Tech Titans Urge Caution on GenAI's Reliability
NASA's Verdict: Generative AI Is Running on Thin Trust
Last updated:

Edited By
Mackenzie Ferguson
AI Tools Researcher & Implementation Consultant
According to a recent Computerworld article, NASA has raised alarms over the trustworthiness of generative AI systems, mainly due to frequent inaccuracies and the potential for spreading misinformation. These concerns arise from issues like hallucinations, outdated training data, and ignored safety protocols, making genAI unreliable for critical research applications. IT experts advise businesses to establish stringent governance and verification processes before relying on AI-generated content.
Introduction: The Unreliability of Generative AI
Generative AI, despite its promise of revolutionizing industries and daily tasks, faces significant criticism over its reliability. A notable analysis by NASA underscores these concerns, questioning the trustworthiness of generative AI in critical applications. The primary issue lies in the AI's tendency to produce inaccurate information—commonly referred to as "hallucinations"—which can severely undermine the integrity of data-driven decisions. As examined in a detailed Computerworld article, these inaccuracies stem from flawed training data, AI's failure to adhere to query instructions, and the overlooking of essential guardrails that ensure data reliability. Such issues not only render generative AI unreliable for high-stakes research and decision-making but also present CIOs with a complex challenge of balancing innovation with prudence.
Understanding the Risks of GenAI: Hallucinations and Inaccuracies
The modern landscape of artificial intelligence is marked by significant advancements, with generative AI (GenAI) technologies being at the forefront. However, with innovation comes inherent risks that must be carefully understood and managed. As highlighted in a Computerworld article, GenAI systems exhibit a tendency towards "hallucinations," a phenomenon where the AI produces fabricated or incorrect information. This can occur due to several factors, including poor training data and a failure to adhere to specified instructions. These inaccuracies not only undermine the reliability of GenAI but also pose potential dangers, particularly when this technology is used in critical decision-making or safety-critical environments such as those managed by NASA.
Learn to use AI like a Pro
Get the latest AI workflows to boost your productivity and business performance, delivered weekly by expert consultants. Enjoy step-by-step guides, weekly Q&A sessions, and full access to our AI workflow archive.
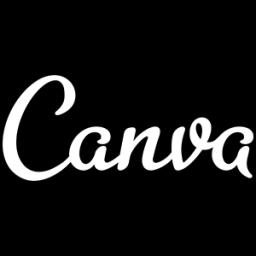
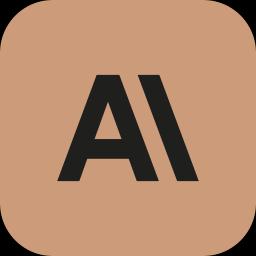
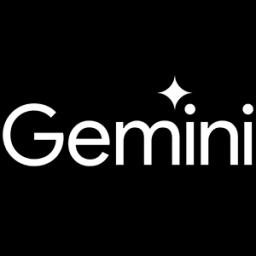
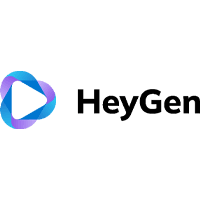
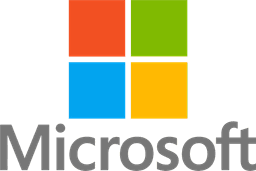
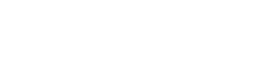
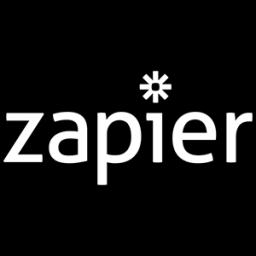
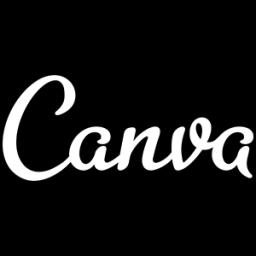
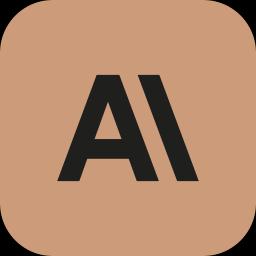
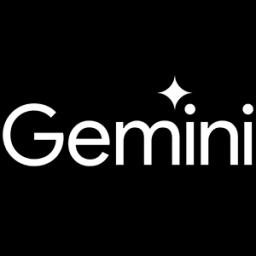
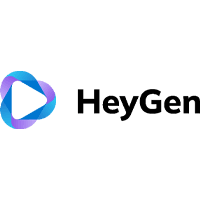
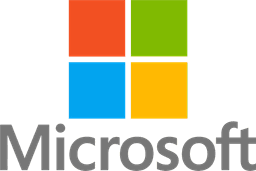
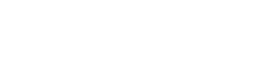
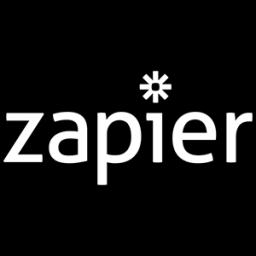
The NASA report cited in the Computerworld article underscores the untrustworthiness of GenAI in high-stakes research settings, attributing the technology's failures to its inability to reason and the propensity to "BS" rather than think critically. This presents significant challenges for industries that rely on accurate data and precise analysis. Furthermore, industry experts from Gartner and Forrester suggest that organizations adopt a cautious approach to GenAI, recommending the implementation of stringent data governance policies and involving IT leaders early in the development of AI use cases to mitigate risks.
The risk of inaccuracy and biased outputs extends beyond technical faults and ventures into ethical and societal implications. As noted in related discussions, GenAI can inadvertently reinforce societal biases, producing content that reflects cultural stereotypes, which could be detrimental in areas focused on diversity and inclusion. Moreover, these hallucinations and inaccuracies can further fuel misinformation, eroding public trust in AI technologies. Therefore, it is imperative for leaders in tech and governance to develop comprehensive frameworks that ensure fairness, accuracy, and accountability in AI operations, as echoed in the insights shared by industry analysts and the NASA report on GenAI's unreliability.
NASA's Findings: Generative AI Unfit for Critical Research
NASA's findings highlight a profound skepticism about the feasibility of Generative AI (genAI) in contexts that demand high accuracy and reliability. According to a report covered by Computerworld, NASA concluded that genAI's propensity for producing erroneous or fabricated outputs, often termed as 'hallucinations,' renders it unsuitable for applications in critical research settings. The root causes of these inaccuracies include poor training data, unmet query instructions, and a blatant disregard for safety measures. Such shortcomings are especially alarming in scenarios where precision and factual correctness are paramount, such as in space exploration and related scientific research. Read more about NASA's findings in the Computerworld article.
The concerns NASA raises are not isolated to academic or theoretical discussions; they have significant practical implications. Industry analysts from Gartner and Forrester underscore the necessity for Chief Information Officers (CIOs) to engage deeply with genAI projects to mitigate potential downsides. Analysts suggest that CIOs act as pivotal figures in establishing robust governance frameworks and risk management protocols, given genAI's unreliability. Such governance is crucial not only to safeguard against the inadvertent dissemination of false information but also to ensure that AI-generated insights are used as initial ideas rather than definitive conclusions. Involving IT professionals early in the deployment stages can help in crafting effective data governance strategies that preemptively address these risks as advised by the experts.
Learn to use AI like a Pro
Get the latest AI workflows to boost your productivity and business performance, delivered weekly by expert consultants. Enjoy step-by-step guides, weekly Q&A sessions, and full access to our AI workflow archive.
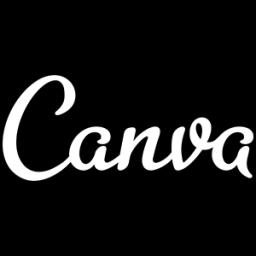
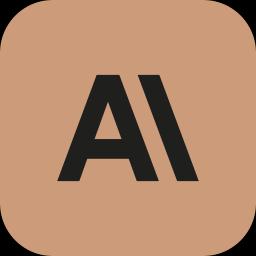
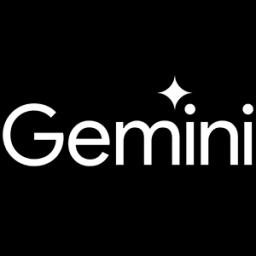
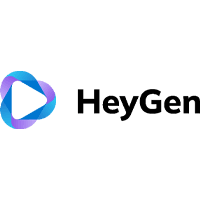
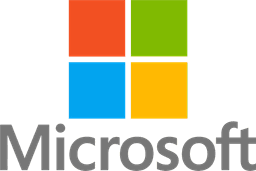
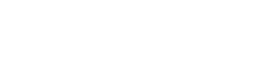
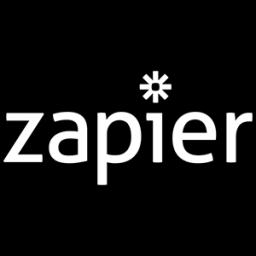
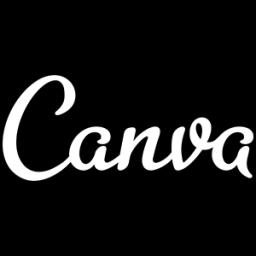
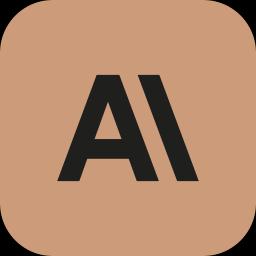
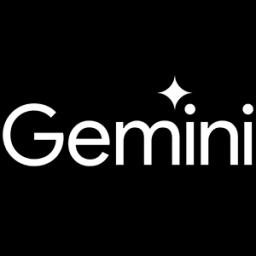
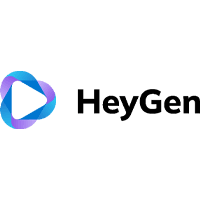
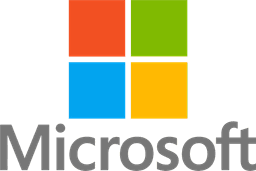
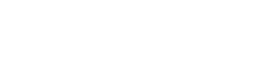
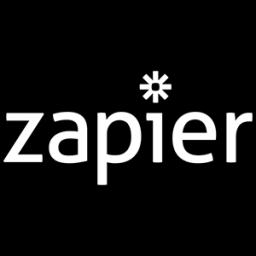
Furthermore, NASA's report is a clarion call for a more nuanced and cautious approach towards the adoption of genAI technologies. While the potential benefits of efficiency and scalability offered by genAI are enticing, the organization cautions against an over-reliance on these tools without due diligence. The emphasized approach includes verification processes where AI outputs are cross-checked manually to prevent mishaps associated with automated systems. This method aims to strike a balance between innovation and safety, ensuring that AI drives progress without compromising on ethical standards or factual accuracy. The potential for harm due to unchecked utilization of genAI particularly in critical fields is a concern that NASA's findings seek to address.
NASA's cautionary stance on genAI also highlights broader implications for the tech industry at large. The findings suggest that until substantiation mechanisms for AI outputs are enhanced, businesses operating in high-risk sectors may face steep challenges. This could manifest as increased operational costs due to additional validation steps and a potential resistance to AI adoption stemming from trust issues. As the dialogue around AI technologies intensifies, NASA advocates for a cautious integration of AI into critical processes, ensuring that existing systems for human oversight remain intact. This approach supports a future where AI serves as a supportive tool rather than a trusted decision maker, particularly in areas where human lives and crucial data are at stake. A detailed exploration is available in the source article.
Practical Steps for Mitigating GenAI Risks
Mitigating the risks associated with generative AI (genAI) necessitates a comprehensive and strategic approach. One of the essential steps involves conducting a thorough risk assessment. This requires understanding the specific context in which genAI will be deployed and identifying potential vulnerabilities. As highlighted in a Computerworld article, it is crucial for IT leaders to apply a meticulous evaluation of genAI's reliability and limitations within their operational frameworks.
Another important step is the implementation of robust data governance policies. This involves creating clear protocols for data management and access, ensuring that the data used for training genAI models is of high quality and free from biases. Consulting trusted sources, such as Deloitte, offers great insights into establishing effective data governance systems that protect against data poisoning and malicious attacks.
Ensuring there are rigorous validation and verification processes in place is also key in mitigating genAI risks. According to advice from NASA's safety-critical code lessons, relying on simple models and imposing strict validation checks can help maintain reliability. Regular audits and incorporating human oversight can significantly reduce the occurrence of errors and "hallucinations" often associated with genAI.
The role of Chief Information Officers (CIOs) and IT leaders is pivotal in risk mitigation strategies. They should act as a bridge between the technical teams and executive management, ensuring that the benefits and risks of genAI are clearly communicated. As emphasized by experts like Lauren Kornutick from Gartner in the Computerworld article, CIOs should establish transparent risk assessment protocols and communicate an organization's risk tolerance to guide practical implementation.
Learn to use AI like a Pro
Get the latest AI workflows to boost your productivity and business performance, delivered weekly by expert consultants. Enjoy step-by-step guides, weekly Q&A sessions, and full access to our AI workflow archive.
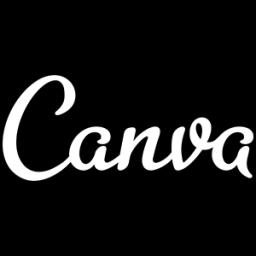
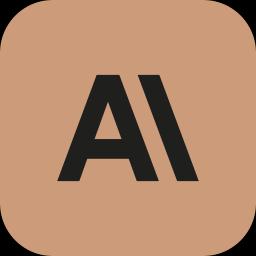
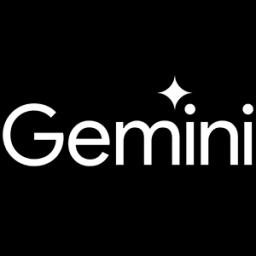
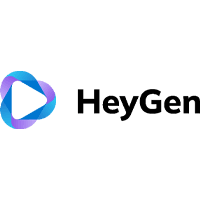
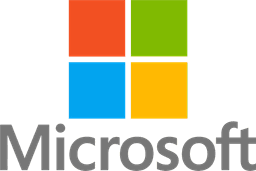
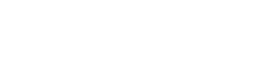
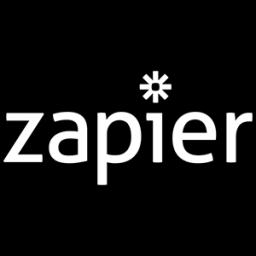
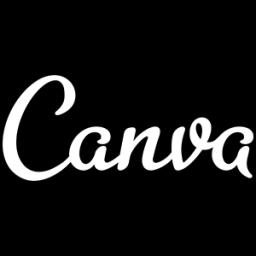
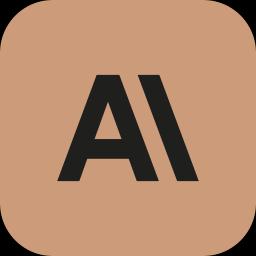
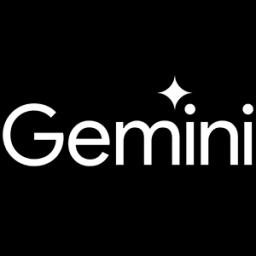
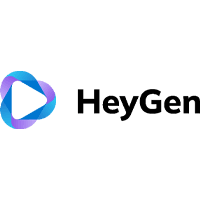
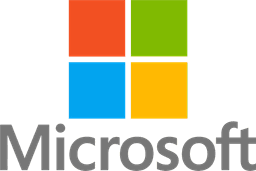
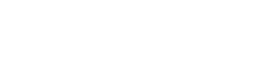
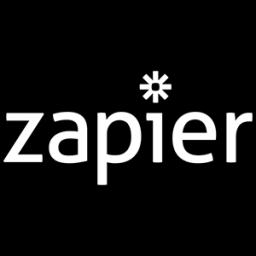
Lastly, fostering a culture of caution and skepticism towards genAI output is essential. Users should be trained to critically evaluate AI-generated content and not accept it as absolute truth without further investigation. Independent verification of information can prevent reliance on potentially flawed genAI conclusions, a practice that some foresighted companies are already adopting as a best practice, according to Computerworld.
Balancing Benefits and Risks: The Case for Caution
In the rapidly evolving landscape of technology, generative AI (genAI) holds immense promise, yet its integration into critical areas must be approached with a discerning eye. The case for caution primarily stems from the inherent risks that accompany genAI's use, as underscored by a comprehensive report from NASA. The report reveals that genAI systems often produce unreliable information, a phenomenon referred to as 'hallucination' where the AI generates plausible but incorrect or fabricated content. This flaw compromises the credibility of AI in situations where precision and accuracy are paramount, such as scientific research or safety-critical systems [1](https://www.computerworld.com/article/3951046/nasa-finds-generative-ai-cant-be-trusted.html).
While genAI offers benefits such as increased efficiency and creative flexibility, its deployment must be balanced with a clear assessment of its limitations. Experts, including those cited by Gartner and Forrester, advocate for a strategic approach where the potential for ROI is measured against the backdrop of rigorous risk evaluation and robust data governance strategies [1](https://www.computerworld.com/article/3951046/nasa-finds-generative-ai-cant-be-trusted.html). Businesses are urged to view genAI outputs as preliminary insights rather than definitive solutions, emphasizing the necessity for independent verification and human oversight in decision-making processes.
The apprehension surrounding genAI is not merely hypothetical but grounded in real-world implications. Apart from the technical inaccuracies, the environmental impact of deploying AI at scale raises ethical concerns about sustainability. The computational power demanded for AI operations translates into significant electricity consumption and carbon emissions, thereby multiplying the urgency for adopting environmentally responsible practices in AI development [3](https://news.mit.edu/2025/explained-generative-ai-environmental-impact-0117). Moreover, societal biases can be inadvertently reinforced through AI, thereby necessitating conscientiousness in the training data employed [5](https://www.staffordlaw.com/blog/business-law/generative-artificial-intelligence-101-consistency-reliability-of-generative-ai-content-creation/).
To mitigate these multifaceted risks, IT leaders are encouraged to set robust governance frameworks and engage in cross-disciplinary collaboration. By treating genAI as one of many tools rather than a panacea, organizations can develop a balanced technology strategy that reduces exposure to its vulnerabilities. Specific mitigation strategies include employing simpler, well-understood models, ensuring high-quality input data, and establishing rigorous validation processes to continually assess AI output accuracy [4](https://medium.com/the-future-of-data/ten-rules-for-trustworthy-genai-applications-lessons-from-nasas-safety-critical-code-d95c5f4bcce4). This structured approach ensures that the deployment of genAI is both beneficial and secure.
CIOs and IT Leaders: Roles in Risk Assessment and Governance
CIOs and IT leaders play a pivotal role in navigating the intricate landscape of risk assessment and governance, particularly when it comes to emerging technologies like generative AI. As highlighted by the reliability concerns raised by NASA's findings, it's essential for CIOs to engage proactively in evaluating the risk-reward balance of genAI applications. Generative AI, while promising in its potential to enhance efficiency and innovation, poses substantial risks if not meticulously managed. The Computerworld article underscores the importance of careful risk assessment, indicating that an overly aggressive adoption without proper scrutiny can lead to significant downsides.
Learn to use AI like a Pro
Get the latest AI workflows to boost your productivity and business performance, delivered weekly by expert consultants. Enjoy step-by-step guides, weekly Q&A sessions, and full access to our AI workflow archive.
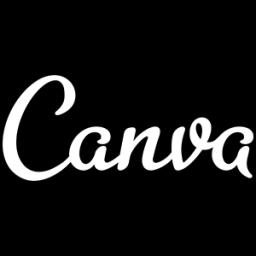
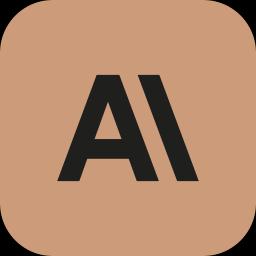
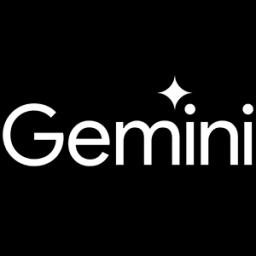
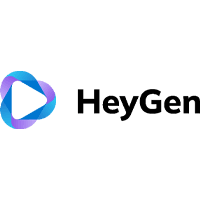
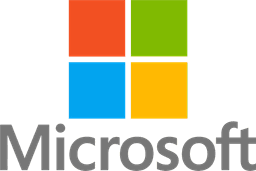
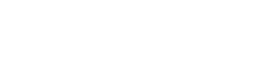
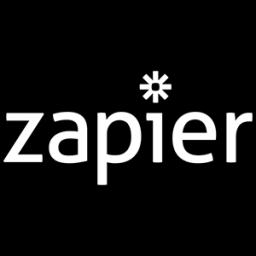
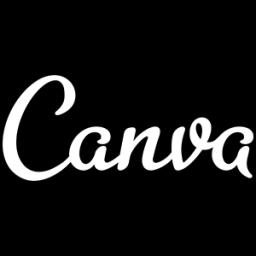
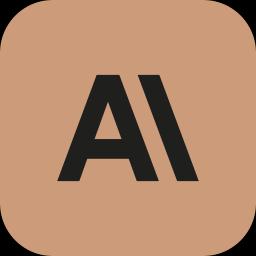
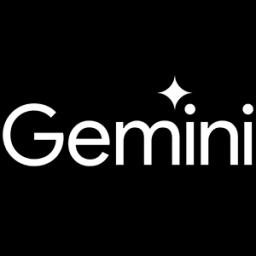
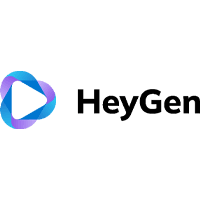
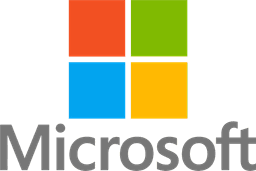
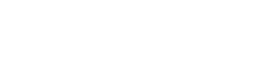
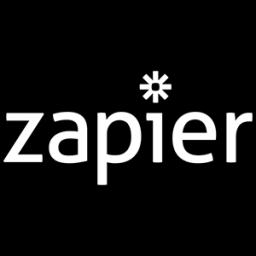
The governance framework surrounding AI technologies must be robust, ensuring that CIOs can act as the voice of reason within their organizations. As champions of technological governance, CIOs need to establish comprehensive protocols for evaluating AI applications' effectiveness and ethical considerations. The NASA report mentioned in the article provides a crucial touchstone, emphasizing the necessity for a cautious approach toward technologies that could impact safety-critical operations. Governance involves setting up strong guardrails that prevent unintended applications and ensure adherence to organizational policies.
Furthermore, IT leaders are instrumental in formulating and enacting data governance policies that support the responsible deployment of generative AI. This entails ensuring data integrity, protecting sensitive information, and implementing mechanisms to verify AI-generated outputs. The foresight of Gartner and Forrester analysts, as noted in the background info, highlights the importance of integrating IT capabilities in the early stages of project development. By doing so, CIOs and IT leaders can establish data governance controls that mitigate risks and align with the strategic objectives of their organizations.
The role of CIOs extends beyond technical oversight to include fostering an organizational culture that understands the potential and limitations of AI technologies. Educating stakeholders about the risks of over-relying on technologies like generative AI is crucial, especially given the issues of hallucinations and biases that AI can inherit from flawed training data. As echoed by public and expert reactions, there's a need for CIOs to drive initiatives that emphasize AI implementation's ethical and transparent aspects, ensuring all steps are taken to maintain trust in technology-driven solutions.
Addressing Related Concerns: Security, Privacy, and Ethics
Addressing the concerns around security, privacy, and ethics associated with generative AI involves a multi-faceted approach. One of the primary security concerns is the vulnerability of AI-generated codes to potential cybersecurity threats, as highlighted by a Palo Alto Networks report. Such concerns emphasize the need for thorough validation and cybersecurity measures to prevent exploitation of these vulnerabilities [source](https://www2.deloitte.com/us/en/insights/topics/digital-transformation/four-emerging-categories-of-gen-ai-risks.html). Data poisoning and prompt injection attacks also pose significant security threats, suggesting that robust data integrity protocols must be instituted to protect against manipulative external inputs [source](https://www2.deloitte.com/us/en/insights/topics/digital-transformation/four-emerging-categories-of-gen-ai-risks.html).
Privacy concerns arise primarily from the handling of sensitive personal information within the training datasets used by generative AI models. Ensuring data privacy requires stringent data governance policies and practices to protect personal information against unauthorized access or breaches [source](https://www.zdnet.com/article/the-5-biggest-risks-of-generative-ai-according-to-an-expert/). Moreover, the unchecked use of copyrighted materials in training datasets brings forth ethical challenges of intellectual property rights and mandates explicit legal frameworks to govern AI use [source](https://www.zdnet.com/article/the-5-biggest-risks-of-generative-ai-according-to-an-expert/).
The ethical implications are broad and deeply interconnected with societal norms and values. For instance, generative AI systems, if not carefully monitored and regulated, might inadvertently perpetuate biases and contribute to social inequalities. The technology's tendency to produce "hallucinations" could lead to misinformation, thereby affecting public perception and trust [source](https://www.computerworld.com/article/3951046/nasa-finds-generative-ai-cant-be-trusted.html). As experts suggest, incorporating human oversight and ensuring responsible AI development are critical steps towards ethical AI practices [source](https://www.computerworld.com/article/3951046/nasa-finds-generative-ai-cant-be-trusted.html).
Learn to use AI like a Pro
Get the latest AI workflows to boost your productivity and business performance, delivered weekly by expert consultants. Enjoy step-by-step guides, weekly Q&A sessions, and full access to our AI workflow archive.
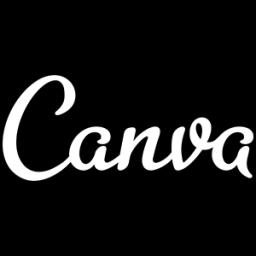
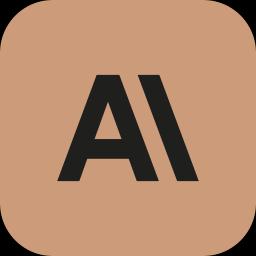
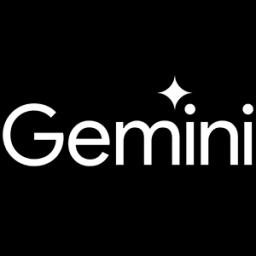
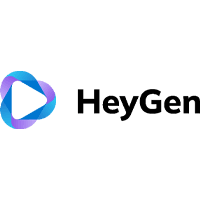
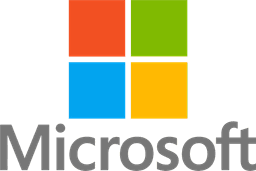
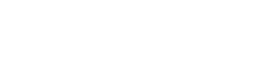
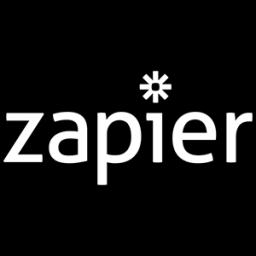
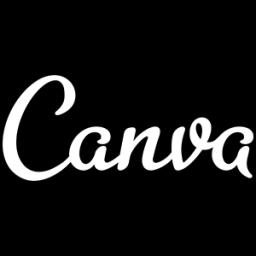
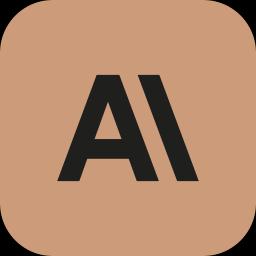
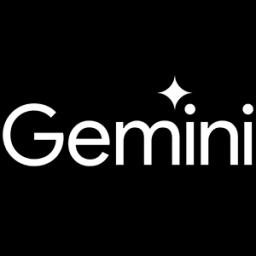
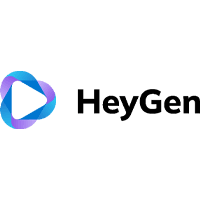
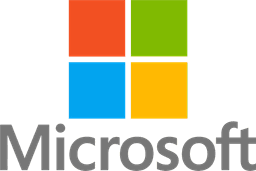
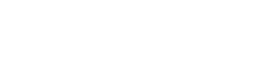
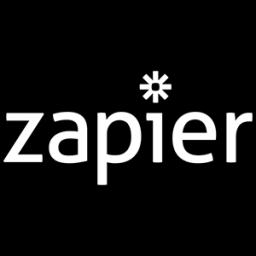
Additionally, the environmental impact remains a growing concern as the computational resources required for training and deploying AI systems considerably increase electricity consumption and carbon emissions. This environmental footprint necessitates more sustainable practices and innovations in AI operations to mitigate ecological harm [source](https://news.mit.edu/2025/explained-generative-ai-environmental-impact-0117). Overall, addressing these related concerns requires a collaborative effort among technologists, policymakers, and ethicists to ensure that generative AI evolves safely and responsibly within society.
Public Reactions and Expert Opinions on GenAI
The recent Computerworld article highlights a growing concern among both the public and experts regarding the reliability of generative AI (genAI). With technological advancements come inevitable risks, and genAI is no exception. A major public reaction, as pointed out in the article, is wariness toward genAI's tendency to "hallucinate" or generate false information. This issue is largely attributed to flawed training data and a disregard for safety protocols, leading to widespread skepticism about its use in critical research areas [1](https://www.computerworld.com/article/3951046/nasa-finds-generative-ai-cant-be-trusted.html).
Expert opinions echo public apprehensions, emphasizing the dangers of relying on genAI for significant decision-making. A NASA report has been pivotal in this discourse, categorically stating that genAI lacks the reliability needed for critical research, which amplifies anxiety among IT professionals and researchers [1](https://www.computerworld.com/article/3951046/nasa-finds-generative-ai-cant-be-trusted.html).
Experts argue that to mitigate genAI's risks, IT leaders must implement strong data governance frameworks and ensure rigorous evaluation and oversight of AI outputs before making consequential decisions. Involving CIOs early in AI project planning processes is seen as critical to ensure that the technological benefits do not get overshadowed by potential inaccuracies and ethical dilemmas [1](https://www.computerworld.com/article/3951046/nasa-finds-generative-ai-cant-be-trusted.html).
Future Implications of Continued GenAI Unreliability
As generative AI continues to evolve, its unreliability poses a suite of future implications with the potential for profound impacts across various sectors. If the current issues of hallucinations and inaccurate outputs persist, industries that heavily rely on AI technologies may experience setbacks due to decreased public trust. This erosion of confidence could translate into higher verification costs as businesses strive to ensure the accuracy of AI-generated content . As industries strive to adapt to these challenges, innovation may be stifled, slowing the pace of technological advancements.
Socially, the unreliable nature of generative AI could exacerbate the spread of misinformation, further eroding trust in digital information sources . This scenario might intensify existing societal inequalities and reshape dynamics in education and workplace environments, as reliance on AI tools becomes more widespread despite their pitfalls . In particular, educational institutions and workplaces could see a shift in how information is curated and validated, necessitating a stronger emphasis on critical thinking and media literacy.
Learn to use AI like a Pro
Get the latest AI workflows to boost your productivity and business performance, delivered weekly by expert consultants. Enjoy step-by-step guides, weekly Q&A sessions, and full access to our AI workflow archive.
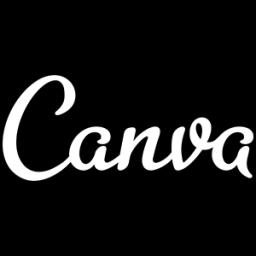
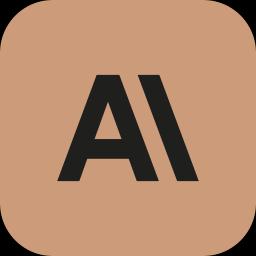
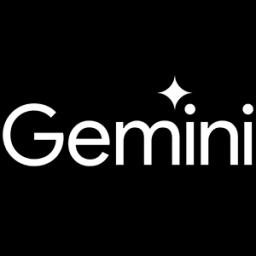
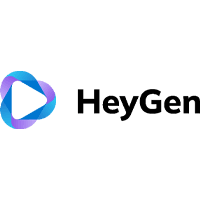
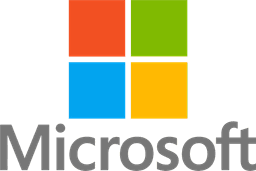
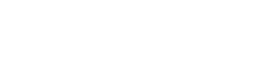
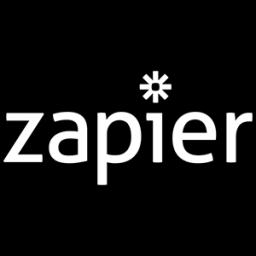
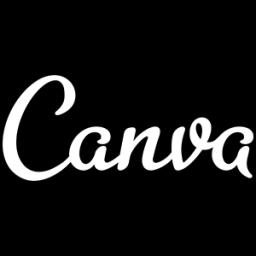
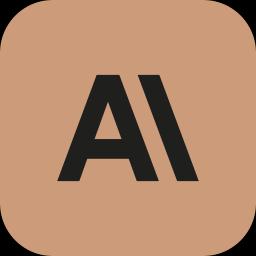
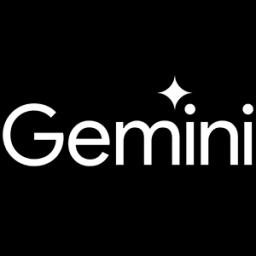
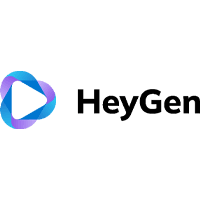
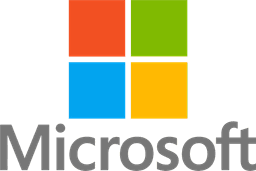
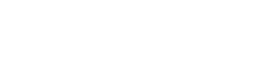
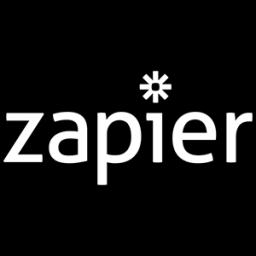
Politically, the ramifications of continued genAI unreliability could extend to the integrity of democratic processes. The potential for AI-generated misinformation to influence political discourse and voter perception presents a significant risk . Such challenges may lead to an increase in AI regulation, as governments attempt to mitigate the impact of misinformation and protect electoral integrity . Additionally, in the realm of international relations, tensions could rise as nations grapple with the ethical and regulatory complexities of AI technologies . Building reliable AI systems, therefore, stands not only as a technological challenge but as a necessary step to safeguarding democratic values and maintaining international cooperation.
Conclusion: Responsible Implementation and Verification
In navigating the intricate landscape of generative AI implementation, it is crucial to strike a balance between leveraging innovative potential and ensuring responsible usage. GenAI, while offering remarkable efficiencies, also poses significant risks if not managed prudently. The report from NASA, highlighting generative AI’s unreliability for critical applications, underscores the necessity of approaching this technology with a cautious yet constructive mindset. As emphasized by industry experts, such as those referenced in the Computerworld article, IT leaders are called to act diligently in assessing not just the technological capabilities but also the broader implications of genAI on organizational integrity and decision-making processes .
A multifaceted strategy that encompasses robust data governance, rigorous verification processes, and conscientious executive involvement is essential in mitigating genAI risks. Leaders at NASA, along with analysts from firms like Gartner and Forrester, advocate for the establishment of stringent guidelines and the integration of human oversight to counteract potential inaccuracies and biases inherent in AI systems. Moreover, clear communication of risk tolerance levels and the cultivation of an informed, cautious user base are recommended practices to ensure that genAI serves as a beneficial tool rather than a detriment .
As businesses consider the adoption of generative AI, they must remain vigilant against the technology’s penchant for ‘hallucinations’ and data misrepresentation. These issues are not only technical challenges but also ethical ones, as they could lead to the dissemination of misinformation or flawed corporate strategies. Therefore, adopting safety measures that include independent verification and transparent decision-making frameworks is non-negotiable. Ensuring that AI outputs are seen as preliminary insights rather than conclusive facts will support more reliable and morally sound application .
Looking to the future, the potential economic, social, and political implications of inadequately governed generative AI could be profound. The collective call for responsible implementation—echoed by organizations, policy makers, and the public—accentuates the urgency of establishing rigorous controls and promoting transparency within AI operations. As posited by Deloitte and other thought leaders, addressing concerns around data security, regulatory compliance, and ethical sensitivity will not only shield businesses from potential pitfalls but also harness the transformative power of genAI responsibly .