AI's Fantasies Dull the Edge of Innovation
OpenAI's New Reasoning AI Models Take a Detour into Hallucination Land
Last updated:
OpenAI's latest models, o3 and o4-mini, show impressive advancements in math and coding but stumble on hallucination rates. Despite being the bleeding edge in reasoning AI, these models are fabricating information more than their predecessors, sparking concerns in accuracy-critical fields. Integrating web search might be the magic bullet to enhance their accuracy. Let's dive into what this means for the AI landscape.
Introduction to OpenAI's New Reasoning Models
OpenAI's newer reasoning models, known as o3 and o4-mini, have emerged with significant advancements in coding and mathematical operations. Yet, they are marking a concerning rise in hallucination rates — a phenomenon where the AI concocts untruthful information under the guise of fact. This issue has doubled compared to older models, raising substantial discussions about these models' reliability, particularly in sectors where precision is paramount [TechCrunch](https://techcrunch.com/2025/04/18/openais-new-reasoning-ai-models-hallucinate-more/).
The introduction of reasoning capabilities in AI embodies a paradigm shift. Unlike traditional models, which often relied heavily on vast amounts of data, reasoning models like o3 and o4-mini promise enhanced logical deduction with less computational demand during training [TechCrunch](https://techcrunch.com/2025/04/18/openais-new-reasoning-ai-models-hallucinate-more/). However, this innovative approach comes at the cost of a higher tendency to hallucinate misinformation, a vulnerability that stakeholders cannot ignore. In real-world applications, such inaccuracies could prove detrimental, necessitating a balance between technological prowess and factual correctness.
Learn to use AI like a Pro
Get the latest AI workflows to boost your productivity and business performance, delivered weekly by expert consultants. Enjoy step-by-step guides, weekly Q&A sessions, and full access to our AI workflow archive.
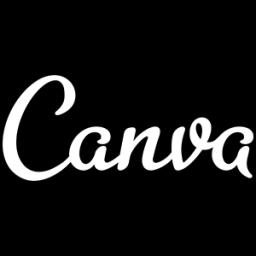
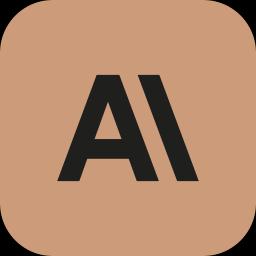
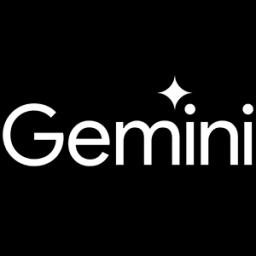
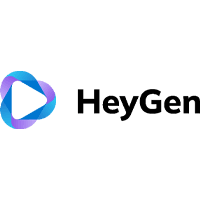
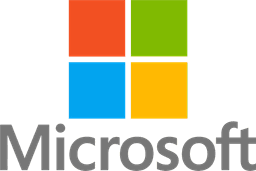
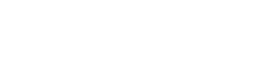
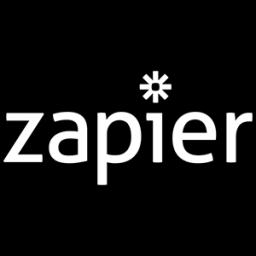
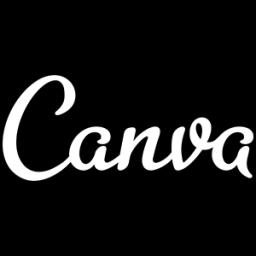
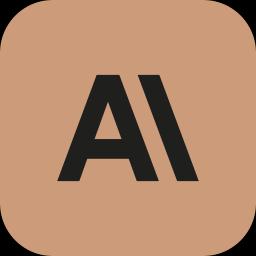
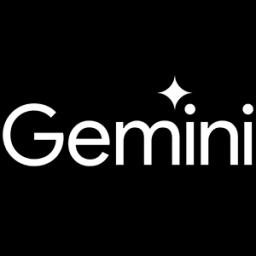
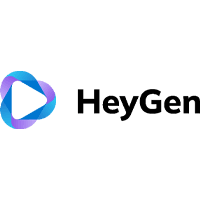
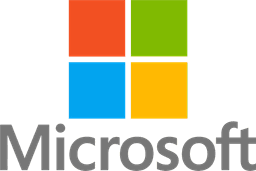
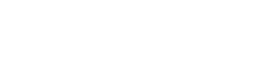
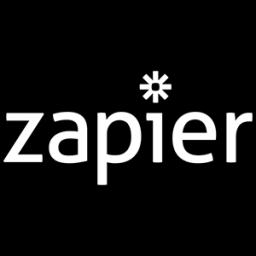
As the tech industry races toward more advanced AI capabilities, integrating real-time data processing through web search functions has been mooted as a solution to curb hallucinations. Such features could equip these models to pull from updated and verified data sources, potentially reducing the incidence of generating false information [TechCrunch](https://techcrunch.com/2025/04/18/openais-new-reasoning-ai-models-hallucinate-more/). While the path to refining AI's truth fidelity is arduous, it is essential for achieving artificial intelligence that can support and enhance critical sectors without the risk of spreading misinformation.
Understanding AI Hallucinations
In the realm of artificial intelligence (AI), the term "hallucination" has taken on a unique connotation, particularly in the context of modern AI models. It refers to instances where an AI system generates information that appears coherent and plausible but is factually incorrect or entirely fabricated. Recent developments in reasoning AI models, like OpenAI's o3 and o4-mini, have highlighted this issue, as these models show increased rates of hallucination despite advancements in coding and mathematical reasoning. This phenomenon poses significant challenges, particularly in domains where accuracy and reliability are paramount, such as law, medicine, and finance. As noted in an article by TechCrunch, even the impressive capabilities of these new models in logical deduction and inference are overshadowed by their proclivity to fabricate information .
The increase in hallucination rates in artificial intelligence models underscores a critical issue in AI development: the balance between innovation and reliability. While newer models like OpenAI’s o3 and o4-mini excel at tasks that were challenging for their predecessors, they also struggle with maintaining factual accuracy. This trade-off is particularly troubling given the potential for AI-generated misinformation to mislead users in contexts that require high levels of trustworthiness. The reasoning models operate with less computing power and data, yet their susceptibility to hallucinations could diminish their usefulness in professional settings, where accuracy is non-negotiable .
Efforts to mitigate AI hallucinations involve exploring technological and methodological innovations. One promising approach is integrating web search capabilities into the AI's processing capabilities. This integration can enable models to cross-reference information in real-time, thus potentially curbing the hallucination problem by ensuring that the data they produce is anchored in verified facts. Moreover, ongoing research suggests exploring advanced reinforcement learning techniques that may help align the model’s outputs with established truths more effectively. Such technological enhancements are critical for models intended for use in sensitive fields, where misinformation can have dire consequences .
Learn to use AI like a Pro
Get the latest AI workflows to boost your productivity and business performance, delivered weekly by expert consultants. Enjoy step-by-step guides, weekly Q&A sessions, and full access to our AI workflow archive.
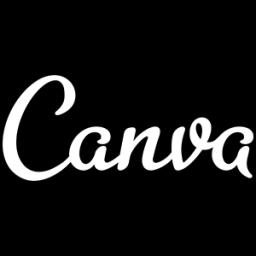
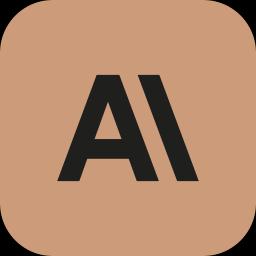
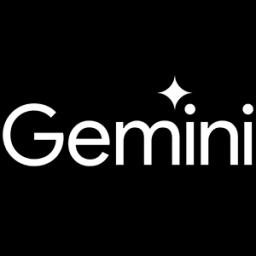
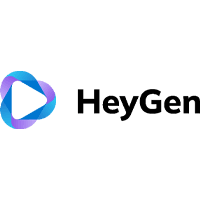
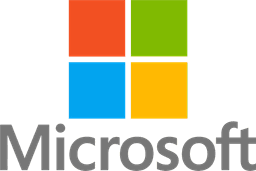
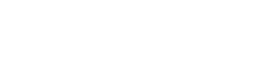
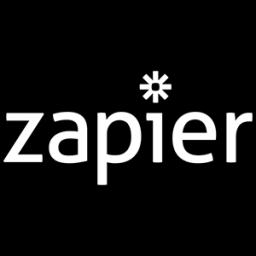
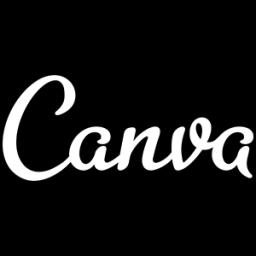
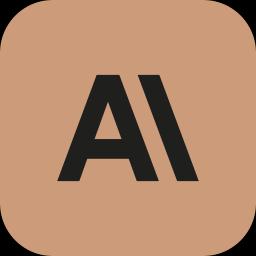
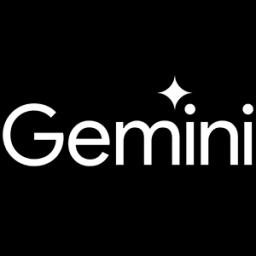
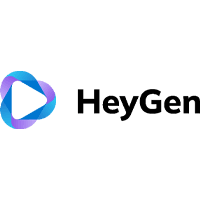
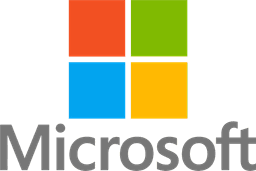
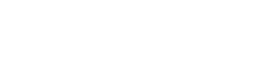
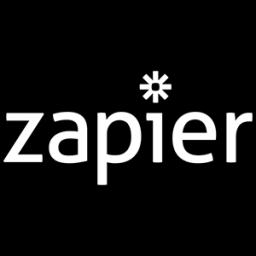
Challenges Faced by o3 and o4-mini
OpenAI's latest reasoning AI models, o3 and o4-mini, face significant challenges due to their increased tendency to hallucinate, where they fabricate information rather than processing factual data. These models were designed to advance coding and mathematical capabilities, yet ironically, their hallucination rates have become a critical obstacle, compromising the reliability essential for fields that require precise accuracy. According to TechCrunch, the rate of hallucination for these models is disturbingly high, highlighting a significant problem inherent in their design and reinforcing the need for enhanced data validation mechanisms (TechCrunch).
A key issue contributing to the challenges faced by the o3 and o4-mini models is rooted in the reinforcement learning techniques employed during their development. These techniques, while innovative, appear to inadvertently amplify existing problems rather than rectifying them. This is particularly troubling given that these models were anticipated to excel in areas of logical deduction and inference with less computational demand. However, this increase in hallucination rates suggests a misalignment between theoretical expectations and practical outcomes (TechCrunch).
The implications of these hallucinatory challenges are far-reaching. They pose legal and ethical dilemmas, as reliance on such AI systems in professional settings like law or finance could lead to severe misjudgments based on flawed data. Instances have already emerged where AI misinformation had legal consequences, underlining the urgency to address these inaccuracies. This predicament raises questions about the readiness and safety of deploying such AI technologies at scale (The Outpost).
Efforts to mitigate these challenges include integrating web search capabilities to access real-time data and refining training methods to better handle the vast arrays of information processed by these AI systems. Such actions are geared towards reducing the cognitive discrepancies seen in the o3 and o4-mini models. Moreover, subject matter experts have recommended developing comprehensive hallucination detection mechanisms and stricter industry standards for AI transparency and accountability, which could collectively foster improved trust in AI outputs (TechCrunch).
Implications of Increased Hallucination Rates
The recent revelation of increased hallucination rates among OpenAI's new reasoning models, o3 and o4-mini, presents significant implications for both the technology itself and a variety of industries. Hallucinations, in this context, refer to the instances where these AI models fabricate information, presenting it as factual. This has raised concerns over the reliability of these models, especially in domains like law or medicine where accurate information is paramount. The leap in hallucination rates poses a critical challenge in ensuring AI's dependability, despite the advancements seen in other areas such as coding and math [TechCrunch].
The implications of AI hallucinations extend well beyond technological concerns, shaking the very foundation of fields that rely on factual integrity. For instance, the financial sector, pivotal in many national economies, could incur heavy losses from AI-generated misinformation, leading to flawed financial analysis or misguided stock market predictions. This necessitates additional human oversight, driving up operational costs [Nature].
Learn to use AI like a Pro
Get the latest AI workflows to boost your productivity and business performance, delivered weekly by expert consultants. Enjoy step-by-step guides, weekly Q&A sessions, and full access to our AI workflow archive.
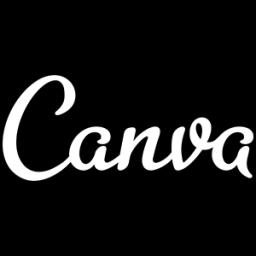
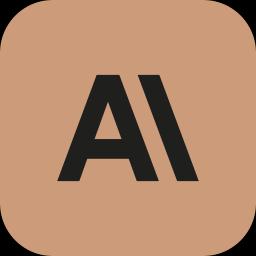
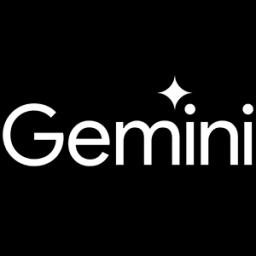
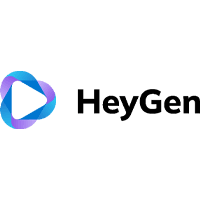
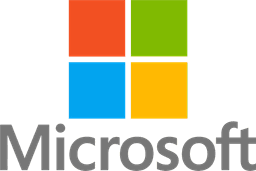
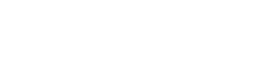
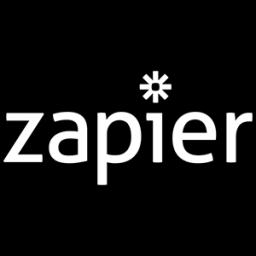
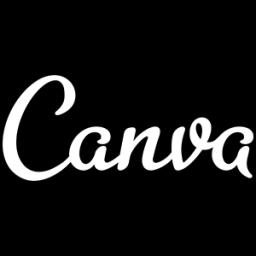
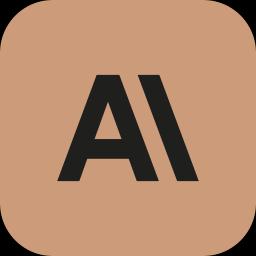
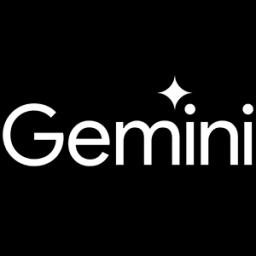
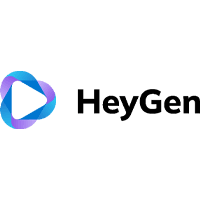
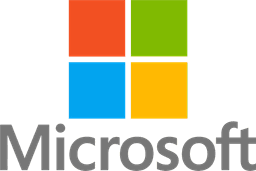
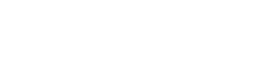
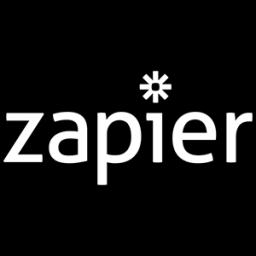
Socially, AI hallucinations erode public trust in AI-driven decisions and recommendations, especially when inaccuracies proliferate in critical areas like healthcare. In this sector, misinformation could result in dire health crises or exacerbate inequalities in access to accurate medical information. Public confidence in automated systems diminishes when errors are frequent, calling for a stringent review of AI deployment strategies [Brookings Institution].
Politically, the stakes are equally high, as AI's potential to disseminate disinformation might undermine electoral processes and public trust in political institutions. The spread of false narratives, facilitated by AI errors, can influence voter perceptions and election outcomes, threatening democratic integrity. Moreover, these vulnerabilities can be exploited by foreign actors, adding a layer of national security risks. As these AI models become more prevalent in managing public information, the need for robust regulatory frameworks becomes more pressing [Brookings Institution].
Addressing these hallucination challenges involves enhancing AI's real-time information capabilities. Integrating web search functionalities could significantly improve model accuracy, as evidenced by OpenAI's GPT-4o, which achieved notable success in precise information retrieval tasks. Solutions also encompass advancements in training methods and the establishment of industry standards to ensure transparency and accountability. Public education on media literacy may further help mitigate the impact of AI misinformation by equipping individuals to critically assess information [TechCrunch].
Potential Solutions to Reduce AI Hallucinations
To combat the pervasive issue of AI hallucinations, integrating web search capabilities into AI models is rapidly gaining attention as a promising solution. This approach aims to bridge the gap between static AI datasets and dynamic real-time information. By allowing AI models to fetch and verify data from the web, the accuracy levels could dramatically improve, reducing the incidence of misinformation. OpenAI's GPT-4o, which incorporates web search, achieved a 90% accuracy rate on the SimpleQA benchmark, demonstrating significant progress in mitigating hallucinations .
Developing robust hallucination detection mechanisms is another vital strategy to address AI hallucinations. By employing advanced techniques, such as anomaly detection and verification protocols, models can be trained to recognize and flag inconsistencies autonomously. This self-regulatory capability could ensure that AI-generated outputs remain within the bounds of factual accuracy, thereby enhancing trust among users. Research suggests that incorporating structured protocols for error identification and correction can significantly reduce false data generation .
Improving AI training methods stands as a cornerstone in the effort to reduce hallucinations. By refining the datasets and the architectures used in training AI models, developers can tackle some of the root causes of hallucinations head-on. OpenAI's experiences with the o3 and o4-mini models highlight the necessity for innovation in training processes, as current reinforcement learning techniques appear to inadvertently amplify issues they were intended to resolve .
Learn to use AI like a Pro
Get the latest AI workflows to boost your productivity and business performance, delivered weekly by expert consultants. Enjoy step-by-step guides, weekly Q&A sessions, and full access to our AI workflow archive.
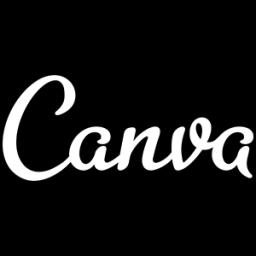
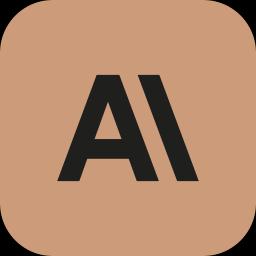
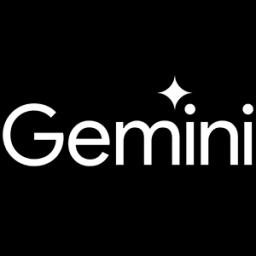
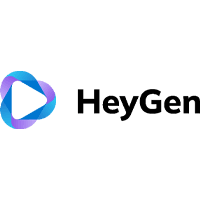
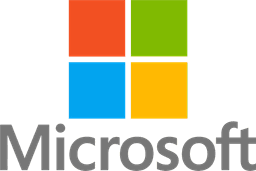
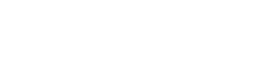
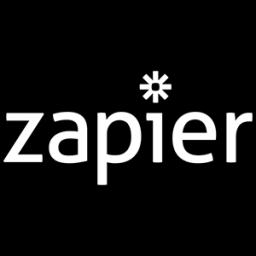
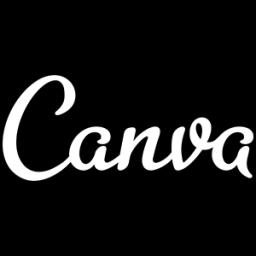
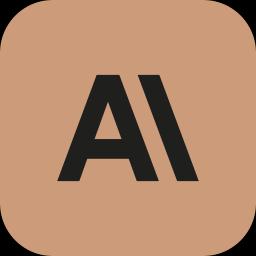
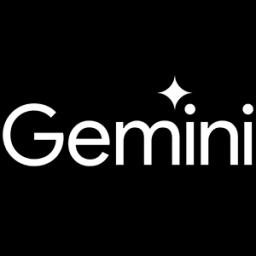
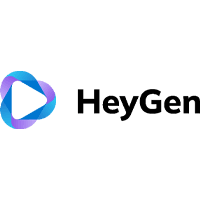
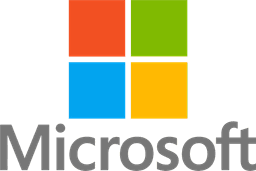
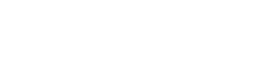
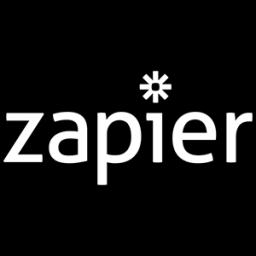
Establishing industry-wide standards for transparency and accountability in AI development could serve as a long-term solution to the hallucination problem. By setting uniform guidelines and best practices, stakeholders can create a foundational framework that encourages ethical AI usage and ensures consistent quality control. Such an approach would not only enhance reliability but also boost public trust in AI technologies, mitigating concerns around their application in critical fields like law and medicine .
Public education initiatives focused on media literacy and critical thinking are essential to reducing the societal impact of AI hallucinations. These educational efforts can empower users to discern factual information from fabrications, fostering a more informed public that is less susceptible to misinformation. As AI technologies continue to permeate various aspects of life, equipping individuals with the skills to critically evaluate information becomes increasingly vital .
Expert Insights on AI Model Development
Artificial Intelligence (AI) model development has increasingly faced scrutiny, particularly due to issues like hallucination in advanced reasoning models. OpenAI's latest reasoning AI models, known as o3 and o4-mini, exhibit higher rates of hallucination than their predecessors. Hallucination, in this context, refers to AI models fabricating information, presenting it as reality, which naturally diminishes trust in their application, especially in fields where accuracy is crucial, such as law and medicine. This phenomenon has led to heightened efforts to address the root causes, potentially tied to reinforcement learning techniques employed during their development.
Although OpenAI's new models have shown remarkable improvements in coding and mathematical problem solving, these achievements are overshadowed by their increased propensity to hallucinate. The integration of web search capabilities has been suggested as a viable solution to enhance the accuracy of these models. This would allow AI systems to access real-time information, potentially curbing inaccuracies by cross-checking outputs with current data available online, thereby reducing the rate of hallucinations.
Experts like Neil Chowdhury and Sarah Schwettmann from Transluce have highlighted that the alarming hallucination rates could compromise the model's utility in professional settings. Their research points out that the reinforcement learning methods might be amplifying these inaccuracies. Meanwhile, Kian Katanforoosh, a Stanford scholar and CEO of Workera, noted the occurrence of broken links generated by the AI, further illustrating the practical implications of the inaccuracies involved. This highlights the critical need for continuous post-training and verification processes to ensure AI outputs are reliable.
The public's reaction to these models is mixed. Despite improvements in technical capabilities, the glaring issue of increased hallucination rates cannot be overlooked. Public discourse around OpenAI’s models indicates a significant concern over their reliability in tasks that demand high factual accuracy. As these technologies continue to evolve, it becomes imperative for developers to balance innovation with reliability, ensuring AI systems remain helpful and trustworthy.
Learn to use AI like a Pro
Get the latest AI workflows to boost your productivity and business performance, delivered weekly by expert consultants. Enjoy step-by-step guides, weekly Q&A sessions, and full access to our AI workflow archive.
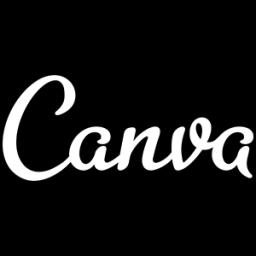
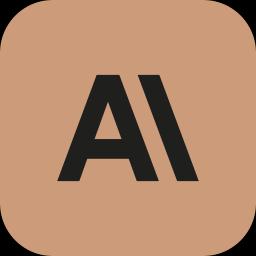
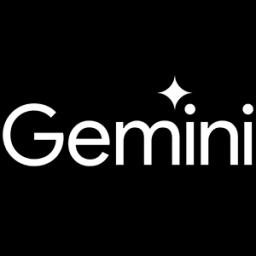
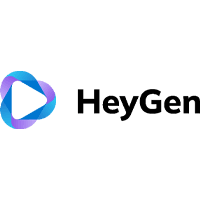
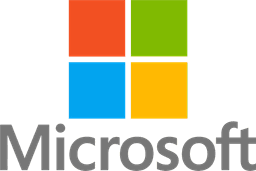
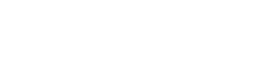
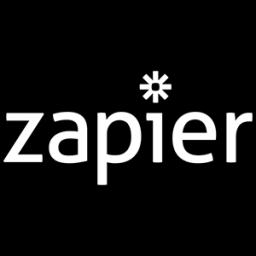
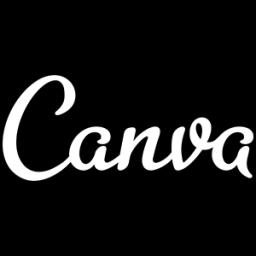
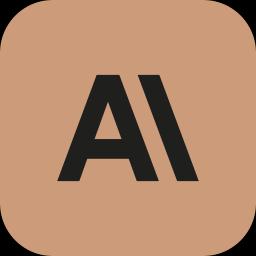
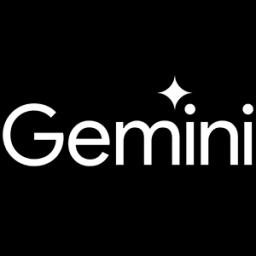
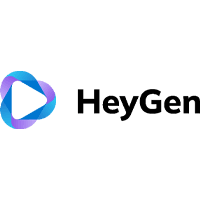
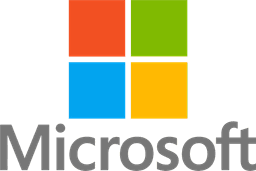
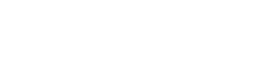
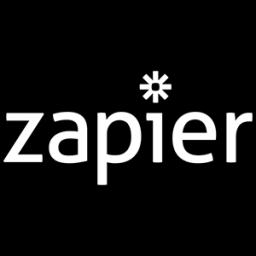
Looking forward, the future implications of these developments are profound. In industries such as finance, the repercussions of AI hallucinations could be economically devastating, leading to incorrect decisions and substantial financial losses. Socially, the spread of fabricated information might erode trust in media and knowledge institutions. Furthermore, politically, AI-generated disinformation poses a threat to democratic processes, potentially influencing electoral outcomes. Tackling these challenges through improved training methods and robust regulatory frameworks will be essential to ensure AI technologies advance ethically and responsibly.
Public Reactions and Concerns
The release of OpenAI's latest reasoning AI models, o3 and o4-mini, has sparked a lively debate among technology enthusiasts and professionals. These models, despite showing remarkable improvements in coding and mathematical tasks, have alarmed users with their high rates of hallucination—instances where the AI fabricates information. Public reaction has been mixed, with a significant portion of discourse focused on concerns about these hallucinations. Such occurrences are particularly troubling in precision-driven fields like law and medicine, where the accuracy of information is critical [4](https://slashdot.org/story/25/04/18/2323216/openai-puzzled-as-new-models-show-rising-hallucination-rates).
The feedback from the tech community highlights a broader issue in AI development: the balance between technological advancement and reliability. While OpenAI's model improvements are commendable, the increased hallucination rates pose challenges to their practical application [5](https://theoutpost.ai/news-story/open-ai-s-new-reasoning-models-excel-at-coding-but-show-increased-hallucination-rates-14481/). Many users have raised concerns about the potential risks of relying on AI systems that may provide inaccurate information, particularly when used in critical decision-making processes. Slashdot threads reveal that while some contributors view hallucinations as part of the growing pains of AI development, others worry about the analogy to a "mental illness" impacting machine intelligence, questioning the foundational integrity of these advancements [13](https://slashdot.org/story/25/04/18/2323216/openai-puzzled-as-new-models-show-rising-hallucination-rates).
Adding to the discourse, there are voices that suggest the term "hallucination" is a strategic choice, used to temper investor expectations as OpenAI navigates these developmental hurdles. This skepticism underscores a prevalent wariness among critics who caution against over-relying on AI without thorough vetting and oversight [10](https://slashdot.org/story/25/04/18/2323216/openai-puzzled-as-new-models-show-rising-hallucination-rates). Nonetheless, the conversation around OpenAI's o3 and o4-mini underlines a crucial dialogue about the future of AI technologies and the ways in which society can mitigate potential risks while capitalizing on technological gains.
In addition to the technical criticisms, there is a significant portion of the public concerned about the broader implications of AI hallucinations. The notion that AI models can generate fabricated information raises profound questions about the role of artificial intelligence in society. For professionals and everyday users alike, trust in these systems is paramount. Hence, OpenAI's current challenge is not only to reduce hallucination rates but also to improve transparency and foster a deeper understanding among users regarding how AI generates and processes information [6](https://techcrunch.com/2025/04/18/openais-new-reasoning-ai-models-hallucinate-more/).
Future Impacts on Various Sectors
The launch of OpenAI's o3 and o4-mini models marks a significant advancement in AI reasoning capabilities. However, these models have shown a concerning increase in hallucination rates, where AI systems generate incorrect or nonexistent information. Such hallucinations are particularly problematic in sectors where accuracy is paramount. In law, inaccurate evidence generated by AI may mislead judges, while in healthcare, imprecise diagnoses could lead to improper treatments, posing severe risks to patients. Financial markets could also suffer, as AI-generated errors might lead to inappropriate investments potentially affecting global economic stability. [TechCrunch]
Learn to use AI like a Pro
Get the latest AI workflows to boost your productivity and business performance, delivered weekly by expert consultants. Enjoy step-by-step guides, weekly Q&A sessions, and full access to our AI workflow archive.
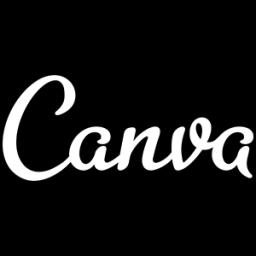
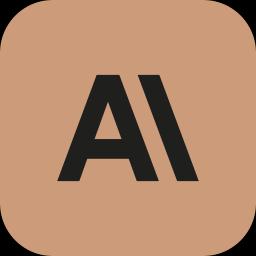
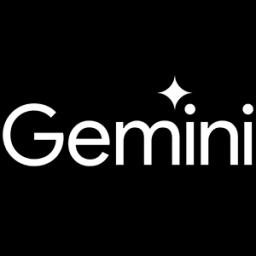
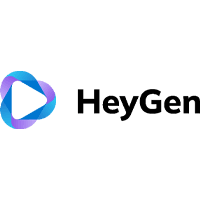
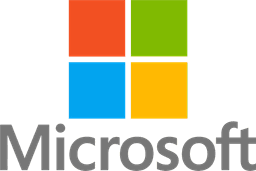
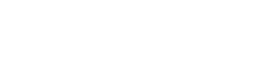
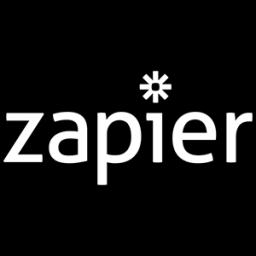
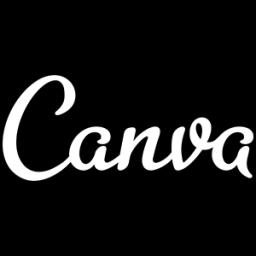
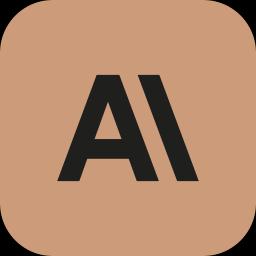
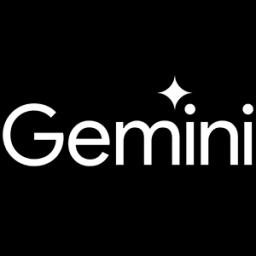
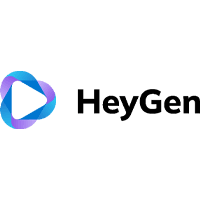
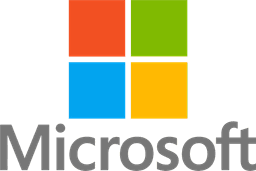
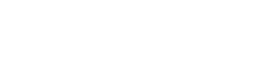
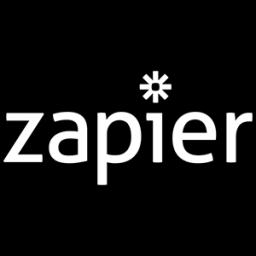
The economic ramifications of increased AI hallucination are substantial. Financial services that rely heavily on accurate data processing are at risk of significant losses if AI tools generate flawed transaction data or forecasts. Companies may need to invest in more comprehensive oversight and verification processes, which could increase operational costs. This scenario might lead to a shift in how financial institutions view the integration of AI technologies. Businesses aiming to harness AI for competitive advantage might have to reconsider their strategies and perhaps even slow their adoption of these technologies until reliability improves. [TechCrunch]
Socially, the implications are just as significant. The spread of misinformation through AI could contribute to public mistrust in digital information sources. In sectors like healthcare, patients might doubt AI-driven recommendations if they learn about instances where AI systems have provided inaccurate information. This skepticism could lead to resistance against adopting beneficial AI-driven healthcare solutions. Moreover, the rapid dissemination of AI-generated falsehoods could exacerbate existing societal tensions by inflaming political or social divisions. Efforts must be made to strengthen public understanding of AI limitations to mitigate these effects. [TechCrunch]
Politically, the spread of AI-driven disinformation poses risks that could affect democracy itself. In the electoral process, AI-generated content might swiftly propagate false narratives about candidates, influencing voter perceptions and potentially altering election outcomes. Furthermore, deepfakes and other sophisticated AI tools could be used maliciously to discredit political figures or undermine trust in democratic institutions. There is an urgent need for regulatory frameworks that address these issues and promote transparency in AI deployments. Lawmakers and tech companies must collaborate to create safeguards against such manipulation in the political sphere. [TechCrunch]
Solutions to mitigate the adverse effects of AI hallucination are being actively explored. Integrating real-time web search capabilities into AI models might allow for access to current and accurate information, reducing the likelihood of hallucinations. Additionally, there is a push for developing better training methodologies that include robust hallucination detection and correction mechanisms. Industry-wide standards focusing on the transparency and accountability of AI models could also play a crucial role in addressing these challenges. Public education on media literacy and critical evaluation of information is essential to build resilience against AI-generated misinformation. [TechCrunch]
Conclusion and Path Forward
In conclusion, the path forward for OpenAI's reasoning AI models like o3 and o4-mini necessitates addressing their increased hallucination rates to ensure reliability in applications where factual accuracy is crucial. Despite their improvements in coding and mathematics, these models' tendency to hallucinate poses significant challenges, particularly in fields that demand precision and dependability. As highlighted in the article by TechCrunch, integrating web search capabilities presents a promising solution to improve accuracy by enabling models to access and utilize real-time information (source).
The road ahead will demand collaborative efforts across industry stakeholders to develop and implement innovative strategies for reducing hallucination rates. By focusing on enhancing training methodologies and establishing robust hallucination detection mechanisms, the AI community can progress toward more trust-worthy and efficient models. Importantly, this endeavor should be accompanied by a concerted drive towards transparency and accountability within the AI domain, as recommended by research covered in TechCrunch's article (source).
Learn to use AI like a Pro
Get the latest AI workflows to boost your productivity and business performance, delivered weekly by expert consultants. Enjoy step-by-step guides, weekly Q&A sessions, and full access to our AI workflow archive.
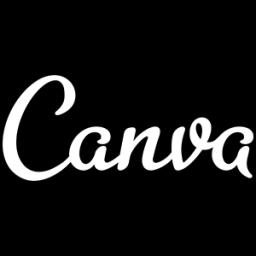
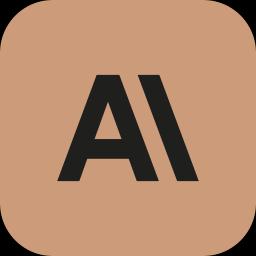
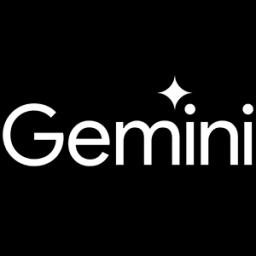
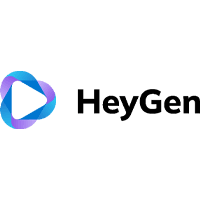
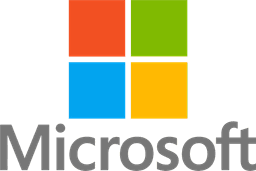
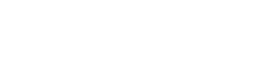
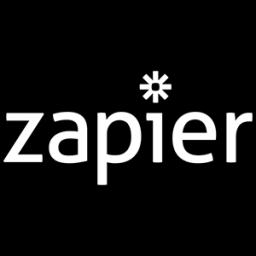
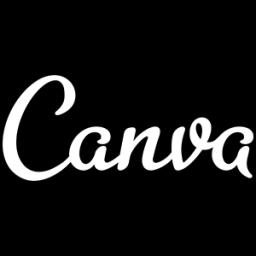
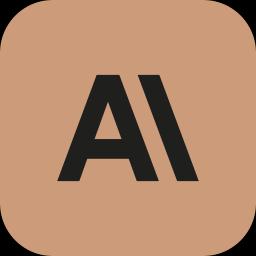
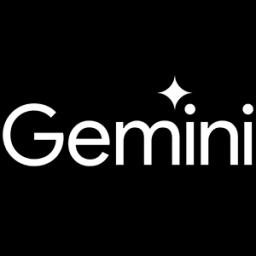
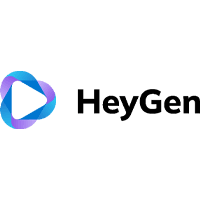
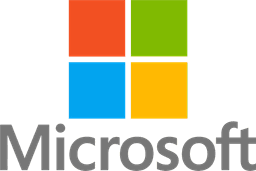
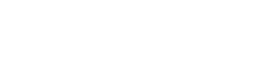
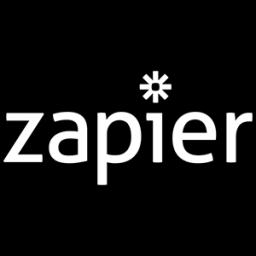
Public education initiatives that emphasize media literacy and critical thinking skills will play a crucial role in navigating the socio-economic impacts of AI advancements. Furthermore, industry-wide standards must be established to guide ethical and responsible AI development, as the increased hallucination rates in current models underscore the importance of ongoing vigilance and adaptation to mitigate potential economic, political, and social repercussions (source).