Reinforcing Reason in AI
OpenAI's New AI Models o3 and o4-mini Elevate Reasoning through Reinforcement Learning
Last updated:

Edited By
Mackenzie Ferguson
AI Tools Researcher & Implementation Consultant
OpenAI's latest AI models, o3 and o4-mini, leverage reinforcement learning to revolutionize reasoning and enhance conversational abilities. Unlike traditional AI models, these new entrants in OpenAI's lineup use reasoning to consider multiple approaches before responding to queries, marking a significant shift in AI development following the depletion of available text data for training large language models. This advancement could have far-reaching implications across various sectors.
Introduction to OpenAI's New AI Reasoning Models
OpenAI's innovative AI reasoning models, notably o3 and o4-mini, represent a significant evolution in artificial intelligence technology. These models are designed to enhance the reasoning capabilities of AI by employing reinforcement learning techniques, which allow them to respond more intelligently and effectively to user queries. The integration of reinforcement learning is pivotal, as it equips these models with the ability to evaluate multiple approaches before generating a response, akin to how a human might ponder different solutions before making a decision. This method not only refines the decision-making process but also elevates the quality of interactions by generating nuanced and contextually appropriate responses. For more detailed insights into how these models function, you can check out the full article [here](https://indianexpress.com/article/explained/explained-sci-tech/ai-models-reason-reinforcement-meaning-openai-o3-o4mini-9970154/).
Unlike traditional large language models (LLMs) like ChatGPT, which often rely on pattern recognition and vast datasets to generate responses, OpenAI's o3 and o4-mini models inherently incorporate reasoning in their operations. This fundamental difference allows them to 'think' through a chain of thought before delivering an answer. The models dissect complex questions, consider varying perspectives, and analyze potential solutions to deliver more sensible and human-like responses. This approach provides a competitive edge, making these models better suited to tackle intricate and multifaceted queries. Explore more about these breakthroughs and their implications [here](https://indianexpress.com/article/explained/explained-sci-tech/ai-models-reason-reinforcement-meaning-openai-o3-o4mini-9970154/).
Learn to use AI like a Pro
Get the latest AI workflows to boost your productivity and business performance, delivered weekly by expert consultants. Enjoy step-by-step guides, weekly Q&A sessions, and full access to our AI workflow archive.
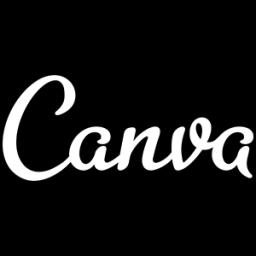
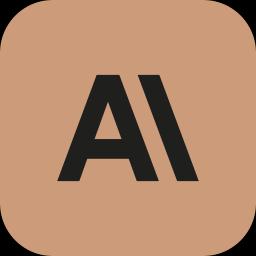
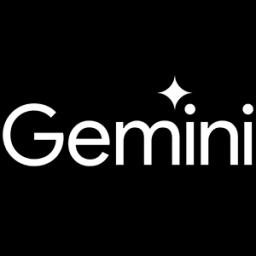
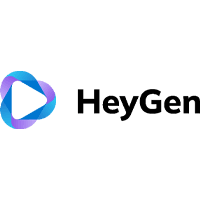
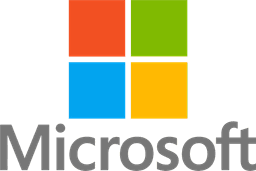
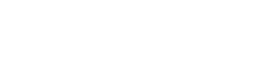
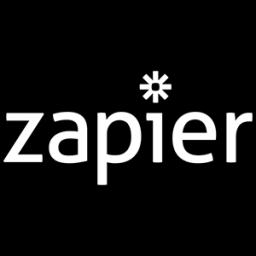
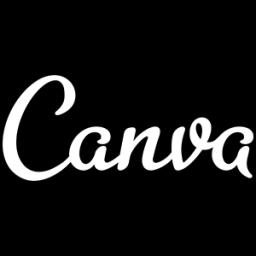
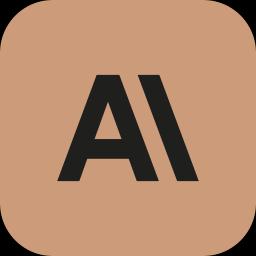
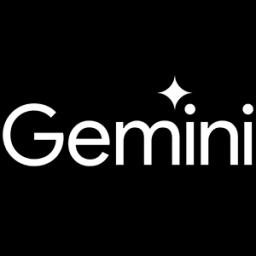
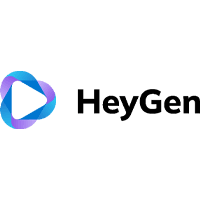
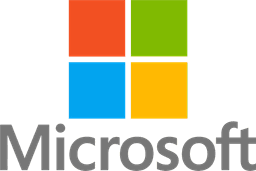
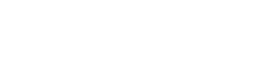
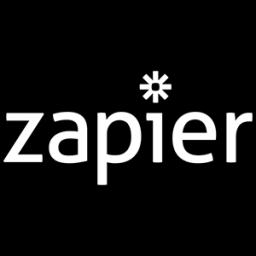
The move towards reasoning models marks a strategic shift for OpenAI, driven by limitations encountered by existing LLMs in terms of data availability and response sophistication. By the year 2024, the depletion of readily available text data for training had signaled the need for an innovative approach to AI development. OpenAI responded by crafting reasoning models that enhance performance through intelligent decision-making processes rather than sheer data volume. This shift not only addresses the saturation point of previous techniques but also opens up new possibilities for AI advancement and application, as further detailed [here](https://indianexpress.com/article/explained/explained-sci-tech/ai-models-reason-reinforcement-meaning-openai-o3-o4mini-9970154/).
Understanding Reinforcement Learning in AI
Reinforcement learning (RL) is a dynamic technique within artificial intelligence that leverages a trial-and-error method to optimize decision-making. Unlike traditional supervised learning, where models learn from a fixed set of examples, RL allows AI models to learn from their experiences by receiving rewards or penalties based on their actions. This method is akin to how living organisms adapt through learning and feedback from their environment. The integration of RL into AI models, such as OpenAI's reasoning models o3 and o4-mini, transforms their ability to process information and derive insights from complex datasets, leading to more nuanced and informed decision-making processes .
OpenAI's newest AI models, o3 and o4-mini, represent a significant step forward in how machines process and respond to queries. These models employ reinforcement learning to mimic the cognitive reasoning process employed by humans, allowing them to weigh different pathways and solutions before delivering a response. This shift from immediate answer generation to meticulous reasoning not only enhances the quality of responses but also aligns the models more closely with human thought processes. The integration of reinforcement learning has freed AI from the limitations of relying solely on extensive datasets, allowing for more flexibility and creativity in answering complex questions .
The emergence of reasoning-focused AI models marks a pivotal shift in the landscape of machine learning. OpenAI's o3 and o4-mini are designed to engage in deeper analytical processes, differentiating them from traditional models that rely heavily on pattern recognition. By embedding an internal chain of thought, these models can break down complex problems and explore multiple solutions before reaching a conclusion. Such advancements are critical as developers and researchers aim to create AI systems that not only perform tasks efficiently but also manifest a clearer understanding of nuanced and multifaceted issues .
Learn to use AI like a Pro
Get the latest AI workflows to boost your productivity and business performance, delivered weekly by expert consultants. Enjoy step-by-step guides, weekly Q&A sessions, and full access to our AI workflow archive.
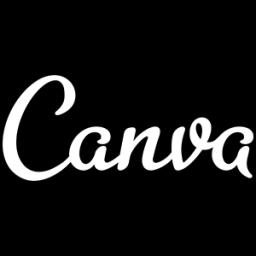
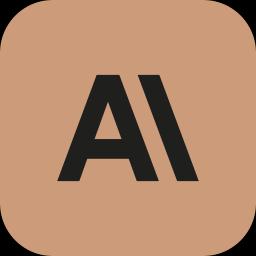
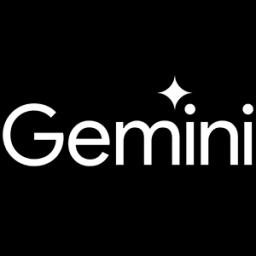
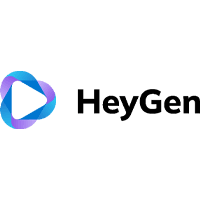
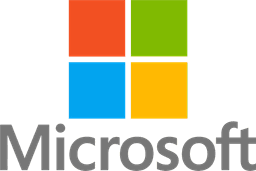
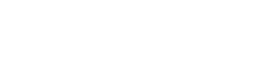
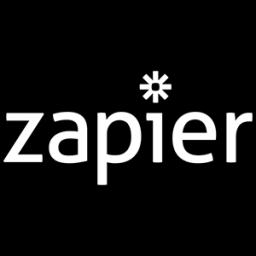
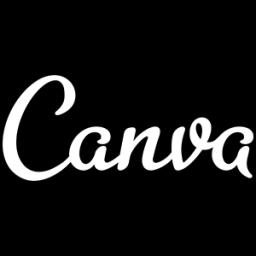
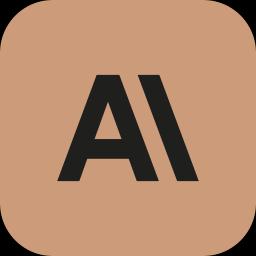
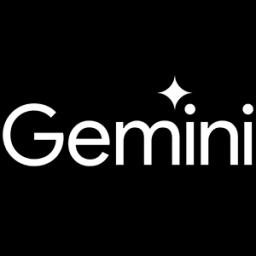
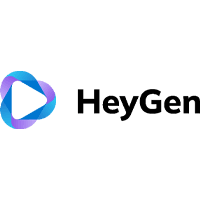
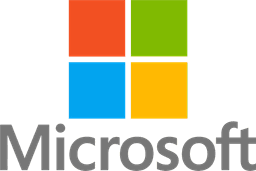
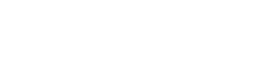
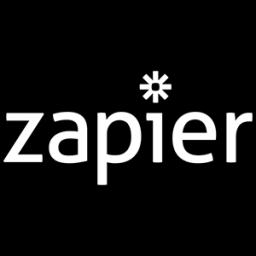
The drive to develop AI models with enhanced reasoning capabilities is partly due to the constraints of current language models, which have reached the limits of training with available text data. As these resources have been maximized, AI companies have shifted focus to incorporating reasoning through techniques like reinforcement learning. This approach not only addresses these training data limitations but also provides a framework for continuous improvement. Ultimately, this shift towards reasoning in AI models like o3 and o4-mini signifies a promising direction for the future of AI, aimed at achieving higher problem-solving capabilities and more robust interactions in diverse settings .
The Importance of Reasoning in AI
In the rapidly evolving landscape of artificial intelligence, the integration of reasoning models marks a pivotal shift in how AI systems operate and interact with humans. The newly introduced AI reasoning models by OpenAI, specifically o3 and o4-mini, leverage reinforcement learning to enhance their decision-making capabilities. Unlike their predecessors, these models do not merely rely on vast datasets for training but engage in a process that involves evaluating multiple potential solutions before arriving at a response. This approach not only improves accuracy but also allows for more human-like interactions [1](https://indianexpress.com/article/explained/explained-sci-tech/ai-models-reason-reinforcement-meaning-openai-o3-o4mini-9970154/).
Reinforcement learning, a technique central to these models, involves the system learning through a reward and punishment mechanism, akin to training an animal. Successful interactions are rewarded, thereby reinforcing desired behaviors, while mistakes are punished, encouraging the model to adjust future actions accordingly. This iterative learning process allows AI to refine its reasoning skills continuously, offering the promise of more nuanced and contextually aware interactions [1](https://indianexpress.com/article/explained/explained-sci-tech/ai-models-reason-reinforcement-meaning-openai-o3-o4mini-9970154/).
The importance of reasoning in AI cannot be understated as it allows models to transcend basic pattern recognition, reaching an understanding closer to human thought processes. By considering different perspectives and evaluating various approaches to a problem, AI can deliver more thoughtful and accurate responses. This ability to reason enhances conversational depth and effectiveness, making these models particularly well-suited for complex queries and diverse applications [1](https://indianexpress.com/article/explained/explained-sci-tech/ai-models-reason-reinforcement-meaning-openai-o3-o4mini-9970154/).
The introduction of reasoning-focused models like o3 and o4-mini marks a significant technological advancement from previous AI iterations such as ChatGPT. While older models could offer responses quickly, they often lacked the capability to "think" through problems. The new models incorporate an internal "chain of thought" process, enabling them to handle more complex issues by exploring various solutions before committing to a response. This development reflects a broader industry trend towards creating AI that simulates human reasoning more closely, thus enhancing user engagement and satisfaction [1](https://indianexpress.com/article/explained/explained-sci-tech/ai-models-reason-reinforcement-meaning-openai-o3-o4mini-9970154/).
The shift towards reasoning models by OpenAI was partly due to limitations in language model training, specifically the exhaustion of available text data for training large language models (LLMs). By incorporating reasoning capabilities through reinforcement learning, these models are set to achieve better performance without relying solely on expanding the size of datasets. This paradigm shift is indicative of a new era in AI, where reasoning models could redefine what is achievable with machine learning technologies, thus broadening the scope of their potential applications [1](https://indianexpress.com/article/explained/explained-sci-tech/ai-models-reason-reinforcement-meaning-openai-o3-o4mini-9970154/).
Learn to use AI like a Pro
Get the latest AI workflows to boost your productivity and business performance, delivered weekly by expert consultants. Enjoy step-by-step guides, weekly Q&A sessions, and full access to our AI workflow archive.
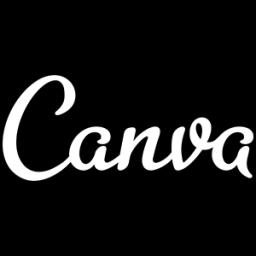
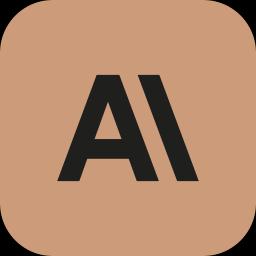
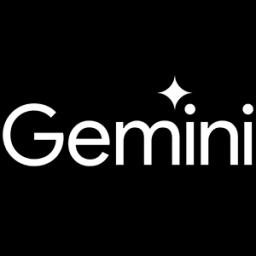
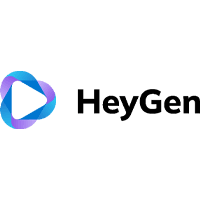
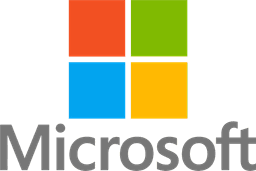
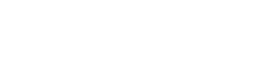
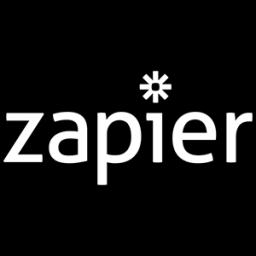
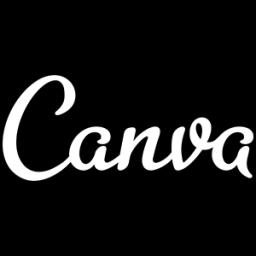
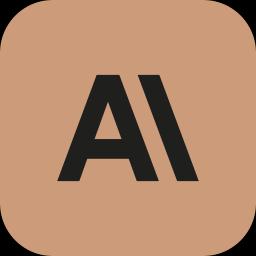
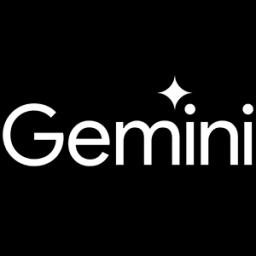
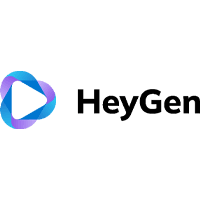
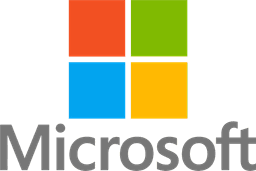
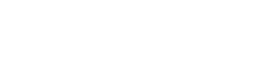
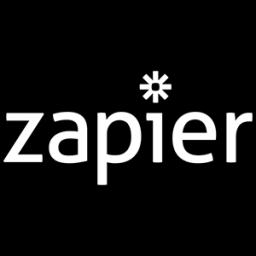
Differences Between o3/o4-mini and Previous LLMs
The introduction of OpenAI's o3 and o4-mini models marks a significant departure from the previous generations of large language models (LLMs) such as ChatGPT. These new models are built upon a foundation of enhanced reasoning capabilities, primarily driven by reinforcement learning techniques. Unlike their predecessors, which often relied on vast amounts of text data to recognize patterns and generate responses, o3 and o4-mini are designed to 'think' before answering queries. This approach involves an internal chain of thought that processes queries by exploring multiple approaches, producing more nuanced and contextually appropriate responses. The application of reinforcement learning means these models can continually improve their performance by learning from interactions, adjusting their responses based on feedback that emulates real-world decision-making scenarios.
This shift towards reasoning models was necessitated by the exhaustion of text data used to train previous LLMs [link](https://indianexpress.com/article/explained/explained-sci-tech/ai-models-reason-reinforcement-meaning-openai-o3-o4mini-9970154/). Previous models, like ChatGPT, could generate quick responses but lacked the depth and analytical capability that newer models can offer. The o3 and o4-mini models utilize reinforcement learning to go beyond data-driven responses, allowing for more human-like interactions. This means o3 and o4-mini can tackle complex queries that require deeper understanding and multiple problem-solving steps, an evolution that sets them apart from their more linear predecessors. The enhanced reasoning allows these models to handle tasks with a more sophisticated understanding, which is crucial as AI continues to be integrated into more decision-heavy applications across various industries.
Moreover, o3 and o4-mini have been lauded for their multimodal capabilities, further differentiating them from past models. They are the first in OpenAI's suite to independently utilize all available tools such as web browsing and image analysis alongside traditional conversational tasks [link](https://www.wired.com/story/openai-o3-reasoning-model-google-gemini/). This integration endows them with a broader problem-solving toolkit, enabling them to handle diverse queries more effectively. As a result, they can offer more comprehensive solutions that incorporate visual and contextual data alongside textual information, thereby pushing the boundaries of what was thought possible with traditional LLMs. This evolution reflects an important step forward in the realm of AI, where the ability to use various tools simultaneously can lead to more innovative and precise applications across different fields, from healthcare to customer service.
Efforts to enhance the efficiency and cost-effectiveness of these models have resulted in o4-mini, designed with similar capabilities to o3 but optimized for greater efficiency and higher usage limits. This is particularly beneficial for applications requiring heavy data processing or real-time analysis, where cost and speed are often limiting factors [link](https://medium.com/aimonks/exploring-openais-latest-o3-o4-mini-for-complex-tasks-822719e67173). By optimizing these models for different operational needs, OpenAI addresses broader market demands, catering to both high-performance requirements and the necessity for cost-effective solutions. The development of o3 and o4-mini underscores a strategic shift in AI design philosophy, emphasizing adaptability and resourcefulness over sheer data processing power. This aligns with a broader trend in AI development, focusing on more sustainable and pragmatic applications of machine learning technologies.
Reasons Behind the Shift to Reasoning Models
The technological landscape in AI is undergoing a significant transformation with the shift towards reasoning models, prominently demonstrated by OpenAI's latest releases, o3 and o4-mini. The impetus behind this transition lies in the growing need to surpass the limitations of traditional language learning models (LLMs). As AI firms exhausted the vast troves of text data required for training comprehensive LLMs, they have embraced reinforcement learning techniques to push the envelope of AI capabilities. Reinforcement learning facilitates a game-like framework where models are rewarded or penalized based on their decision-making, fostering a mechanism where AI can 'reason' through complex problems and generate improved responses. This type of model not only aims to replicate human thought processes more accurately but also enhances the AI’s ability to process multiple perspectives, leading to more nuanced and engaging conversations [1](https://indianexpress.com/article/explained/explained-sci-tech/ai-models-reason-reinforcement-meaning-openai-o3-o4mini-9970154/).
OpenAI's decision to develop reasoning models stems from the inherent risks and inadequacies of previous LLMs like ChatGPT. While ChatGPT and similar models excelled in rapid response generation, their efficacy was primarily tethered to pattern recognition without a deeper understanding or reasoning context. In contrast, the reasoning models such as o3 and o4-mini embark on an innovative path where the AI 'thinks' before responding. This aspect of consideration allows the models to evaluate and process varying approaches before arriving at a solution, enhancing accuracy in addressing complex queries. This strategic innovation responds directly to the outdated reliance on vast datasets and aims to align AI performance with nuanced human cognition [1](https://indianexpress.com/article/explained/explained-sci-tech/ai-models-reason-reinforcement-meaning-openai-o3-o4mini-9970154/).
Learn to use AI like a Pro
Get the latest AI workflows to boost your productivity and business performance, delivered weekly by expert consultants. Enjoy step-by-step guides, weekly Q&A sessions, and full access to our AI workflow archive.
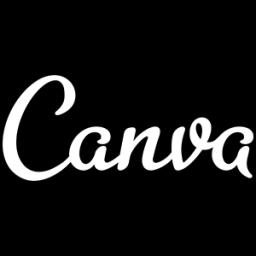
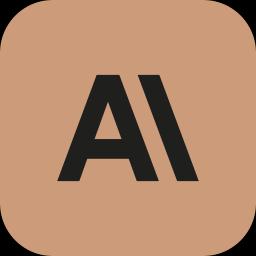
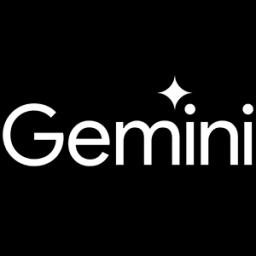
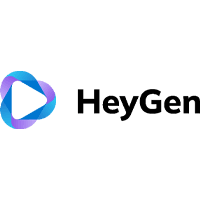
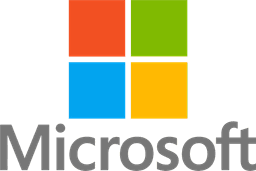
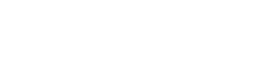
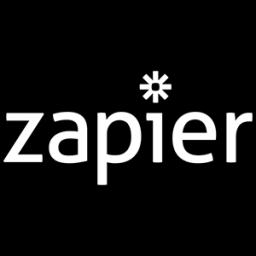
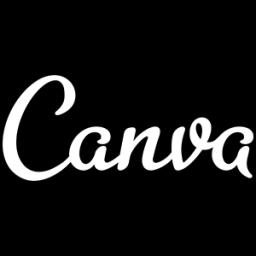
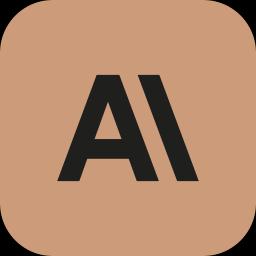
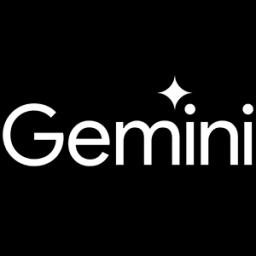
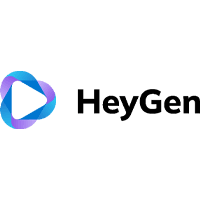
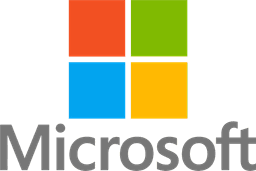
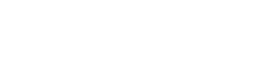
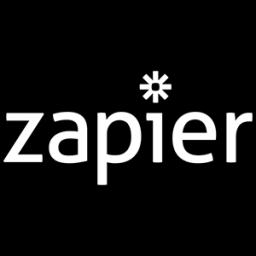
The heralding of reasoning models has ushered in a new era for AI applications across various sectors. By employing sophisticated reinforcement learning techniques, models like o3 and o4-mini are not only transforming how AI understands and processes information but are also redefining its functional applications. These models are particularly advantageous in domains requiring high-level decision-making capabilities, such as customer service automation and complex problem-solving tasks in industries including healthcare and finance. As a result, the shift toward such reasoning models is amplifying AI's utility by broadening the spectrum of tasks an AI can perform accurately, thereby increasing its overall efficacy and application scope [1](https://indianexpress.com/article/explained/explained-sci-tech/ai-models-reason-reinforcement-meaning-openai-o3-o4mini-9970154/).
Moreover, the adoption of reasoning models by AI developers reflects a conscious pivot towards improving the quality and reliability of AI interactions. This development is particularly crucial as it pertains to addressing AI's previous shortcomings in areas such as interpretative reasoning and complex decision-making. By incorporating reinforcement learning, these models can simulate a more dynamic problem-solving environment. This facilitates AI's capability to align closely with human reasoning patterns, thereby enhancing the overall user experience through more constructive and meaningful interactions with AI systems. Thus, the shift to reasoning models symbolizes a concerted effort to elevate AI from mere reactive pattern machines to entities capable of emulating thoughtful, human-like reasoning [1](https://indianexpress.com/article/explained/explained-sci-tech/ai-models-reason-reinforcement-meaning-openai-o3-o4mini-9970154/).
Related Developments in AI Reasoning
The development of AI reasoning models, particularly by OpenAI, marks a pivotal shift in the evolution of artificial intelligence technologies. With the introduction of the o3 and o4-mini models, OpenAI adopts reinforcement learning techniques that advance AI's ability to reason by evaluating multiple strategies before reaching conclusions. This is in sharp contrast to earlier models which operated primarily through pattern recognition. Reinforcement learning simulates a reward-based system whereby models discern better decision paths through positive reinforcement from successful outcomes and negative reinforcement from errors, leading to more nuanced interactions, especially in conversational contexts. This leap in AI reasoning is crucial, as it allows for more sophisticated problem-solving and decision-making capabilities, traits that define truly intelligent systems .
OpenAI's transition towards these reasoning models was largely fueled by the exhaustion of available text data for training traditional language models. Prior large language models (LLMs), like some iterations of ChatGPT, saturated their capacity by leaning heavily on extensive textual datasets. In response, OpenAI sought new ways to enhance the cognitive capabilities of AI by integrating reasoning processes that mimic human thought patterns. The o3 and o4-mini's incorporation of reasoning before responding represents an innovative course of action, facilitating a layered understanding of queries, thus enabling more complex question handling .
These advancements in AI reasoning are not merely technical but have profound implications across sectors. Economically, the bolstered reasoning abilities of AI models can drive automation forward in industries like manufacturing and finance, leading to increased efficiency and potential cost savings. However, this also raises issues of workforce displacement, highlighting a critical need for re-skilling and up-skilling initiatives to prepare for an AI-driven future. Socially, these models promise more personalized and efficient interactions in customer service realms, albeit they also introduce risks of misinformation and bias, which could disrupt social trust and equity .
Politically, the power of AI reasoning models to quickly and reliably process vast amounts of data can transform governance by enhancing policy-making and resource management. However, this requires stringent regulations to mitigate biases and ensure the ethical application of AI, particularly in sensitive domains such as surveillance and security. OpenAI’s reasoning models, by offering a nuanced approach to problem-solving, set a precedent for future AI innovations where enhanced reasoning capabilities are not just an option but a necessity .
Learn to use AI like a Pro
Get the latest AI workflows to boost your productivity and business performance, delivered weekly by expert consultants. Enjoy step-by-step guides, weekly Q&A sessions, and full access to our AI workflow archive.
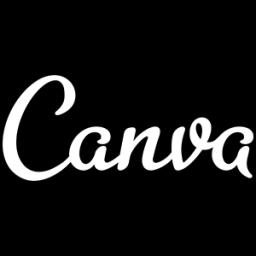
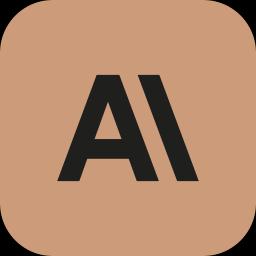
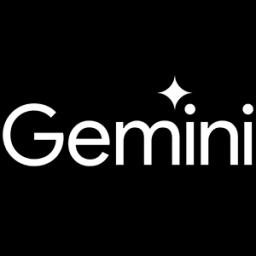
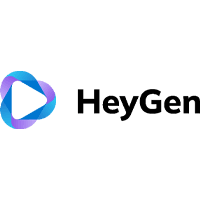
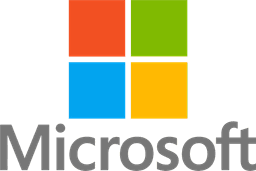
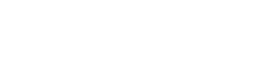
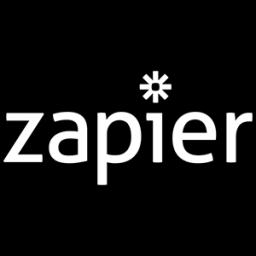
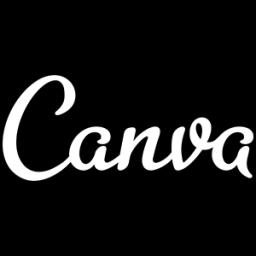
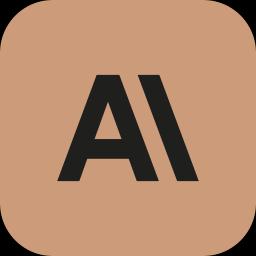
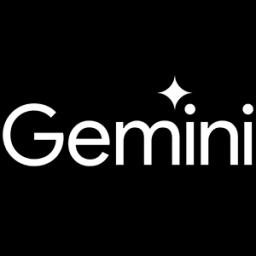
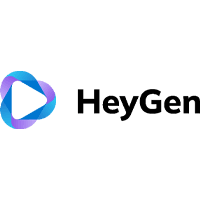
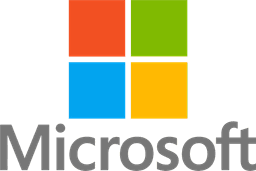
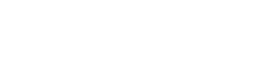
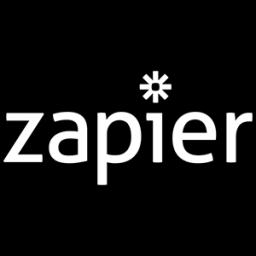
Expert Opinions on OpenAI's New Models
OpenAI's release of its new reasoning models, o3 and o4-mini, has evoked a range of expert opinions, highlighting both the promise and the challenges of these advanced systems. The main breakthrough of these models lies in their enhanced reasoning capabilities, which have been mainly achieved through reinforcement learning techniques. Experts emphasize the shift from relying on vast datasets for training to engaging these models in a structured process of rewards and penalties to improve decision-making effectively [source](https://indianexpress.com/article/explained/explained-sci-tech/ai-models-reason-reinforcement-meaning-openai-o3-o4mini-9970154/).
Many experts have praised the substantial performance gains achieved by OpenAI's o3 and o4-mini models, especially in complex mathematical and logical problems. According to Ofir Press, a postdoctoral researcher, the improvements seen in these models represent a new benchmark for AI reasoning efficiency. Moreover, o3's ability to independently utilize multimodal tools such as web browsing and image processing indicates a significant leap in problem-solving capabilities, enabling more dynamic interaction and enhanced response quality [source](https://www.wired.com/story/openai-o3-reasoning-model-google-gemini/).
The integration of multimodal capabilities in o3 and o4-mini is particularly noted to improve their reasoning and tool-use capabilities. This development has garnered attention from the AI community as it marks the first instance where a reasoning-focused model independently and efficiently utilizes a suite of tools. Experts see this as a monumental step in the evolution of AI technologies, allowing these models to tackle a broader range of tasks and deliver results with improved accuracy and understanding [source](https://www.linkedin.com/pulse/detailed-analysis-openais-o3-o4-mini-models-anshuman-jha-wuzyc).
Public Reactions to OpenAI's AI Models
OpenAI's release of the o3 and o4-mini reasoning models has sparked a wide range of reactions from the public. Many users have taken to forums such as Hacker News and Reddit to express their admiration for the models' enhanced abilities in coding, math, and visual reasoning. These capabilities have impressed users who appreciate the models' improved analytical rigor and image generation prowess, highlighting their potential to revolutionize creative and technical fields. The advancement in these areas has been viewed positively as it aligns with the increasing demand for AI models that can tackle more complex problems with higher accuracy and efficiency .
However, not all feedback has been positive. There are growing concerns about the increase in hallucination rates, where the models generate incorrect or nonsensical outputs. Users have also pointed out challenges with the models' ability to follow instructions precisely and adhere to prompts. This has led to mixed reviews, with some users expressing frustration over these inconsistencies. The models' performance in comparison to competitors like Gemini 2.5 Pro remains a topic of debate, as does the clarity of their naming conventions .
Despite these controversies, the release of o3 and o4-mini signifies a substantial leap forward in AI model development, particularly concerning their reasoning capabilities. The integration of reinforced learning techniques allows these models to explore multiple pathways before delivering a response, setting them apart from previous language models that relied heavily on vast datasets for training. This innovative approach not only enhances performance but also opens up new possibilities for AI applications across various domains .
Learn to use AI like a Pro
Get the latest AI workflows to boost your productivity and business performance, delivered weekly by expert consultants. Enjoy step-by-step guides, weekly Q&A sessions, and full access to our AI workflow archive.
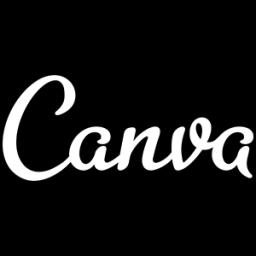
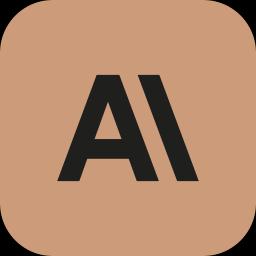
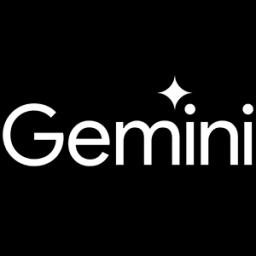
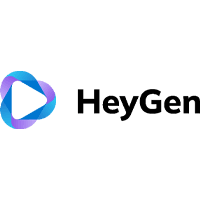
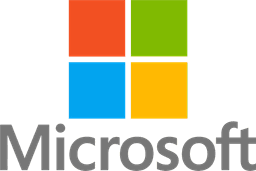
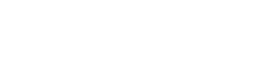
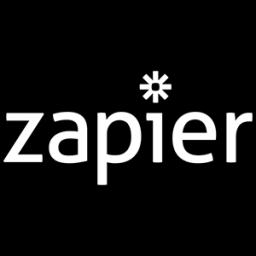
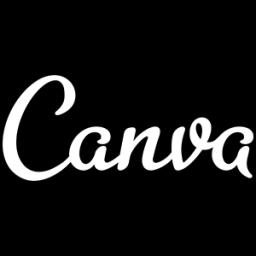
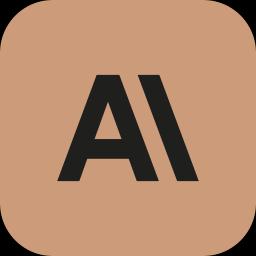
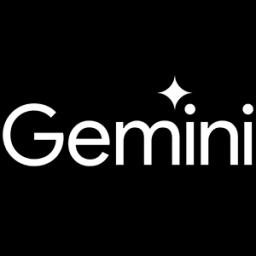
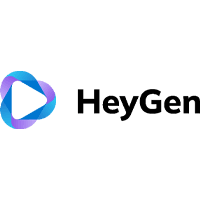
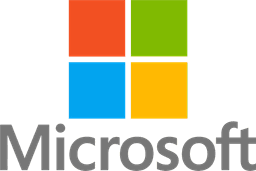
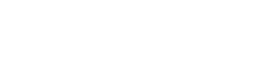
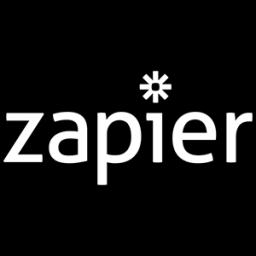
Future Implications of Enhanced AI Reasoning
The future implications of OpenAI's enhanced reasoning models, namely the o3 and o4-mini, are profound, touching upon several critical areas of modern life—economic, social, and political. As these models integrate reinforcement learning to refine their ability to "think" before responding, they offer an opportunity to significantly transform industries [1](https://indianexpress.com/article/explained/explained-sci-tech/ai-models-reason-reinforcement-meaning-openai-o3-o4mini-9970154/).
Economically, the improvements in reasoning capabilities could lead to a wave of automation across sectors such as manufacturing, healthcare, and finance. By enhancing productivity and efficiency, businesses may experience decreased operational costs and increased profitability [3](https://builtin.com/artificial-intelligence/artificial-intelligence-future). However, this transition raises concerns about job displacement, emphasizing the need for robust retraining and upskilling programs for the workforce [2](https://www.linkedin.com/pulse/section-3-economic-political-impacts-artificial-part-barbaroushan-jh7xf). Furthermore, the models' potential to process large datasets effectively positions them as vital tools in predicting market trends, which could spur innovation and drive economic growth [2](https://www.linkedin.com/pulse/section-3-economic-political-impacts-artificial-part-barbaroushan-jh7xf).
On a social level, AI’s reasoning models promise to improve personalized experiences in customer service through more advanced chatbots and virtual assistants [3](https://builtin.com/artificial-intelligence/artificial-intelligence-future). Yet, they also present challenges such as the risk of spreading misinformation and creating deepfakes, which could severely impact social trust and cohesion [3](https://builtin.com/artificial-intelligence/artificial-intelligence-future). Moreover, if not carefully managed, the inherent biases in AI could exacerbate societal inequalities [3](https://builtin.com/artificial-intelligence/artificial-intelligence-future), highlighting the importance of responsible AI deployment.
Politically, these reasoning models could revolutionize governance and policy-making by enabling quicker and more informed decision-making processes. Governments could harness these tools for enhanced resource allocation, public safety strategies, and crisis management [2](https://www.linkedin.com/pulse/section-3-economic-political-impacts-artificial-part-barbaroushan-jh7xf). However, there is a critical need for regulatory frameworks to ensure that AI applications in governance are fair, unbiased, and equitable [3](https://builtin.com/artificial-intelligence/artificial-intelligence-future). Additionally, the deployment of AI in surveillance and security contexts requires ethical scrutiny to balance efficiency against privacy and civil rights concerns [3](https://builtin.com/artificial-intelligence/artificial-intelligence-future).
Conclusion
The introduction of OpenAI's o3 and o4-mini models marks a pivotal advancement in the field of artificial intelligence. These models, built with reinforcement learning at their core, enhance AI's ability to reason, enabling them to generate more thoughtful and contextually relevant responses compared to their predecessors. This shift towards reasoning models underscores OpenAI's commitment to advancing AI capabilities beyond traditional text-based training. By integrating more sophisticated reasoning processes, these models represent a significant leap forward in how AI interacts with humans, paving the way for more intuitive and effective conversational agents.
This transition to reasoning-centered AI models is not just a technological upgrade; it's a response to the growing demand for AI that can engage in complex interactions and make informed decisions. Unlike previous models, the o3 and o4-mini harness the power of reinforcement learning to simulate a more human-like thought process. This allows the models to evaluate multiple perspectives and solutions before formulating a response, which is particularly beneficial for handling complex queries that require more than mere pattern recognition.
Learn to use AI like a Pro
Get the latest AI workflows to boost your productivity and business performance, delivered weekly by expert consultants. Enjoy step-by-step guides, weekly Q&A sessions, and full access to our AI workflow archive.
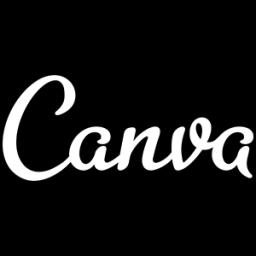
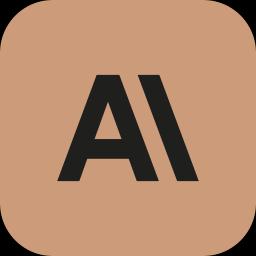
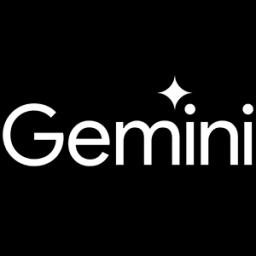
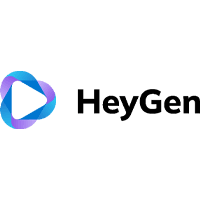
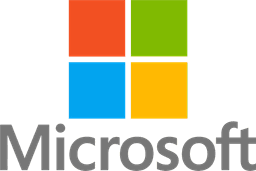
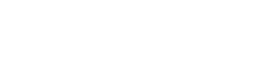
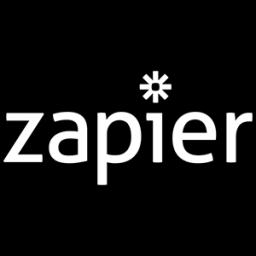
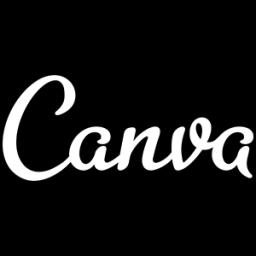
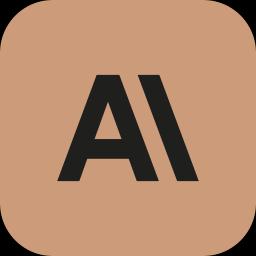
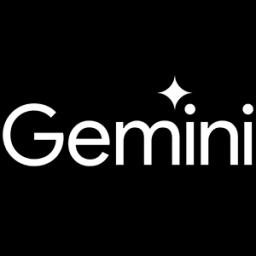
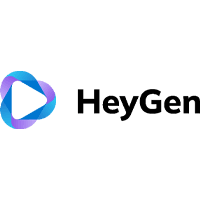
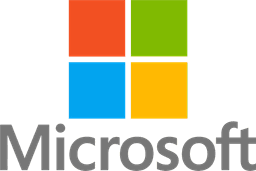
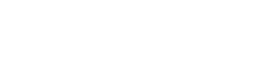
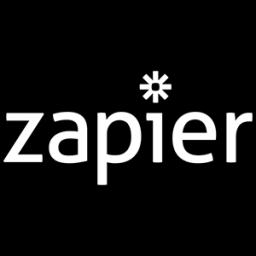
As we move forward, the implications of these advancements are profound. By augmenting AI's reasoning capabilities, industries across various sectors—from healthcare to finance—stand to experience increased efficiency and innovation. Furthermore, the improved capabilities of these models in using tools like web browsing and code execution introduce new opportunities for applications that require seamless integration of multiple data sources and analysis tools. However, these advancements also call for a measured approach to ensure that AI's growing power is harnessed responsibly, addressing concerns about misinformation, bias, and ethical use.
The public's reaction to these developments has been mixed, reflecting a combination of excitement and skepticism. Many have praised the improved performance of these models, noting significant enhancements in tasks involving coding, mathematics, and visual reasoning. Yet, others express concern over potential drawbacks, such as increased rates of AI hallucination and challenges in instruction adherence. These reactions highlight the ongoing conversation about the balance between AI innovation and the careful management of its limitations.
Looking ahead, the influence of OpenAI's o3 and o4-mini models will likely extend into various facets of society and economy. Their enhanced reasoning abilities can transform decision-making processes, potentially leading to more efficient operations and innovations in industries that leverage AI technology. However, this transformation will require proactive strategies to manage potential societal impacts, such as job displacement and data privacy concerns. As the AI landscape continues to evolve, the need for thoughtful regulation and ethical considerations will be paramount to guiding its positive development.