Revolutionary strides and steep costs
OpenAI's o3 Model: A New Era in AI Scaling and Costs
Last updated:

Edited By
Mackenzie Ferguson
AI Tools Researcher & Implementation Consultant
OpenAI's latest AI model, o3, has made significant leaps in reasoning, coding, and logic capabilities, edging closer to human-level tasks. However, these advancements come with hefty computational costs, sparking debates about accessibility and the digital divide. As AI progresses, balancing innovation with practicality becomes crucial.
Introduction
OpenAI has recently unveiled its latest AI model, o3, which stands out for its enhanced reasoning capabilities. This model surpasses its predecessors, like GPT-4, in handling complex tasks such as coding and logical problem-solving with improved accuracy. Nevertheless, while o3 marks a significant advancement in AI technology, it still faces challenges in overcoming certain reasoning hurdles, sometimes producing incorrect or nonsensical outputs. A particularly pertinent issue arising with the development of such sophisticated models is the substantial computational resources they require, which poses questions about the scalability and accessibility of AI technology for broader audiences. This marks the beginning of a 'second era of scaling laws' in artificial intelligence, where the focus shifts towards streamlining model architecture and training for efficiency, rather than solely increasing model size.
Advancements in AI Reasoning: o3's Capabilities
The advancement of AI reasoning capabilities in OpenAI's o3 is seen as a major step forward in the field. The model boasts improved reasoning skills, particularly in areas like coding and logical problem-solving, when compared to its predecessors, such as GPT-4. This enables it to tackle more complex tasks with better accuracy across various benchmarks. However, despite these advancements, the model is not without its challenges; it still struggles with certain reasoning tasks, often producing incorrect or nonsensical outputs. This highlights the ongoing need for research to resolve these limitations and enhance overall performance.
Learn to use AI like a Pro
Get the latest AI workflows to boost your productivity and business performance, delivered weekly by expert consultants. Enjoy step-by-step guides, weekly Q&A sessions, and full access to our AI workflow archive.
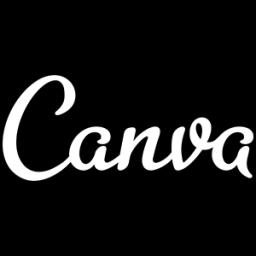
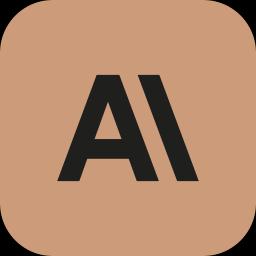
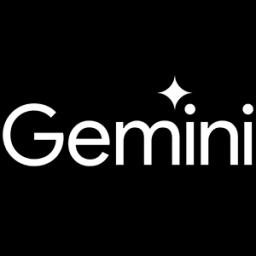
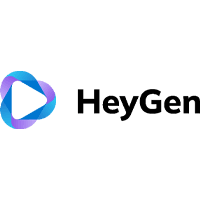
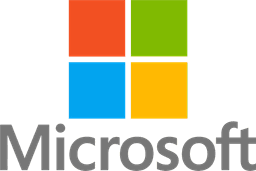
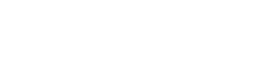
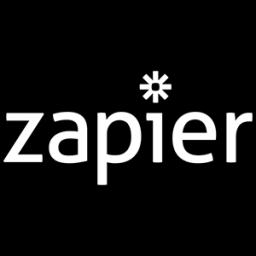
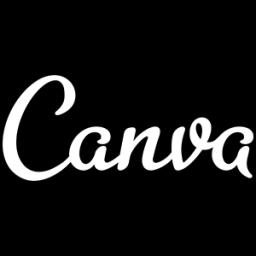
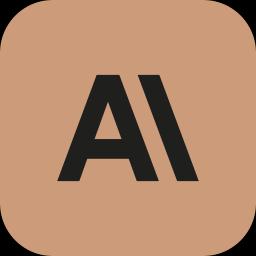
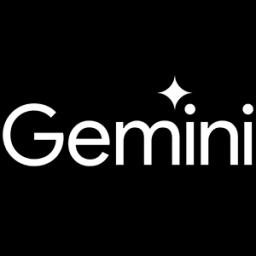
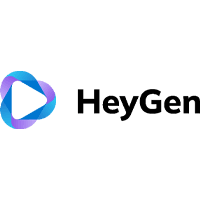
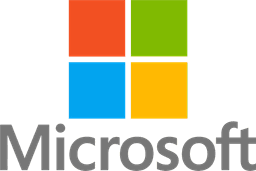
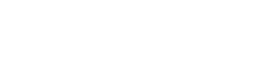
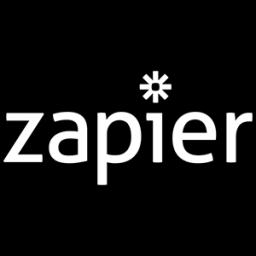
One of the major trade-offs of developing sophisticated AI models like o3 is the significant computational cost it incurs. The high resources required for both training and running these models raise concerns regarding their scalability and accessibility. Such costs pose potential barriers for researchers and developers, especially those with limited resources, to engage with the latest advancements in AI. Thus, scaling laws are now being viewed with a shift in focus: rather than merely increasing model size, efforts are directed towards optimizing model architecture and training techniques for better efficiency and performance.
The broader implications of o3 on the future of AI are multifaceted. While it represents a notable advance in reasoning capabilities, it underscores the importance of balancing performance gains with computational efficiency. This balance is crucial as researchers and developers continue to explore new methods and innovations to push the boundaries of AI development. Economic and ethical considerations also play a significant role, as high costs might exacerbate inequalities, leading to digital divides. The ongoing dialogue about AI governance and the necessity for regulations ensuring ethical development further complicate the landscape as AI models approach tasks with human-like proficiency.
o3's Limitations and Challenges
OpenAI's o3 model represents a major advancement in artificial intelligence, showcasing improved reasoning abilities compared to its predecessors. Despite these advancements, o3's development highlights significant challenges and limitations in the field of AI.
One prominent issue with o3 is its struggles with certain types of reasoning challenges. While it excels in complex tasks such as coding and logical problem-solving, o3 can still produce incorrect or nonsensical outputs. This inconsistency raises questions about the reliability and robustness of sophisticated AI models such as o3.
Learn to use AI like a Pro
Get the latest AI workflows to boost your productivity and business performance, delivered weekly by expert consultants. Enjoy step-by-step guides, weekly Q&A sessions, and full access to our AI workflow archive.
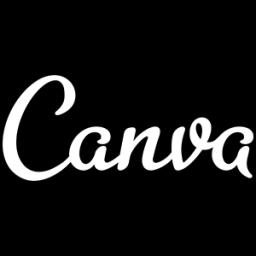
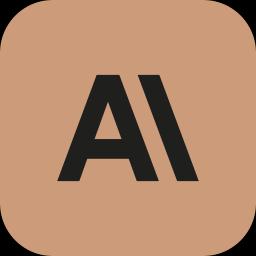
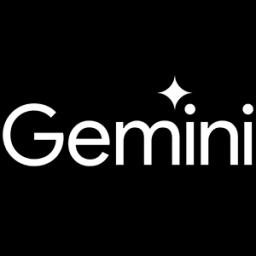
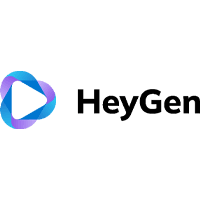
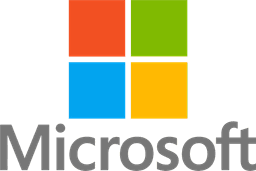
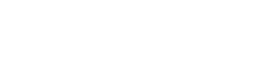
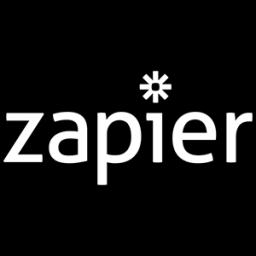
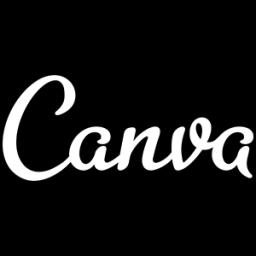
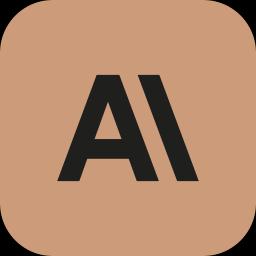
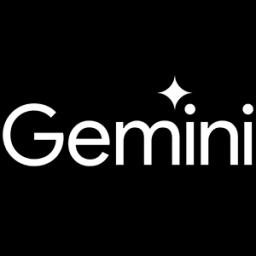
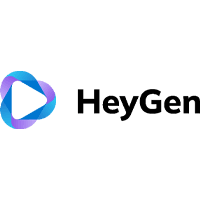
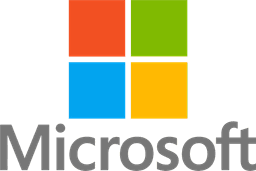
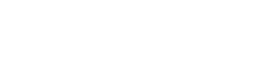
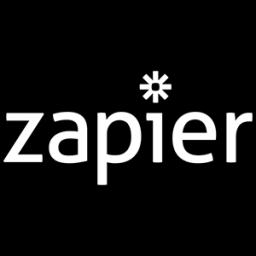
Moreover, the development of advanced AI models like o3 comes with substantial computational costs. The resources required for training and operating these models are significant, raising concerns about the scalability and accessibility of such technologies. This economic impact could limit the wider adoption of AI, creating barriers for researchers and developers who lack substantial funding.
Another challenge that o3 faces is the "second era of scaling laws" in AI. This era focuses on optimizing model architecture and training techniques rather than merely increasing model size, as previously explored. The emphasis is placed on finding balance between performance gains and computational efficiency.
Overall, while o3 underscores the potential for continued improvements in AI reasoning capabilities, it also calls attention to the necessity of addressing computational and reasoning limitations. This underscores the need for innovative approaches to AI model development that consider both performance and efficiency.
The Economic Implications of High Computational Costs
The release of OpenAI's latest AI model, o3, has shone a spotlight on the substantial economic implications of high computational costs in the development of sophisticated AI technologies. This model, which boasts significant advancements over its predecessors in reasoning capabilities, also illustrates the increasingly prohibitive costs associated with cutting-edge AI research and development. As AI models continue to scale, the economic burden of training and deploying these systems grows, raising important questions about the sustainability and accessibility of future AI innovations.
At the core of these economic challenges is the high cost of computational resources required to both train and operate models like o3. Such costs are not only measured in financial terms but also in energy consumption and environmental impact, which can be substantial. This reality underscores the need for a balanced approach to AI innovation, where the benefits of enhanced capabilities are carefully weighed against the economic and environmental costs associated with achieving them.
The increasing computational costs are likely to impact various sectors, potentially accelerating job displacement in fields that involve complex reasoning tasks, such as software development, engineering, and data analysis. As companies and industries adapt to these changes, there may be a growing demand for professionals who can integrate AI technologies effectively into their workflows, alongside an emphasis on developing skills that complement AI rather than compete with it.
Learn to use AI like a Pro
Get the latest AI workflows to boost your productivity and business performance, delivered weekly by expert consultants. Enjoy step-by-step guides, weekly Q&A sessions, and full access to our AI workflow archive.
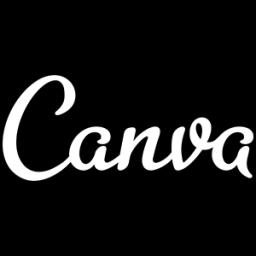
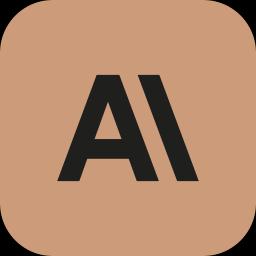
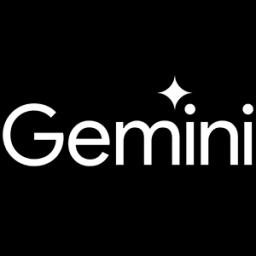
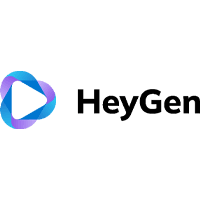
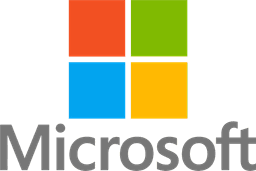
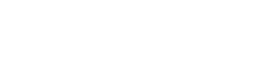
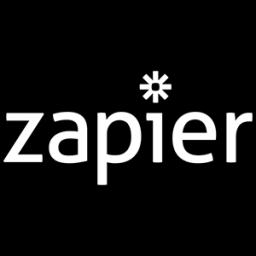
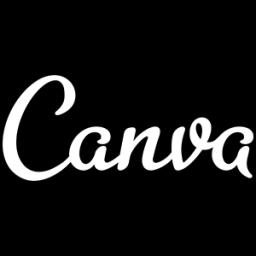
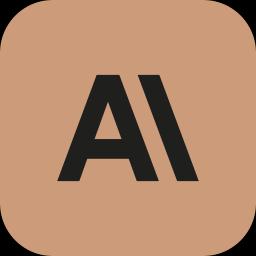
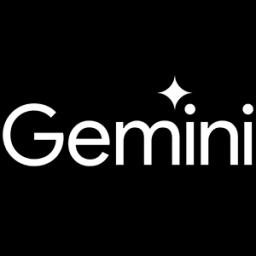
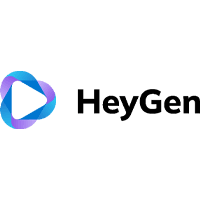
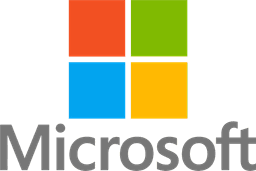
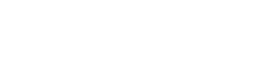
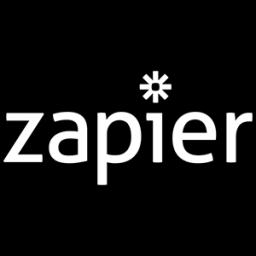
Moreover, the high barriers to entry presented by the cost of cutting-edge AI development may limit wider participation in AI research and innovation. Smaller companies and researchers with limited resources could find themselves at a disadvantage, potentially exacerbating existing inequalities and concentrating AI capabilities within a few large, well-resourced entities. This could hinder diversity in AI development and limit the range of applications and perspectives that shape future AI solutions.
In response to these challenges, there is an emerging focus on the "second era of scaling laws" in the AI field. This approach shifts the emphasis from merely increasing model size to optimizing architectures and training techniques for greater efficiency. Such innovations are critical to making advanced AI more economically viable and environmentally sustainable, ensuring that the future of AI development is inclusive and accessible to a broader range of stakeholders.
Second Era of Scaling Laws in AI
The Second Era of Scaling Laws in AI signifies a transformative period in artificial intelligence development where the emphasis shifts from merely increasing the size of AI models to optimizing their architecture and training techniques. This evolution in approach aims to enhance performance and efficiency, as seen with OpenAI's o3 model, which showcases improved reasoning capabilities while also highlighting the challenges of computational costs.
OpenAI's recent release of the o3 model marks a significant milestone, showcasing enhanced reasoning capabilities, particularly in complex tasks like coding and logical problem-solving. Compared to its predecessors such as GPT-4, o3 demonstrates a higher level of proficiency, although it still faces challenges with certain reasoning tasks and can output incorrect information. The model's development underscores a broader trend in AI research towards optimizing architectural efficiency and training techniques, stepping beyond the traditional scaling approach of simply enlarging model size.
The advancement represented by o3 suggests the 'Second Era of Scaling Laws' in AI, where researchers focus on architectural and training optimization rather than size increases alone. This shift is driven by the need to balance performance improvements with the ever-growing computational costs associated with developing sophisticated AI models. The exploration of innovative approaches in AI continues as researchers seek to maximize efficiency and effectiveness within these new paradigms.
From a developmental perspective, the o3 model highlights both the potential and the challenges inherent in the latest scaling strategies. While it excels in complex reasoning tasks, it also illuminates the substantial computational resources required to achieve such performance levels. This development raises fundamental questions regarding the economic viability and accessibility of advanced AI technologies, emphasizing the importance of optimizing efficiency in future models.
Learn to use AI like a Pro
Get the latest AI workflows to boost your productivity and business performance, delivered weekly by expert consultants. Enjoy step-by-step guides, weekly Q&A sessions, and full access to our AI workflow archive.
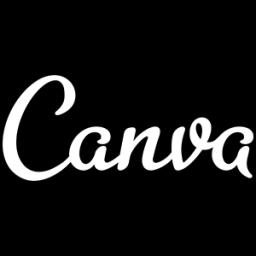
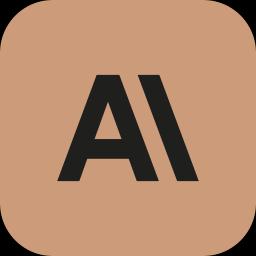
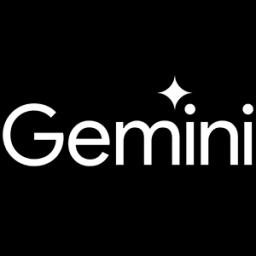
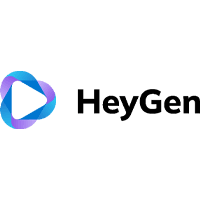
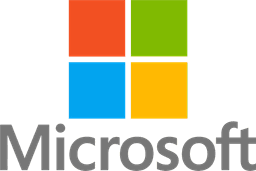
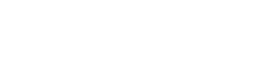
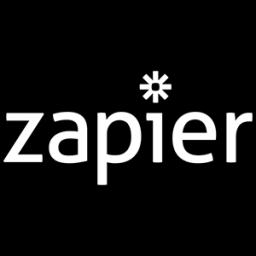
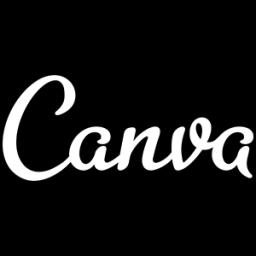
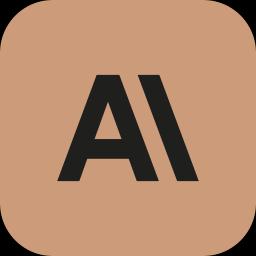
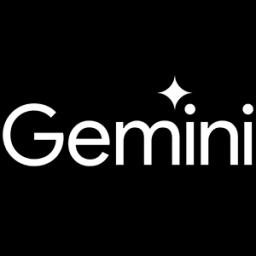
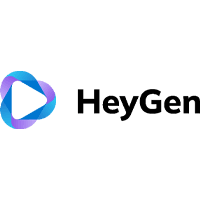
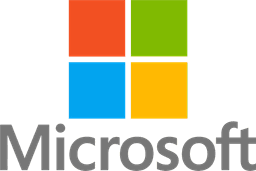
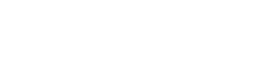
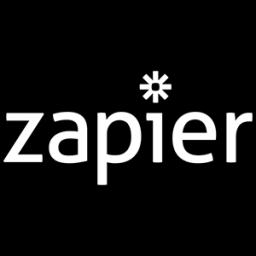
The concept of the Second Era of Scaling Laws suggests a forward-thinking approach in AI development, encouraging innovation in how models are built and trained. This includes exploring methods that may enhance the reasoning capabilities of AI without proportionally increasing the computational burden. As the AI community continues to delve into this new era, the ultimate balance between model sophistication and resource efficiency remains a primary focus of research efforts.
Impact of o3 on Future AI Development
OpenAI's latest AI model, o3, marks a significant milestone in the development of artificial intelligence due to its advanced reasoning capabilities. Unlike its predecessors, such as GPT-4, o3 excels in tasks that require complex problem-solving skills, including coding and logical reasoning. Despite this progress, the model still faces challenges with various reasoning tasks, often producing incorrect outputs. The immense computational costs associated with developing such sophisticated AI models also present barriers to broader adoption and present potential accessibility issues for smaller organizations and researchers with limited resources.
o3's advancements underscore the shift towards the 'second era of scaling laws' in AI, where the focus is increasingly on optimizing model architecture and training methods rather than merely scaling up model size. This approach aims to enhance AI performance and efficiency, paving the way for innovative model developments beyond traditional practices. However, this also emphasizes the need to find a balance between enhancing performance and maintaining computational efficiency to ensure AI development remains sustainable and accessible to a wider audience.
Despite its impressive reasoning abilities on several benchmarks, o3 is not without its limitations. It struggles with simpler reasoning tasks, producing incorrect results at times, and fails to solve numerous visual puzzle tasks. This indicates that while the model represents significant progress, it is still far from achieving Artificial General Intelligence (AGI), and substantial hurdles remain.
The development and deployment of models like o3 are accompanied by high computational costs, which could have far-reaching implications for AI development's scalability and accessibility. These costs may restrict adoption to only well-funded institutions and technology companies, potentially widening the digital divide. This raises economic and ethical concerns, particularly regarding the viability of using such advanced AI technologies in a broad range of applications.
Public reactions to the release of o3 have been varied, reflecting both excitement and skepticism. Many AI enthusiasts praise its potential to revolutionize fields reliant on complex reasoning, while others are concerned about the high computational costs and accessibility. Discussions on social media often debate whether o3 represents a true breakthrough towards AGI or merely an incremental improvement over existing technologies.
Learn to use AI like a Pro
Get the latest AI workflows to boost your productivity and business performance, delivered weekly by expert consultants. Enjoy step-by-step guides, weekly Q&A sessions, and full access to our AI workflow archive.
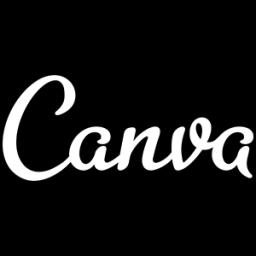
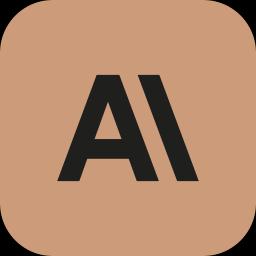
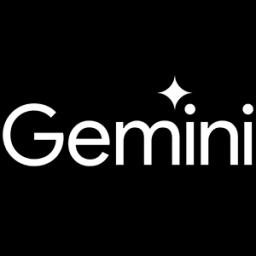
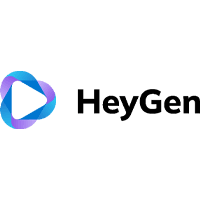
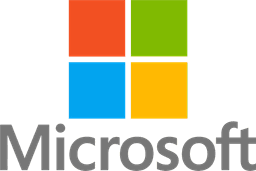
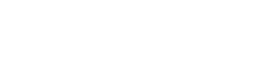
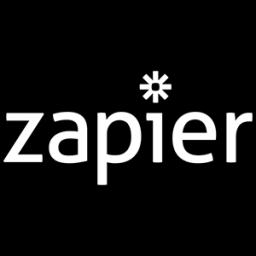
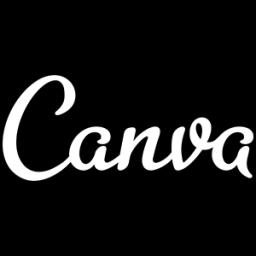
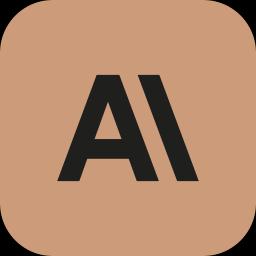
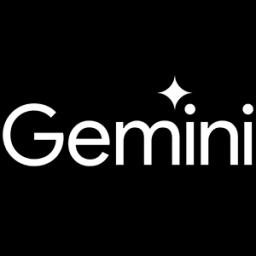
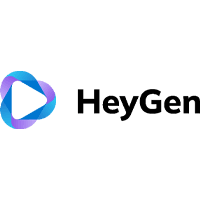
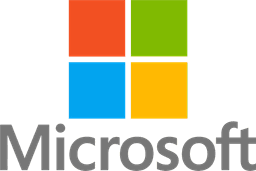
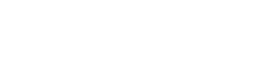
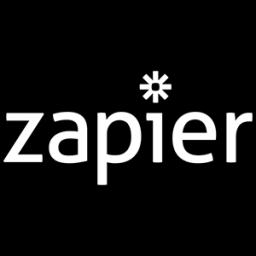
Looking ahead, the development of o3 could have profound implications across economic, social, political, and scientific domains. Economically, the increased demand for high-performance computing might drive up costs and accelerate job displacement in fields relying on AI-like reasoning. Socially, education systems may need to shift focus to skills that complement AI capabilities. Politically, there will likely be pressure to create comprehensive regulations governing AI usage. Scientifically, advancements in o3 highlight the need for more energy-efficient AI hardware and suggest a future research direction emphasizing model and training optimizations over mere scaling.
Significant Related Events in AI
The release of OpenAI's latest AI model, o3, marks a significant milestone in the field of artificial intelligence, particularly in the realm of reasoning capabilities. Compared to its predecessor, GPT-4, o3 showcases improved performance in handling complex tasks such as coding and logical problem-solving. However, despite these advancements, it continues to face challenges with some reasoning tasks, occasionally producing incorrect or nonsensical outputs. The development of such sophisticated models also brings to light the escalating computational costs associated with their training and deployment. As these costs rise, questions about the scalability and accessibility of advanced AI technologies surface, potentially limiting their broader adoption among researchers and developers with constrained resources.
In the wake of o3's development, several key events have emerged in the AI landscape. Notably, Anthropic's launch of the Claude 3 model family introduces improved capabilities in reasoning, task completion, and multimodal understanding. This advancement complements Meta's FAIR lab breakthrough in few-shot learning, a technique that allows AI models to learn new tasks with minimal examples, enhancing their adaptability. Meanwhile, DeepMind's unveiling of AlphaFold 3, capable of predicting protein structures with high accuracy, promises to revolutionize drug discovery and biological research. Similarly, Intel's introduction of the second-generation neuromorphic chip, Loihi 2, aims to replicate brain functions while enhancing energy efficiency for AI applications. These developments, alongside the implementation of the European Union's AI Act, which sets global standards for AI regulation, form a framework influencing future AI innovation and governance.
o3's capabilities have sparked a range of reactions from both experts and the public. François Chollet, creator of the ARC-AGI benchmark, acknowledges the progress o3 has made but emphasizes that it remains far from achieving Artificial General Intelligence (AGI). He points out its struggles with simpler tasks, highlighting the substantial challenges ahead. Melanie Mitchell, an AI researcher, critiques the reliance on brute-force computation, which might undermine the essence of the ARC Challenge, a tool for testing AI reasoning. Despite its high computational power, o3 failed to solve numerous visual puzzles. Economically, the cost of running o3 raises concerns about its viability and accessibility, with expenditures ranging significantly based on task complexity.
Public reactions to o3's release have been mixed. While AI enthusiasts celebrate its achievements, particularly in STEM disciplines, others express skepticism. High computational costs stand as a barrier to accessibility, potentially exacerbating the digital divide. This has led to debates regarding whether o3 represents a true breakthrough or merely an incremental improvement. The public discourse is enriched by humorous takes on o3’s capabilities and limitations, as well as discussions about its implications for the future of employment within coding and complex reasoning fields. Additionally, questions arise about whether o3's advancements inch us closer to AGI, sparking polarized opinions across various platforms.
The future implications of OpenAI's o3 model are far-reaching, with potential impacts spanning economic, social, political, and scientific domains. Economically, the increasing demand for high-performance computing resources could drive up costs, thereby accelerating job displacement in technical fields. This may contribute to widening the digital divide, which could favor large tech conglomerates over smaller entities. Socially, o3 may prompt shifts in education and workforce training, steering them towards complementing AI rather than competing with it. Politically, there might be increased pressure to craft regulations that uphold ethical standards in AI development. Scientifically, similar to breakthroughs like AlphaFold 3 and innovations in energy-efficient chips, there is momentum towards refining AI model architecture and training techniques to enhance efficiency and performance.
Learn to use AI like a Pro
Get the latest AI workflows to boost your productivity and business performance, delivered weekly by expert consultants. Enjoy step-by-step guides, weekly Q&A sessions, and full access to our AI workflow archive.
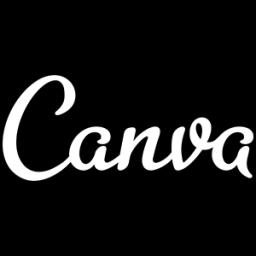
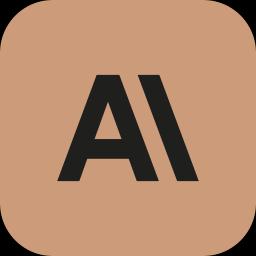
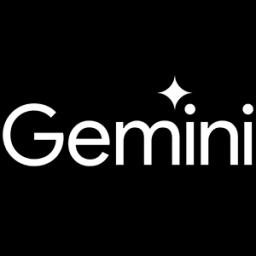
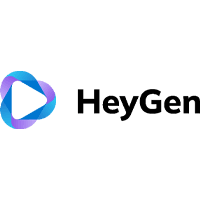
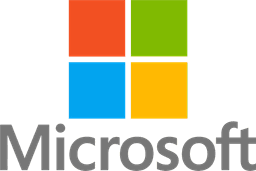
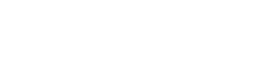
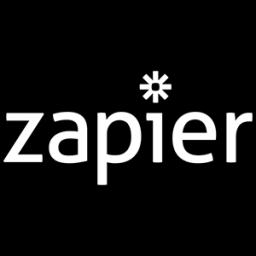
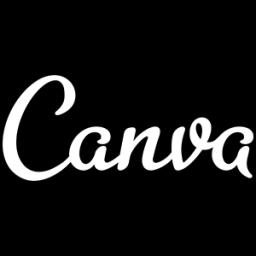
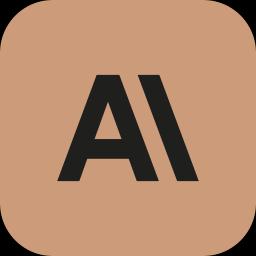
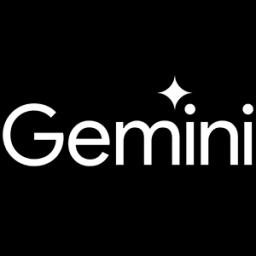
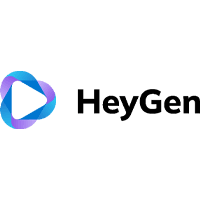
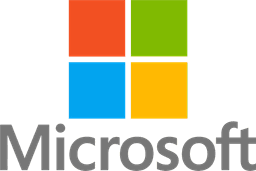
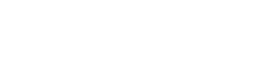
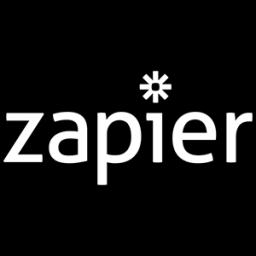
Expert Opinions on o3's Progress and Limitations
OpenAI's o3 has garnered diverse opinions from AI experts, heralding both its breakthroughs and its ongoing limitations. François Chollet, who devised the ARC-AGI benchmark, admires o3's achievements yet underscores its distance from true Artificial General Intelligence (AGI). Despite surpassing previous models on complex tasks, such as those measured by ARC-AGI, Chollet points out significant hurdles in o3's ability to handle simpler challenges effectively. This gap suggests that while o3 showcases the potential for enhanced reasoning, it remains mired in issues that must be resolved before it can mimic human-like understanding.
Similarly, Melanie Mitchell, an esteemed AI researcher, expresses skepticism over o3's reliance on brute-force computation to achieve high scores. She questions whether this approach undermines the genuine purpose of evaluating reasoning abilities, particularly as o3 falters in visual puzzle tasks—an area demanding nuanced understanding. Such challenges bring to light the complexities involved in the development of AI systems that truly comprehend the world around them.
The development of o3 has also sparked discussions about the economic and ethical implications of its computational demands. With operations costing thousands of dollars per problem, experts worry about the accessibility and economic feasibility of deploying such models on a wide scale. As OpenAI pushes boundaries, the high costs associated with running these models raise concerns about exacerbating inequalities, particularly for researchers and developers with limited funding.
Moreover, there's a noticeable consensus among experts about the progress represented by o3—viewed as a leap forward in AI reasoning without breaching the realm of AGI. This consensus calls for more sophisticated evaluation metrics to genuinely assess AI performance. The ongoing debate underscores a recognition of o3's potential impact on the field while acknowledging its limitations, inviting more nuanced discussions on AI capabilities and the future of machine intelligence.
Public Reactions to o3: Enthusiasm, Skepticism, and More
The release of OpenAI's o3 model has been met with a wide range of public reactions, reflecting the diverse perspectives on advanced AI development. Enthusiasts and tech experts have been quick to praise o3 for its remarkable improvements in reasoning capabilities, particularly in STEM fields. Many see it as a significant leap forward in the journey towards achieving artificial general intelligence (AGI). Discussions on platforms like Reddit and Twitter abound with optimistic predictions about the model's potential to revolutionize fields such as coding and complex problem-solving.
However, alongside these positive reactions, a significant amount of skepticism and concern has been voiced by various stakeholders. One major point of contention is the high computational cost associated with running o3, which may exacerbate existing digital divides by limiting access to well-funded institutions. The economic implications extend further, with debates about potential job displacement in sectors reliant on reasoning-intensive tasks. These concerns are compounded by calls from the public and experts alike for more transparency from OpenAI in terms of the model's capabilities and limitations.
Learn to use AI like a Pro
Get the latest AI workflows to boost your productivity and business performance, delivered weekly by expert consultants. Enjoy step-by-step guides, weekly Q&A sessions, and full access to our AI workflow archive.
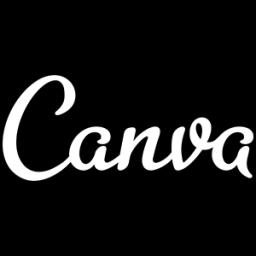
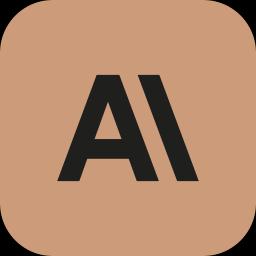
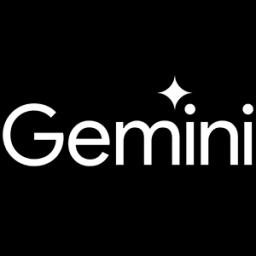
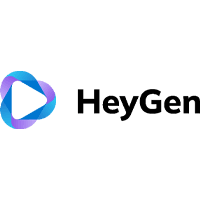
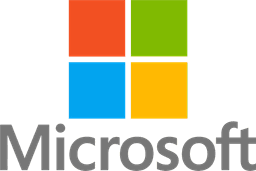
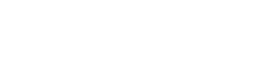
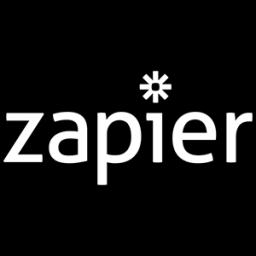
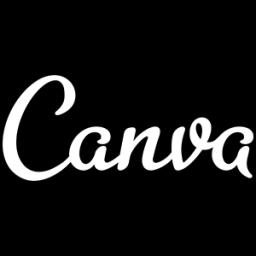
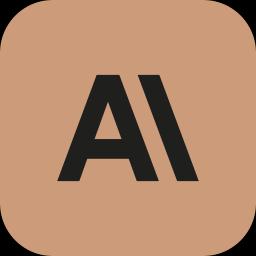
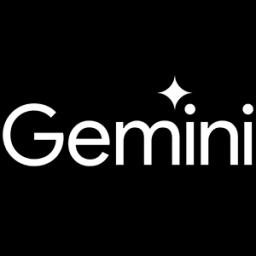
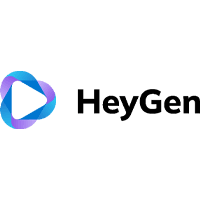
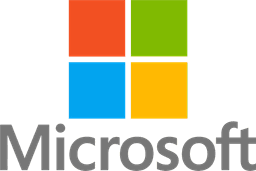
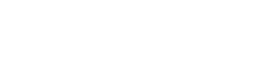
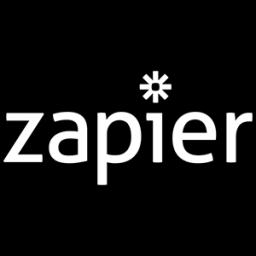
Humor and curiosity have also found their place in the discourse, with social media users making light-hearted jokes about why OpenAI seemed to skip an 'o2' model, attributing it to trademark issues, and poking fun at o3's peculiar shortcomings in certain tasks. Meanwhile, the debate on whether o3 should be considered a step toward AGI remains highly polarized. Experts caution against overstatement, suggesting that while o3 represents progress, it is far from achieving the sort of true, general intelligence characteristic of human cognition.
Overall, the public's reaction to o3 encapsulates a mix of enthusiasm and skepticism, humor and curiosity, optimism for the future and concern over socio-economic implications. This multifaceted response underscores the complex landscape of AI advancement where technological achievements are continuously assessed against their broader impacts on society and economy.
Future Implications of o3 in Society and Industry
The advent of OpenAI's o3 model underscores a pivotal shift in the landscape of AI, revealing both its promising capabilities and considerable costs. The model's enhanced reasoning skills have been lauded for making significant strides in complex tasks such as coding and logical problem-solving, even surpassing previous benchmarks like GPT-4. However, these advanced abilities come with a significant caveat—the need for immense computational power and resources, which raises critical issues about the scalability and accessibility of such technology. These challenges may restrict the widespread adoption of models like o3, particularly for smaller entities lacking extensive resources. Moreover, the high costs associated with running such models could exacerbate existing inequalities, favoring larger tech companies and research institutions. Despite its potential, o3 remains far from reaching Artificial General Intelligence (AGI), with researchers pointing out its struggles with simpler reasoning tasks and occasional production of nonsensical outputs. This indicates that while o3 represents an advancement in AI development, it also necessitates continued research to optimize its efficiency and efficacy. The "second era of scaling laws" in AI suggests a promising avenue for addressing these challenges by focusing on innovative training techniques and model architecture optimizations, rather than merely increasing model size. The economic implications of o3's development are manifold. There is an anticipated increase in demand for high-performance computing resources, which could drive up prices and intensify the digital divide. Furthermore, o3's capabilities might accelerate job displacement within fields that require complex reasoning, prompting a recalibration in workforce development and education systems to focus on complementary cognitive skills. Socially, the rise of models like o3 is likely to provoke deeper public discourse around the definitions of intelligence and consciousness, as these AI systems begin to mirror human-level performance in certain areas. Coupled with this is the growing importance of addressing AI safety and ethical considerations, which are likely to foster more robust and globally-conscious discussions on AI governance. Policymakers will face increased pressure to craft regulations that not only promote innovation but also ensure ethical deployment and use of AI technologies to prevent misuse and ensure equitable access. Finally, the scientific community is poised to benefit from the advancements driven by models like o3. AI breakthroughs are expected to accelerate progress in areas such as drug discovery and biological research, further building on innovative projects like AlphaFold 3. There is also a potential shift towards developing energy-efficient AI hardware solutions, inspired by neuromorphic advancements such as Intel's Loihi 2 chip. Overall, o3 acts as a catalyst for reevaluating current AI development paradigms, pushing the boundaries of what's possible while reiterating the necessity for balancing technological prowess with sustainability and ethical responsibility.