AI Breakthrough: More Efficient Models Emerge
New AI Model Surpasses DeepSeek, Requiring 86% Less Data!
Last updated:

Edited By
Mackenzie Ferguson
AI Tools Researcher & Implementation Consultant
Discover how a newly developed AI model is challenging the status quo by outperforming DeepSeek while needing a fraction of the data. This breakthrough could herald a new era of efficient AI solutions that are not only faster but also more accessible.
Background Information on AI in Data Usage
Artificial Intelligence (AI) has revolutionized the way data is utilized across various industries. The deployment of AI systems allows organizations to process and analyze large volumes of data efficiently, leading to insightful decision-making. A recent development in this field comes from a new AI model that remarkably outperformed the DeepSeek model, achieving similar results with 86% less data. This advancement is highlighted in an article on Techzine, showcasing the potential of AI to enhance data efficiency and efficacy without requiring extensive data sets.
The significance of AI in transforming data usage lies in its ability to uncover patterns and correlations that are often imperceptible through traditional data processing methods. AI technologies can augment data-driven strategies by offering predictive analytics, which enable businesses to anticipate market trends and consumer behaviors more accurately. The introduction of new AI models that minimize data requirements, as reported by Techzine, exemplifies a shift towards more sustainable computing practices, catering to industries that aim to reduce data storage costs and energy consumption.
Learn to use AI like a Pro
Get the latest AI workflows to boost your productivity and business performance, delivered weekly by expert consultants. Enjoy step-by-step guides, weekly Q&A sessions, and full access to our AI workflow archive.
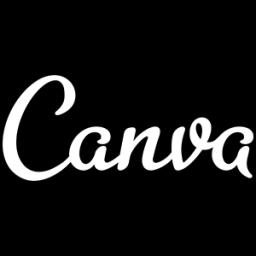
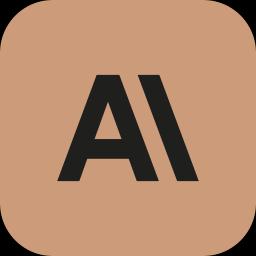
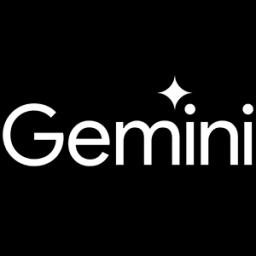
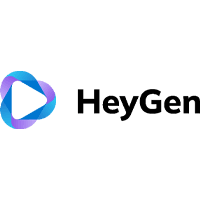
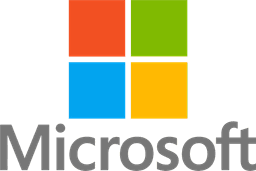
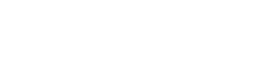
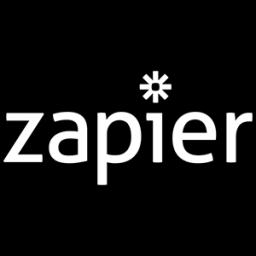
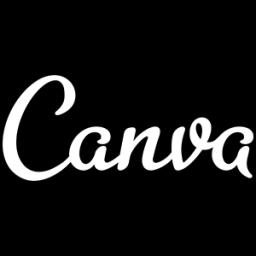
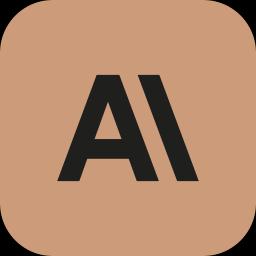
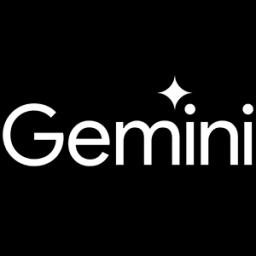
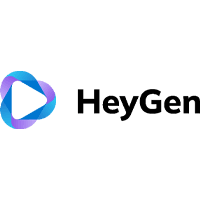
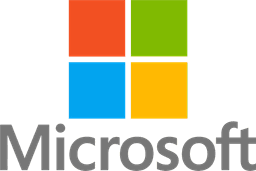
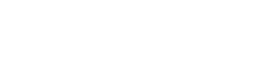
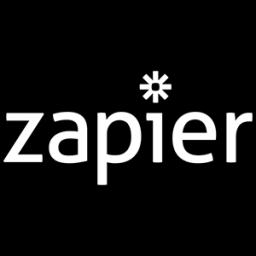
Public reactions to these advancements in AI data usage have been largely positive, as stakeholders—including businesses and consumers—recognize the benefits of reduced resource consumption without compromising results. Experts believe that such innovations will pave the way for broader AI adoption across sectors that were previously hesitant due to high data demands. Future implications of this trend may include a new era in AI development where the focus shifts towards achieving more with less, enhancing not only operational efficiencies but also promoting environmentally friendly tech practices.
With AI technology continually evolving, future implications of these advancements are promising. The ability to perform advanced data analytics with minimal data opens doors to a wide array of applications previously thought impossible. From improving healthcare diagnostics to refining supply chain management, the implications are vast and varied. As highlighted in Techzine's article, the continual development of data-efficient AI models could lead to enhancements across numerous fields, making AI an indispensable part of digital transformation strategies worldwide.
Overview of the New AI Model
The landscape of artificial intelligence (AI) has taken a significant step forward with the introduction of a groundbreaking new AI model that has surpassed previous benchmarks in efficiency and performance. This model stands out particularly because it requires 86% less data than its predecessor, DeepSeek, to achieve superior results, as highlighted by Techzine. The implications of such an advancement are vast, offering the potential to not only speed up the development and deployment of AI technologies but also to reduce the associated costs significantly.
Unlike previous models that demanded large volumes of annotated data to learn and perform tasks, this new model leverages an innovative architecture that optimizes learning efficiency. This efficiency gain could redefine the standards for data requirements in AI training, making it more accessible and versatile across various sectors and applications. As detailed in the Techzine article, such reduced data dependency not only enhances the model's speed but also broadens its applicability in real-world scenarios where data may be scarce or difficult to acquire.
Learn to use AI like a Pro
Get the latest AI workflows to boost your productivity and business performance, delivered weekly by expert consultants. Enjoy step-by-step guides, weekly Q&A sessions, and full access to our AI workflow archive.
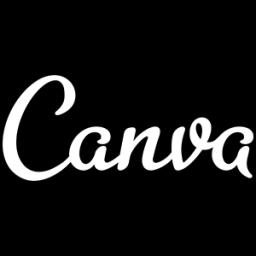
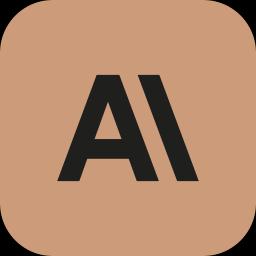
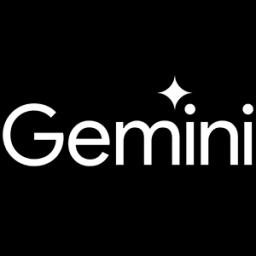
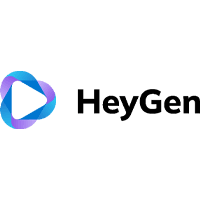
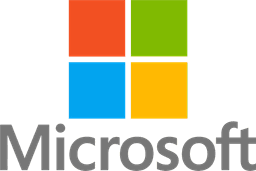
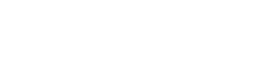
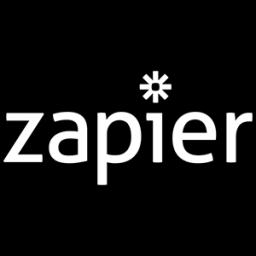
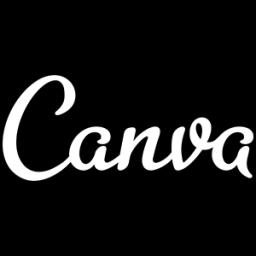
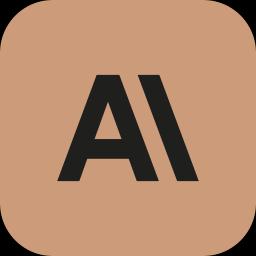
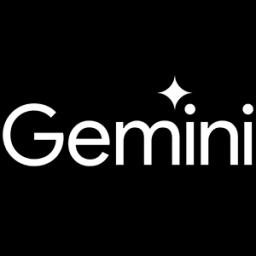
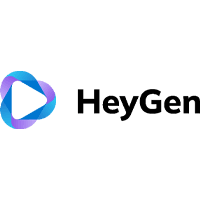
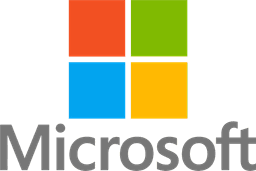
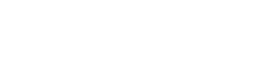
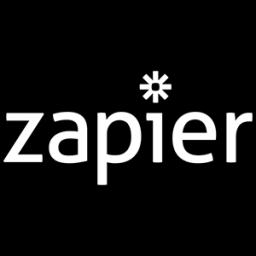
The success of this model is attributed to the collaborative efforts of a team that aimed to address the limitations seen in previous AI models, which often struggled with scalability and adaptability. By prioritizing a more resource-efficient approach, developers have positioned this new AI model as a frontrunner in the race towards more intelligent and sustainable technology solutions. According to insights from industry experts cited in Techzine, the model's ability to function effectively with less data may accelerate the integration of AI into industries that have been traditionally data-poor.
Comparative Analysis: New AI Model vs. DeepSeek
The global technology landscape is witnessing a significant evolution with the advent of a new AI model that reportedly outperforms the established DeepSeek system. According to a recent report, this innovative model achieves high efficiency, requiring 86% less data than DeepSeek to reach comparable, if not superior, levels of performance. Such advancements underscore the accelerating pace at which AI technologies are evolving, bringing forth new opportunities and challenges in the field (Techzine).
This breakthrough in AI capabilities highlights a shift in the approach towards data utilization. Traditionally, models like DeepSeek have relied on extensive datasets to train and refine their algorithms. The new AI model's ability to operate with substantially less data indicates a more efficient data processing and modeling strategy, which could have profound implications for sectors engaging with large data sets, such as healthcare, finance, and autonomous systems. The reduced data dependency not only promises lower operational costs but also a faster deployment cycle of AI innovations (Techzine).
While the technical specifics behind this new AI model’s architecture remain under wraps, industry insiders speculate that advancements in neural network structures and algorithm optimization are pivotal contributors to its success. Expert opinions also suggest that this model may leverage sophisticated machine learning techniques that prioritize pivotal data points, thereby minimizing redundancy and maximizing efficiency. This transformative approach not only enhances performance but also aligns with the growing demand for sustainable and scalable AI solutions (Techzine).
Data Efficiency in AI Models
As artificial intelligence continues to transform various industries, data efficiency in AI models has become a critical factor in advancing AI technologies. Unlike traditional models that rely heavily on vast amounts of data, the next generation of AI models is increasingly focused on achieving high performance with less data. This shift is essential not only for operational efficiency but also for democratizing AI applications, making them more accessible to organizations with limited data resources.
The development of AI models that require significantly less data is exemplified by recent breakthroughs, such as the new AI model that has reportedly beaten DeepSeek while utilizing 86% less data. According to TechZine, this model’s success underscores the advancements being made in creating more data-efficient algorithms. These innovations are particularly important as they help in reducing the computational costs and time associated with training AI models, making AI technology more sustainable and scalable.
Learn to use AI like a Pro
Get the latest AI workflows to boost your productivity and business performance, delivered weekly by expert consultants. Enjoy step-by-step guides, weekly Q&A sessions, and full access to our AI workflow archive.
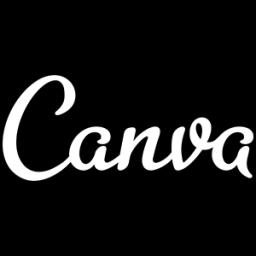
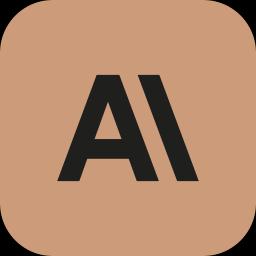
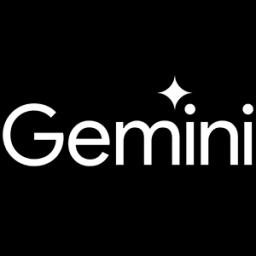
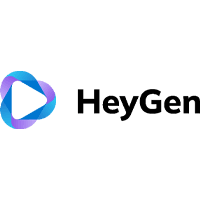
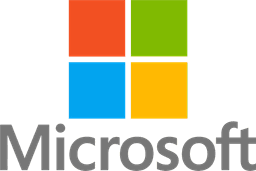
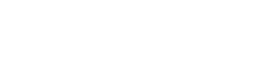
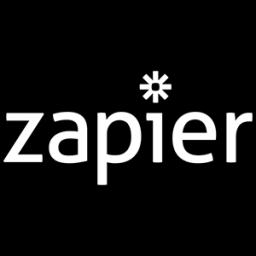
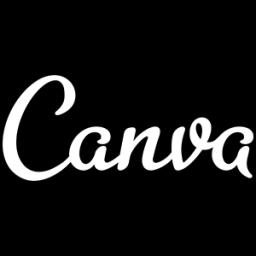
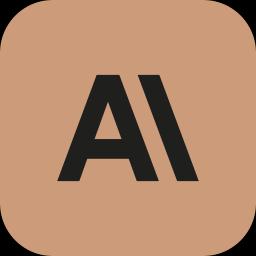
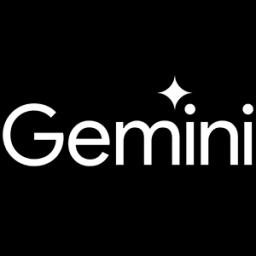
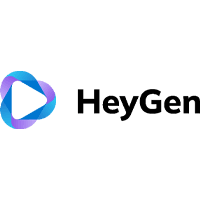
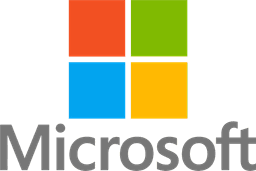
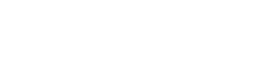
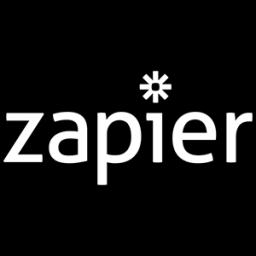
Data efficiency in AI is likely to have a profound impact on future AI development. By lowering the data barrier, more researchers and companies can experiment with AI technologies, leading to more innovation and potential breakthroughs. Moreover, such efficiency can lead to more robust models that perform well even with less-than-perfect datasets, enhancing their applicability in real-world scenarios where data is often noisy or incomplete.
Experts argue that focusing on data efficiency not only benefits the tech industry but also opens up opportunities for other sectors. For instance, in healthcare and agriculture, where data can be sparse or hard to obtain, efficient AI models can make a significant difference in outcomes by providing reliable insights and predictions with minimal data. This potential makes data efficiency a pivotal area of focus in AI research and development moving forward.
Related Developments in AI Technology
Recent advancements in artificial intelligence (AI) demonstrate a continual evolution of technology with far-reaching implications. Notably, a groundbreaking development has emerged, highlighting a new AI model that significantly surpasses the capabilities of its predecessors with remarkable efficiency. This model outperforms the DeepSeek AI technology, achieving similar results while requiring 86% less data, thus marking a significant stride in data-efficient learning techniques.
The significance of reducing data dependency cannot be overstated, as it directly influences the scalability and accessibility of AI systems. By minimizing the amount of data required for effective performance, this new AI model paves the way for more affordable and versatile applications, especially in domains where data collection poses substantial challenges.
These developments also resonate with ongoing discussions among experts concerning the future direction of AI. There is a growing consensus that models which can do more with less will lead the next wave of AI technologies, pointing towards a paradigm shift from data-intensive processes to smarter, leaner algorithms. This transformation is expected not only to enhance existing applications but also to unlock new potentials across various sectors.
Public reactions to such innovations have been mixed, reflecting both excitement for the technological possibilities and concerns over data privacy and ethical use. As AI models become more sophisticated and capable of functioning with limited datasets, stakeholders urge a balanced approach that incorporates robust ethical frameworks and transparent data handling processes.
Learn to use AI like a Pro
Get the latest AI workflows to boost your productivity and business performance, delivered weekly by expert consultants. Enjoy step-by-step guides, weekly Q&A sessions, and full access to our AI workflow archive.
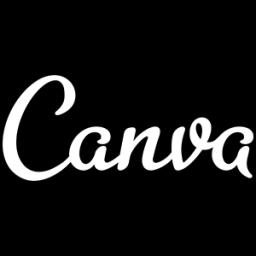
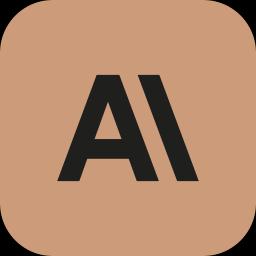
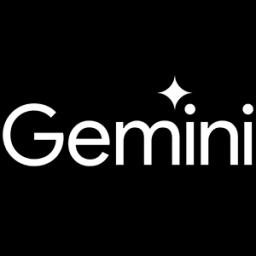
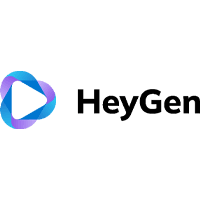
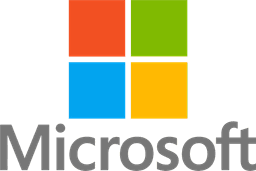
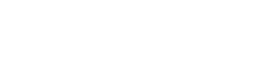
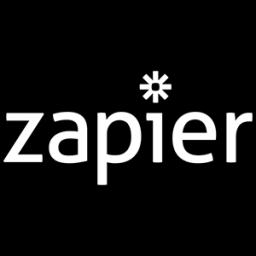
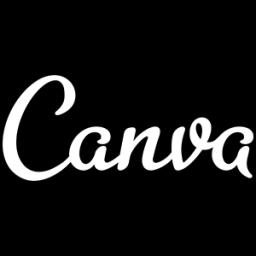
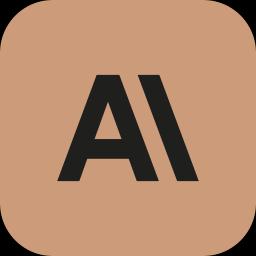
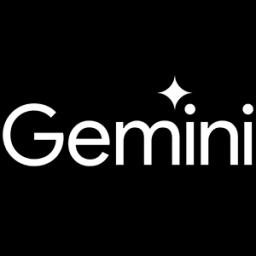
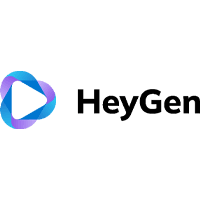
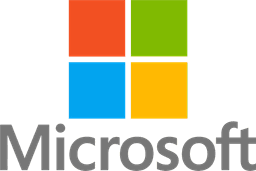
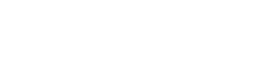
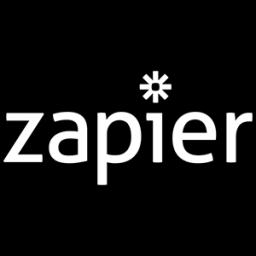
Looking ahead, these advancements may influence regulatory perspectives and policy-making in AI technology. Governments and industries might need to adapt to ensure that the benefits of new AI models are maximized while minimizing potential risks. This ongoing innovation in AI is poised not only to redefine current capabilities but also to extend the reach and impact of AI across numerous fields globally.
Expert Opinions on AI Advancements
The advancement of AI technologies continues to be a subject of both intrigue and debate among experts worldwide. Recently, a groundbreaking development was reported by Techzine, where a new AI model has been created that surpasses the performance of the renowned DeepSeek model. Remarkably, this new model operates with 86% less data, challenging the prevailing assumption that more data is always necessary for superior AI performance. For more details on this advancement, you can read the full article here.
Experts in the AI field have expressed a variety of opinions on the implications of such advancements. Some view this innovation as a positive trend towards more efficient AI models that do not require vast amounts of data, potentially making AI technology more accessible and less resource-intensive. On the other hand, some caution against over-reliance on these advancements without fully understanding the underlying mechanisms and potential loopholes. By innovating towards more efficient models, researchers may ensure broader applicability in diverse environments, consequently fostering a wider adoption of AI technologies globally.
The new model's triumph in beating DeepSeek with significantly less data has sparked a conversation about the future direction of AI research and application. There are discussions among researchers and developers on whether future AI models will follow this trend of emphasizing efficiency and minimal data utilization. Such a shift could pave the way for the next phase in AI evolution, where models not only perform better but also do so with reduced environmental impact and costs. This approach could democratize AI by making it feasible for smaller companies and educational institutions, who often cannot compete with the data-fed giants, to develop competitive AI solutions.
Public Reaction to the New Model
The unveiling of the new AI model that reportedly outperforms DeepSeek, while utilizing 86% less data, has sparked a variety of public reactions. According to a detailed report by Techzine, the news has resonated especially well with tech enthusiasts who are eager to see more efficient and powerful AI solutions. Many have taken to social media to express their optimism about what this development could mean for future AI applications, lauding the innovation as a significant step forward in AI technology.
On the other hand, some experts and casual observers alike have raised concerns about the broader implications of the new model's efficiency. Discussions have centered around questions of accessibility and the nature of data used to train such models. A variety of online forums have seen users engage in spirited debates, with comments under Techzine's article noting that while reduced data usage is a positive development, understanding and controlling the quality and origin of training data remains critical.
Learn to use AI like a Pro
Get the latest AI workflows to boost your productivity and business performance, delivered weekly by expert consultants. Enjoy step-by-step guides, weekly Q&A sessions, and full access to our AI workflow archive.
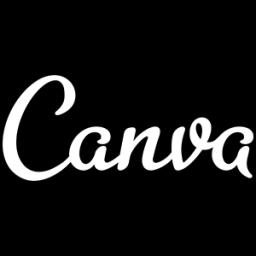
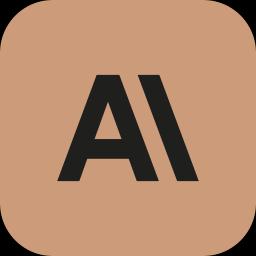
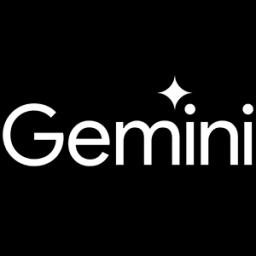
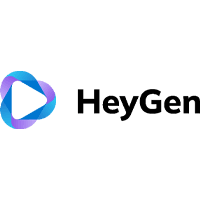
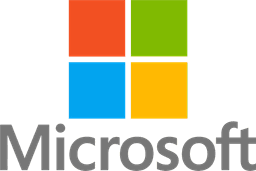
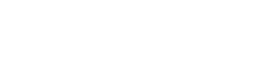
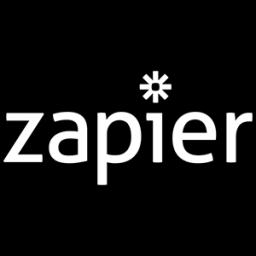
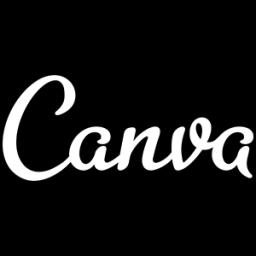
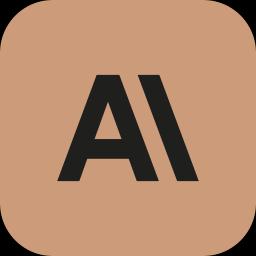
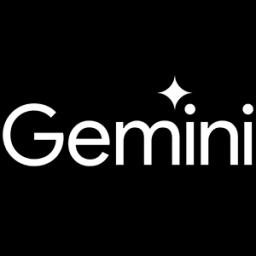
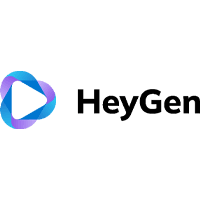
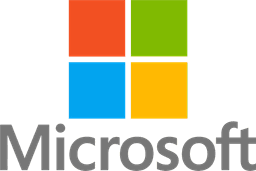
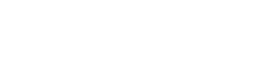
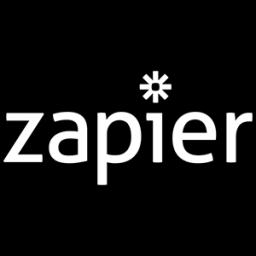
Public interest is also piqued concerning the potential shifts this might cause in the AI landscape. Many are speculating on whether such advancements will translate into widespread adoption across different sectors or stay confined within niche applications. This speculation highlights a growing curiosity about how new AI models like this one will integrate with existing technologies and what new possibilities they might unlock, as widely discussed in the recent article by Techzine.
Future Implications for AI and Data Usage
The landscape of artificial intelligence is witnessing a paradigm shift with the introduction of new AI models that require significantly less data to outperform existing technologies. One noteworthy development in this arena is the emergence of a new AI model that has managed to surpass DeepSeek while utilizing 86% less data, as reported by Techzine. This breakthrough holds substantial implications for the future trajectory of AI, particularly in enhancing the efficiency of data usage.
The innovation in reducing data dependency could democratize AI development, making it more accessible to organizations with limited resources. This could lead to a wider dissemination of AI tools across various sectors, enhancing productivity and innovation. The public, however, might hold mixed reactions, as concerns over data privacy and security remain prevalent. Regardless, the potential to train robust AI systems with less data might mitigate risk factors associated with large data collection and storage, as noted by experts in the field.
Moreover, the implications extend beyond just technological advancements; they venture into economic and ethical domains. The reduction of data required for AI training not only translates into cost savings for companies but also promotes a more sustainable approach in tech development. As the industry's giants and startups alike pay heed to this shift, the overarching narrative points towards a future where data frugality becomes a core tenet of AI advancement, reshaping both market strategies and regulatory frameworks globally.