AI Smackdown: Llama vs. GPT
Llama 3.1 and GPT-4 Go Head to Head: Open-Source AI Rivals Proprietary Models in Medical Diagnostics
Last updated:

Edited By
Mackenzie Ferguson
AI Tools Researcher & Implementation Consultant
Llama 3.1 405B delivers a stunning performance in medical diagnostics, matching GPT-4's accuracy in diagnosing complex cases from the New England Journal of Medicine. This marks a significant milestone for open-source AI, offering data privacy, customizability, and economic benefits to healthcare while reshaping the dialogue around AI in medicine.
Introduction to AI in Medical Diagnostics
The integration of artificial intelligence (AI) in medical diagnostics represents a significant advancement in healthcare, offering new avenues for enhancing diagnostic precision and efficiency. AI technologies, such as the open-source model Llama 3.1 405B, have shown promise in performing complex diagnostic tasks. According to recent findings, Llama 3.1 has matched the diagnostic accuracy of established proprietary models like GPT-4, achieving 70% accuracy on complex medical cases published in the *New England Journal of Medicine*. This capability highlights the potential of AI to transform traditional diagnostic practices by improving the accuracy and speed of diagnosis, thereby mitigating patient mortality and reducing the overall cost of healthcare. The open-source nature of models like Llama 3.1 enhances these benefits by offering data privacy and allowing health institutions to customize AI solutions according to their specific needs [1](https://medicalxpress.com/news/2025-03-source-ai-proprietary-tough-medical.html).
In an era where healthcare challenges are increasingly complex, AI-based diagnostics emerge as indispensable tools for clinicians. These tools promise to reduce inaccuracies in diagnoses and offer dynamic support systems that assist healthcare providers in swift decision-making processes. The use of AI not only serves as a valuable aid in the diagnostic phase but also acts as a catalyst for evolving patient care strategies. Health facilities utilizing open-source AI models can maintain complete control over patient data, aligning with stringent regulations on data security and privacy. Consequently, AI-driven diagnostics signify a shift toward a more secure, efficient, and patient-centered healthcare model, as it enables quick, reliable, and consistent healthcare service delivery for better patient outcomes [1](https://medicalxpress.com/news/2025-03-source-ai-proprietary-tough-medical.html).
Learn to use AI like a Pro
Get the latest AI workflows to boost your productivity and business performance, delivered weekly by expert consultants. Enjoy step-by-step guides, weekly Q&A sessions, and full access to our AI workflow archive.
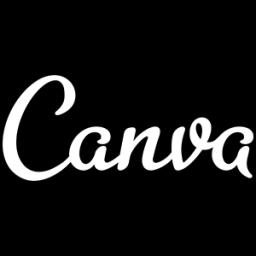
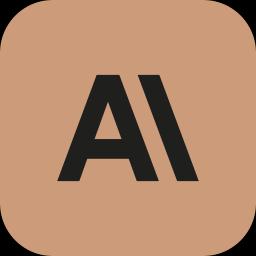
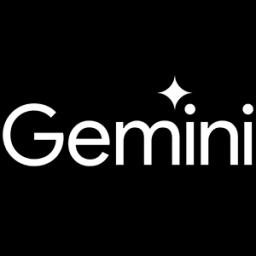
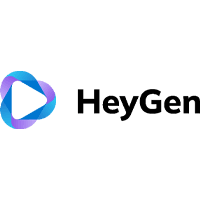
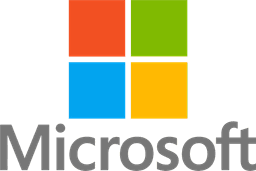
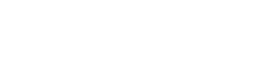
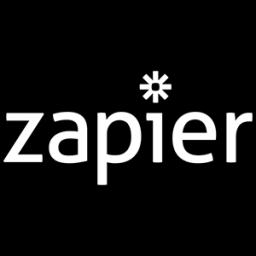
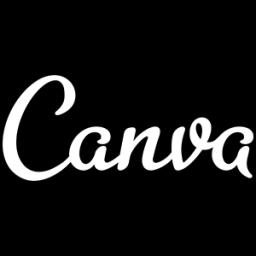
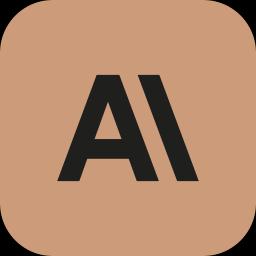
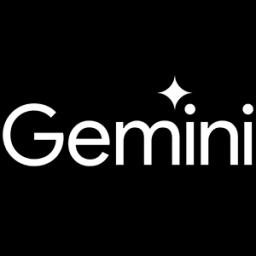
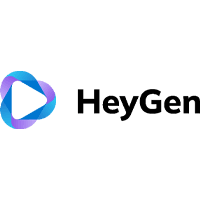
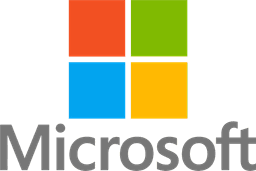
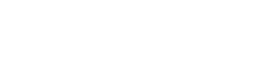
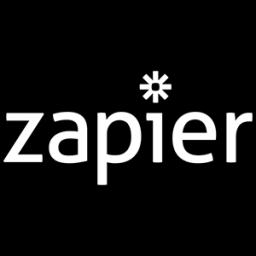
The use of AI in medical diagnostics also represents a leap towards more inclusive and equitable healthcare. By lowering the costs associated with diagnostic tools and improving data customization and privacy, open-source AI makes it possible for smaller clinics and practices, especially in underserved areas, to access cutting-edge diagnostic capabilities. This democratization of healthcare technology promises to reduce disparities in health care quality and availability, offering advanced solutions to communities that have traditionally been marginalized in terms of healthcare access. As AI continues to evolve, its role in transforming our approach to healthcare through improving diagnostic accuracy and reducing unnecessary costs becomes increasingly apparent. The continued development and integration of AI tools into medical diagnostics are poised to revolutionize healthcare delivery on a global scale [1](https://medicalxpress.com/news/2025-03-source-ai-proprietary-tough-medical.html).
Comparison of Open-Source and Proprietary AI Models
The debate between open-source and proprietary AI models in medical diagnostics is a reflection of broader trends in the AI field. Open-source models, like Llama 3.1, offer enhanced data privacy and customizability, allowing users to host models on-premises. This local hosting reduces concerns about data breaches and regulatory compliance associated with data sharing on external servers, a requirement with proprietary models such as GPT-4. Customizability allows hospitals to tailor AI solutions to their specific patient demographics and healthcare needs, potentially improving diagnostic accuracy and healthcare outcomes ().
Conversely, proprietary AI models often provide advantages in ease of integration and professional support. These systems are typically designed to work seamlessly with existing hospital IT infrastructures, reducing the technical burden on internal IT staff. Moreover, continuous professional support ensures that any technical issues can be swiftly resolved, maintaining healthcare service provision without significant downtime. However, these benefits come with higher costs, as proprietary models usually operate on a subscription or pay-per-use basis, requiring ongoing financial commitments from healthcare providers ().
The performance of Llama 3.1 405B, an open-source AI model, demonstrates that open-source alternatives can match the capabilities of proprietary giants like GPT-4 in complex medical diagnostics. Tested under rigorous conditions using cases from the *New England Journal of Medicine*, Llama achieved 70% diagnostic accuracy, a performance indicative of its potential to support clinical decision-making in real-world healthcare settings. This comparable performance suggests that open-source models are not only viable but competitive, offering cost-effective solutions for healthcare institutions looking to leverage AI technology without the financial implications of proprietary systems ().
Learn to use AI like a Pro
Get the latest AI workflows to boost your productivity and business performance, delivered weekly by expert consultants. Enjoy step-by-step guides, weekly Q&A sessions, and full access to our AI workflow archive.
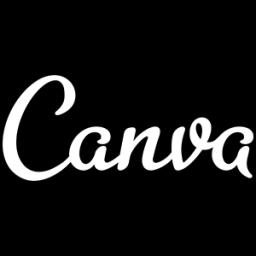
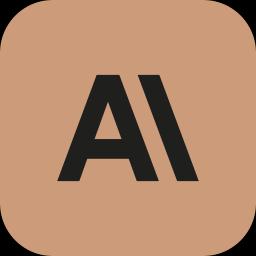
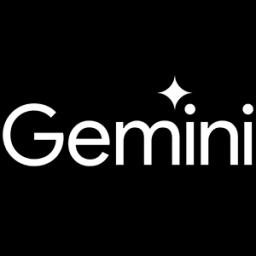
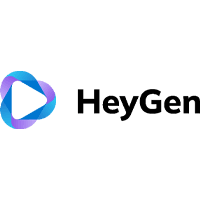
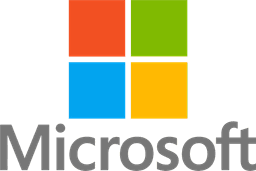
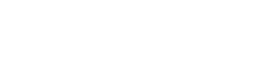
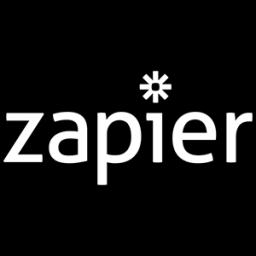
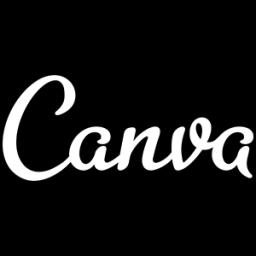
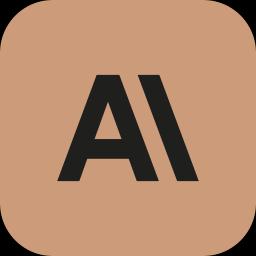
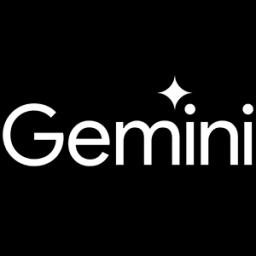
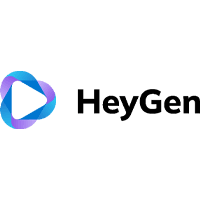
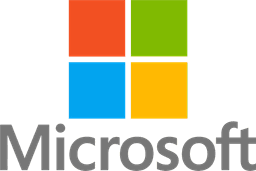
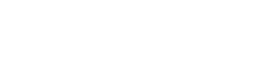
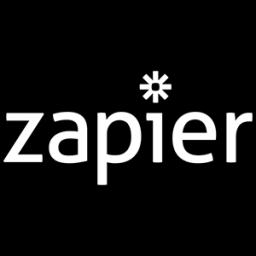
The rise of open-source AI models like Llama underscores a shift towards more democratized access to AI technologies in healthcare. By reducing cost barriers and offering flexibility in deployment, open-source models provide opportunities for resource-limited healthcare facilities to access state-of-the-art diagnostic tools, potentially leading to improvements in healthcare delivery and patient outcomes globally. The innovations driven by open-source communities also contribute to the continual enhancement of these models, fostering a collaborative approach to technological advancement in the medical field ().
Performance and Testing of Llama 3.1 405B
The performance and testing of Llama 3.1 405B reveal significant advancements in the field of medical diagnostics, positioning open-source AI as a formidable alternative to proprietary counterparts like GPT-4. Llama 3.1 405B achieved a commendable 70% accuracy on an array of challenging cases from the *New England Journal of Medicine*. This level of performance underscores the potential of open-source tools to match the diagnostic prowess of top-tier proprietary models, thereby challenging the conventional reliance on closed-source AI for medical diagnostics. Not only does this present a cost-effective solution, but it also advances data privacy and customization due to the capabilities inherent in open-source frameworks. For clinicians and researchers, this marks a shift towards more democratized and accessible healthcare solutions, especially in settings where resource constraints limit access to proprietary AI models. By nearly equalling the performance of GPT-4, Llama 3.1 405B brings to light crucial discussions around openness, collaboration, and innovation in AI, making it a noteworthy development in the AI-powered healthcare landscape. For further insights, the study comparing Llama 3.1 405B and GPT-4 is available in the JAMA Health Forum study publication.
Testing of Llama 3.1 405B involved rigorous comparisons against GPT-4, using clinical cases sourced from renowned medical journals. It demonstrated its capabilities by diagnosing cases both included in its training and up-to-date cases, ensuring a robust evaluation framework. The model's ability to tackle complex medical queries emphasizes its strong potential as an AI-assisted diagnostic tool. Moreover, by operating within an open-source paradigm, Llama 3.1 405B allows for greater interpretability and transparency, facilitating trust and adaptability among medical professionals. Its deployment can thus lead to enhanced healthcare delivery that aligns with data governance standards and supports effective decision-making in clinical environments. For details on Llama's diagnostic assessment and its implications, visit the full report at Medical Xpress here.
Evaluating the performance of Llama 3.1 405B further highlights the crucial role AI plays in transforming medical diagnostics. The AI's proficiency in analyzing complex medical cases highlights its utility in environments where traditional diagnostic tools might fall short. Given its open-source nature, Llama 3.1 405B stands out by offering a flexible, adaptable tool that democratizes high-quality healthcare by providing ubiquitous access regardless of economic or geographic constraints. This positions it as a catalyst for change within an industry that is progressively moving towards open-source solutions to foster innovation and collective progress. The enthusiasm and optimism surrounding its widespread application invite a broader discussion regarding ethical practices, training data transparency, and the frameworks required to ensure equitable AI adoption. Detailed insights can be found in our source article on Medical Xpress.
Potential Benefits of AI in Diagnostics
Artificial Intelligence (AI) is increasingly being recognized for its potential to revolutionize medical diagnostics, offering numerous benefits that can enhance healthcare delivery. One of the primary advantages of integrating AI into diagnostic processes is the improvement in accuracy and speed of diagnoses, which is critical for patient outcomes. AI systems such as Llama 3.1 405B, an open-source model, have shown diagnostic accuracy comparable to that of proprietary models like GPT-4. This accuracy can significantly reduce diagnostic errors and enhance the reliability of medical assessments [1](https://medicalxpress.com/news/2025-03-source-ai-proprietary-tough-medical.html).
The potential of AI tools to process and analyze vast amounts of medical data quickly means that they can assist clinicians in identifying complex patterns and correlations that might not be immediately apparent through traditional diagnostic methods. This capability not only supports more precise diagnostics but also accelerates the diagnostic process itself. As a result, patients can receive timely interventions, which is particularly vital in life-threatening situations such as stroke or cancer diagnosis [1](https://medicalxpress.com/news/2025-03-source-ai-proprietary-tough-medical.html).
Learn to use AI like a Pro
Get the latest AI workflows to boost your productivity and business performance, delivered weekly by expert consultants. Enjoy step-by-step guides, weekly Q&A sessions, and full access to our AI workflow archive.
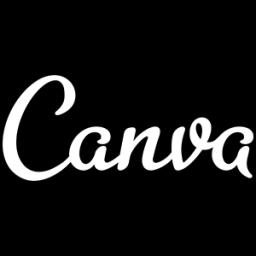
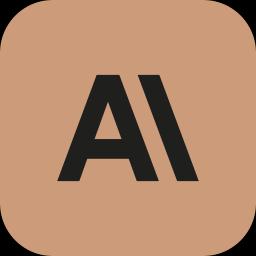
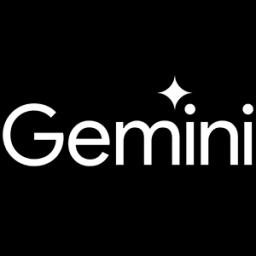
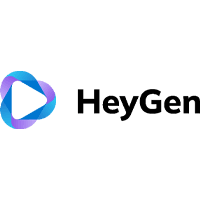
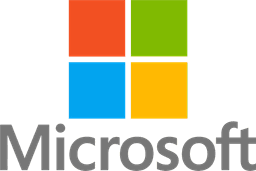
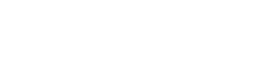
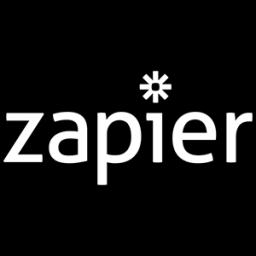
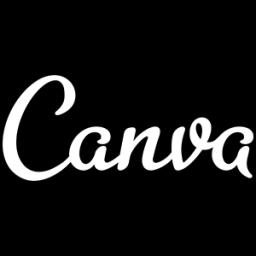
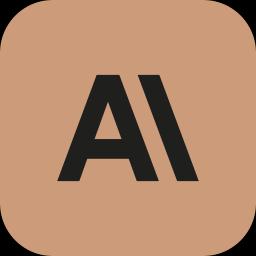
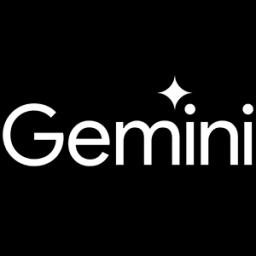
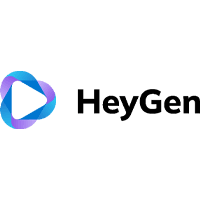
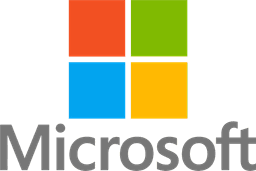
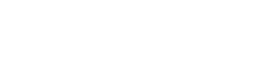
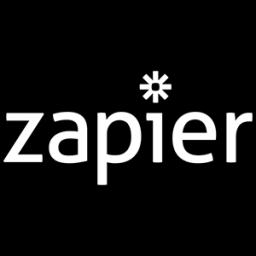
AI's predictive abilities are another significant benefit, as they can anticipate patient conditions and suggest personalized treatment plans. Such precision medicine approaches have the potential to optimize patient care and reduce healthcare costs by tailoring treatments to individual patient needs [1](https://medicalxpress.com/news/2025-03-source-ai-proprietary-tough-medical.html). The growing competitiveness of open-source AI models in diagnostics presents an exciting opportunity for healthcare systems to adopt these technologies widely, potentially democratizing access to advanced diagnostic capabilities and contributing to improved healthcare equity.
Additionally, the adaptability of AI tools means they can be customized to meet specific healthcare settings' needs, from large urban hospitals to remote clinics with limited resources. This adaptability enables broader access to high-quality diagnostic tools, which is especially important in underserved areas. By hosting open-source AI models on local infrastructure, healthcare providers can also ensure better data privacy and control over patient information, addressing one of the significant concerns associated with cloud-based, closed-source models [1](https://medicalxpress.com/news/2025-03-source-ai-proprietary-tough-medical.html).
As the capabilities of AI systems continue to expand, so too will their role in supporting healthcare professionals. These AI tools are not intended to replace human clinicians but to act as valuable diagnostic aides that enhance decision-making and optimize outcomes. The integration of AI in diagnostics is further spurred by its capacity to handle repetitive tasks swiftly, such as image analysis, freeing up healthcare professionals to focus on more complex clinical decision-making processes. Embracing AI in diagnostics offers promising paths forward in terms of improving patient care and healthcare efficiency [1](https://medicalxpress.com/news/2025-03-source-ai-proprietary-tough-medical.html).
Current Events in AI-Driven Medical Advances
Recent developments in artificial intelligence (AI) have significantly impacted the field of medical diagnostics, with open-source models demonstrating tremendous potential. One such model, Llama 3.1 405B, has emerged as a credible alternative to proprietary models like GPT-4, showcasing similar diagnostic accuracy. According to recent reports, this open-source AI achieved a 70% accuracy rate on challenging cases published in the *New England Journal of Medicine*. By offering comparable performance, open-source AI models are helping to democratize healthcare, ensuring broader access to advanced diagnostic capabilities without the often prohibitive costs associated with proprietary solutions.
The performance of AI in medical diagnostics is reshaping the healthcare landscape. Open-source models such as Llama 3.1 405B provide benefits in terms of data privacy and customizability, allowing healthcare providers to tailor these models to their specific needs. Meanwhile, the proprietary models usually come with ready-made integration options and professional support. As these models evolve, they promise to enhance diagnostic accuracy and speed, potentially reducing patient mortality and healthcare costs, as outlined in articles discussing AI's role in healthcare advancements (see source).
Open-source AI models are not only expanding the possibilities for medical diagnostics but also introducing new ethical and operational challenges. The ability to run AI models locally supports data privacy, a significant concern in handling sensitive patient information. Moreover, these models can be customized for various patient demographics, enhancing precision in diagnostics. However, the rise of such technologies also raises questions about the security of open-source platforms and the potential misuse in scenarios not fully anticipated by developers (source: medicalxpress.com).
Learn to use AI like a Pro
Get the latest AI workflows to boost your productivity and business performance, delivered weekly by expert consultants. Enjoy step-by-step guides, weekly Q&A sessions, and full access to our AI workflow archive.
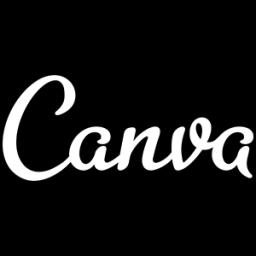
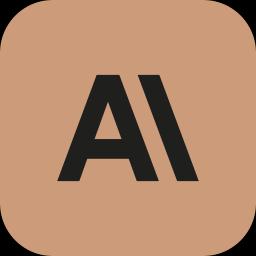
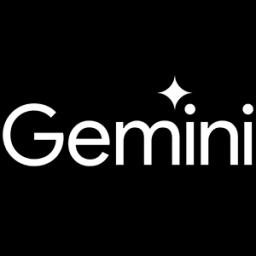
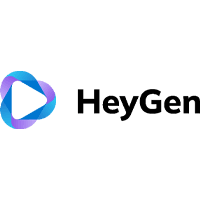
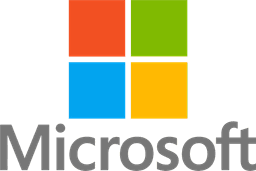
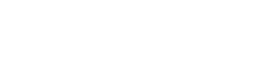
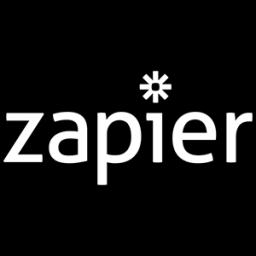
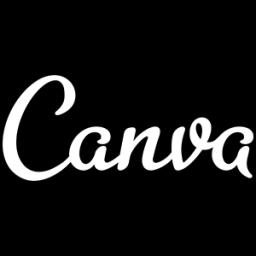
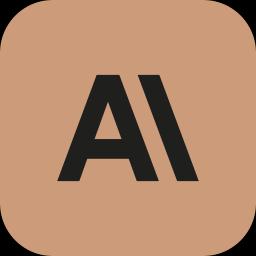
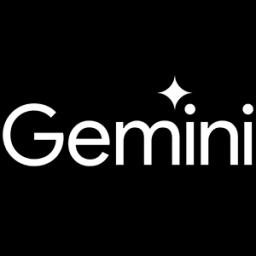
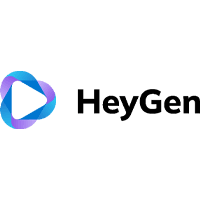
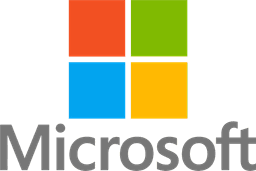
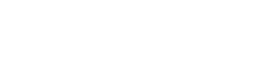
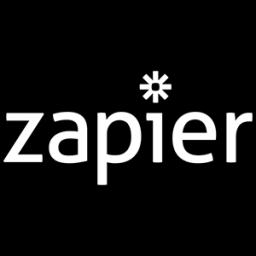
The future of AI in medical diagnostics looks promising with the continued development of open-source models like Llama 3.1. Their integration into healthcare systems can lower the entry barriers for smaller hospitals and clinics by reducing costs typically associated with closed-source solutions. Innovations driven by the AI community are paving the way for more inclusive healthcare solutions that cater to specific regional needs and challenges. As noted by experts, the rapid progression of these technologies is a testament to the collaborative potential of the global tech community and its ability to tackle complex medical issues, as highlighted in this report.
Expert Opinions on AI Models in Healthcare
The integration of AI models into the healthcare sector has generated substantial discussion among experts, particularly focusing on the comparison between open-source and proprietary systems. Llama 3.1 405B, an open-source AI model, has offered a compelling case by matching the diagnostic accuracy of the proprietary GPT-4 in complex medical cases. Expert opinions underscore the rapid advancement of open-source models like Llama, which are catching up quickly with their proprietary counterparts. This shift is being hailed as a momentous change, as it provides the healthcare industry with a broader range of options and the potential for significant innovation.
Open-source models provide notable advantages, such as increased data privacy and customizability, which are particularly appealing in a field as sensitive as healthcare. This reflects a paradigm shift where researchers and practitioners now have tools that align more closely with their specific needs, without compromising patient confidentiality. The performance of open-source models like Llama 3.1 in challenging diagnostic scenarios is not only a testament to technological advancements but also indicates a growing trend toward democratizing AI technology in healthcare settings.
There is a consensus among industry experts that while technological advancements with open-source models are exciting, caution is necessary to ensure these tools are integrated responsibly into healthcare systems. Experts advocate for active physician involvement in AI integration to maintain ethical standards and to ensure that these innovations effectively serve the objectives of improved patient care. Experts highlight that while these tools offer substantial promise, there must be a continued focus on ensuring their deployment is aligned with ethical practices and patient safety.
The broader impact of AI models like Llama 3.1 extends beyond healthcare solutions; it symbolizes a critical shift in the AI landscape by presenting feasible, competitive alternatives to proprietary models. This development is pivotal as it could lead to increased competition and spurred innovation within the AI and healthcare industries. By providing healthcare professionals with diverse AI tools, the sector is likely to see advancements that allow for more personalized and accurate patient care, ultimately benefiting patients through enhanced medical outcomes.
Public Reactions to AI Diagnostics
Public reactions to the advancement of AI diagnostics, particularly with the emergence of open-source models like Llama 3.1, are multifaceted and reflect a mix of enthusiasm and concern. On one hand, there's a growing optimism about the potential of AI systems to significantly enhance medical diagnostic accuracy and efficiency. The fact that Llama 3.1 achieved a 70% accuracy rate on challenging cases from the *New England Journal of Medicine* suggests a promising future where AI aids in reducing patient mortality and healthcare costs by minimizing diagnostic errors [1](https://medicalxpress.com/news/2025-03-source-ai-proprietary-tough-medical.html). People especially appreciate the open-source nature of these models, which offers greater control over data privacy and customization capabilities [2](https://pmc.ncbi.nlm.nih.gov/articles/PMC11498353/).
Learn to use AI like a Pro
Get the latest AI workflows to boost your productivity and business performance, delivered weekly by expert consultants. Enjoy step-by-step guides, weekly Q&A sessions, and full access to our AI workflow archive.
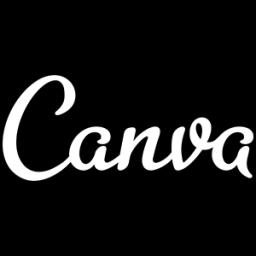
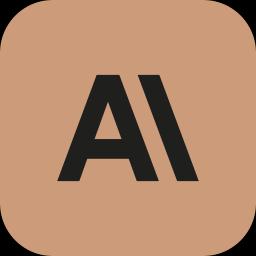
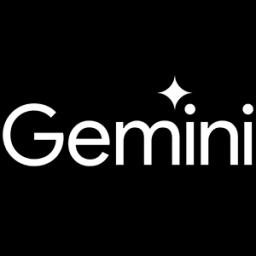
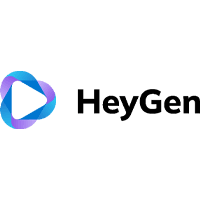
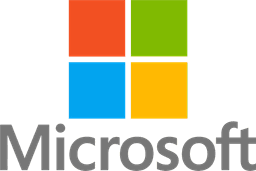
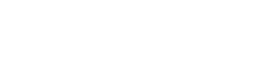
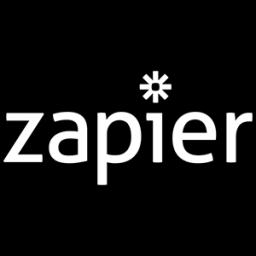
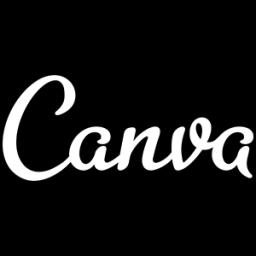
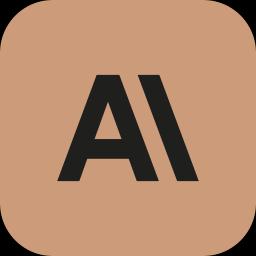
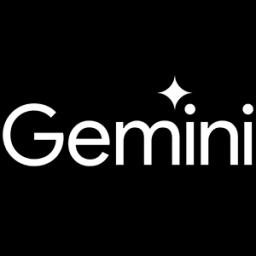
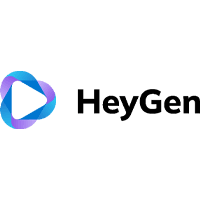
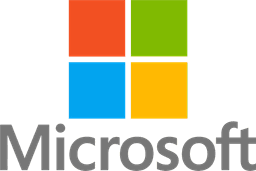
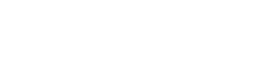
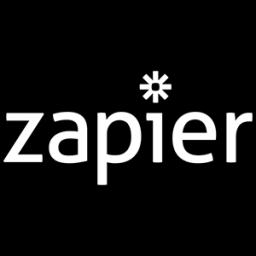
However, the path forward is not without its challenges. Many individuals express worries about potential job displacement within the healthcare industry as AI becomes more integrated into diagnostic processes [3](https://community.openai.com/t/llama-3-1-better-than-chatgpt-4-for-coding-and-programming/883557). There are also concerns regarding the reliability of AI in making accurate diagnoses without human oversight and the need for substantial validation to ensure efficacy and safety [2](https://pmc.ncbi.nlm.nih.gov/articles/PMC11498353/). Ethical issues, including the transparency of AI training data and the risk of misuse, are also prevalent topics of discussion among the public and professionals alike [2](https://pmc.ncbi.nlm.nih.gov/articles/PMC11498353/).
Despite these concerns, the general sentiment towards AI diagnostics remains cautiously optimistic, appreciating the benefits while advocating for responsible and ethical integration into the healthcare system. This balance of enthusiasm and reservation is indicative of a broader societal shift towards acceptance of AI as a crucial component of modern medicine, albeit one that must be handled with care and oversight [4](https://hms.harvard.edu/news/open-source-ai-matches-top-proprietary-llm-solving-tough-medical-cases). The evolution of AI in healthcare is likely to continue to provoke mixed reactions, as stakeholders navigate the complexities of this powerful technology [5](https://hms.harvard.edu/news/open-source-ai-matches-top-proprietary-llm-solving-tough-medical-cases).
Future Implications of Open-Source AI in Healthcare
The future implications of open-source AI in healthcare signal a transformative shift in how medical diagnostics and patient care are conceptualized. The demonstrated capabilities of Llama 3.1 405B, which matches the diagnostic prowess of established proprietary systems like GPT-4, underscore the potential for these tools to revolutionize the healthcare industry. Highlights of Llama 3.1 include its affordability, accessibility, and the provision of customizable solutions tailored to specific healthcare environments. These factors render AI tools not only more approachable for healthcare providers of varying scales but also integral in advancing clinical accuracy and service efficiency. As AI solutions become increasingly embedded within healthcare practices, the potential for widespread benefits, including reduced patient mortality and healthcare costs, becomes achievable.
The competitive performance of open-source AI technologies like Llama 3.1 positions them as viable alternatives to traditional proprietary models in healthcare diagnostics. By offering robust analytical capabilities without the substantial costs associated with closed-source models, these AI tools democratize access to high-level diagnostic systems. Healthcare institutions, particularly those in resource-limited settings, stand to benefit from the cost-efficiencies and reduced dependency on expensive third-party services provided by open-source AI. This development fosters an inclusive technology framework that supports a variety of healthcare needs while maintaining patient data privacy and the potential for local customization.
Incorporating open-source AI into healthcare heralds a new era of innovation characterized by speed, accuracy, and cost-effectiveness. The flexibility and transparency inherent in open-source solutions allow for rapid adaptations to evolving clinical needs and medical knowledge, fostering an environment of perpetual learning and development. Furthermore, these models encourage community involvement and collaboration, which can drive faster problem-solving and knowledge-sharing across medical fields, potentially accelerating the pace of innovation in diagnostics and treatment methodologies.
Economic Impacts of Open-Source AI Models
The advent of open-source AI models like Llama 3.1 is poised to deliver transformative economic impacts within the healthcare sector. Primarily, these models offer a drastic reduction in costs for healthcare providers, as they are not subject to the licensing and subscription fees typically associated with closed-source models. This cost-effective nature enables even smaller or resource-constrained facilities to integrate advanced AI diagnostic tools into their operations, potentially leveling the playing field in terms of access to cutting-edge technology. Moreover, since these models can be tailored and customized to fit the specific needs of a healthcare provider or patient population, they facilitate more efficient resource use, potentially reducing waste and enhancing the precision of diagnostic procedures, which could lead to substantial long-term savings for healthcare systems. However, the shift to open-source AI does come with upfront investment requirements in terms of infrastructure and expertise, presenting a substantial initial financial hurdle for some institutions to overcome.
Learn to use AI like a Pro
Get the latest AI workflows to boost your productivity and business performance, delivered weekly by expert consultants. Enjoy step-by-step guides, weekly Q&A sessions, and full access to our AI workflow archive.
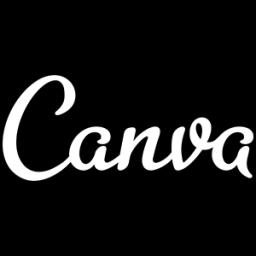
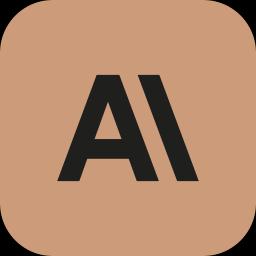
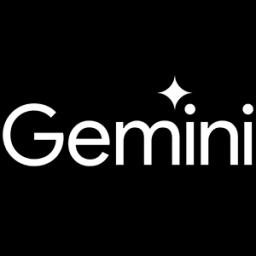
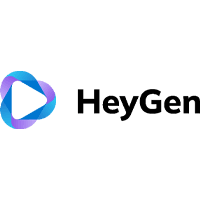
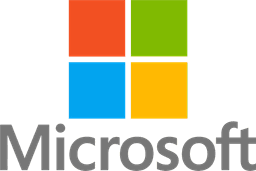
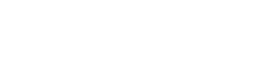
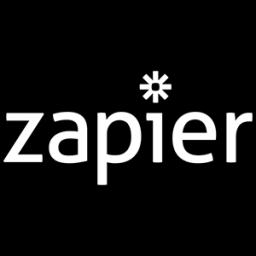
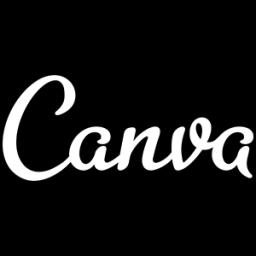
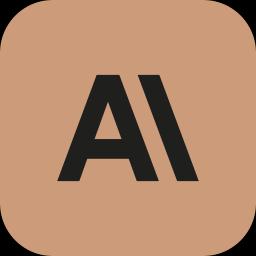
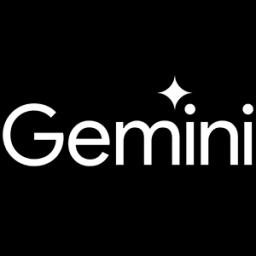
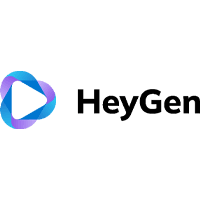
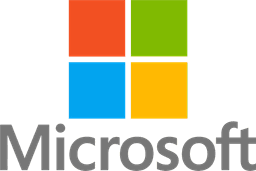
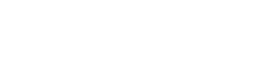
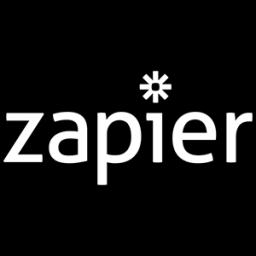
Open-source models like Llama 3.1 also play a crucial role in fostering economic dynamism and innovation within the AI and medical industries. By being openly available, these models encourage collective improvements and refinements from a global community of developers and researchers. This collaborative ecosystem could accelerate the pace of technological advancements and lead to breakthroughs in AI applications in healthcare. The customization capabilities of open-source models mean healthcare institutions can adapt AI tools to better suit unique clinical requirements or emerging public health challenges, further highlighting their economic value as flexible and adaptive solutions for medical diagnostics. Furthermore, the widespread adoption and success of such models could drive increased competition in the AI market, potentially pushing proprietary developers to innovate at a faster pace or reduce their prices in order to remain competitive.
The economic ramifications of embracing open-source AI models extend beyond direct financial savings and innovation; they also include considerable market shifts and employment changes. As open-source models become more prevalent, there may be a disruption in the current job landscape, particularly within roles related to traditional diagnostic processes. However, this transition could also spur the creation of new jobs focused on AI development, customization, and maintenance, thereby reshaping the employment landscape within the tech and healthcare sectors. Additionally, the increasing reliance on AI for diagnostic purposes could further drive the necessity for interdisciplinary collaboration, merging fields such as data science, engineering, and healthcare, which could lead to richer, more diverse career paths and educational requirements within these industries.
The public sector may also experience economic benefits as open-source AI becomes more widely adopted. Governments and public health bodies could leverage these models to enhance the efficiency of public health initiatives and medical research without incurring significant expenses tied to proprietary technology licensing. This could lead to more budget-friendly advancements in public health infrastructure and programs aiming to improve population health outcomes. The availability of low-cost, high-accuracy diagnostic tools in public healthcare systems could thus significantly improve healthcare access and equity, addressing some of the economic disparities that currently exist in many regions across the globe. These models enable a more sustainable approach to healthcare innovation, ensuring that advancements are not solely dependent on private sector investments but are accessible to a broader range of institutions.
Social Impacts and Healthcare Equity
In the realm of healthcare equity, the rise of AI diagnostic tools powered by open-source technology is set to revolutionize access to quality medical care. Open-source AI, such as the Llama 3.1 model, has demonstrated an impressive capability to match the performance of proprietary systems like GPT-4. This advancement [is profiled in a study published in *JAMA Health Forum*](https://medicalxpress.com/news/2025-03-source-ai-proprietary-tough-medical.html), where it was shown to achieve a 70% accuracy rate in diagnosing complex medical cases. Such a development is particularly significant for underserved communities, often found in rural areas or regions with limited healthcare resources. The customizable nature of open-source AI allows for tailored solutions that can cater to specific community health needs, thereby closing the gap in healthcare quality and accessibility [2](https://hms.harvard.edu/news/open-source-ai-matches-top-proprietary-llm-solving-tough-medical-cases).
The social implications of integrating AI into healthcare go beyond simple technological advances. By democratizing access to these powerful diagnostic tools, there is potential for reducing healthcare costs for both providers and patients. For instance, with the potential reduction in diagnostic errors and faster, more accurate diagnoses, we can anticipate a decrease in unnecessary procedures and hospital stays. This not only benefits the healthcare system economically but also leads to better health outcomes overall. Data privacy, a major concern in the digital era, is further bolstered by open-source models, as they allow institutions to retain control of their own data without relying on external servers. Such autonomy can significantly increase patient trust and participation in digital health initiatives, fostering a more inclusive healthcare environment [2](https://hms.harvard.edu/news/open-source-ai-matches-top-proprietary-llm-solving-tough-medical-cases)[6](https://medicalxpress.com/news/2025-03-source-ai-proprietary-tough-medical.html).
As open-source AI continues to prove its value in medical diagnostics, one of the most profound social impacts is the shift in public perception and confidence in healthcare systems. With successes like those achieved by Llama 3.1, there is growing optimism about the role of AI in enhancing health services, particularly in diagnosing and treating complex conditions. These tools provide an opportunity to alleviate pressures from overworked healthcare professionals, potentially transforming how patient care is delivered. However, it is crucial to develop governance frameworks that ensure these technologies are implemented effectively and ethically, safeguarding against potential misuse while encouraging innovation [6](https://medicalxpress.com/news/2025-03-source-ai-proprietary-tough-medical.html).
Learn to use AI like a Pro
Get the latest AI workflows to boost your productivity and business performance, delivered weekly by expert consultants. Enjoy step-by-step guides, weekly Q&A sessions, and full access to our AI workflow archive.
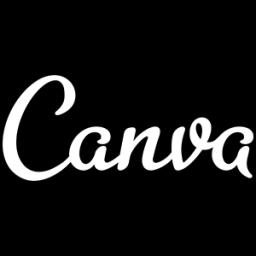
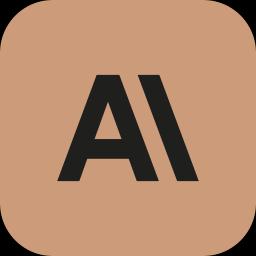
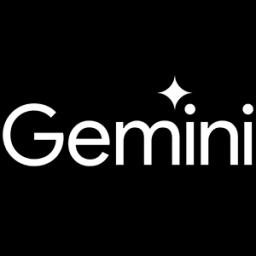
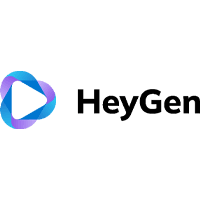
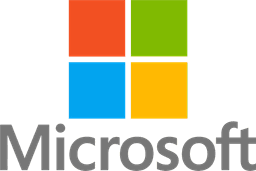
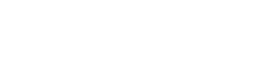
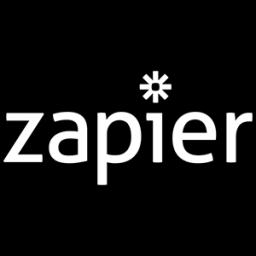
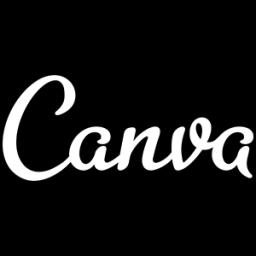
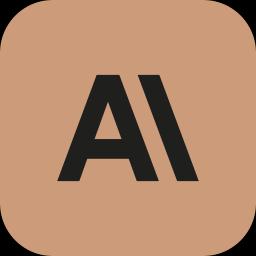
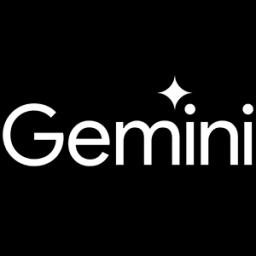
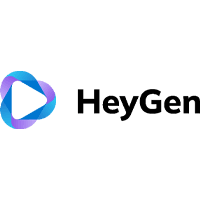
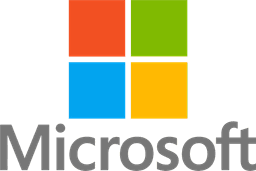
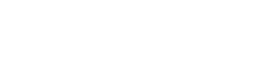
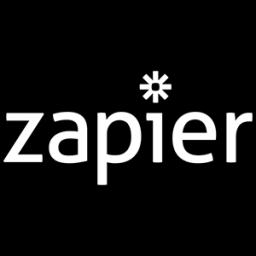
Political Challenges and Regulatory Considerations
The development and implementation of AI technologies in the medical field bring forth a myriad of political challenges and regulatory considerations. As open-source AI models like Llama 3.1 start to compete vigorously with proprietary systems like GPT-4, regulatory bodies face the difficult task of ensuring that these advancements do not compromise patient safety or data privacy. Policymakers must strike a delicate balance between fostering innovation and safeguarding public health, necessitating stringent guidelines and frameworks to oversee AI's deployment in healthcare sectors. The increasing reliance on AI for medical diagnostics raises questions about accountability, particularly concerning erroneous outputs or biases inherent in AI algorithms, leading to potential patient harm [4](https://bioengineer.org/open-source-ai-rivals-leading-proprietary-models-in-tackling-complex-medical-cases/).
The open-source nature of AI models like Llama 3.1 brings additional regulatory scrutiny, especially concerning intellectual property rights and the management of sensitive data. Countries are grappling with how to manage these issues, as AI's role in healthcare becomes more prominent. Legislative measures must address the protection of intellectual property while encouraging innovations that could enhance medical outcomes. Moreover, with open-source AI, there is the likelihood of increased misuse, which could prompt calls for stronger international cooperation to standardize regulations and ensure that these technologies are used responsibly [6](https://hms.harvard.edu/news/open-source-ai-matches-top-proprietary-llm-solving-tough-medical-cases).
Furthermore, the global nature of healthcare challenges and AI development necessitates a collaborative approach to regulation. Governments across the globe are urged to harmonize their regulatory standards to prevent discrepancies that might hinder AI's potential benefits in medicine. This situation presents both opportunities for cross-border collaboration and challenges related to different regulatory environments and legal systems [5](https://hms.harvard.edu/news/open-source-ai-matches-top-proprietary-llm-solving-tough-medical-cases).
Ethical considerations also play a crucial role in the political landscape of AI-driven healthcare. As AI tools have the capacity to significantly alter the way diagnoses and treatments are provided, ensuring ethical deployment becomes paramount. Policymakers, healthcare providers, and AI developers must work together to create frameworks that prioritize patient well-being, uphold ethical standards, and guarantee that AI applications are transparent and equitable. Effective regulation will be essential to build public trust and facilitate the integration of these powerful tools into everyday medical practices [4](https://bioengineer.org/open-source-ai-rivals-leading-proprietary-models-in-tackling-complex-medical-cases/).
Innovation and Development in Open-Source AI
The landscape of Artificial Intelligence (AI) in healthcare is undergoing rapid transformation as open-source models begin to rival traditional proprietary systems. A significant example of this is the Llama 3.1 405B model, which has demonstrated remarkable performance in diagnosing complex medical cases, on par with the well-known GPT-4. This achievement signals a shift in the potential role of open-source AI within the medical sector . In essence, the innovation in AI focuses not just on improving technological capabilities but also on enhancing the accessibility and customizability of these advanced systems, making them more adaptable to various healthcare settings.
One of the primary benefits that open-source AI models like Llama 3.1 offer is the promise of enhanced data privacy and customization. Unlike closed-source models, which require data to be processed externally, open-source systems can be tailored to operate within a healthcare provider’s existing IT infrastructure. This flexibility allows institutions to maintain strict control over their patients’ data, ensuring compliance with data protection regulations while simultaneously leveraging the diagnostic power of AI .
Learn to use AI like a Pro
Get the latest AI workflows to boost your productivity and business performance, delivered weekly by expert consultants. Enjoy step-by-step guides, weekly Q&A sessions, and full access to our AI workflow archive.
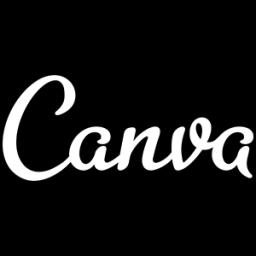
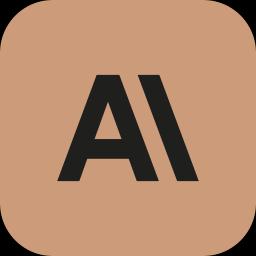
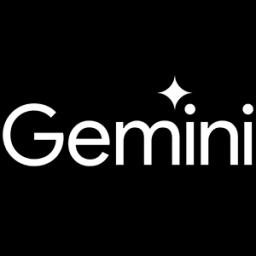
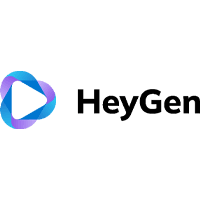
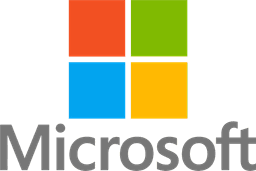
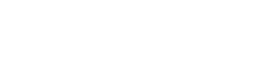
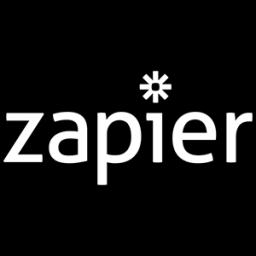
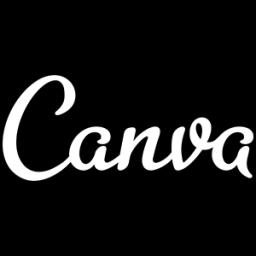
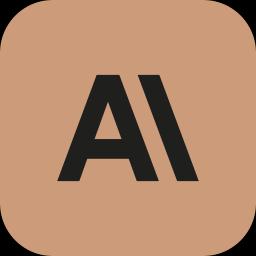
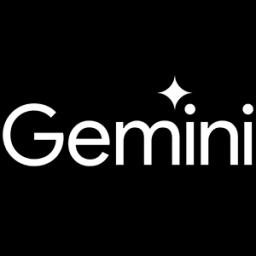
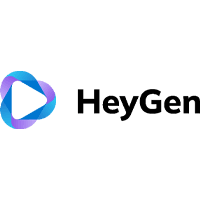
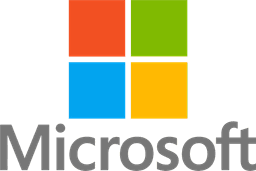
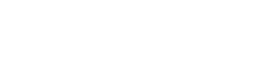
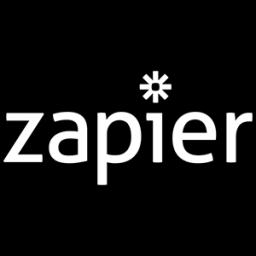
Furthermore, the inherent modifiability of open-source models encourages innovation at a grassroots level. Healthcare providers and developers can collaborate to refine AI algorithms to better suit specific medical contexts, driving advancements in diagnostic techniques and tools. This adaptability not only enhances the effectiveness of AI applications in varying medical scenarios but also plays a crucial role in fostering a community-driven evolution of AI capabilities .
The rise of open-source AI as a viable alternative to proprietary systems highlights a trend towards democratization in healthcare technology. This movement is particularly impactful in developing regions, where traditional AI solutions may be cost-prohibitive. By deploying open-source models, these areas gain access to state-of-the-art diagnostic tools that might otherwise be inaccessible due to financial constraints .
However, with great innovation comes the need for responsibility. The integration of AI into healthcare must be approached with caution to ensure ethical standards are maintained and potential risks are mitigated. This includes addressing challenges related to AI transparency and accountability, where the open-source community must establish rigorous validation frameworks to ensure the reliability of AI-driven diagnostics .
As open-source AI models continue to evolve, they may fundamentally alter the competitive dynamics in the AI industry. With improvements in efficiency and accuracy, open-source systems could challenge the dominance of proprietary models, leading to a rich, competitive landscape that benefits both developers and users. This evolution supports a more inclusive approach to AI development, breaking down barriers between organizations and enabling a collaborative future for AI technology .
Conclusion: The Role of AI in Healthcare
The integration of Artificial Intelligence (AI) in healthcare heralds a transformative era for medicine, promising enhancements in diagnostics, personalized treatment plans, and patient outcomes. AI algorithms, such as Llama 3.1, are driving significant advances in the accuracy and speed of diagnosing complex medical conditions. These algorithms have the potential to significantly reduce diagnostic errors, hence lowering patient mortality rates and healthcare costs . As AI continues to develop, its ability to handle large datasets will be crucial for advancing predictive analytics in healthcare, leading to more proactive and preventive care strategies.
Open-source AI models, like Llama 3.1, are changing the landscape of medical diagnostics by providing comparable accuracy to proprietary models such as GPT-4 . These models offer significant advantages in terms of cost-effectiveness, data privacy, and customizability. Healthcare organizations adopting these open-source solutions can tailor AI tools to better meet specific clinical requirements, ensuring that innovations in AI-driven diagnostics are aligned with particular health care needs and contexts.
Learn to use AI like a Pro
Get the latest AI workflows to boost your productivity and business performance, delivered weekly by expert consultants. Enjoy step-by-step guides, weekly Q&A sessions, and full access to our AI workflow archive.
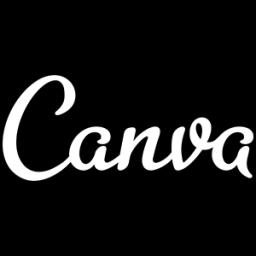
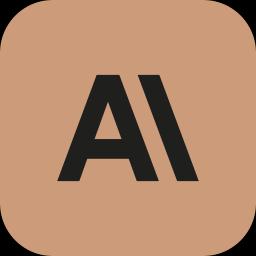
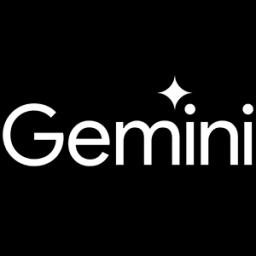
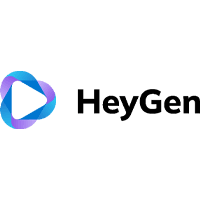
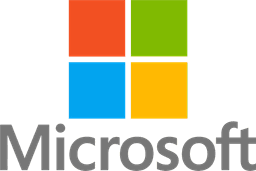
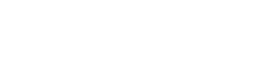
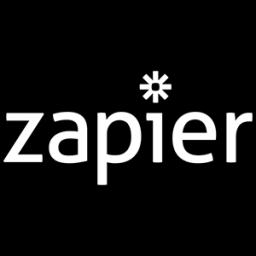
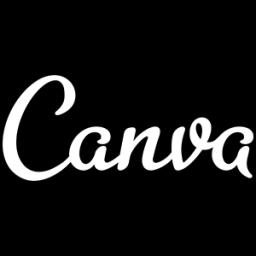
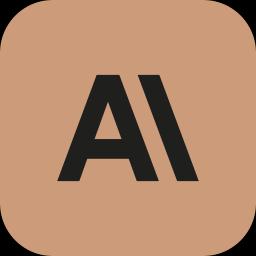
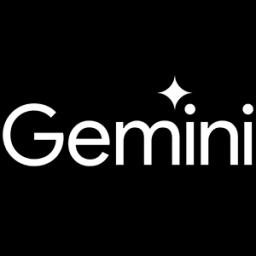
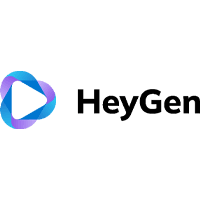
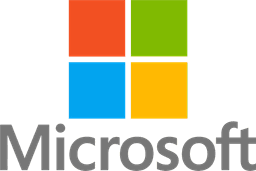
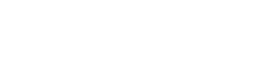
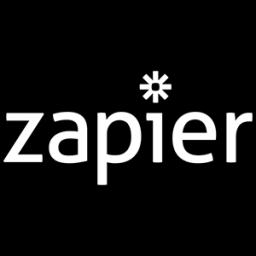
The rise of AI in healthcare is also a story of enhanced collaboration and innovation. Open-source models foster a global community of developers and researchers who contribute to ongoing improvements and adaptations, thereby accelerating the pace of technological advancements in medical diagnostics . With input from diverse perspectives and expertise, AI tools will continue to evolve, offering more precise and efficient solutions for complex medical challenges.
However, the role of AI in healthcare is not without challenges. The deployment of AI solutions necessitates robust ethical frameworks and governance to address potential issues such as data privacy and accountability. Moreover, healthcare professionals must be trained to effectively incorporate these technologies into their practice, ensuring that AI enhances rather than replaces the human elements essential in medical care . The integration of AI must be supplemented with continuous oversight and evaluation to navigate the ethical and practical considerations inherent in its use.
Ultimately, the burgeoning role of AI in healthcare stands to democratize medical expertise, making high-quality healthcare more accessible to diverse populations globally. By overcoming geographical and economic barriers, AI models like Llama 3.1 can play an instrumental role in delivering equitable healthcare solutions. The challenge will be to balance the technological possibilities of AI with the human-centric approach necessary for compassionate and effective medical care .