When Math Meets AI and Controversy
OpenAI's Math Test Controversy: A Benchmarking Brouhaha
Last updated:

Edited By
Mackenzie Ferguson
AI Tools Researcher & Implementation Consultant
The AI world is abuzz with the recent controversy surrounding OpenAI's so-called privileged access to the FrontierMath benchmark. Critics are questioning the transparency and ethics of AI benchmarking, as claims of manipulation gain traction. Public trust is wavering as the call for industry-standard testing and verification protocols grows louder, emphasizing the need for clearer ethical guidelines in AI research.
Introduction to the OpenAI Math Test Controversy
The OpenAI math test controversy revolves around accusations of lack of transparency and potential unfair advantages granted to OpenAI in a benchmark test known as FrontierMath. This controversy was triggered by revelations of OpenAI's undisclosed relationship with another entity, Epoch AI, which allegedly provided them privileged access to test problems and solutions. Such access has raised questions regarding the authenticity and credibility of OpenAI's reported results.
The controversy has sparked wider discussions in the AI community about the importance of independent verification and transparency in AI benchmarking processes. With concerns over potential manipulation of results and unfair advantages for certain companies, there is growing demand for establishing standardized testing protocols to ensure the reliable evaluation of AI capabilities.
Learn to use AI like a Pro
Get the latest AI workflows to boost your productivity and business performance, delivered weekly by expert consultants. Enjoy step-by-step guides, weekly Q&A sessions, and full access to our AI workflow archive.
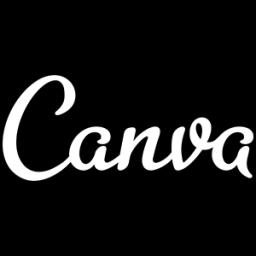
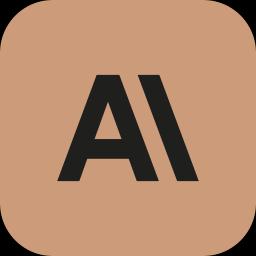
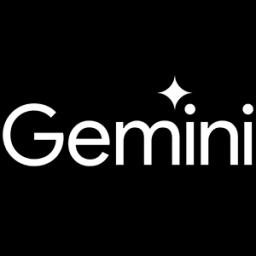
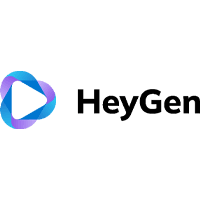
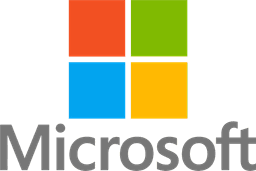
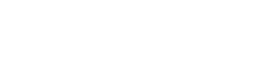
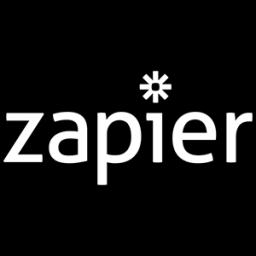
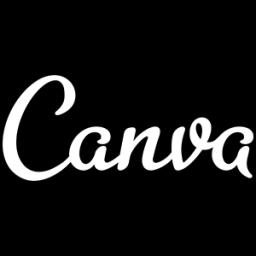
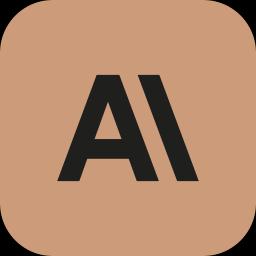
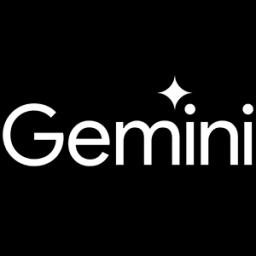
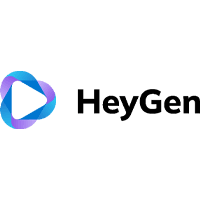
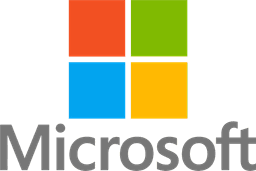
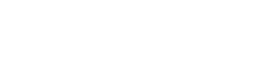
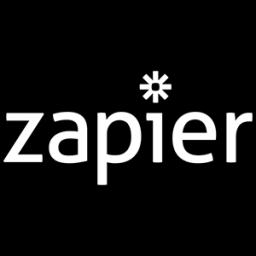
These events have highlighted a significant tension within the field of AI between rapid progress and the need for stringent ethical standards. The OpenAI math test incident is just one example of the challenges faced in creating robust systems for verifying the legitimacy of AI advancements. It underscores the need for cohesive industry standards that promote fairness and transparency.
Furthermore, this situation has major implications for public trust and the credibility of AI companies. Public skepticism could hinder further investments and technological advancements within the sector, pressuring companies and researchers to adopt clearer disclosure policies, much like those seen in Meta's FAIR Lab Benchmark Reform Initiative.
Overall, addressing these issues is crucial for maintaining integrity within the AI industry, fostering trust among stakeholders, and ensuring that AI technologies are developed responsibly and ethically. The controversy surrounding the OpenAI math test serves as a critical case study on the necessity for rigorous oversight in AI benchmarking and validation processes.
Background: The Role and Evolution of AI Benchmarking
The field of AI benchmarking has evolved rapidly over the past few years, becoming a critical component in evaluating the progress and capabilities of artificial intelligence systems. Initially, AI benchmarks served as a means to quantify the performance of AI models in specific tasks, such as image recognition, natural language processing, and machine learning. As AI technologies advanced, so did the complexity and scope of benchmarks, necessitating more rigorous and comprehensive evaluations.
Learn to use AI like a Pro
Get the latest AI workflows to boost your productivity and business performance, delivered weekly by expert consultants. Enjoy step-by-step guides, weekly Q&A sessions, and full access to our AI workflow archive.
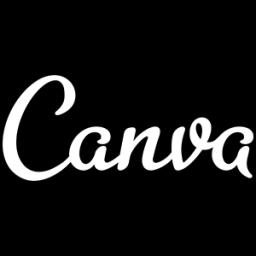
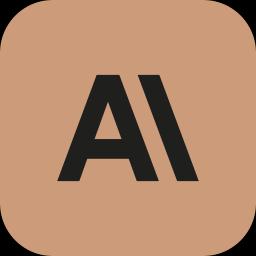
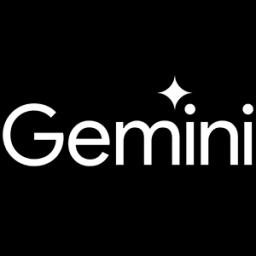
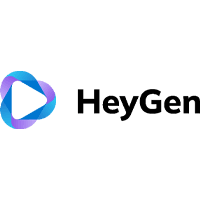
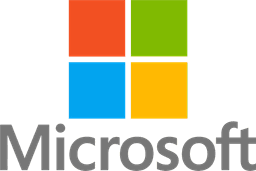
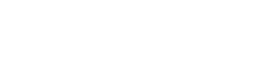
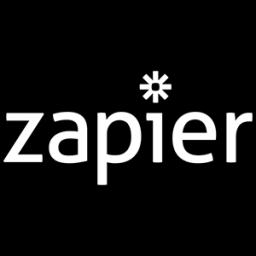
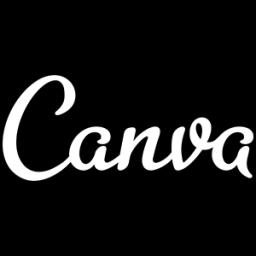
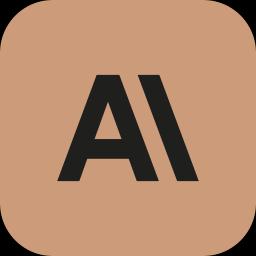
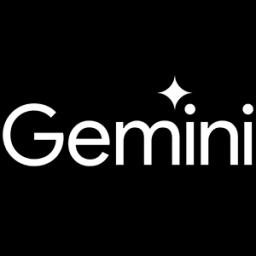
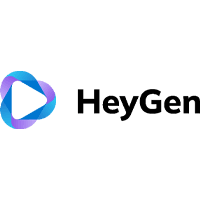
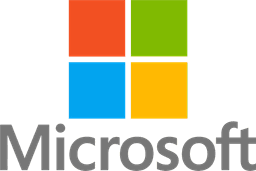
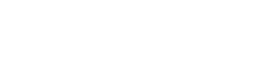
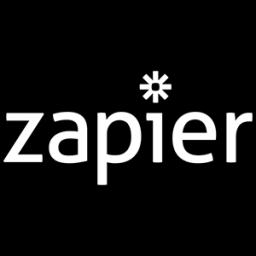
AI benchmarking not only provides a mechanism for measuring AI performance but also facilitates transparency and comparability across different AI systems. This transparency is crucial for researchers, developers, and stakeholders to understand and trust the capabilities and limitations of AI technologies. However, the growing influence and implications of these benchmarks have also sparked controversies, particularly regarding their validity and the potential for manipulation. These concerns underscore the need for robust benchmarking standards and independent verification processes.
The controversy surrounding OpenAI's math test and the FrontierMath benchmark highlights the challenges faced in AI benchmarking. Access restrictions and lack of transparency about the benchmarking process have led to skepticism and raised concerns about the integrity of AI evaluations. This incident illustrates the broader issues within the industry and the necessity for established protocols that ensure fair and unbiased testing environments.
Despite these challenges, notable advancements continue to be made in the field. Google's DeepMind, Meta's FAIR Lab, and Anthropic have all made significant contributions to developing transparent and reliable benchmarking methods. Their efforts emphasize the importance of transparency, independent evaluation, and standardized testing protocols in maintaining the credibility of AI research and development.
Looking forward, the establishment of international standards and coalitions, like the International AI Testing Standards Coalition, represents a positive step toward mitigating the controversies associated with AI benchmarking. These efforts contribute to the creation of a more trustworthy framework, where AI technologies can be evaluated fairly and accurately, ultimately reinforcing public confidence in AI advancements.
Recent Events in AI Testing and Benchmarking
In recent months, a series of events has drawn significant attention to the domain of AI testing and benchmarking. Central to this discussion is the controversy surrounding OpenAI's math test known as FrontierMath, which has been criticized for a lack of transparency and potential manipulation. This has sparked broader conversations about the integrity of AI benchmarking processes.
The background information highlights the challenges in accessing certain articles for complete details. A recent headline suggested controversy surrounding an OpenAI math test, yet without availability to the full article, understanding the nuances remains difficult. To responsibly cover such topics, access to complete articles and official statements, such as those from OpenAI, would be essential.
Learn to use AI like a Pro
Get the latest AI workflows to boost your productivity and business performance, delivered weekly by expert consultants. Enjoy step-by-step guides, weekly Q&A sessions, and full access to our AI workflow archive.
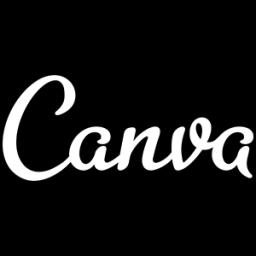
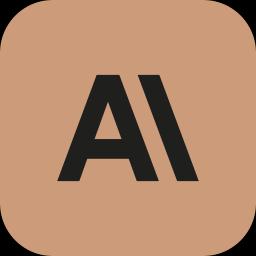
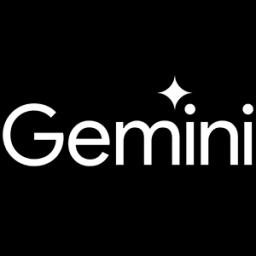
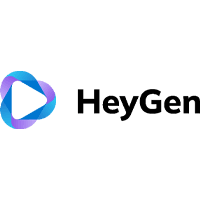
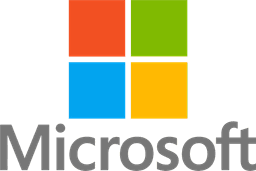
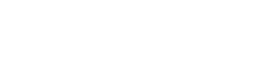
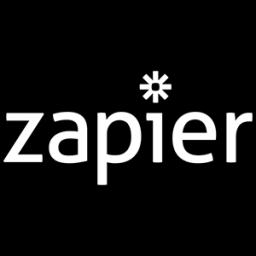
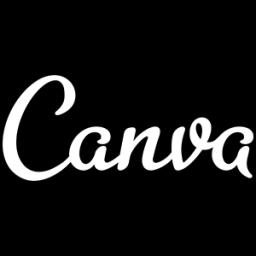
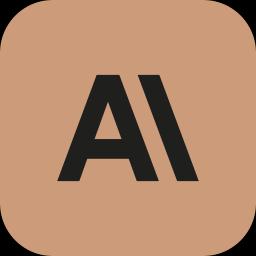
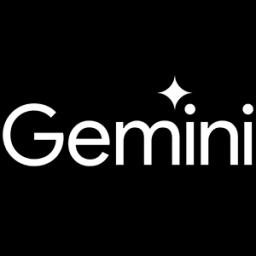
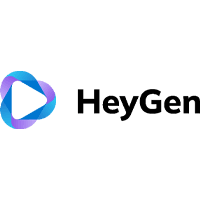
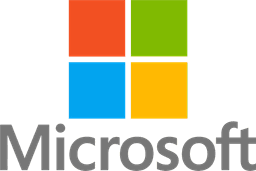
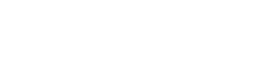
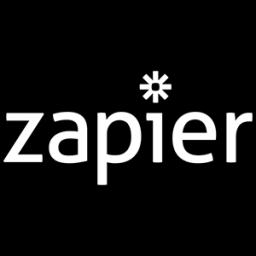
Relatedly, various key events have underscored the importance of transparency and independent verification in AI testing. DeepMind's success with AlphaGeometry, Meta's initiative to reform AI benchmarking standards, Claude 3's independent mathematics evaluation, and the formation of an international AI testing standards coalition showcase a collective effort to establish fairness and reliability in AI assessments.
Expert opinions are divided on the FrontierMath controversy. While some see OpenAI's privileged access to benchmarking problems as problematic, others defend the legitimacy of their results though they agree on the importance of independent verification. This dichotomy reflects an ongoing debate about ethical standards and transparency in AI research.
Public reaction to these revelations has been largely critical, with accusations of unfair practices levied against OpenAI. Concerns over undisclosed relationships and privileged access have led to demands for more stringent ethical guidelines in AI benchmarking. The lack of a publicly binding contract between involved parties exacerbates public mistrust.
The future implications of these controversies are profound. Trust in AI technology may dwindle, leading to more rigorous oversight and disclosure requirements. An increased emphasis on independent verification could not only enhance AI benchmarking processes but also foster better collaboration between academic and commercial entities, albeit potentially at the cost of slower technological advancement.
Analysis of Expert Opinions on the OpenAI Math Test
The OpenAI math test has sparked considerable discourse among experts, with varied interpretations of the circumstances surrounding its execution and subsequent analysis. Tamay Besiroglu, an associate director at Epoch AI, expressed concerns about the transparency of OpenAI's access to the FrontierMath problems, admitting a missed opportunity for clarity in disclosure agreements. He reflected on the lack of a negotiated right for full transparency until the test's announcement, suggesting this as an oversight in their initial collaboration agreements.
Conversely, Elliot Glazer, the lead mathematician at Epoch AI, has stood by the integrity of OpenAI's results, affirming that he believed the scores to be genuine. Glazer asserted that OpenAI held no interest in distorting their outcomes, although he acknowledged the necessity for independent scrutiny to validate these outcomes. This emphasizes an ongoing narrative in AI development: the demand for independent evaluation to maintain credibility and prevent biases.
Learn to use AI like a Pro
Get the latest AI workflows to boost your productivity and business performance, delivered weekly by expert consultants. Enjoy step-by-step guides, weekly Q&A sessions, and full access to our AI workflow archive.
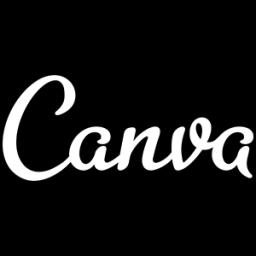
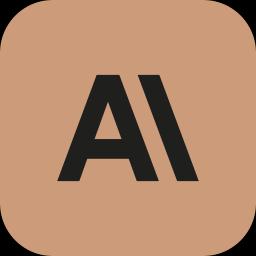
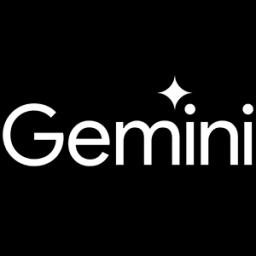
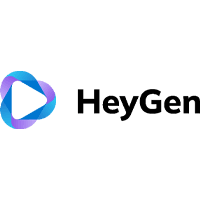
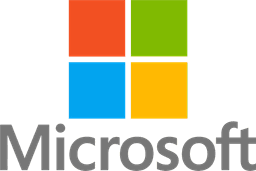
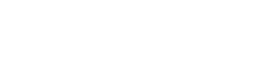
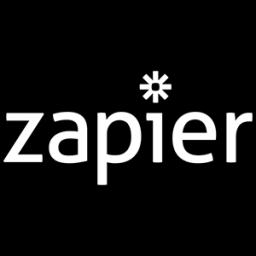
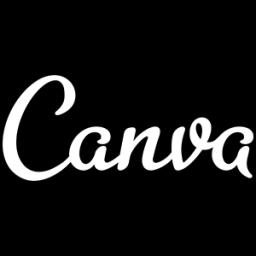
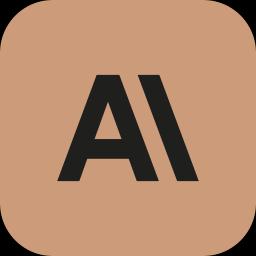
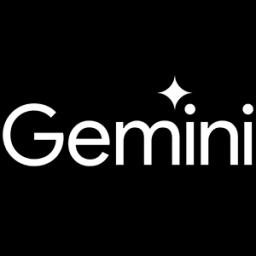
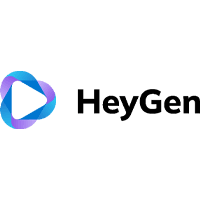
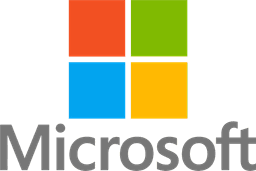
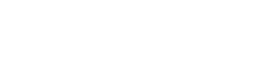
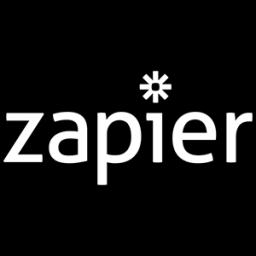
The dichotomy in expert opinions underscores a broader issue in AI testing and validation — the balance between proprietary advancements and the ethical expectation for transparency and verifiable claims. Such debates are crucial in maintaining the legitimacy and progression of artificial intelligence as a field and are pivotal in shaping how future collaborations and testings are approached within the industry. This debate also highlights the persistent need for stringent and transparent methodologies in AI evaluations to ensure the community’s trust and the field’s progression.
Public Reactions to the OpenAI-Epoch Benchmarking Scandal
The OpenAI-Epoch benchmarking scandal has been met with significant public backlash, casting a spotlight on issues of transparency and ethics in AI research. Following revelations that OpenAI had a previously undisclosed financial relationship with Epoch AI and privileged access to the FrontierMath benchmark, critical and skeptical responses have dominated public discourse.
Social media erupted with accusations of manipulation and unethical behavior. Platforms like Reddit saw users expressing disappointment, while on X (formerly Twitter), critiques pointed to a lack of transparency from OpenAI, underscoring that even the creators of the benchmark were unaware of OpenAI's access. These reactions underline broad concerns that prioritize ethics and fairness in AI development.
On forums like LessWrong, users demanded greater transparency in benchmark usage to ensure fair development of AI capabilities. Despite attempts by Epoch AI to defend their partnerships and decisions, these explanations have done little to mitigate public distrust. The ongoing scandal has prompted calls for the establishment of stronger ethical guidelines and transparency measures in AI benchmarking standards.
Implications for Trust and Credibility in AI
The recent controversy surrounding OpenAI's handling of an AI math test highlights significant concerns about trust and credibility in the field of artificial intelligence. The case underscores the perception of excessive privileged access and opacity surrounding AI performance claims, which stirs public skepticism and critics' accusations of manipulation. Such incidents risk eroding public trust, which is crucial for the industry's continued growth and acceptance. Public confidence is a foundational aspect upon which AI innovation is built. If users and stakeholders lose faith due to repeated occurrences of unreliability or potential duplicity, broader AI integration could be substantially impeded.
Additionally, the controversy provokes discussions about the necessity for transparent disclosure and consistent ethical standards within AI research and development. Current events and expert opinions indicate an increasing demand for verifiable results apart from the presenting institution. External validation, akin to that pursued by Anthropic's Claude 3 and supported by organizations like Meta and the International AI Testing Standards Coalition, could potentially address these credibility issues. The move towards enhanced oversight and the publishing of transparent methodologies reinforce the need for independent evaluation to corroborate reported AI capabilities.
Learn to use AI like a Pro
Get the latest AI workflows to boost your productivity and business performance, delivered weekly by expert consultants. Enjoy step-by-step guides, weekly Q&A sessions, and full access to our AI workflow archive.
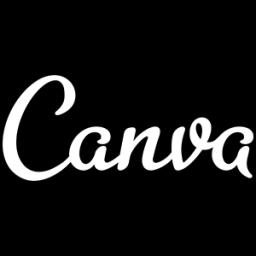
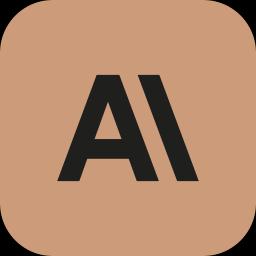
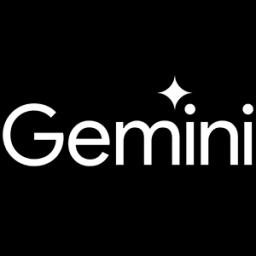
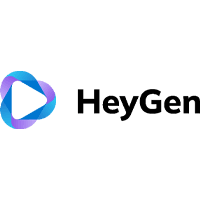
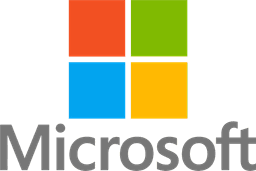
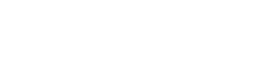
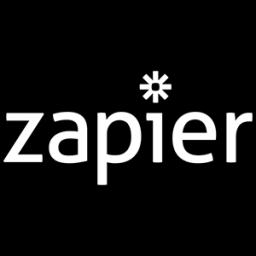
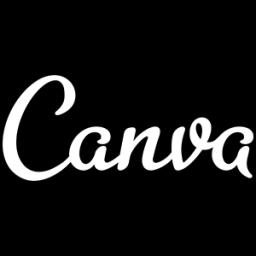
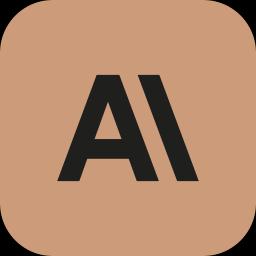
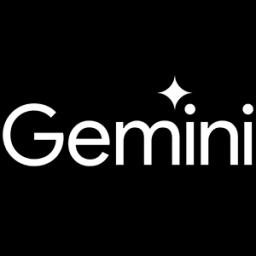
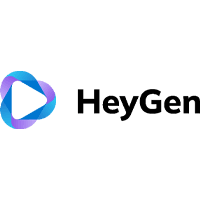
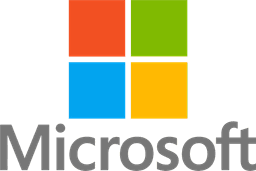
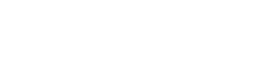
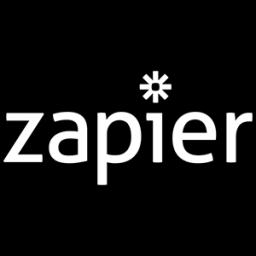
As a consequence, the AI industry's credibility may increasingly depend on adopting foolproof verification methods for benchmarking. Establishing universally acknowledged standards backed by independent third parties might reassure stakeholders of an AI system's real-world proficiency. This movement, albeit possibly imposing greater adherence costs, could shape a more dependable AI environment and discourage overblown or hedged claims about technological milestones.
Furthermore, the situation encourages a reevaluation of competitive dynamics within the AI sector. If transparency and trust become indispensable currencies, smaller enterprises may confront heightened barriers resulting from compliance demands. However, this shift could simultaneously level the playing field by prioritizing quality and integrity over sheer performance metrics or pioneering breakthroughs packaged without evidential robustness. Such a refocus might redefine the trajectory of AI advancements, emphasizing sustainable and ethical innovation over rapid, unchecked progress.
Lastly, stronger partnerships between academic bodies and private AI companies, governed by strict verification protocols, may enhance both parties' credibility. As the need for independent assessments grows, academic institutions could act as impartial validators, potentially revamping the traditional collaboration model with private firms. By ensuring that high standards align with commercial objectives, the AI development landscape could experience a transformation that prioritizes genuine impact and responsible stewardship over short-term gains.
The Future of Industry Standards and AI Testing Protocols
The ongoing debate around AI testing and industry standards is exemplified by recent controversies surrounding OpenAI's involvement in a math benchmark test. This controversy has brought to light significant issues concerning transparency, independent verification, and the propriety of testing protocols within the artificial intelligence sector.
The article titled 'An OpenAI Math Test Raises Eyebrows' underscores the importance of having full access to proprietary information when assessing the veracity of such controversies. Without it, any analysis remains speculative, highlighting the need for open disclosure from all parties involved in AI testing.
This necessity for transparency in AI testing has been echoed in various related events in the industry, such as DeepMind's verified achievements and Meta's move toward transparent benchmarking. These incidents showcase a trend towards a more rigorous and open approach to AI development standards.
Learn to use AI like a Pro
Get the latest AI workflows to boost your productivity and business performance, delivered weekly by expert consultants. Enjoy step-by-step guides, weekly Q&A sessions, and full access to our AI workflow archive.
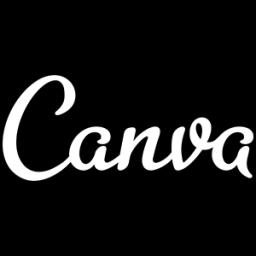
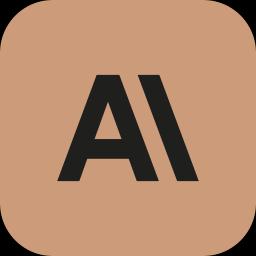
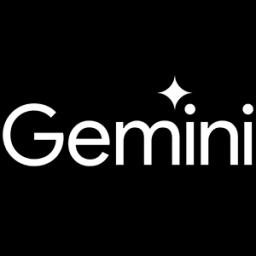
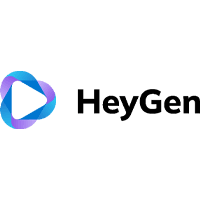
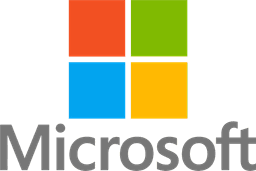
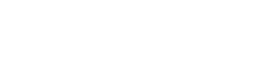
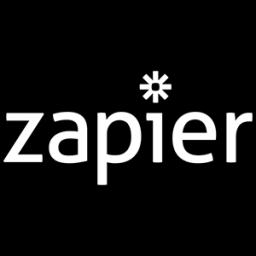
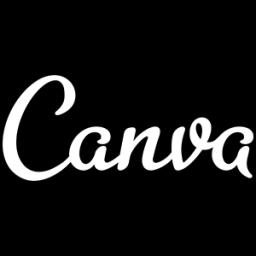
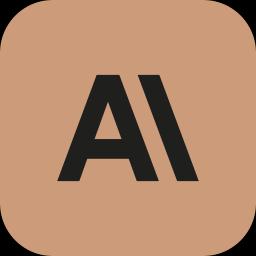
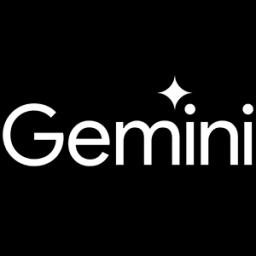
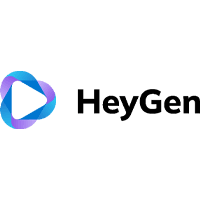
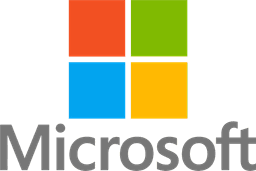
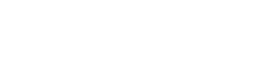
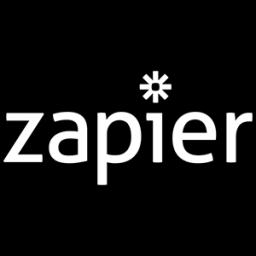
Expert opinions on the OpenAI controversy range from concerns over improper advantage due to undisclosed accesses, to views defending the integrity of the tests conducted, despite issues of transparency. The spectrum of these views indicates the ongoing complexity and diversity of perspectives within the AI and tech community.
Public reaction has largely been one of skepticism and critique, calling for more stringent ethical guidelines and clear transparency in AI testing protocols. This sentiment reflects a growing demand for accountability and fairness in AI development and deployment.
For the future of industry standards, this controversy suggests that more robust frameworks may need to be established to ensure that AI technologies are assessed accurately and fairly. This could involve independent verification, transparent processes, and possibly regulatory oversight to bolster public trust and industry credibility.
In terms of competition and innovation, any increase in compliance costs associated with new standards might affect smaller entities in the AI domain more significantly. However, the push for transparency could ultimately foster a more reliable development process that benefits the overall progress of AI technologies.
Impact on Competition and Innovation in AI
The controversy surrounding OpenAI's FrontierMath performance raises critical issues about competition and innovation in AI. As the capabilities of AI systems grow, so do concerns about how these technologies are evaluated and compared. OpenAI's undisclosed partnership with Epoch AI and privileged access to the FrontierMath benchmark highlight significant transparency issues that could affect market dynamics and trust in AI advancements.
The backlash from the public and experts alike underscores a vital point: for AI innovations to be accepted and integrated, they must be accompanied by rigorous and transparent validation processes. Companies that fail to adhere to these standards risk damaging their credibility, which could impede technological progress and adoption.
Learn to use AI like a Pro
Get the latest AI workflows to boost your productivity and business performance, delivered weekly by expert consultants. Enjoy step-by-step guides, weekly Q&A sessions, and full access to our AI workflow archive.
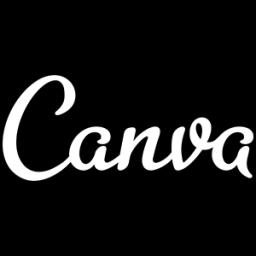
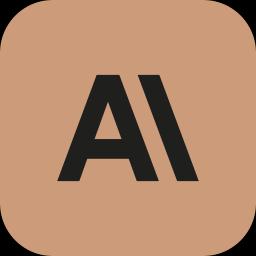
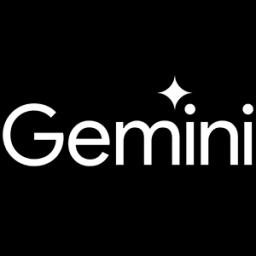
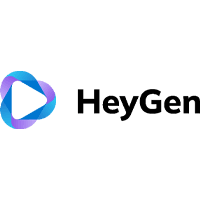
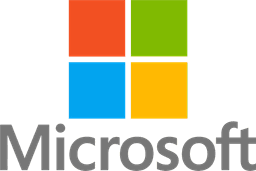
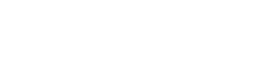
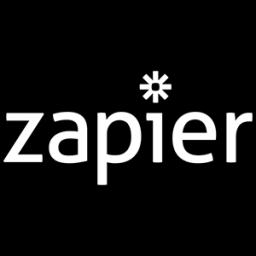
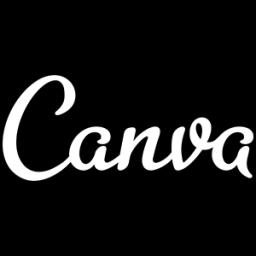
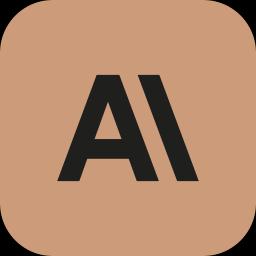
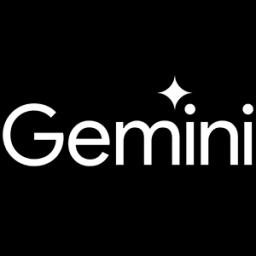
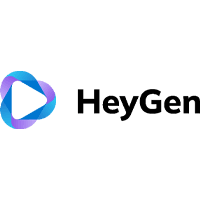
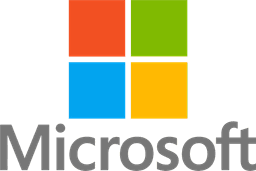
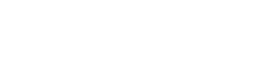
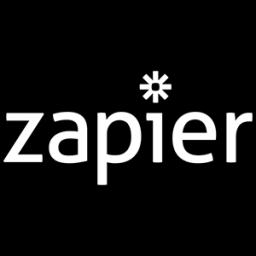
In response to this controversy, there could be a shift towards more stringent benchmarking standards and oversight in the AI industry, much like Meta's FAIR Lab Benchmark Reform Initiative. Independent validation and standardization efforts are crucial for fostering fair competition and innovation that is credible and trustworthy.
Moreover, the implications for smaller AI firms are profound. Increased compliance and transparency requirements might impose additional burdens, potentially stifling innovation due to resource constraints. However, these measures are necessary to ensure that all entities are held to the same ethical and performance standards, fostering an environment where innovation can thrive on merit rather than advantage or exclusivity.
These developments signify potential changes in how AI research collaborates with private companies. Stricter disclosure protocols and the demand for independent verification might push academic institutions and private firms to innovate more cautiously yet collaboratively, ultimately benefiting the field's integrity and progress.
Enhancing Collaboration Between Academia and Private AI Entities
The potential for collaboration between academic institutions and private AI companies is vast and promising. To unlock this potential, it’s crucial for both parties to cultivate an environment of mutual trust and transparency. National and international organizations can play a vital role by setting clear guidelines for ethical and open collaboration practices. Developing partnerships with clearly defined roles, shared benefits, and stringent ethical standards could enhance the quality and credibility of AI research and applications.
Current controversies surrounding AI benchmarking and transparency, as illustrated by the OpenAI-FrontierMath situation, underline the importance of independent assessments in AI testing. Academic institutions, with their robust research methodologies and focus on integrity, are well-positioned to conduct these evaluations. Collaborating with private companies can thus ensure that AI systems are tested under fair and neutral conditions, which builds trust not only among industry stakeholders but also with the general public.
Academic institutions offer an excellent platform for nurturing AI innovations and can help companies by providing a wide array of resources and a rigorous framework for testing and validation. By leveraging the strengths of academia, such as specialized knowledge, critical scrutiny, and an emphasis on ethical responsibilities, private AI entities can achieve advancements with greater public confidence and accountability.
Learn to use AI like a Pro
Get the latest AI workflows to boost your productivity and business performance, delivered weekly by expert consultants. Enjoy step-by-step guides, weekly Q&A sessions, and full access to our AI workflow archive.
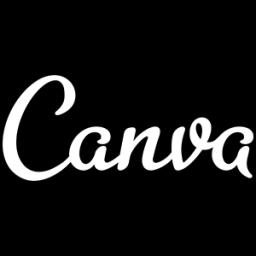
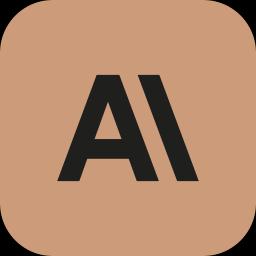
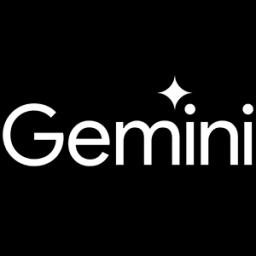
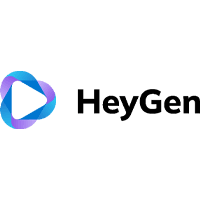
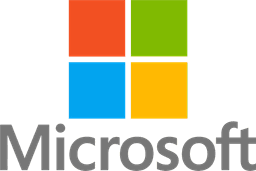
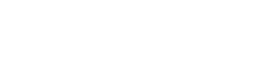
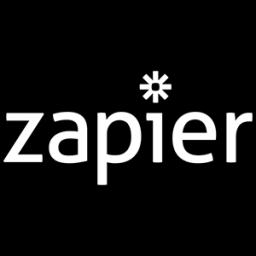
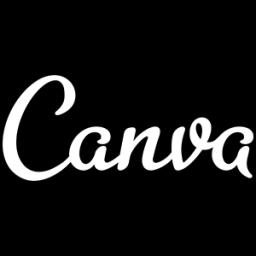
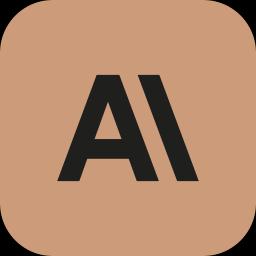
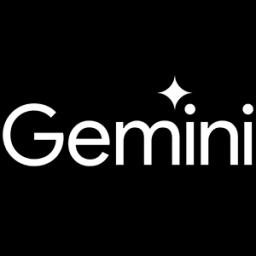
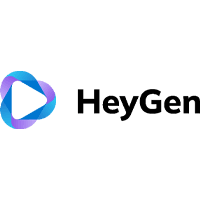
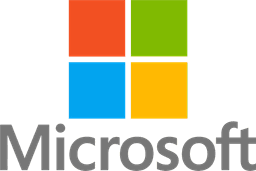
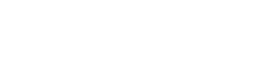
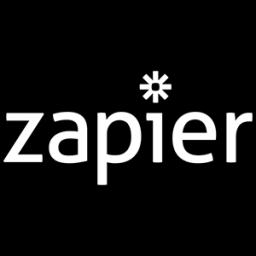
Both academia and private AI companies have much to gain from working together. Academia can benefit from the technical expertise, funding, and real-world applications that private companies offer. Meanwhile, private companies stand to benefit from academia’s extensive research capabilities and emphasis on long-term, impactful results that are ethically responsible and scientifically valid. This synergy can lead to breakthroughs that neither entity could achieve alone.
Future collaborations between academia and private AI firms should focus on transparency, ethical obligations, and the mutual benefits of shared knowledge. Together, they can form a balanced ecosystem that promotes innovation while also addressing public concerns about AI’s role in society. Establishing collaborative models that prioritize these elements can help mitigate controversies and enhance the overall trust in AI technologies.
Conclusion: Navigating Challenges in AI Benchmarking
The controversy surrounding AI benchmarking, particularly the recent OpenAI and FrontierMath affair, illuminates various challenges and opportunities involved in navigating AI advancements. AI benchmarking plays a crucial role in assessing artificial intelligence technologies and setting standards, yet the field is often marred with questions regarding transparency and credibility.
The issue of transparency is paramount. As evidenced by OpenAI's situation, the lack of disclosure about privileged access to benchmark datasets can lead to public distrust. The call for journalistic integrity in such matters highlights the need for press and media to ensure information accuracy before publicly disseminating findings. This situation underscores the importance of full disclosure and clarity regarding any relationships or conditions under which AI benchmarks are conducted.
The need for independent verification of AI benchmark claims also gained significant attention, as seen in the calls for Epoch AI to reassess OpenAI's results independently. As benchmarks serve to guide industry standards, independent third-party evaluations become critical to maintaining benchmark integrity, preventing manipulation, and ensuring that results are genuinely representative of AI performance.
In light of these challenges, several related events and initiatives by organizations such as Meta and the formation of the International AI Testing Standards Coalition reflect a broader move towards establishing more rigorous standards and transparent methodologies. These efforts indicate a shared recognition within the AI community of the need for standardized frameworks and transparent benchmarks to promote fair and credible evaluations.
Learn to use AI like a Pro
Get the latest AI workflows to boost your productivity and business performance, delivered weekly by expert consultants. Enjoy step-by-step guides, weekly Q&A sessions, and full access to our AI workflow archive.
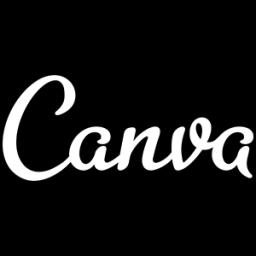
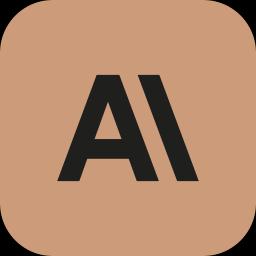
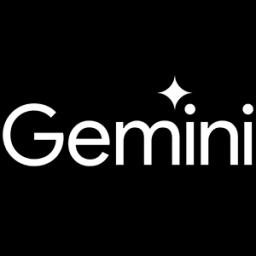
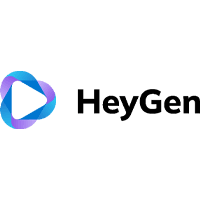
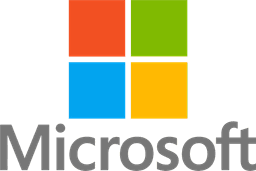
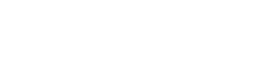
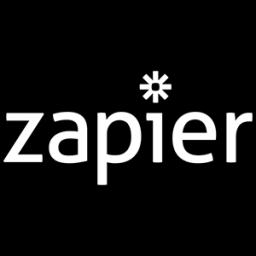
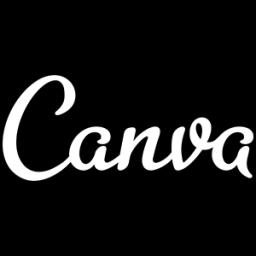
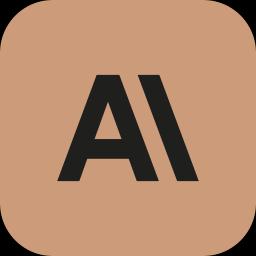
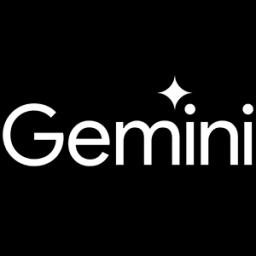
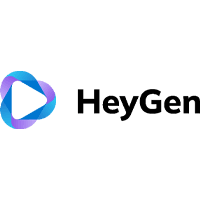
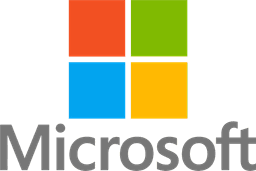
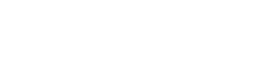
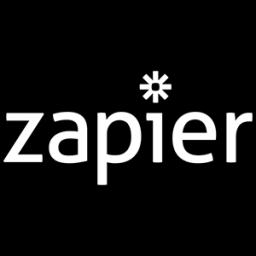
Public reactions to the OpenAI news have been largely critical, underscoring the critical role of public perception and trust in AI advancements. The skepticism in reaction to potential benchmark manipulation draws attention to the ethical considerations and the broader implications of AI testing standards on trust and industry credibility.
Looking ahead, the controversy stresses the importance of setting clear, impartial, and ethically sound benchmarking procedures. As AI technologies continue to proliferate, forging pathways towards transparent and truthful representations of AI capabilities becomes not only necessary but vital for the advancement and acceptance of artificial intelligence innovations. Overall, collaboration across academia, industry, and regulatory bodies is essential for ensuring the integrity of AI benchmarking processes in navigating these challenges.