Diagnosis, Treatment, and Control in a Digital Age
LLMs and Tuberculosis: The AI Trio Battles Medical Queries
Last updated:

Edited By
Mackenzie Ferguson
AI Tools Researcher & Implementation Consultant
A new study explores how large language models (LLMs) like ChatGPT, Gemini, and Copilot handle medical questions about tuberculosis. While ChatGPT leads in information relevance, all models show weaknesses, particularly in source citation. Dive into how these AI marvels stand up to healthcare's crucial needs, examine their limitations, and foresee their impact on future medical practices.
Introduction to Large Language Models in Medicine
The introduction of Large Language Models (LLMs) into the realm of medicine presents a promising frontier for enhancing healthcare delivery and patient outcomes. With the exponential growth of technology, these models like ChatGPT, Gemini, and Copilot are poised to revolutionize medical practice, particularly in areas requiring vast information synthesis, such as diagnosing complex diseases like tuberculosis (TB). A recent study published in Nature highlights that LLMs can significantly assist in answering medical queries, offering support in TB diagnosis, treatment, and management by processing extensive datasets and providing accessible insights to both practitioners and patients.
In evaluating the performance of these models, the study found that each has its unique strengths and weaknesses. For instance, ChatGPT was noted for its relevance in information delivery, albeit with shortcomings in source citation and timeliness, reinforcing the need for continuous updates and auditing processes. Similarly, Gemini stood out in the TB prevention and control domain, yet it struggled somewhat with treatment specifics, requiring further refinement and adaptation to the rapidly evolving medical knowledge landscape.
Learn to use AI like a Pro
Get the latest AI workflows to boost your productivity and business performance, delivered weekly by expert consultants. Enjoy step-by-step guides, weekly Q&A sessions, and full access to our AI workflow archive.
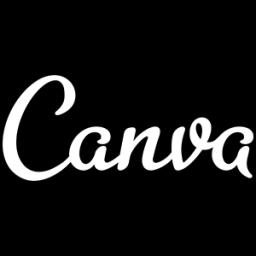
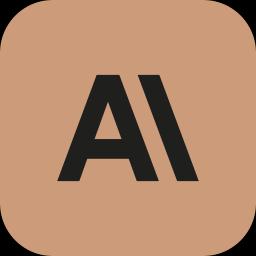
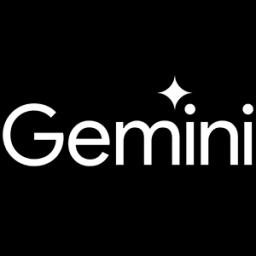
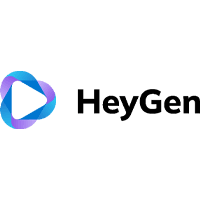
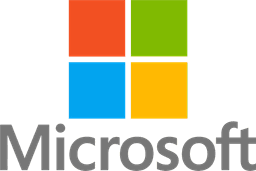
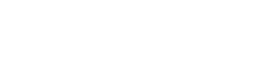
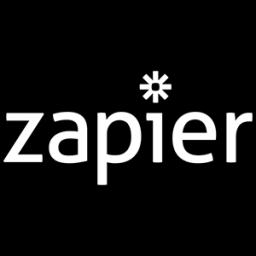
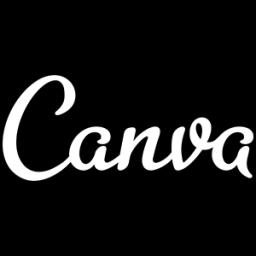
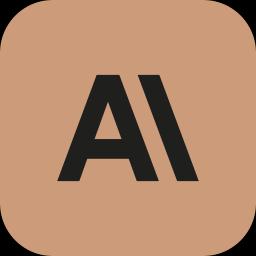
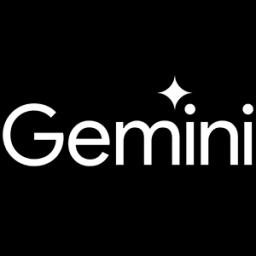
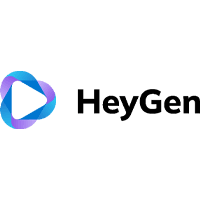
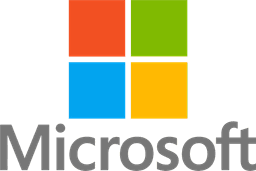
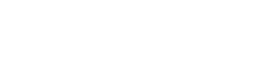
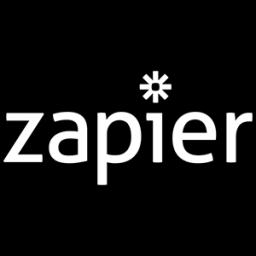
A critical aspect of deploying LLMs in medicine is understanding their limitations and addressing them to ensure reliability and trustworthiness. As noted in the study, models like Copilot, although offering some benefits, lag behind in areas such as disease management. Such insights underscore the importance of iterative development and rigorous evaluation against real-world scenarios to fine-tune their application in clinical settings. Furthermore, integrating robust citation frameworks and mechanisms to report uncertainties could enhance their credibility among healthcare professionals and patients alike.
Evaluating LLM Performance in Tuberculosis Management
The evaluation of Large Language Models (LLMs) in the management of tuberculosis is a crucial step in harnessing the potential of artificial intelligence in healthcare. According to a comprehensive study published by *Nature*, these models were scrutinized for their ability to address tuberculosis management questions, covering critical areas such as diagnosis, treatment, disease management, and prevention and control. In this evaluation, ChatGPT emerged as the most effective model overall, demonstrating superior capabilities in delivering relevant information [1](https://www.nature.com/articles/s41598-025-03074-9). However, it wasn’t without its limitations, as it often failed to provide appropriate source citations and to express uncertainties in its responses, which are essential for maintaining credibility in medical information.
On the other hand, Gemini showed exceptional performance in the domain of prevention and control of tuberculosis, making it a valuable tool in public health strategies aimed at disease spread mitigation [1](https://www.nature.com/articles/s41598-025-03074-9). Its focus on preventive measures aligns closely with global health initiatives which emphasize the prevention of disease as a cost-effective health strategy. Meanwhile, Copilot was noted for its struggles particularly in the field of disease management, highlighting a significant gap where improvement is needed. Its shortcomings in providing comprehensive management solutions indicate the necessity for further refinement and robust training using diverse medical databases to enhance accuracy and applicability across healthcare settings [1](https://www.nature.com/articles/s41598-025-03074-9).
Evaluating LLMs within the context of tuberculosis is pivotal not only because of the historical and ongoing global impact of this infectious disease but also due to the critical nature of accessible and accurate medical information. Tuberculosis remains a leading cause of mortality worldwide, affecting millions [1](https://www.nature.com/articles/s41598-025-03074-9). The ability of LLMs to facilitate better management of tuberculosis cases through enhanced diagnostic and treatment support can revolutionize how healthcare is delivered, particularly in regions with limited access to medical expertise and resources.
Learn to use AI like a Pro
Get the latest AI workflows to boost your productivity and business performance, delivered weekly by expert consultants. Enjoy step-by-step guides, weekly Q&A sessions, and full access to our AI workflow archive.
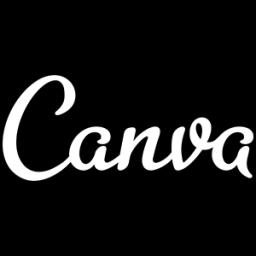
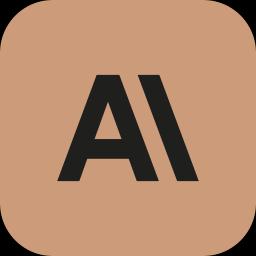
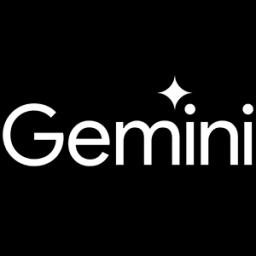
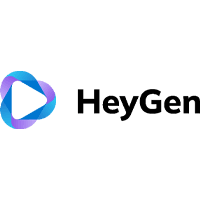
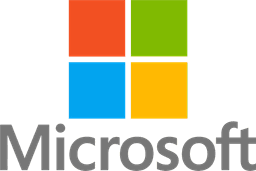
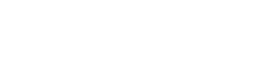
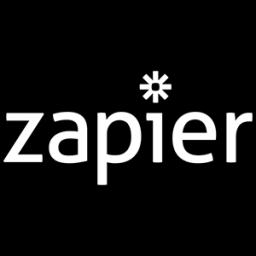
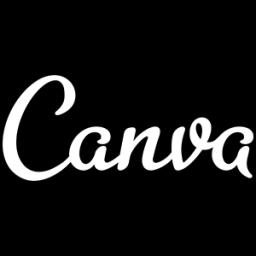
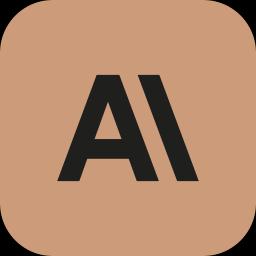
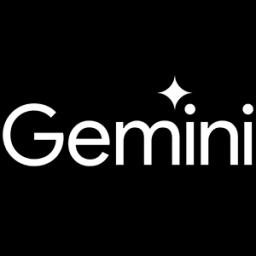
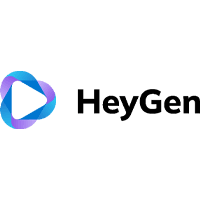
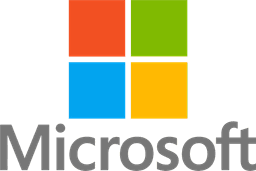
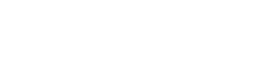
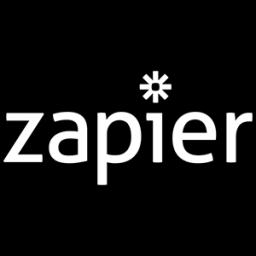
Further insights into the limitations of LLMs revealed by the study underscore the importance of addressing challenges associated with data transparency and the articulation of medical uncertainties. Efforts to improve the reliability of medical information delivered by these models could include integrating real-time data updates, enhancing source citation practices, and employing language that clearly indicates confidence levels and associated risks [1](https://www.nature.com/articles/s41598-025-03074-9). Such improvements would not only enhance the accuracy of these models but also build greater trust among healthcare professionals and patients alike.
Comparison of ChatGPT, Gemini, and Copilot
In a comparative analysis of ChatGPT, Gemini, and Copilot, each Large Language Model (LLM) demonstrates unique strengths and weaknesses, particularly in the context of addressing medical questions related to tuberculosis. ChatGPT consistently outperformed its peers in delivering relevant information across various domains, though it fell short in citing its sources and lacked temporal accuracy, which is crucial for medical information. Meanwhile, Gemini shone in the area of prevention and control, an aspect vital for managing the spread of tuberculosis. However, it didn't perform as well in handling treatment queries, possibly limiting its usefulness in certain clinical scenarios. Copilot, on the other hand, struggled the most with disease management, a critical component for patient care and health outcomes, highlighting a need for further enhancement in this area [Nature Article].
The assessment of these models underscores an essential aspect of artificial intelligence in health care – the balance between data processing capabilities and the reliability of the information provided. The reliance on these models requires a robust framework that addresses their current limitations such as insufficient source attribution and the handling of uncertain or ambiguous medical situations. Integrating more comprehensive databases and updating mechanisms could enhance their reliability. Furthermore, improvements in how these models document confidence levels in their responses could play a significant role in their effectiveness in a clinical setting [Nature Article].
The significance of evaluating LLMs like ChatGPT, Gemini, and Copilot in the framework of tuberculosis extends beyond academic interest. Tuberculosis remains a global health challenge, and the potential of these models to provide accurate, timely, and actionable information could significantly impact patient outcomes. Their evaluation is critical as it could guide the development and implementation of AI tools in effectively managing this infectious disease. However, the outcomes of this evaluation also emphasize caution, underscoring the necessity for healthcare professionals to scrutinize these tools' results thoroughly and ensure they are aligned with established medical guidelines before applying them in clinical decision-making [Nature Article].
Moreover, the study's findings have broader implications for the role of AI in healthcare. While LLMs offer promise in enhancing patient education and supporting clinical decisions, the choice of model is crucial. Each model's unique strengths and weaknesses mean that thorough evaluation and understanding of their capabilities are essential for their successful implementation. For patients, this means that while LLMs can offer accessible medical information, they should never replace professional medical advice. Always consulting healthcare professionals ensures that the guidance they receive is comprehensive and tailored to their specific health needs [Nature Article].
In summary, the ongoing refinement and validation of LLMs for tuberculosis-related medical inquiries will play an integral role in shaping future healthcare solutions. The value they add hinges on their continuous improvement, particularly in areas such as source transparency and uncertainty management. As AI continues to grow its footprint in healthcare domains, embracing these tools comes with responsibilities for both technology developers and users to ensure these models enhance and not hinder the quality of care provided [Nature Article].
Learn to use AI like a Pro
Get the latest AI workflows to boost your productivity and business performance, delivered weekly by expert consultants. Enjoy step-by-step guides, weekly Q&A sessions, and full access to our AI workflow archive.
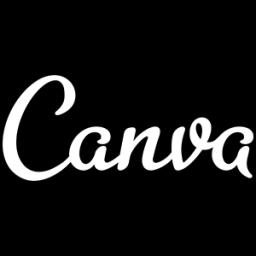
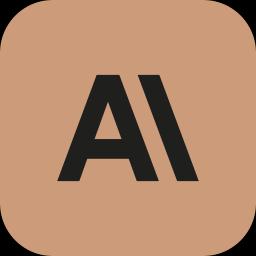
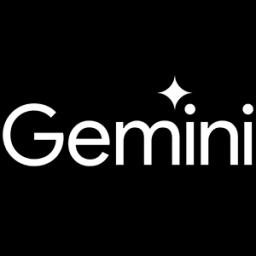
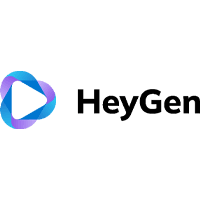
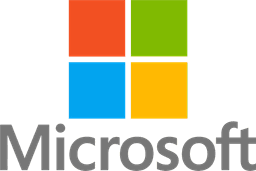
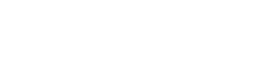
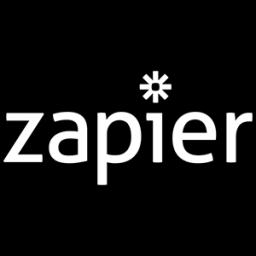
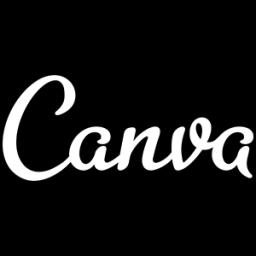
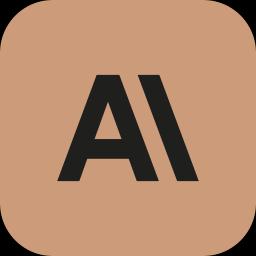
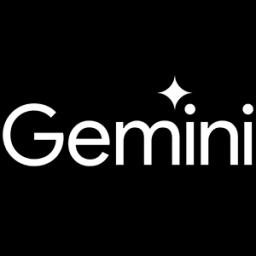
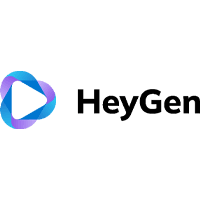
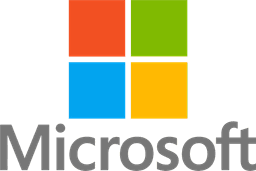
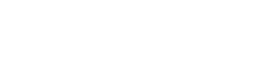
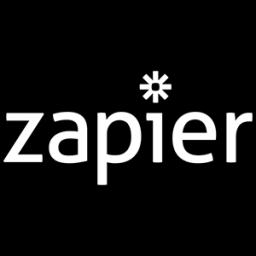
Strengths and Weaknesses of LLMs
Large Language Models (LLMs) have been at the forefront of advancements in artificial intelligence, demonstrating both strengths and weaknesses in diverse applications. One of the notable strengths of LLMs is their ability to process and generate human-like text based on vast amounts of data. This capability has proven especially beneficial in answering complex medical questions, where LLMs like ChatGPT have excelled in delivering relevant information consistently across various domains, including diagnosis and treatment [1](https://www.nature.com/articles/s41598-025-03074-9).
However, LLMs also exhibit significant weaknesses, particularly in the areas of source citation and transparency. Despite their adeptness in generating coherent responses, these models often fail to acknowledge the limitations and uncertainties inherent in their outputs. The lack of citation for the sources of their information can lead to questions about the reliability and trustworthiness of the content they produce [1](https://www.nature.com/articles/s41598-025-03074-9).
For instance, in the context of tuberculosis management, the study found that while ChatGPT performed highly across multiple aspects, it struggled with adequately citing sources and dating the information provided. This issue is compounded by the fact that such models are trained on data that may not always be up-to-date or free from biases, potentially leading to misinformation and perpetuating existing disparities in healthcare [1](https://www.nature.com/articles/s41598-025-03074-9).
Moreover, the evaluation of other LLMs such as Gemini and Copilot revealed nuanced strengths and weaknesses. Gemini outperformed in prevention and control but was less effective in treatment scenarios, whereas Copilot faced challenges in disease management [1](https://www.nature.com/articles/s41598-025-03074-9). These discrepancies highlight the need for continuous evaluation and refinement of LLMs to ensure they provide accurate, reliable, and fair information, especially in critical fields like healthcare.
To address these weaknesses, several improvements have been proposed. Incorporating robust mechanisms for regular updates, implementing better source citation, and providing clearer indications of uncertainties in responses are essential steps forward. Additionally, integrating structured data from reputable medical databases and utilizing functionalities that highlight the date of information and specify confidence levels could further enhance the credibility and utility of LLMs in medical information delivery [1](https://www.nature.com/articles/s41598-025-03074-9).
Importance of LLM Evaluation in Tuberculosis Context
The evaluation of Large Language Models (LLMs) in the tuberculosis context is pivotal for several reasons. Tuberculosis, being one of the top infectious killers worldwide, requires precise and timely information dissemination to manage effectively. Studies underscore that LLMs, like ChatGPT, Gemini, and Copilot, can potentially bridge gaps in information accessibility, providing real-time support in understanding diagnosis, treatment, and prevention measures. However, it's crucial to recognize the limitations observed in these models, such as insufficient source citation and ambiguity in data interpretation, which could lead to misinformation and affect patient safety. By evaluating LLMs, healthcare providers and policymakers can harness their capabilities while mitigating risks, leading to improved healthcare delivery. This ensures that such technologies significantly contribute to global TB control efforts, aligning with goals set by workshops like the "2nd Artificial Intelligence in Infectious Diseases Workshop 2025" that emphasizes AI's role in disease management.
Learn to use AI like a Pro
Get the latest AI workflows to boost your productivity and business performance, delivered weekly by expert consultants. Enjoy step-by-step guides, weekly Q&A sessions, and full access to our AI workflow archive.
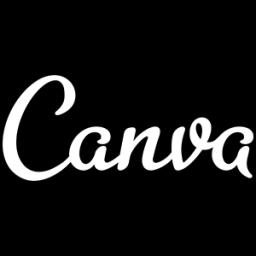
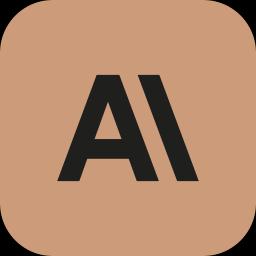
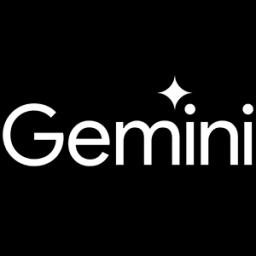
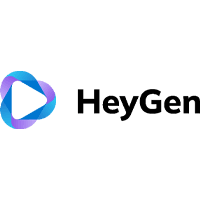
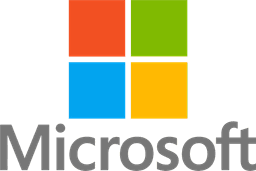
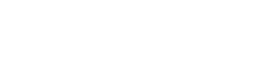
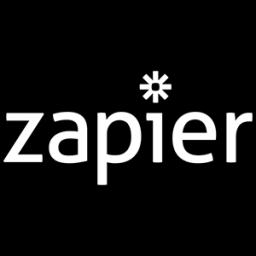
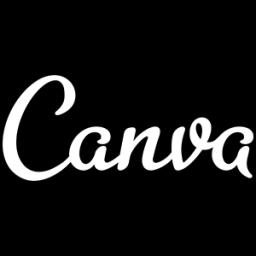
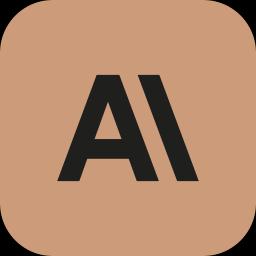
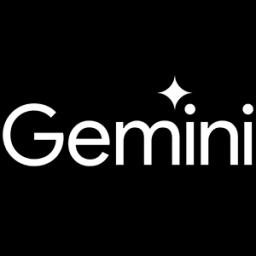
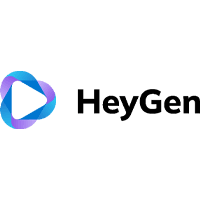
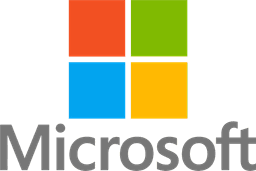
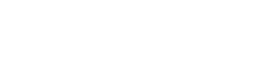
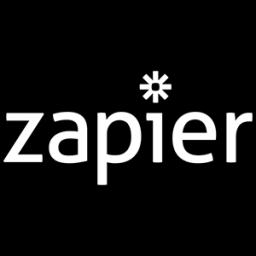
Evaluation Tools: DISCERN-AI and NLAT-AI
In the rapidly advancing field of artificial intelligence, two tools—DISCERN-AI and NLAT-AI—are making significant strides in evaluating Large Language Models (LLMs), particularly in the medical domain. DISCERN-AI, renowned for its structured approach, evaluates information based on quality criteria including reliability, accuracy, and transparency. This ensures that data provided by LLMs is not only factual but presented in a format conducive to informed decision-making. Meanwhile, NLAT-AI takes a more nuanced approach by analyzing the natural language aspects of responses. It assesses coherence, relevance, and logical flow, thus ensuring that LLMs offer information that is not only correct but also contextually suitable [^1^](https://www.nature.com/articles/s41598-025-03074-9).
These tools are particularly relevant in the study of tuberculosis-related queries managed by LLMs like ChatGPT, Gemini, and Copilot. The unique combination of DISCERN-AI’s structured assessment criteria and NLAT-AI’s language evaluation capabilities provides a comprehensive framework for systematically scrutinizing the responses generated by these models. For example, where ChatGPT excels in relevance, its minor deficiencies in source citation are highlighted by DISCERN-AI, enabling precise areas of improvement to be identified [^1^](https://www.nature.com/articles/s41598-025-03074-9).
DISCERN-AI and NLAT-AI not only identify the strengths and weaknesses of different LLMs but also guide modifications that can enhance their reliability and usefulness in medical contexts. The efficiency of these tools is paramount, especially as AI continues to evolve and integrate with healthcare systems worldwide. They underscore the importance of continual assessment and refinement in AI tools to address challenges like insufficient source citation and failure to acknowledge uncertainties, as demonstrated in the assessment of tuberculosis management by LLMs [^1^](https://www.nature.com/articles/s41598-025-03074-9).
Addressing LLM Limitations in Medical Information Delivery
With the increasing use of Large Language Models (LLMs) such as ChatGPT, Gemini, and Copilot in medical domains, addressing their limitations in delivering medical information, especially in conditions like tuberculosis, has become critically important. A study published in Nature evaluates how these models perform in answering complex medical questions. ChatGPT, for instance, is noted for having the highest overall accuracy and relevance, yet it faces significant shortcomings with source citation and dating. Meanwhile, Gemini excels in prevention and control aspects but is less adept in treatment-related queries. Copilot, on the other hand, particularly struggles with disease management. These issues underscore an urgent need for more robust frameworks that enhance transparency and credibility in AI-generated medical responses.
One proposed method to overcome these shortcomings involves integrating comprehensive referencing and uncertainty acknowledgment systems within LLMs. Such mechanisms can significantly improve the quality of the information delivered to healthcare professionals and patients. By incorporating reliable and regularly updated data sources, these models can provide more accurate and timely medical advice. This is fundamental, especially in the context of tuberculosis, a disease that requires quick adaptation to the latest research findings on treatment and diagnostics. The insights from the study align with recommendations for enhancing medical LLMs, including systematic updates, elaborated source citations, and clear indicators of any associated uncertainties in the provided data.
The implications of these limitations extend beyond purely informational aspects. The integration of AI in healthcare, if not managed with due diligence, could potentially lead to a widening of health disparities, especially in regions with limited access to robust digital infrastructure. Ensuring equitable access to improved AI tools is essential to avoid such disparities. Moreover, as highlighted by the ethics-focused discussions from the IBA article, incorporating diverse training data and enabling substantial human oversight can help mitigate some of the biases and challenges inherent in AI systems. This approach not only improves the reliability of medical advice provided by LLMs but also helps in maintaining the critical human touch in healthcare delivery.
Learn to use AI like a Pro
Get the latest AI workflows to boost your productivity and business performance, delivered weekly by expert consultants. Enjoy step-by-step guides, weekly Q&A sessions, and full access to our AI workflow archive.
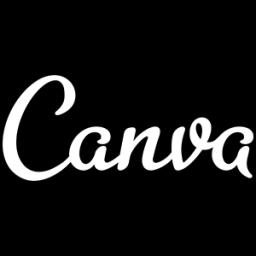
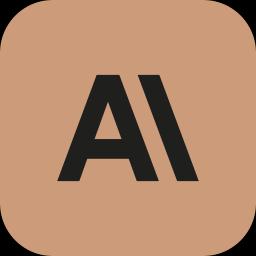
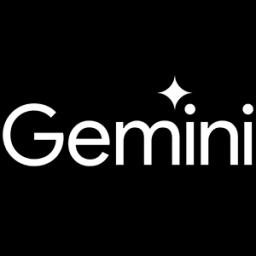
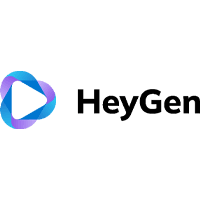
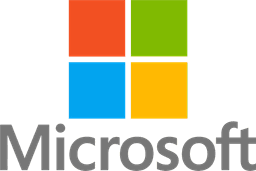
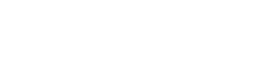
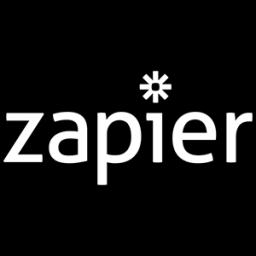
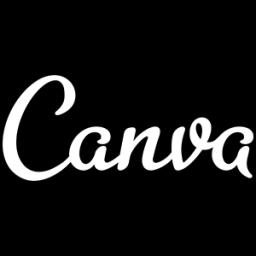
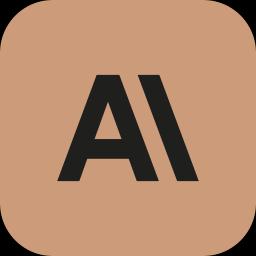
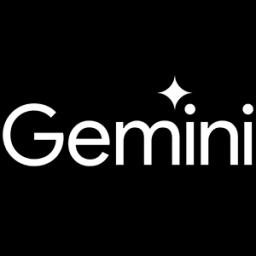
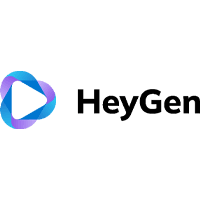
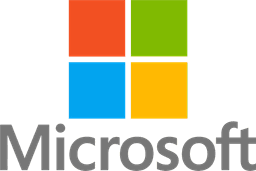
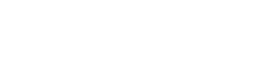
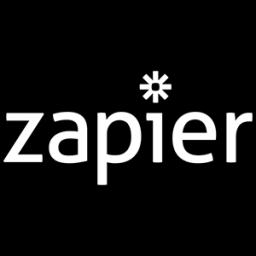
Implications for Healthcare Professionals and Patients
The findings from the study on Large Language Models (LLMs) present profound implications for healthcare professionals and patients alike, revolutionizing their approach to tuberculosis treatment and management. For healthcare professionals, these models can serve as auxiliary tools offering quick access to a vast repository of medical information on tuberculosis diagnosis, treatment options, and preventive measures. By integrating these AI-driven insights into clinical practice, healthcare providers can enhance their decision-making processes and improve patient outcomes. However, it is crucial for these professionals to scrutinize the results generated by LLMs critically, understanding their limitations in source authenticity and acknowledgement of uncertainties. This ensures that the medical advice remains safe, reliable, and beneficial to patients who depend on them for accurate information (source).
For patients, the availability of LLMs offers an unprecedented level of access to detailed and understandable medical knowledge, potentially demystifying tuberculosis treatment processes and empowering them to take active roles in their healthcare journey. By using information from these models, patients can engage more effectively with healthcare providers, ask informed questions, and better adhere to treatment protocols. Nonetheless, it is imperative that patients remain skeptical of the AI-generated outputs due to their occasional shortcomings in transparency and potential for misinformation. Always cross-verifying LLM insights with professional medical advice is the key to leveraging the best of both worlds - the speed of AI and the depth of human empathy and expertise. This balanced approach can significantly enhance the patient-care experience while safeguarding against potential AI-related risks (source).
Furthermore, this study underlines a larger paradigm shift where the role of healthcare professionals might evolve from being sole providers of information to becoming facilitators of a more interactive and informed consultation process. It reflects a collaborative dynamic between AI tools and medical experts where the focus is on optimizing healthcare delivery rather than replacing the human element. Such integration fosters an environment where healthcare practitioners can dedicate more time to complex cases by offloading routine information tasks to LLMs, ultimately advancing comprehensive and efficient patient care. As the reliance on these models grows, maintaining a robust mechanism for evaluating their outputs continuously becomes imperative to ensure quality control and reliability in real-world medical applications (source).
Economic Impacts of LLM Integration in Healthcare
The integration of Large Language Models (LLMs) in healthcare, particularly in tuberculosis treatment and management, presents both opportunities and challenges from an economic perspective. Initially, the implementation of LLM-based systems in healthcare settings requires significant investment. This includes costs associated with infrastructure development, acquiring comprehensive datasets, and conducting training programs for healthcare professionals who will work alongside these technologies. Despite these upfront expenses, the long-term financial benefits may outweigh the costs. By automating routine tasks such as diagnostic image analysis and report generation, LLMs can enhance efficiency, allowing healthcare workers to focus on more complex cases. This shift not only improves workflow but can also lead to a reduction in labor costs and a decrease in patient time spent in hospitals, ultimately curbing overall healthcare expenditures. [1]
Furthermore, the pharmaceutical sector might experience shifts in demand due to LLMs enhancing diagnostic accuracy and treatment timeliness. As these models aid in faster and more precise diagnosis, there could be an increased need for novel treatments, thereby stimulating pharmaceutical innovation and production. However, while the economic benefits are notable, there is a risk that LLM integration could exacerbate existing disparities in healthcare access. Not every healthcare facility may afford these technologies, potentially widening the gap between advanced and resource-limited settings. Addressing this inequity is crucial to ensure that the economic advantages of LLMs are distributed evenly across different populations. [4]
Social Consequences of LLM Use in TB Care
The integration of Large Language Models (LLMs) in tuberculosis (TB) care represents a pivotal shift in how patients may access and interact with medical information. One of the significant social consequences is the empowerment of patients through increased access to information. With models like ChatGPT and Gemini being evaluated for their proficiency in understanding and conveying medical knowledge about TB, patients are able to access information that was previously more difficult to obtain. This access can lead to better informed decision-making in their treatment regimen, potentially improving adherence and health outcomes. However, the study notes that these models sometimes lack in citing sources and indicating uncertainties, which can be problematic if patients take the AI-generated content at face value without consulting healthcare professionals for confirmation (Nature).
Learn to use AI like a Pro
Get the latest AI workflows to boost your productivity and business performance, delivered weekly by expert consultants. Enjoy step-by-step guides, weekly Q&A sessions, and full access to our AI workflow archive.
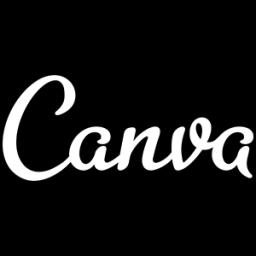
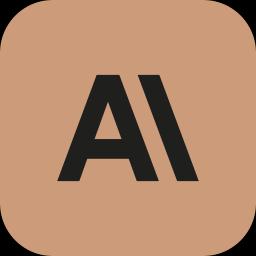
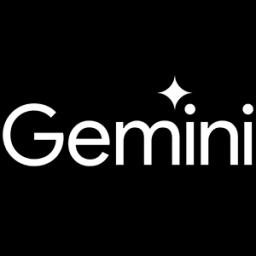
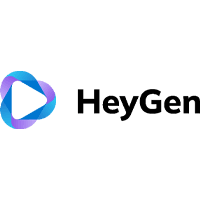
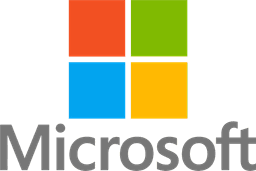
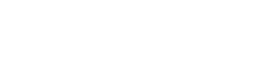
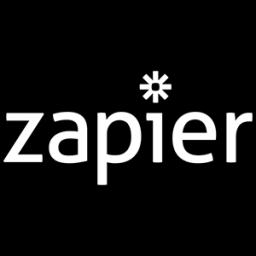
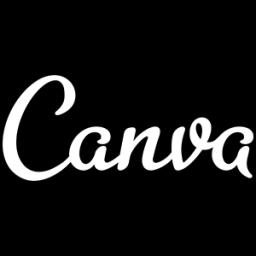
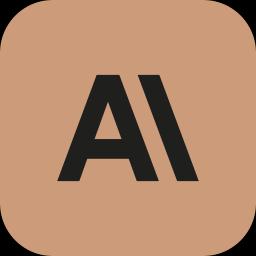
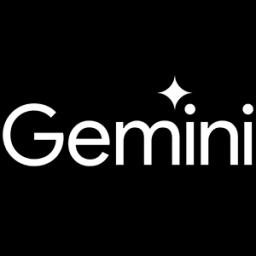
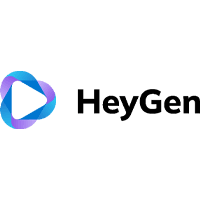
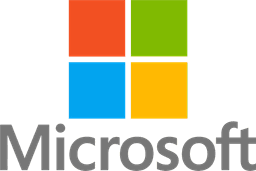
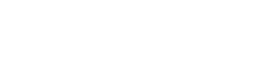
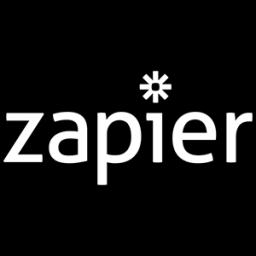
Despite the potential benefits, the use of LLMs in TB care could contribute to the dissemination of misinformation. If models disseminate biased or erroneous information, it might exacerbate existing health inequities. Particularly in communities with limited access to healthcare resources, the trust in LLMs might lead to skewed patient decisions based on inaccurate data. Thus, there is a need for continuous improvement in LLMs to ensure they provide unbiased, accurate information and integrate a system of checks and balances that includes healthcare practitioner oversight. This aligns with discussions from global health meetings, like the "2nd Artificial Intelligence in Infectious Diseases Workshop 2025," which highlights AI's potential and challenges in healthcare settings (AI in Infectious Diseases Workshop).
Moreover, the increasing reliance on LLMs could undermine the traditional doctor-patient relationship. As AI tools become ingrained in medical inquiry and patient interaction, the face-to-face discussions that often serve to reassure patients might diminish. This transformation could shift how patients perceive trust and care, potentially relying more on AI's seemingly objective viewpoints rather than human judgment that includes empathy and nuanced understanding. This change reflects broader ethical discussions in AI integration into healthcare, emphasizing the need for balancing AI benefits with human interaction, as outlined in various reports on AI in healthcare (IBA Article).
Political and Regulatory Considerations for LLMs
The growing integration of large language models (LLMs) like ChatGPT and Gemini into healthcare highlights the urgent need for comprehensive political and regulatory frameworks. With the ability to influence medical decision-making, these models need careful oversight to ensure accuracy and safety. Regulatory bodies must develop guidelines that address the ethical concerns highlighted in AI usage within healthcare (source). Establishing robust data privacy protocols and ensuring algorithmic transparency are crucial steps. Moreover, guidelines for bias mitigation must be solidified to protect against inequitable healthcare outcomes, which is a primary concern when deploying AI technologies (source).
International collaboration will play a pivotal role in shaping the future of LLMs in healthcare. Joint efforts between countries and international health organizations can lead to the development of standardized regulations that ensure equitable access and application of LLMs across diverse healthcare systems (source). Such collaborations can facilitate the sharing of best practices and the establishment of benchmarks for AI deployment. This is particularly relevant for managing infectious diseases such as tuberculosis, where prompt and accurate information dissemination is essential (source).
The potential for misuse, such as creating fraudulent medical documentation using LLMs, necessitates the enforcement of strict regulatory mechanisms. Without stringent oversights, there is a risk of undermining public trust in AI-driven health solutions. A global consensus is essential to address these challenges, ensuring that LLMs augment rather than detract from the quality of healthcare. Regulatory bodies must also ensure that innovations in AI applications do not widen existing healthcare disparities by ensuring fair distribution and access to technology (source).
In summary, while LLMs offer promising advancements in healthcare, particularly in handling diseases like tuberculosis, their integration demands vigilant regulatory oversight to safeguard public health interests. Creating legal and ethical boundaries will not only foster trust but will also enhance the reliability and efficacy of AI applications in medical contexts. Consequently, coherent and cooperative international policies are critical for managing the potential economic, social, and political impacts of LLMs in healthcare (source).
Learn to use AI like a Pro
Get the latest AI workflows to boost your productivity and business performance, delivered weekly by expert consultants. Enjoy step-by-step guides, weekly Q&A sessions, and full access to our AI workflow archive.
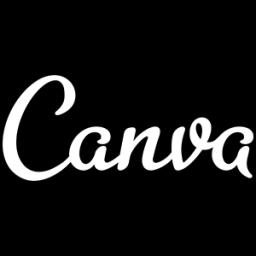
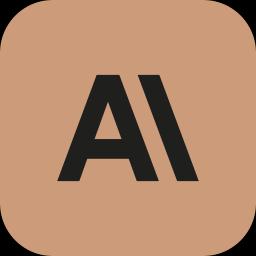
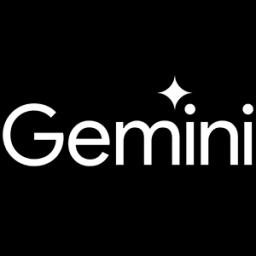
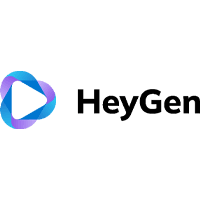
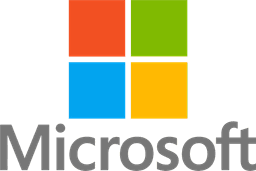
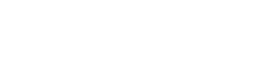
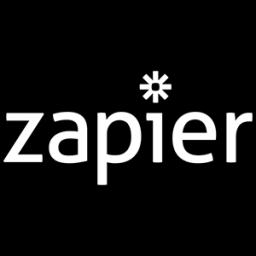
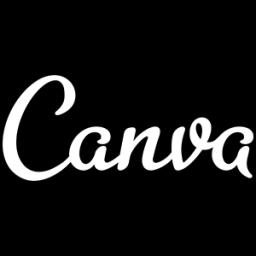
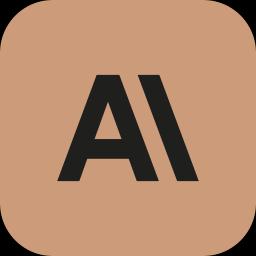
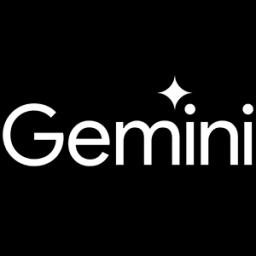
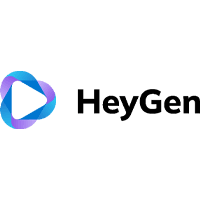
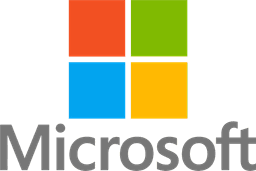
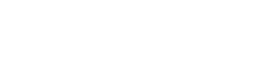
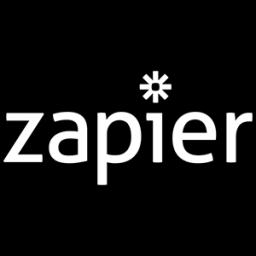
Future Directions in AI and Infectious Disease Management
As artificial intelligence (AI) technology rapidly evolves, its applications in healthcare, particularly in infectious disease management, are gaining momentum. The ability of large language models (LLMs) such as ChatGPT to address complex medical inquiries provides a glimpse into the future of healthcare, where AI could play a pivotal role in diagnosis, treatment, and patient education. The study reported in *Scientific Reports* underscores LLMs' potential in managing diseases like tuberculosis by evaluating their capacity to respond to relevant medical queries .
Looking forward, the incorporation of LLMs and other AI-driven tools in managing infectious diseases will likely hinge on addressing current limitations. As noted in the research, improvements in source citation and the management of data uncertainties are critical to enhancing the reliability and trustworthiness of AI-generated medical information . Such advancements could facilitate their broader integration into health services, allowing for more precise disease monitoring, prevention strategies, and treatment plans .
AI's role in infectious disease management is further highlighted by ongoing advancements in tuberculosis treatment. Recent reports emphasize innovations such as faster diagnostic methods and novel therapies, which signify the groundbreaking potential AI systems may have when they can effectively collaborate with these advancements . The integration of AI in these processes not only aims to enhance outcomes but also seeks to democratize healthcare by providing equitable access to advanced medical technologies .
However, the transition to an AI-augmented healthcare system is not without its challenges. Ethical considerations, especially regarding AI bias and data privacy, remain at the forefront of discussions. As outlined in the *IBA* article, the necessity for diverse training data and robust oversight mechanisms is paramount to avoid discriminatory outcomes . Ensuring that AI tools are transparent in their processes and outcomes is integral to maintaining public trust and achieving sustainable integration into infectious disease management.
In the political realm, the globalization of AI's application in healthcare calls for international collaboration to establish ethical standards and regulatory frameworks. The World Health Organization has accentuated the need for such guidelines to ensure that AI's integration does not exacerbate existing health inequities but instead promotes universal healthcare access . As AI continues to transform healthcare landscapes, these frameworks will be crucial in guiding future innovations and ensuring responsible AI deployment in managing infectious diseases.