Revolutionizing AI: Fairness Meets Precision
MIT's New Technique Tackles AI Bias While Boosting Accuracy!
Last updated:

Edited By
Mackenzie Ferguson
AI Tools Researcher & Implementation Consultant
MIT researchers have unveiled an innovative method to combat bias in AI models, cleverly enhancing accuracy without compromising data integrity. This breakthrough targets unfair predictions linked to underrepresented groups, significantly refining AI fairness initiatives.
Introduction to Bias in AI Models
Artificial intelligence (AI) has transformed various industries, but its susceptibility to biases has raised concerns about fairness and equity. Biases often occur when AI models make skewed predictions for certain subgroups due to imbalanced training datasets. For instance, a model trained predominantly on data from male patients may produce inaccurate predictions for female patients. Thus, addressing bias in AI models has become a pressing issue in developing responsible AI solutions.
In response to these concerns, researchers at MIT have pioneered a novel technique designed to mitigate bias in AI models while maintaining, or even improving, their accuracy. This technique focuses on identifying and removing specific training examples that contribute most significantly to a model's inaccurate predictions for minority subgroups.
Learn to use AI like a Pro
Get the latest AI workflows to boost your productivity and business performance, delivered weekly by expert consultants. Enjoy step-by-step guides, weekly Q&A sessions, and full access to our AI workflow archive.
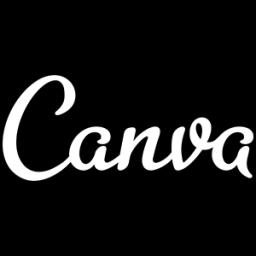
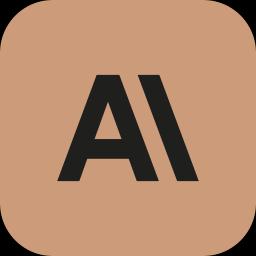
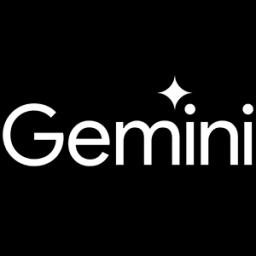
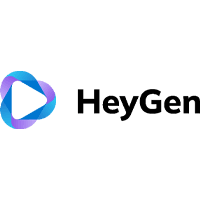
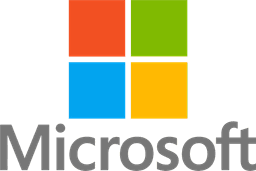
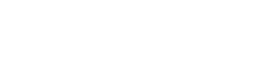
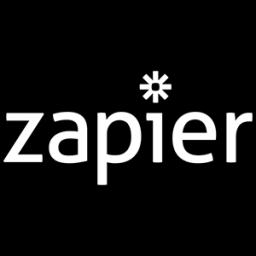
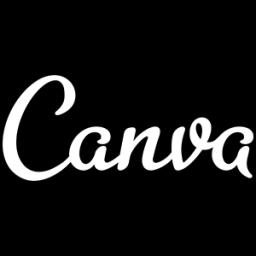
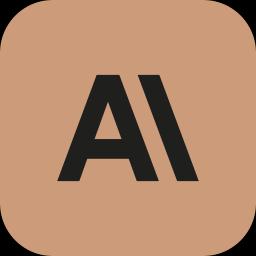
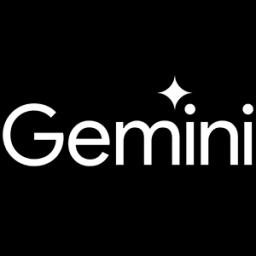
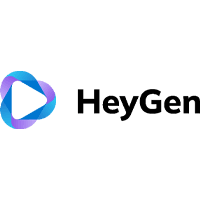
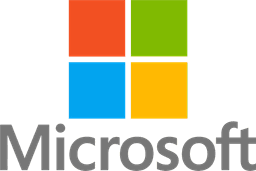
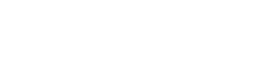
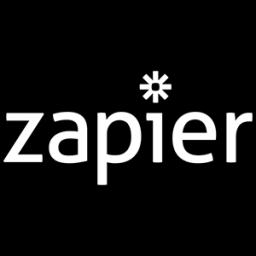
The innovative approach leverages a method known as TRAK (Training example Removal for Augmented Knowledge), which targets and eliminates training samples that adversely affect model performance on underserved subgroups. Consequently, this adjustment ensures that the model's accuracy for these groups is preserved or enhanced without the need for extensively rebalancing the entire dataset.
One of the crucial advantages of this method is its applicability to datasets lacking explicit subgroup labels—a common scenario in fields like healthcare, where equity in AI outcomes is a critical concern. By improving the performance of AI models on minority subgroups, researchers aim to foster fairer and more reliable AI systems across various applications.
The MIT Breakthrough: Reducing Bias While Improving Accuracy
The Massachusetts Institute of Technology (MIT) remains at the forefront of artificial intelligence innovation, with its latest breakthrough addressing the pervasive issue of bias within AI models. This new technique does not just preserve the integrity of AI predictions; it enhances them by focusing on reducing biases that skew results against minority or underserved subgroups within datasets. Traditional methods tried to balance datasets in broad strokes, often removing large amounts of data and occasionally sacrificing accuracy. MIT’s approach is different as it identifies and selectively removes training examples most guilty of introducing bias, thus preserving, if not improving, the model’s accuracy while fostering fairness.
At the heart of MIT’s technique is a methodology known as TRAK, which plays a pivotal role in this innovation. TRAK allows researchers to precisely identify which training examples skew model predictions detrimentally towards minority subgroups. By pruning these off from the model's training data, the research team could retrain AI models, achieving enhanced fairness without a corresponding drop in accuracy. This is particularly crucial for applications where data often lacks explicit subgroup labels—like in healthcare, where ensuring fairness across diverse patient demographics is paramount.
Learn to use AI like a Pro
Get the latest AI workflows to boost your productivity and business performance, delivered weekly by expert consultants. Enjoy step-by-step guides, weekly Q&A sessions, and full access to our AI workflow archive.
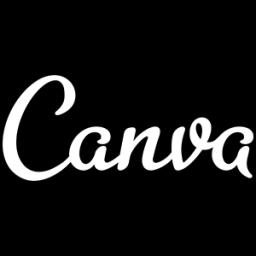
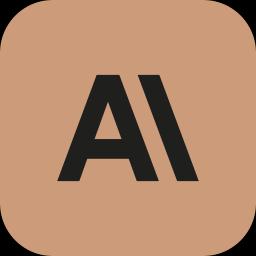
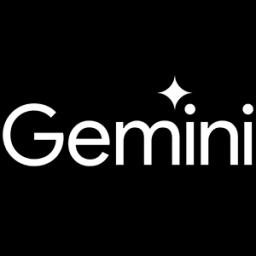
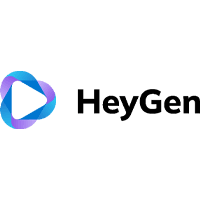
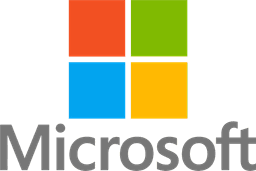
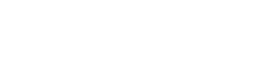
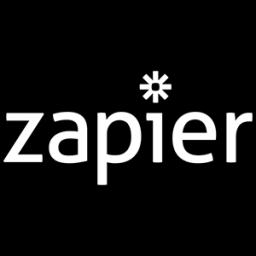
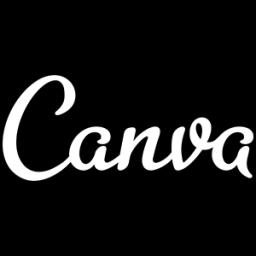
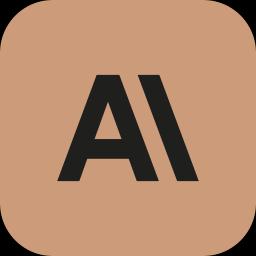
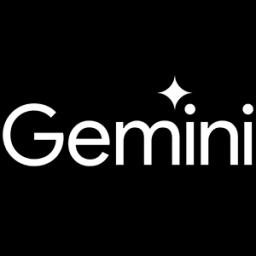
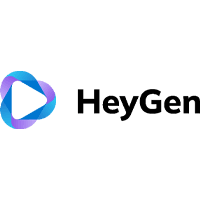
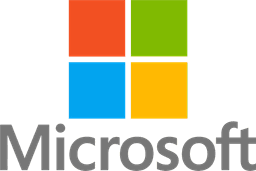
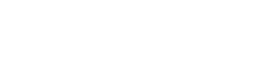
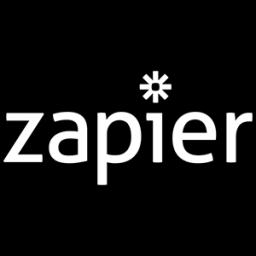
When compared to more conventional approaches to bias mitigation, MIT’s method stands out. Unlike other techniques that require systematically tweaking and often overhauling entire datasets—such as through oversampling or complex data balancing—MIT's method requires far fewer adjustments. It circumvents the need for exhaustive data exclusion and often achieves more effective results across multiple datasets. Challenges remain, however, such as the difficulty in intuitively detecting biases that aren't anchored by predefined subgroups, which the research team is actively working to address.
What makes this technique not only innovative but also broadly applicable is its practicality in real-world scenarios. For example, healthcare AI applications benefit significantly since this methodology can audit and fine-tune training data where subgroup labels aren't readily apparent, ensuring that predictions are both accurate and equitable across various patient groups. Moreover, beyond healthcare, this method can be applied to any machine learning domain where fairness is a concern and subgroup imbalances could skew results.
The implications of MIT's bias reduction method extend far beyond just technical improvements. On an economic level, reducing bias in AI can lead to improved outcomes especially in critical sectors like healthcare. Accurate AI predictions that fairly represent all patient groups could translate into better diagnostics and treatments, reducing overall healthcare costs by preventing misdiagnoses. Socially, this adds an extra layer of equity, promoting trust in AI technologies as systems become genuinely inclusive. Politically, MIT’s progress could encourage the establishment of regulatory standards focused on AI transparency and accountability, potentially paving the way for international cooperation on ethical AI practices.
Understanding the TRAK Method
The TRAK method represents a significant advancement in the pursuit of fair AI by specifically targeting and ameliorating biases during model training. This method allows researchers to pinpoint particular training examples that disproportionately affect model predictions for minority subgroups, enabling targeted removal without the need for overall dataset rebalancing. As a result, this strategy not only mitigates bias but also upholds or enhances accuracy, addressing a critical challenge faced by conventional bias mitigation techniques.
At its core, TRAK leverages an insight-driven approach to identify training samples that induce errors in predicting outcomes for underserved subgroups. These samples are then excised, and the model is retrained on the refined dataset, which mitigates bias. This nuanced adjustment is especially advantageous when subgroup labels are not explicitly available, which is a common hurdle in sensitive areas like healthcare where fairness in AI predictive power is crucial.
When applied to appropriate datasets, this technique has shown to require exclusion of fewer training samples compared to other methods, thereby retaining more of the original data's integrity. Furthermore, the TRAK method has often outperformed traditional techniques across varied datasets. However, there are challenges that come with its application, such as the inherent need for an understanding of subgroup dynamics that could otherwise be overlooked, indicating a need for further refinement of the approach.
Learn to use AI like a Pro
Get the latest AI workflows to boost your productivity and business performance, delivered weekly by expert consultants. Enjoy step-by-step guides, weekly Q&A sessions, and full access to our AI workflow archive.
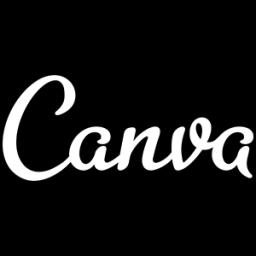
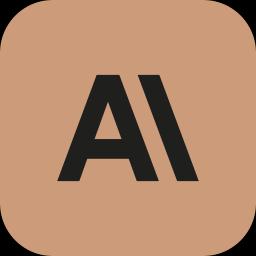
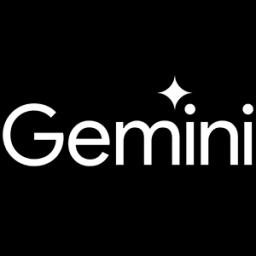
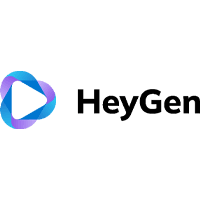
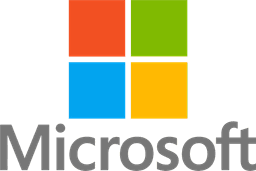
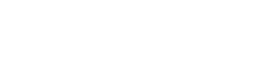
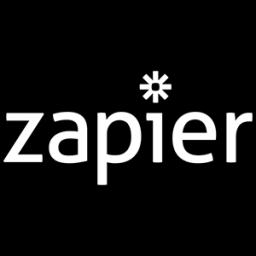
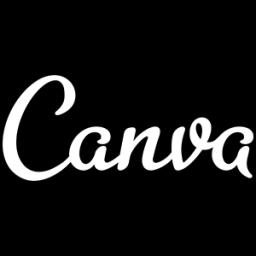
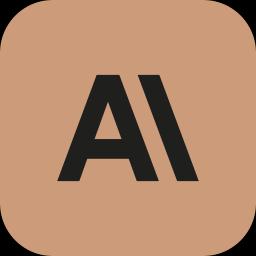
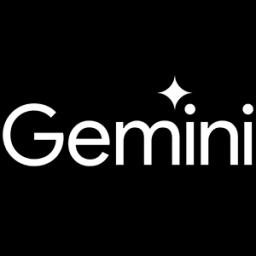
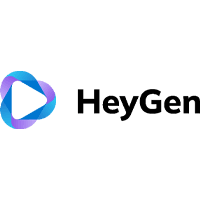
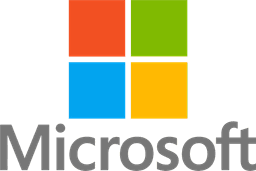
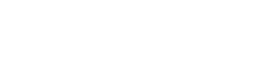
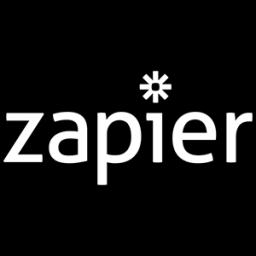
The innovation stands out as an actionable part of a larger movement towards creating equitable AI systems by offering a practical tool for data auditing and bias rectification. This development in bias reduction techniques contributes to ensuring AI models achieve fairness in real-world scenarios, setting new benchmarks for how bias in AI systems is addressed.
Applications and Ideal Use Cases
This technique is particularly impactful in industries where data sets are often biased due to historical or systemic factors, such as healthcare, finance, and criminal justice. For instance, in healthcare, this method could improve patient diagnosis and treatment plans by ensuring that AI models are unbiased and make accurate predictions for all demographic groups. Similarly, in finance, it can prevent discriminatory lending practices by ensuring that credit assessments are fair across different customer profiles.
The ability of the MIT technique to function without the need for explicit subgroup labels makes it ideal for datasets that are typically incomplete or inconsistent in tagging demographic information. This is a common issue in many real-world datasets, especially where privacy concerns limit data collection. Thus, it opens new opportunities for creating fair AI systems even in data-scarce environments, making it valuable for NGOs and governmental organizations that often work in such challenging conditions.
Moreover, the technique's efficiency, in requiring fewer data points to adjust model biases compared to traditional methods, makes it feasible for companies with limited computational resources. Startups and smaller companies that cannot afford extensive data annotation and training can leverage this method to build competitive and fair AI models, leveling the playing field across industries.
By enhancing the fairness of AI models with minimal adjustments to training data, the MIT technique can significantly reduce the computational overhead and time involved in model training and adjustment. This efficiency not only benefits technology companies but also aids academic institutions and research bodies that regularly develop new AI applications, allowing them to implement fair AI solutions more quickly and cost-effectively.
Challenges and Limitations of the New Technique
While the MIT technique is groundbreaking, it faces several challenges and limitations that must be addressed for it to fully realize its potential. Firstly, identifying biases without predefined subgroup labels requires a sophisticated understanding of the dataset, as the biases might not always be apparent. This necessitates intuition or deep domain knowledge, which may not be readily available across different fields. Furthermore, there is a risk of overcorrecting biases, which could lead to other forms of inaccuracies or loss of essential information within the data.
Learn to use AI like a Pro
Get the latest AI workflows to boost your productivity and business performance, delivered weekly by expert consultants. Enjoy step-by-step guides, weekly Q&A sessions, and full access to our AI workflow archive.
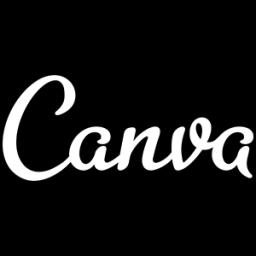
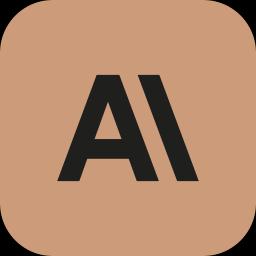
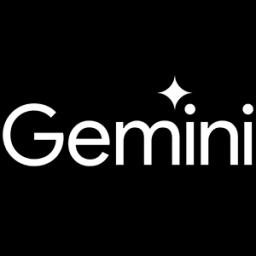
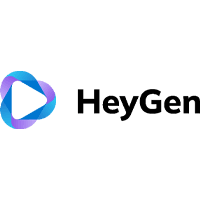
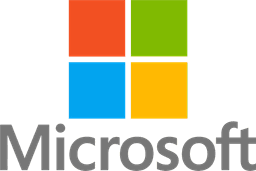
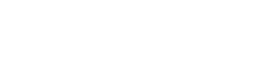
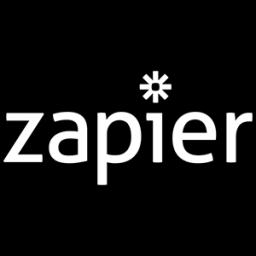
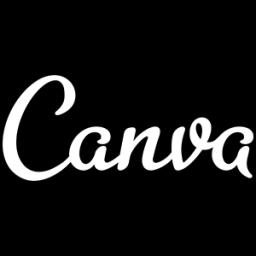
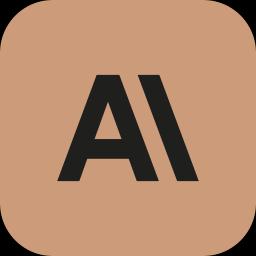
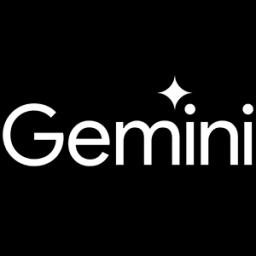
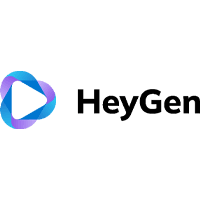
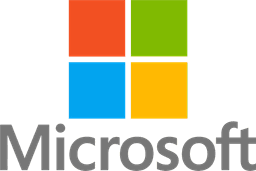
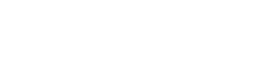
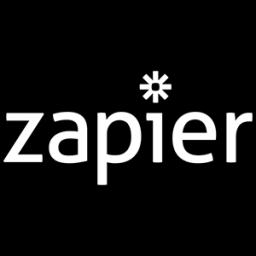
Another limitation lies in the technique’s reliance on TRAK, which, although effective, might not be universally applicable across all types of datasets. It's predominantly advantageous for datasets where subgroup labels are absent, but in scenarios where labels exist or are easily inferred, the technique's usefulness may diminish. Additionally, this approach, while reducing the number of samples needed for exclusion, might still not be as efficient as other emerging techniques tailored for specific domains.
The challenge of integrating this technique into existing workflows also exists. Organizations might be hesitant to overhaul their systems to incorporate new methods, especially when current models still deliver acceptable performance. Training and acclimatization periods for teams to get acquainted with this new approach add another layer of complexity and resourcing need, potentially limiting immediate adoption.
Lastly, its dependence on data-specific modalities could limit the breadth of its application. While it shows promise in the healthcare sector due to the absence of explicit subgroup labels, other sectors with different data characteristics might not see immediate benefits. Continued research, collaboration, and iteration will be necessary to adapt the technique for broader application and to deal with evolving datasets and biases as AI continues to develop.
Impact on Broader AI Fairness Initiatives
AI fairness initiatives are gaining momentum globally, and MIT's newly developed technique for reducing bias in AI models is set to make a significant impact. This technique enhances the ability to address biases that arise in machine learning models, particularly those that fail to accurately predict outcomes for minority subgroups within a dataset. By doing so, it contributes to the broader agenda of creating AI systems that are both fair and unbiased, aligning with initiatives across various sectors seeking to improve equity in AI-related applications.
The breakthrough by MIT researchers involves utilizing a method called TRAK, which identifies and removes specific training examples that most adversely affect model performance for underserved subgroups. Unlike traditional methods that require a broad balancing of datasets, this approach allows models to retain overall accuracy while specifically honing in on and correcting biased predictions for minority groups. As a result, it becomes a valuable tool in the toolkit of AI fairness advocates working to ensure that AI technologies do not perpetuate existing biases, particularly in critical sectors such as healthcare.
The implications of this technique on broader AI fairness initiatives are multifaceted. It provides researchers and developers with a practical mechanism to audit training data more efficiently, helping identify and rectify bias sources that may not be apparent. This aids in enhancing the transparency and accountability of AI systems, bolstering public trust in AI technologies. Moreover, it offers a pathway for developing more equitable AI tools that can better serve diverse populations, thus supporting initiatives aimed at promoting inclusivity and reducing inequities in AI-driven decision-making processes.
Learn to use AI like a Pro
Get the latest AI workflows to boost your productivity and business performance, delivered weekly by expert consultants. Enjoy step-by-step guides, weekly Q&A sessions, and full access to our AI workflow archive.
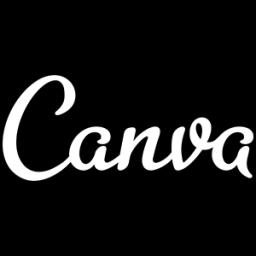
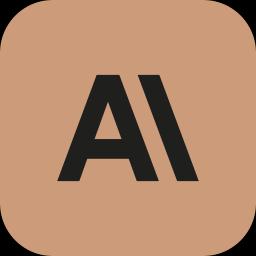
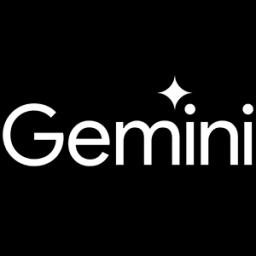
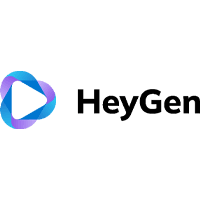
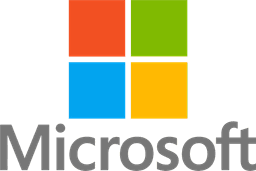
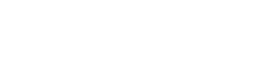
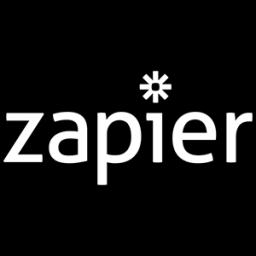
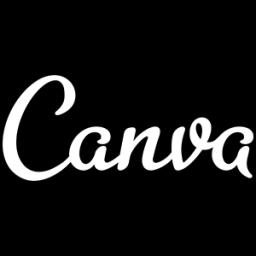
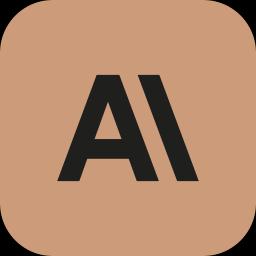
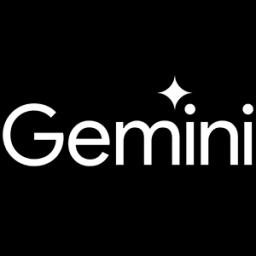
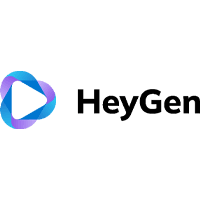
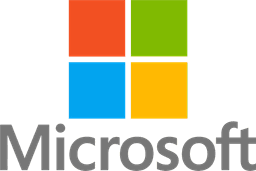
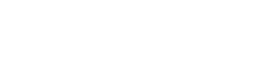
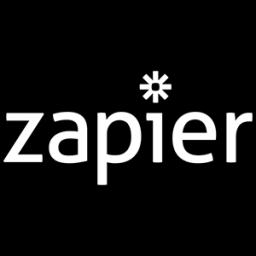
In addition to its direct effects on model accuracy and bias reduction, the technique also accentuates the importance of continual innovation and refinement of AI fairness practices. Related studies and techniques, such as Sony AI's TAB or the University of Washington's FairCL framework, complement this effort by providing alternative methods to tackle bias, suggesting that a collaborative approach to AI fairness is essential. The MIT technique exemplifies how specific interventions, such as targeted removal of biased data points, can significantly advance broader efforts towards equitable AI solutions.
Ultimately, the technique developed at MIT represents a forward leap in the ongoing pursuit to create fairer AI systems. By addressing bias at the data level and offering a novel method for enhancing model performance without sacrificing accuracy, this innovation plays a pivotal role in the broader landscape of AI fairness. It affirms the continuous need for developing robust solutions that can be effectively integrated into existing and future AI systems, guiding the way toward more fair and responsible AI applications worldwide.
Expert Opinions on the MIT Technique
Dr. Emily Carter, a leading AI researcher from Stanford University, remarks that the MIT technique signifies a pivotal development in machine learning, allowing biased datasets to be cleaned of potentially harmful samples without sacrificing performance. She emphasizes the system's ability to adapt to complex datasets where subgroup information is not readily available, a common obstacle in achieving fairness in computational models.
Dr. Michael Lin, an AI ethicist from Harvard, applauds the method's potential for integration into existing AI systems due to its adaptable nature. According to Dr. Lin, this ability to refine training data without extensive recalibration suggests a future where AI models can be dynamically adjusted for fairness, significantly improving accountability in AI processes.
Prof. Anika Ramesh, a data science expert at Carnegie Mellon University, notes that while the MIT technique does not entirely erase the need for comprehensive dataset reviews, it does provide a viable first step towards identifying and mitigating bias. She stresses its importance in sensitive fields, such as predictive analytics in education, where equitable treatment of demographic subgroups can lead to enhanced educational outcomes.
Public Reactions to the AI Bias Reduction Technique
Public reactions towards MIT's new AI bias reduction technique have been a mix of optimism and caution. Enthusiasts on social media and public platforms are hopeful that these technological advancements will significantly reduce existing biases in AI models. Many highlight the potential for improved fairness and accuracy in AI systems across various sectors, particularly in areas like healthcare. This sentiment is fueled by a growing public demand for transparency and effective bias mitigation strategies in AI, aligning with ongoing advocacy for increased data diversity and transparency.
Learn to use AI like a Pro
Get the latest AI workflows to boost your productivity and business performance, delivered weekly by expert consultants. Enjoy step-by-step guides, weekly Q&A sessions, and full access to our AI workflow archive.
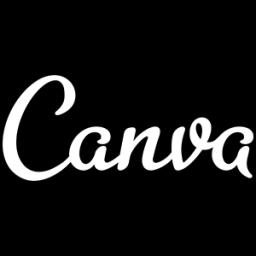
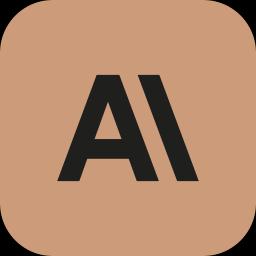
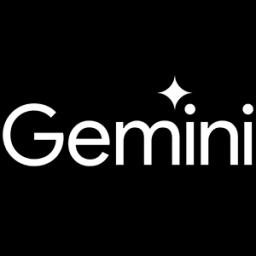
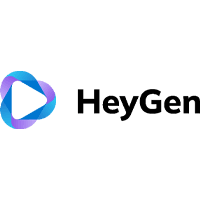
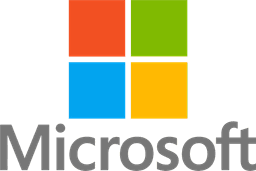
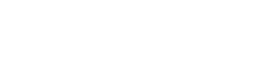
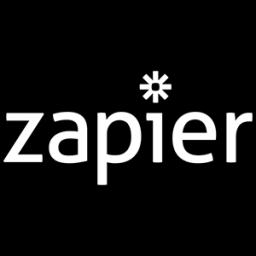
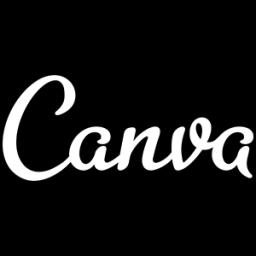
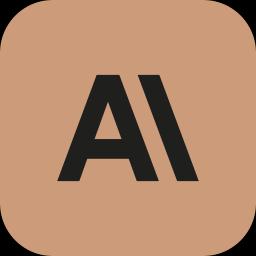
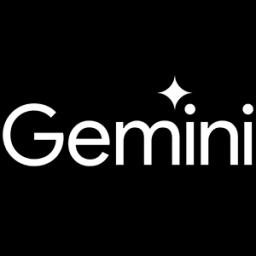
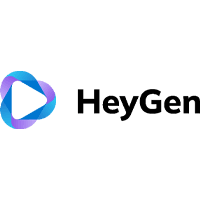
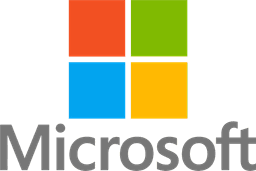
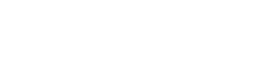
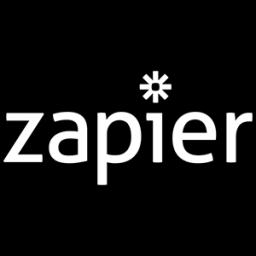
Despite the optimistic outlook, skepticism about the widespread adoption and practical effectiveness of this technique persists. Critics question whether such methods can be seamlessly integrated into existing AI systems without compromising overall performance. Discussions emphasize the need for involving diverse stakeholders in the developmental phase and ensuring there is adequate regulatory oversight. Such measures are viewed as critical to making these promising techniques an integral part of public trust and fairness in AI systems.
Overall, while the public responds positively to MIT's innovative approach, the awareness of potential challenges and the complexity of implementing such solutions indicates a cautious optimism. The discourse continues to focus on how best to ensure these technological advances lead to genuinely equitable and transparent AI solutions. The corresponding community calls for collaborative approaches and rigorous testing to foster confidence in these methods as steps towards more equitable AI applications.
Future Implications of Bias-Free AI Models
The potential future implications of bias-free AI models are vast and transformative. With the successful implementation of MIT's technique for reducing bias in AI models, there could be significant economic benefits, particularly in industries like healthcare. Accurate and unbiased AI can lead to better diagnoses and treatments, reducing disparities and costs that arise from misdiagnoses for underrepresented groups. This improvement in AI reliability can enhance patient trust and lead to broader acceptance and deployment across various sectors.
Socially, ensuring AI models are free from bias can contribute to greater inclusivity and fairness, fostering an environment where people of all backgrounds have equal access to the benefits of AI technologies. As these models become more equitable, public trust in AI applications is likely to increase, facilitating more harmonious interactions between technology and society. The move towards unbiased AI is a step towards leveling the playing field for all users and stakeholders, helping to rectify systemic inequalities perpetuated by historical biases in data.
Furthermore, on a political level, developing fair and unbiased AI systems can encourage regulatory bodies to establish and enforce frameworks that ensure accountability and transparency in AI technologies. In this way, unbiased models can drive advancements in AI regulations, leading to the creation of ethical standards that govern AI development and deployment responsibly. Such frameworks can spur international collaboration, setting the stage for global standards that manage the impacts of AI ethically and sustainably.
By addressing bias, AI models could contribute to a more equitable society, enhancing fairness and justice in technology applications. The integration of such techniques is crucial as AI continues to play an increasingly central role in decision-making and policy formulation. This movement towards fairness in AI has the potential to significantly uplift societal well-being, ensuring that technology serves all communities equitably.
Learn to use AI like a Pro
Get the latest AI workflows to boost your productivity and business performance, delivered weekly by expert consultants. Enjoy step-by-step guides, weekly Q&A sessions, and full access to our AI workflow archive.
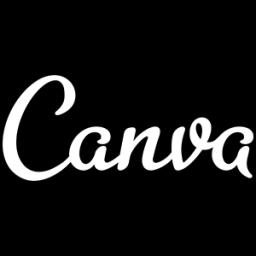
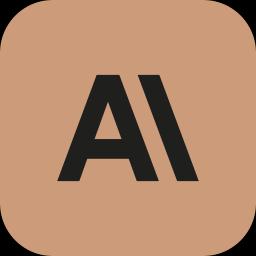
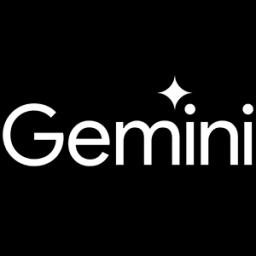
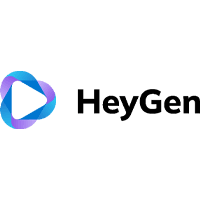
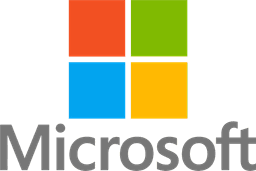
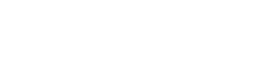
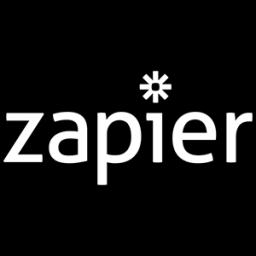
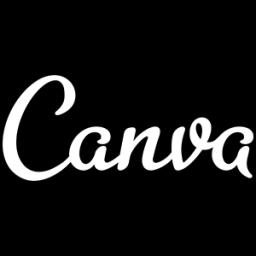
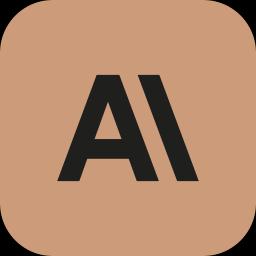
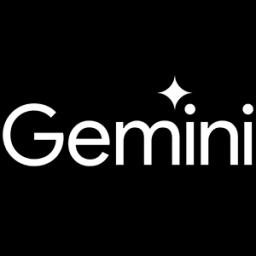
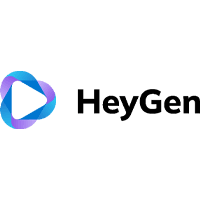
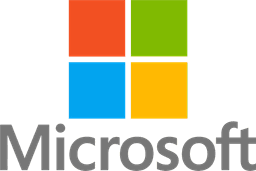
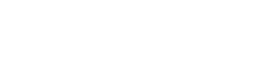
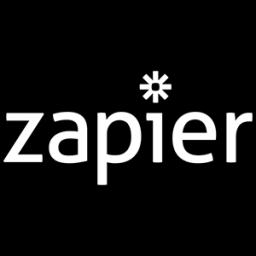