and efficiency, this method could significantly enhance productivity across various domains and open new market avenues. As AI technology becomes more accessible, it fosters economic growth and could lead to job creation in areas related to AI development and maintenance. However, the competitive landscape might drive industry consolidation, as businesses vie to harness these advanced capabilities first ().
Learn to use AI like a Pro
Get the latest AI workflows to boost your productivity and business performance, delivered weekly by expert consultants. Enjoy step-by-step guides, weekly Q&A sessions, and full access to our AI workflow archive.
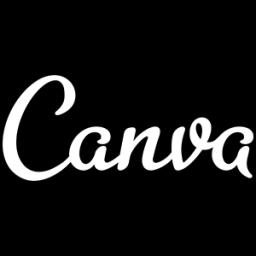
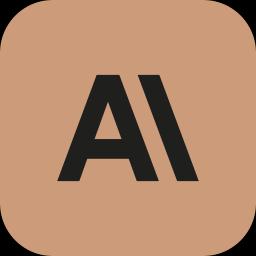
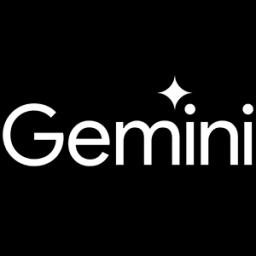
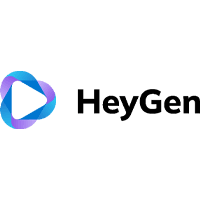
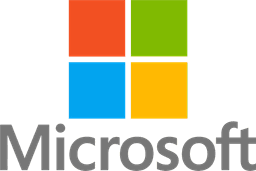
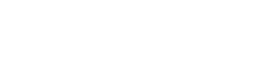
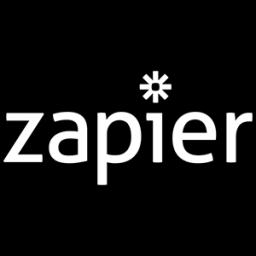
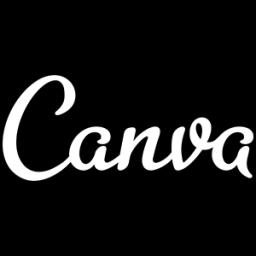
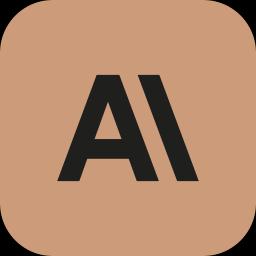
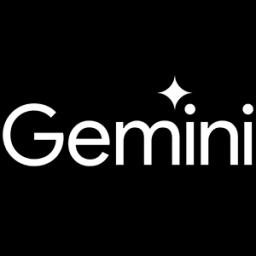
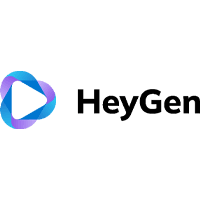
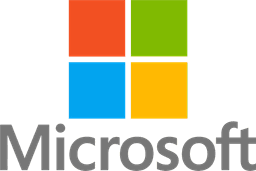
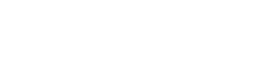
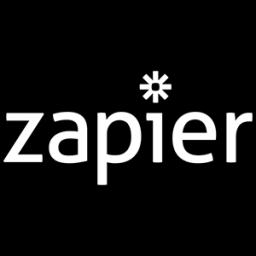
Socially, the implications of more efficient AI could be vast. If the costs associated with AI deployment decrease, these technologies could see wider adoption, potentially reducing the digital divide. Nonetheless, this also raises concerns over job displacement and the need for reskilling programs to prepare the workforce for emerging roles in AI (). Ethical concerns, such as addressing inherent AI biases, remain critical to ensuring that technological progress benefits all societal layers, reinforcing the importance of developing inclusive, fair, and transparent AI systems.
Politically, the rise of sophisticated AI capabilities like inference-time search could heighten global competition, compelling nations to ramp up investment in AI research and development. Consequently, this could lead to stricter regulations as governments strive to manage the ethical, safety, and economic impacts of such technologies. Public trust in AI's advancement hinges on transparent practices and accountability, making regulatory frameworks a pivotal area of focus for policymakers (). These measures will be crucial in balancing innovation with oversight, ensuring that AI's benefits are maximized while its risks are minimized.
Economic, Social, and Political Impacts of AI Scaling
The advancement of artificial intelligence, especially through scaling techniques like inference-time search, brings profound economic impacts. By potentially enhancing AI accuracy and reducing the costs associated with AI development, this innovation could lead to significant productivity boosts across various industries. New markets may emerge as AI becomes more robust and versatile, offering opportunities for growth and innovation. This can also lead to increased competition among tech companies, which might drive industry consolidation. As businesses strive to leverage these advancements, the AI sector may witness a reshaping of its economic landscape, influencing everything from job creation to service delivery .