Local AI Made Easy
Msty Revolutionizes Local AI: Run Models Without the Tech Hassle!
Last updated:

Edited By
Mackenzie Ferguson
AI Tools Researcher & Implementation Consultant
Discover how Msty is transforming the way we run AI models locally! Offering unparalleled privacy, customization, and data control, Msty simplifies the process of integrating various AI models on your desktop. With features like multi-AI chat, knowledge stacks, and more, even the hardware-conscious will find this closed-source marvel free for personal use. Dive into a new era of local AI with Msty!
Introduction to Running AI Locally
Running AI models locally offers several benefits over cloud-based solutions, including increased privacy, customization, and greater control over data. The Hackaday article delves into these advantages, specifically through the lens of an application called Msty. By allowing users to operate AI models on personal computers, Msty addresses significant concerns about data privacy, as users' information does not need to be sent to the cloud. Additionally, local setup enables users to tailor AI models to better fit their personal needs and applications, rather than being restricted to a one-size-fits-all cloud model.
Overview of Msty and Its Key Features
The local AI landscape is rapidly evolving with the introduction of applications like Msty, which simplifies running AI models on personal devices. Msty is a desktop application designed to enable users to run AI models locally, utilizing its key features such as multi-AI chat, which allows simultaneous interaction with multiple AI models. The software offers knowledge stacks, facilitating the organization and retrieval of personal data, as well as prompt management tools that enhance user control over AI operations. Additional features include the ability to attach files, maintain chat histories, and choose from various privacy modes. The application also supports integration with remote AI services and provides options for subscribing to premium services. However, while it brings several advantages, Msty’s closed-source nature raises questions about transparency and data privacy among potential users. Despite its proprietary status, Msty remains free for personal use, offering a valuable solution for AI enthusiasts seeking more personalized and controlled AI experiences.
Learn to use AI like a Pro
Get the latest AI workflows to boost your productivity and business performance, delivered weekly by expert consultants. Enjoy step-by-step guides, weekly Q&A sessions, and full access to our AI workflow archive.
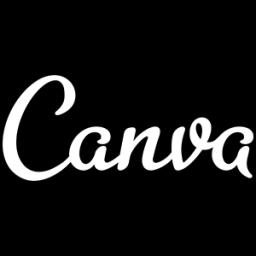
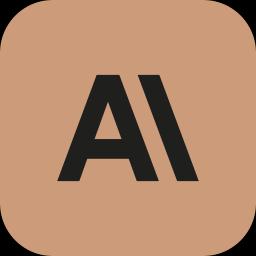
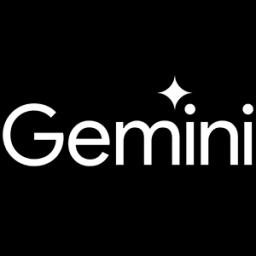
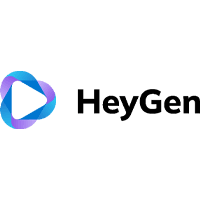
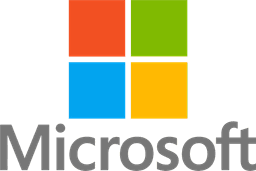
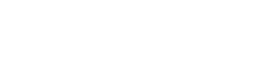
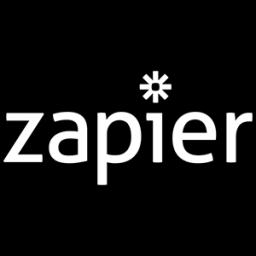
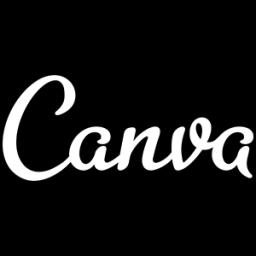
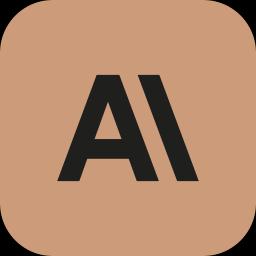
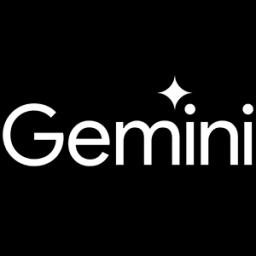
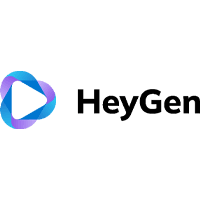
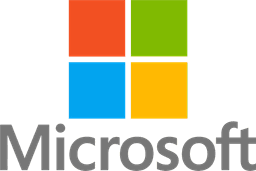
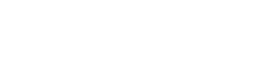
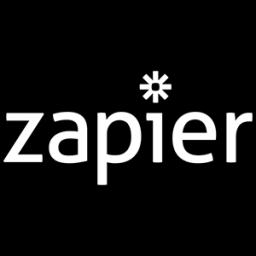
Hardware Requirements for Local AI Applications
Running AI models locally offers significant advantages in terms of privacy, customization, and data control. One standout application in this area is Msty, which simplifies the process of getting AI models up and running on local hardware. Msty streamlines the setup by allowing easy downloads, installations, and execution of various AI models. While it remains closed-source, it is a free option for personal use, providing users with an intuitive multi-AI chat functionality, personal data integration, and efficient prompt management.
However, to leverage Msty's capabilities fully, certain hardware requirements must be met. A key requirement is having a video card with 12GB or more of GPU memory. The article cites an example where an upgrade from a 6GB NVidia 2060 to a 12GB 3060 improved performance notably, underscoring the importance of meeting the hardware criteria for optimal use of the application.
While Msty provides a convenient solution for local AI deployment, it is not the only option. Several open-source alternatives exist, including text-generation-webui, GPT4All, and Ollama. These options offer robust features and are often considered for users looking for more transparency compared to Msty's closed-source nature.
Running AI locally empowers users with enhanced privacy and data control. Users can fine-tune models to meet specific needs, offering unique customization not possible with cloud-based solutions. This flexibility allows individuals to maintain greater control over their AI interactions and data, aligning with privacy-conscious practices.
Learn to use AI like a Pro
Get the latest AI workflows to boost your productivity and business performance, delivered weekly by expert consultants. Enjoy step-by-step guides, weekly Q&A sessions, and full access to our AI workflow archive.
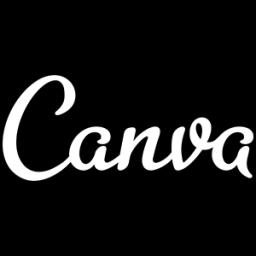
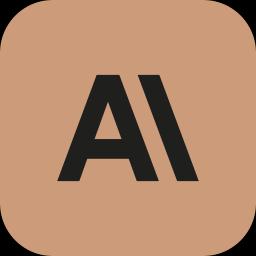
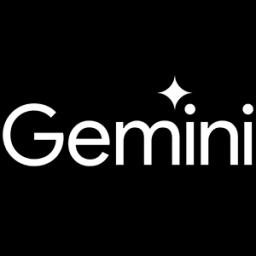
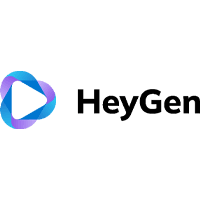
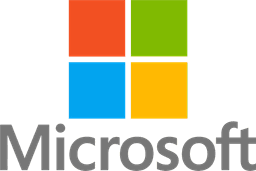
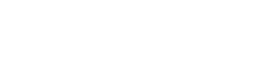
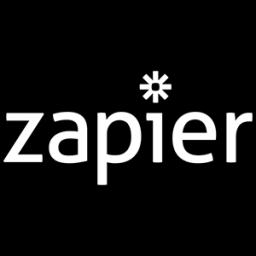
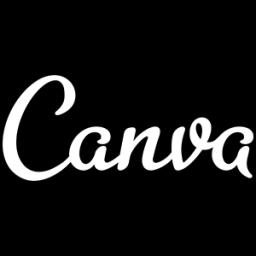
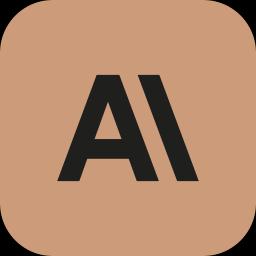
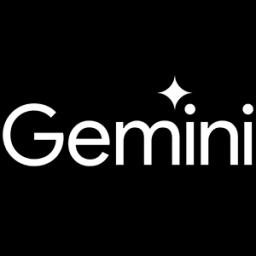
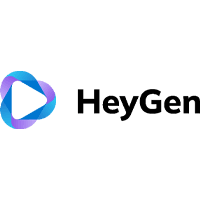
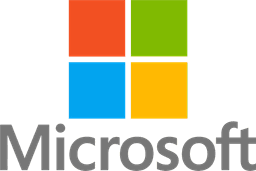
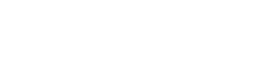
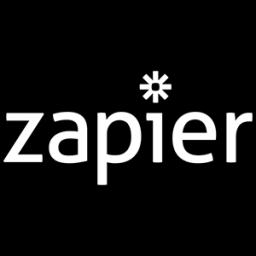
Looking into the future, local AI deployment could drive the demand for high-performance consumer hardware, hinting at a market boost for GPU manufacturers. Additionally, this shift could alter cloud service providers' business models, pushing them toward edge computing solutions. Nevertheless, the emergence of local AI applications introduces potential challenges, including concerns about open-source versus proprietary solutions, the digital divide due to hardware access, and regulatory adjustments.
Comparison of Msty with Open-Source Alternatives
In the burgeoning landscape of AI deployment, local applications such as Msty have carved a niche for themselves by offering users significant advantages over traditional cloud-based solutions. At the heart of these benefits are privacy, customization, and control – essential criteria for many users looking to adopt AI technologies without compromising their data's sanctity. Deploying AI models locally permits users to keep sensitive data on their devices, minimizing the risk of exposure or misuse by third parties.
Msty, though not open-source, provides a simplified interface for installing and managing various AI models, making it an attractive option for users keen on local AI applications. However, the closed-source nature of Msty raises concerns about transparency and potential data harvesting, sparking a debate among users and privacy advocates. This apprehension has led to calls for the application to be open-sourced, which would potentially enhance its credibility and adoptability.
In contrast, open-source alternatives like text-generation-webui, GPT4All, and Ollama offer transparency and community-driven development, which many users believe are crucial for ensuring data control and fostering innovation. Such platforms not only allow for easier scrutiny of underlying mechanisms but also enable custom modifications that cater to specific user needs, further extending the customization capabilities beyond what closed-source solutions can offer.
The hardware demands of running local AI applications also cannot be understated. Msty, for instance, recommends a minimum of 12GB GPU memory, catering primarily to users with capable hardware setups. This requirement sparks an important conversation about accessibility and the potential digital divide that may arise, as not all users can afford or access the necessary hardware upgrades to fully leverage local AI capabilities.
Public discourse around these technologies emphasizes the need for a balance between proprietary solutions like Msty and open-source alternatives. While Msty offers a user-friendly package that simplifies local AI integration, its closed nature contrasts with the transparency and flexibility of open-source platforms, leading to a spirited discussion about the future of AI deployment and data privacy. As technology progresses, these debates will likely intensify, shaping how AI becomes integrated into everyday technology use.
Learn to use AI like a Pro
Get the latest AI workflows to boost your productivity and business performance, delivered weekly by expert consultants. Enjoy step-by-step guides, weekly Q&A sessions, and full access to our AI workflow archive.
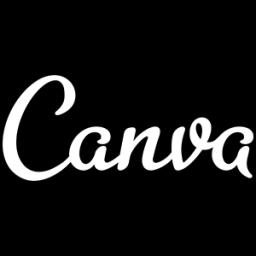
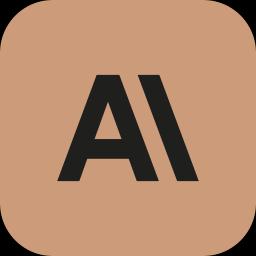
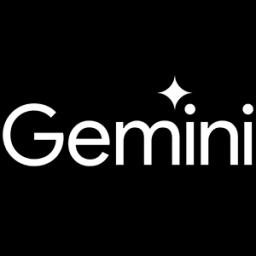
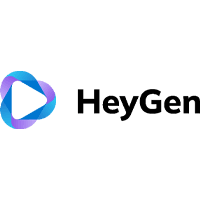
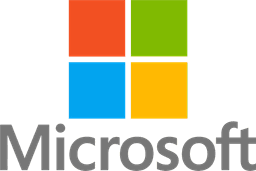
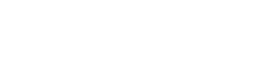
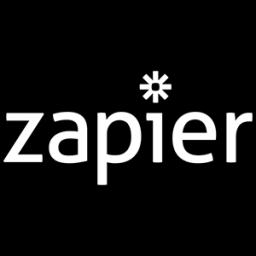
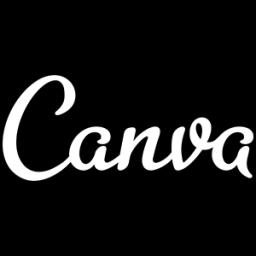
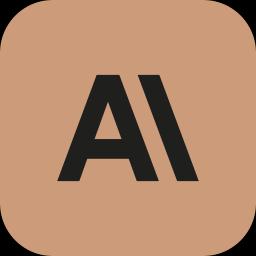
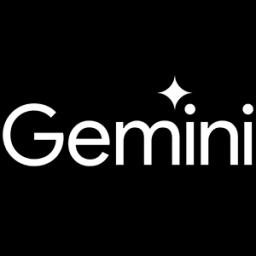
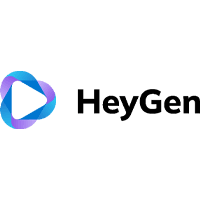
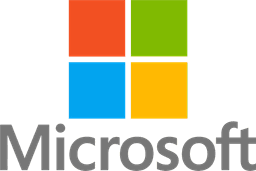
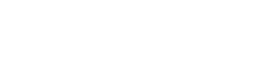
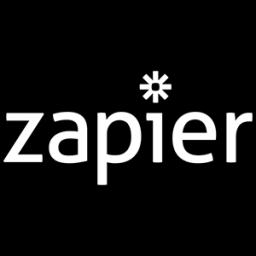
Benefits of Running AI Locally
Running AI locally on personal devices offers numerous significant benefits. One of the primary advantages is enhanced privacy. When AI models are operated locally, users' data is processed on their own devices rather than on remote servers, ensuring that sensitive information remains private and secure from third-party access. This level of privacy is particularly attractive to organizations and individuals who handle confidential data and wish to minimize the risk of data breaches.
Local AI deployment also allows for a high degree of customization. Users can tailor AI models to meet specific needs, adjusting features and functionalities according to their own preferences or use cases. Such customization is invaluable in fields requiring bespoke solutions, such as healthcare or finance, where operations can differ significantly between entities. The ability to fine-tune models locally ensures that the AI can adapt rapidly to unique challenges or requirements without relying on third-party customization services.
Moreover, running AI locally ensures greater control over the AI's performance and functionality. Users can monitor and manage how the AI behaves, which is not as feasible when relying on cloud-based solutions. This control extends to aspects like data usage, processing power distribution, and software updates, granting users the power to optimize the AI's performance to suit their specific operating environments.
Applications like Msty illustrate these benefits by simplifying the process of running local AI models. By supporting features such as multi-AI chat and personal data integration, and requiring a recommended hardware specification of 12GB+ GPU memory, Msty allows users to take full advantage of local AI capabilities efficiently. While Msty is criticized for being closed-source, it remains a free option for personal use, making advanced AI functionalities accessible to a broader audience.
In addition to these benefits, the landscape of local AI is expanding with the development of alternatives such as text-generation-webui, GPT4All, and Ollama, which are open-source and offer varied functionalities for those seeking transparency and flexibility. These alternatives are driving innovation and accessibility in the AI domain, promoting a robust ecosystem that supports diverse user needs and technological advancements.
Challenges and Concerns with Msty
The advent of local AI applications such as Msty has brought forth a number of challenges and concerns, casting a spotlight on various aspects of the technology. While the benefits of running AI models locally, such as enhanced privacy and customization, are significant, they also come with notable caveats and the subject has sparked substantial debate in technology circles.
Learn to use AI like a Pro
Get the latest AI workflows to boost your productivity and business performance, delivered weekly by expert consultants. Enjoy step-by-step guides, weekly Q&A sessions, and full access to our AI workflow archive.
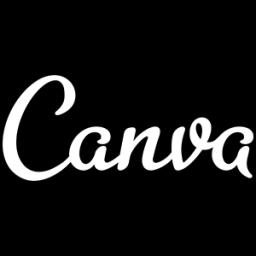
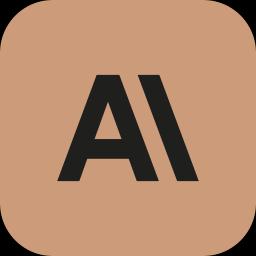
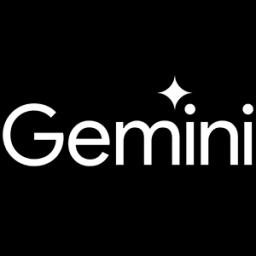
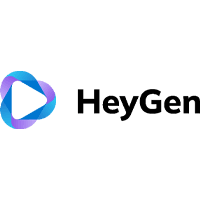
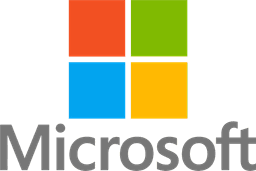
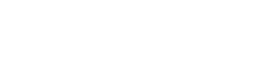
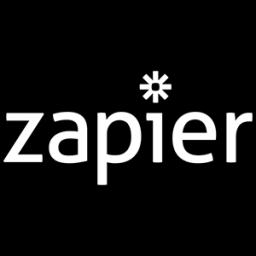
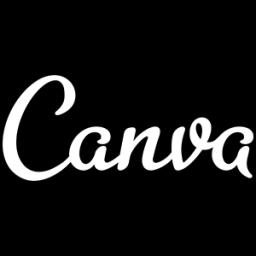
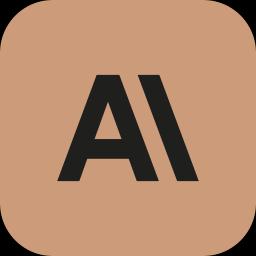
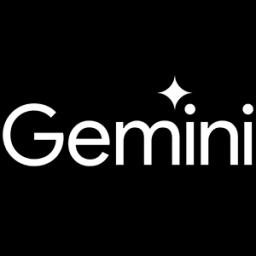
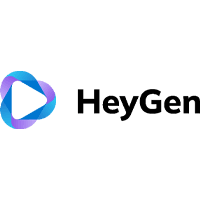
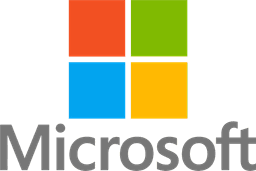
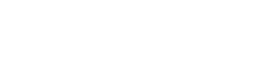
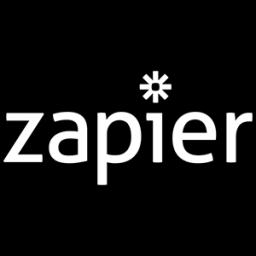
One of the primary concerns with Msty is its closed-source nature. Even though the application is free for personal use, the lack of transparency raises doubts about the potential for data harvesting. This closed-source attribute has led to calls from the community for the application to be open-sourced to bolster trust and transparency.
Moreover, there are apprehensions regarding the hardware requirements needed to effectively run Msty. Although the application offers a rich feature set including multi-AI chat and personal data integration, these capabilities demand significant computational resources. The recommended 12GB of GPU memory, for example, may present a barrier to entry for many potential users.
In addition to technical concerns, there are social implications tied to the use of local AI models. Privacy advocates praise the potential for better data security, but others argue that a digital divide could emerge, with those unable to afford high-end hardware being left behind. This raises questions regarding the equitable distribution of technology.
Furthermore, the sustainability of Msty's free offering has been questioned by some users, who express skepticism about its long-term viability without a clear monetization strategy. These economic considerations are crucial as they influence both the development and adoption of such technologies.
Finally, even as Msty and similar applications redefine the AI landscape, they bring to the fore crucial discussions about the trade-offs between proprietary and open-source solutions. As technology progresses, these discussions will shape the future direction of AI development, underscoring the importance of balancing innovation with ethical considerations.
Public Reactions and Discussions
In recent times, the introduction of Msty, a local AI application, sparked considerable conversation across various platforms. Privacy advocates were at the forefront in praising the application's local AI approach, noting significant advantages in terms of data control and model customization. They emphasized how these features contribute to a more personalized experience, with users retaining full authority over their personal information and interactions with AI models.
Learn to use AI like a Pro
Get the latest AI workflows to boost your productivity and business performance, delivered weekly by expert consultants. Enjoy step-by-step guides, weekly Q&A sessions, and full access to our AI workflow archive.
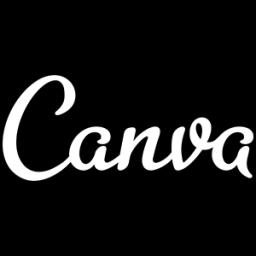
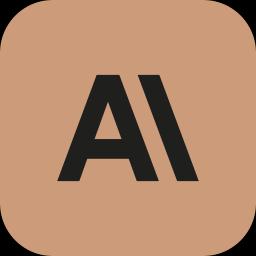
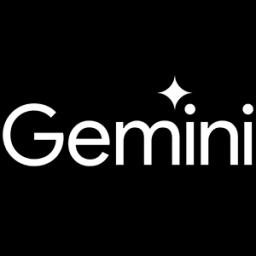
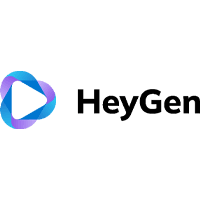
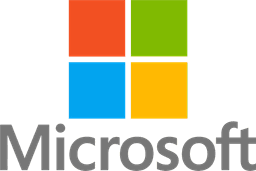
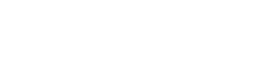
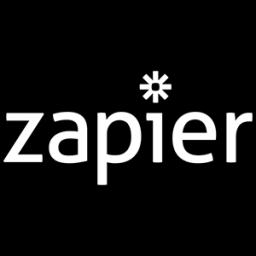
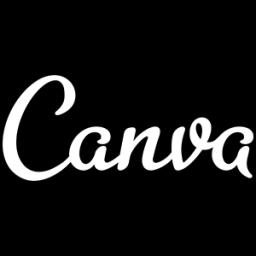
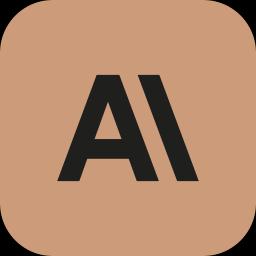
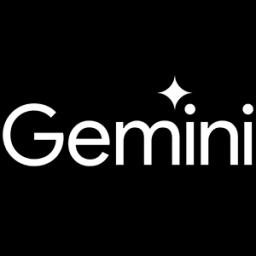
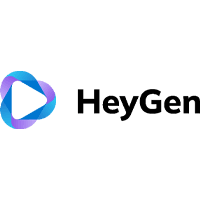
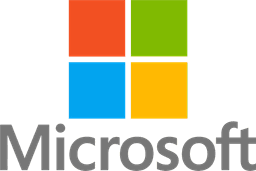
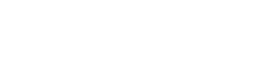
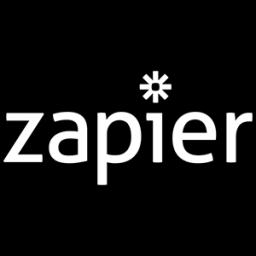
Despite the enthusiastic reception from privacy-conscious groups, there was considerable criticism regarding Msty's closed-source nature. Many voiced concerns over the potential for undisclosed data harvesting, a fear rooted in the lack of transparency inherent to proprietary software. This skepticism was especially prominent on social media and forums, sparking heated debates about the safety of entrusting sensitive data to closed-source applications.
Calls for more transparency and open-sourcing of Msty grew louder, bolstered by the active community's comparisons to existing open-source alternatives such as text-generation-webui and GPT4All. These alternatives are praised not only for their transparency but also for their flexibility and community-driven development models, which many argue result in safer and more robust software offerings.
Further adding to the mixed public reactions, some users expressed doubts about the longevity of Msty’s free model. They questioned the financial sustainability of providing robust AI capabilities without charge, speculating on the eventual introduction of monetization strategies that may contradict its current user-focused ethos.
Technical discussions emerged as well, highlighting the complexity involved in running advanced AI models locally. Topics such as model sizes, quantization, and memory management became focal points, with tech-savvy users dissecting the hardware requirements and limitations involved in local AI deployment. These discussions were marked by their technical depth, pushing the community towards a deeper understanding of the challenges and opportunities presented by local AI solutions.
Future Implications and Developments in Local AI
The future trajectory of local AI applications such as Msty suggests a profound shift across multiple dimensions of technology and society. As these systems gain popularity, high-performance consumer hardware will become more essential, propelling demand for advanced GPUs and components tailored for AI processing. Companies like NVIDIA and ARM are likely to benefit from this trend, as their specialized chipsets are increasingly required for local AI computations. Economically, this surge could potentially disrupt the dominance of cloud-based AI services as more users opt for localized solutions that grant them greater privacy and control over their data.
On a social level, the adoption of local AI models promises significant empowerment through enhanced privacy and the democratization of AI technology. By enabling individuals to maintain control over their data, local AI reduces the risk of privacy invasions commonly associated with cloud-based systems. This shift towards decentralization could also bridge some gaps in technology access, though it may inadvertently widen the digital divide due to the necessary high-end hardware requirements. Consequently, there is a pressing need to address accessibility to prevent disparities from intensifying.
Learn to use AI like a Pro
Get the latest AI workflows to boost your productivity and business performance, delivered weekly by expert consultants. Enjoy step-by-step guides, weekly Q&A sessions, and full access to our AI workflow archive.
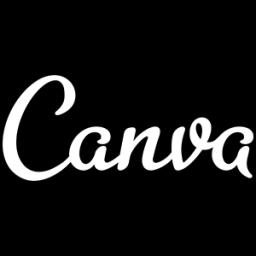
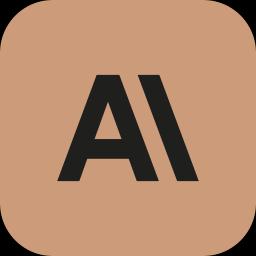
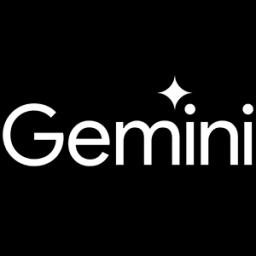
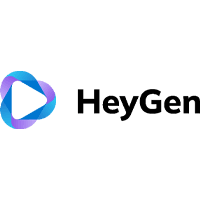
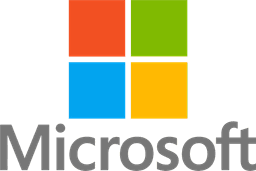
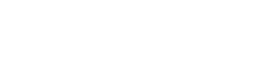
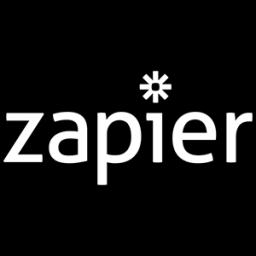
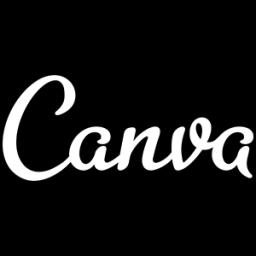
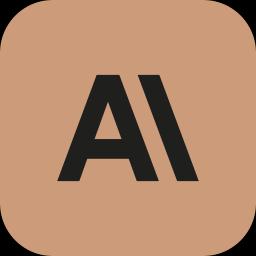
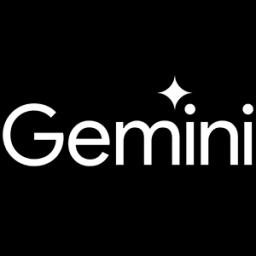
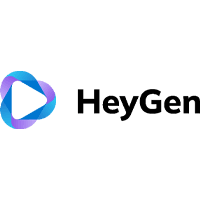
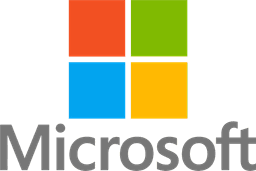
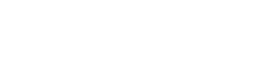
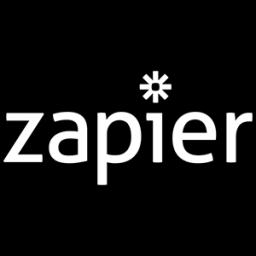
Politically, the increase in local AI adoption could challenge regulatory frameworks that rely on centralized control and monitoring of AI services. These systems, by nature, afford users the ability to operate AI independently, which could diminish governmental oversight capabilities and complicate existing surveillance and data protection laws. This decentralization may also reinvigorate debates over open-source versus proprietary software, potentially leading to new policies and regulations as governments attempt to navigate the changing landscape.
Technological advancements will continue to power the development of more efficient AI model compression technologies, enabling such models to run on a broader range of consumer devices. This could significantly influence the direction of the AI industry, driving more research into edge computing paradigms that emphasize localized processing power. The result is a likely shift in how AI services are delivered, from sprawling cloud data centers to more personalized, device-centric models, opening new frontiers for both developers and consumers.
Expert Opinions on Local vs. Cloud AI Deployment
The debate between local and cloud AI deployment is gaining complexity as both options present unique advantages and challenges. Experts in the field are weighing in on this topic, bringing valuable insights that can guide future deployment strategies. Dr. Emily Bender, a professor of linguistics at the University of Washington, champions the privacy benefits of local models. She points out that running AI locally empowers users by allowing them to maintain control over their data, thereby minimizing the risk of exposure to misuse or third-party exploitation.
Conversely, Andrew Ng, the founder of DeepLearning.AI, underscores the customization potential that local deployment offers. By allowing for fine-tuning of models to specific use cases, local AI can serve specialized industries or cater to proprietary needs more effectively. However, Dr. Dario Amodei, co-founder of Anthropic, offers a cautionary perspective on the substantial computational resources required for local AI models, which he suggests could limit their broad adoption due to high hardware demands.
Professor Fei-Fei Li from Stanford University highlights the inherent trade-offs between privacy and performance in local AI models. While they provide users with enhanced privacy and control, they may also necessitate compromises in model performance and require frequent updates. These varied expert opinions underscore a broader industry conversation around the balance of power between local control and the scalability and accessibility offered by cloud solutions. Each perspective plays a crucial role in understanding the complex landscape of AI deployment.
Related Technological Advancements in Local AI
The field of local AI deployment has been experiencing significant advancements, driven by the demand for privacy, control, and customization. One such advancement is the introduction of Msty, a desktop application developed to streamline the process of downloading, installing, and running AI models locally. By providing features like multi-AI chat, knowledge stack integration, and prompt management, Msty positions itself as a convenient tool for users looking to harness the power of AI on their personal devices. Despite being closed-source, Msty is free for personal use, offering users the ability to integrate personal data while maintaining privacy. The application's performance is optimized for devices with a minimum of 12GB GPU memory, setting a benchmark for hardware requirements in the realm of local AI applications.
Learn to use AI like a Pro
Get the latest AI workflows to boost your productivity and business performance, delivered weekly by expert consultants. Enjoy step-by-step guides, weekly Q&A sessions, and full access to our AI workflow archive.
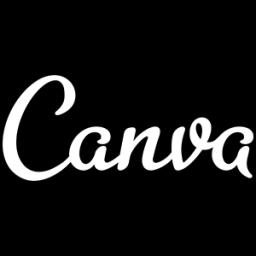
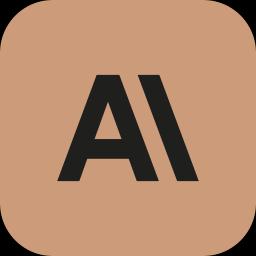
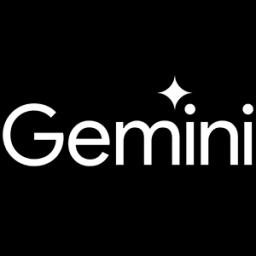
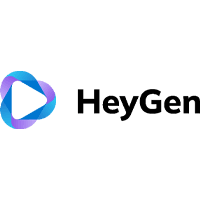
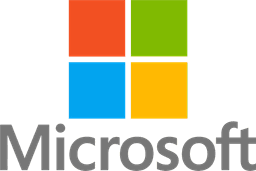
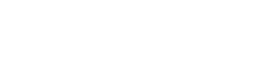
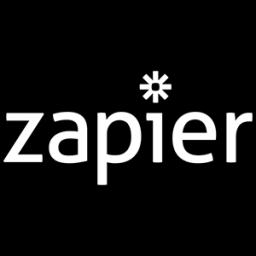
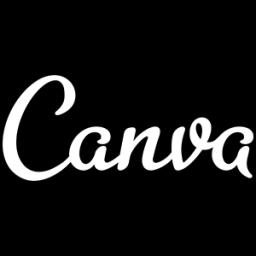
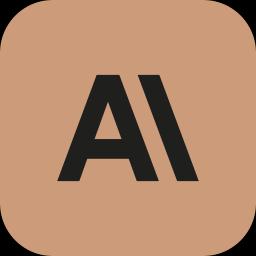
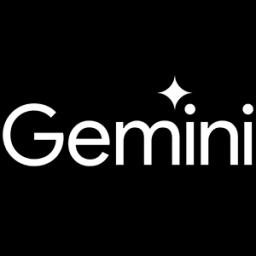
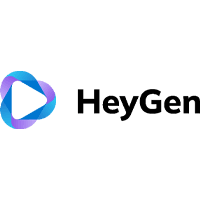
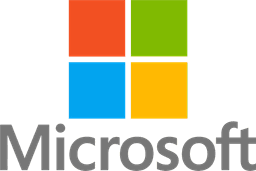
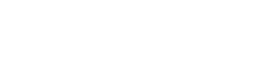
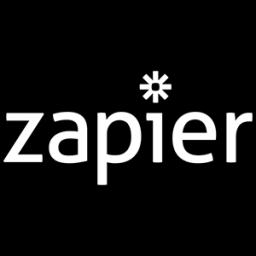
In the landscape of AI technology, several notable events have shaped the way AI models are run locally. The launch of Ollama, an open-source platform, simplifies the local deployment of large language models, enabling users to operate AI processes on personal computers with ease. Concurrently, Apple's advancements in on-device AI have emphasized privacy and offline functionality, showcasing the company's commitment to enhancing AI capabilities directly on iOS devices. Additionally, Google has made significant strides with MobileNetV3, an efficient neural network architecture tailored for mobile and edge devices, reinforcing the push towards on-device machine learning. Meta's breakthrough in AI model compression furthers these efforts, showcasing new techniques that allow powerful models to operate on everyday consumer devices such as smartphones. The rise in demand for AI chipsets also reflects the growing interest and investment in edge computing, signalling a shift in how AI technology is developed and deployed.
Experts in the field have offered varied perspectives on the proliferation of local AI models. Dr. Emily Bender highlights the privacy benefits of running AI models locally, enabling users to retain control over their data, thus minimizing the risk of sensitive information exposure. Andrew Ng, on the other hand, underscores the customization potential that local AI deployments offer, allowing industries to tailor models to specific uses. Despite these advantages, Dr. Dario Amodei warns of the significant computational resources required for local AI models, which could limit widespread adoption. Professor Fei-Fei Li also notes the trade-offs between privacy and performance in local vs cloud-based AI models, advising users to carefully weigh these factors when choosing between deployment models.
Public reaction to Msty has been a mix of enthusiasm and criticism. Privacy advocates have applauded the application for its local data handling capabilities and customization options, while others have expressed concern over its closed-source nature, fearing potential data harvesting. Discussions have been rife, drawing comparisons between Msty and various open-source alternatives like text-generation-webui and GPT4All. These discussions have sparked debates around transparency, privacy, and the viability of proprietary versus open-source solutions. Additionally, skepticism about Msty's long-term viability as a free service has emerged, with calls for increased transparency in its operations.
The future implications of local AI technology are profound and stretch across economic, social, political, and technological dimensions. Economically, there could be a surge in demand for high-performance consumer hardware, potentially disrupting cloud-based AI services and encouraging the growth of the edge AI chipset market. Socially, local AI promises enhanced privacy and data control, democratizing AI technology while posing the risk of a digital divide among those with varying levels of hardware access. Politically, it could challenge government regulation of AI usage and stir debates about open-source policies. Technologically, advancements in AI model compression and edge computing could lead to a paradigm shift away from centralized cloud computing solutions, emphasizing local processing capabilities.