Benchmarking Blues for OpenAI's O3
OpenAI's O3 Model Falls Short on the FrontierMath Benchmark: What's the Real Score?
Last updated:

Edited By
Mackenzie Ferguson
AI Tools Researcher & Implementation Consultant
OpenAI's O3 model, which initially claimed to solve over 25% of complex math problems on the FrontierMath benchmark, was found to have closer to a 10% success rate according to independent tests by Epoch AI. This discrepancy highlights the evolving nature of both the AI models and the benchmarks themselves. The incident underscores the importance of critically evaluating AI performance claims, as newer models like O4 and O3 mini have since outperformed O3 under the updated benchmark conditions.
Introduction to AI Benchmarking and FrontierMath
Artificial Intelligence (AI) benchmarking is an essential process to evaluate and compare the performance of various AI models. Within this field, tests like the FrontierMath benchmark play a crucial role. Developed by Epoch AI, FrontierMath is specifically designed to assess a model's capability in tackling complex mathematical problems. This benchmark serves as a standard to quantify how well different AI models perform in problem-solving scenarios that demand a high level of mathematical understanding and reasoning. Interestingly, the results on such benchmarks—while meant to guide improvements and innovations in the field—are not always straightforward or free from contention.
Recently, OpenAI's generative AI model, known as o3, came under the spotlight due to discrepancies in reported performances on the FrontierMath benchmark, as per a TechRepublic article. Initial reports by OpenAI suggested that o3 could solve over 25% of the test problems, showcasing a significant advancement in AI capabilities. However, independent testing by Epoch AI suggested a much lower performance at around 10%. This gap highlights the complexity involved in AI benchmarking and the nuances of testing AI models across different versions and test setups.
Learn to use AI like a Pro
Get the latest AI workflows to boost your productivity and business performance, delivered weekly by expert consultants. Enjoy step-by-step guides, weekly Q&A sessions, and full access to our AI workflow archive.
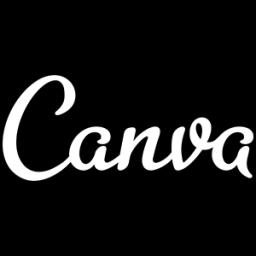
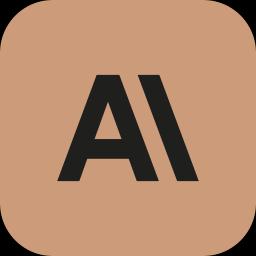
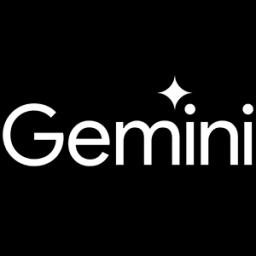
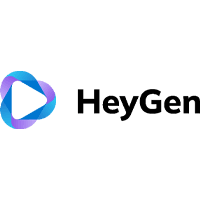
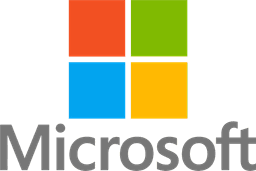
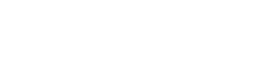
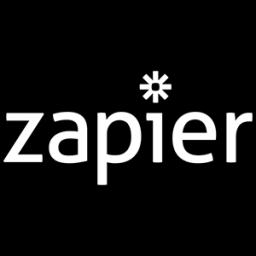
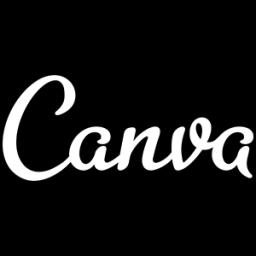
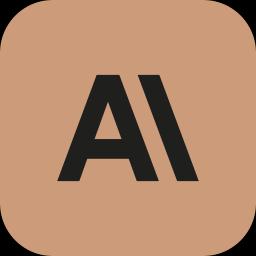
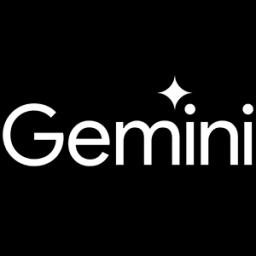
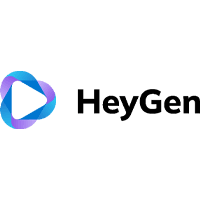
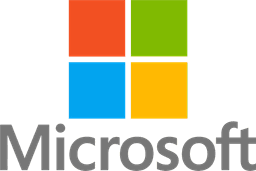
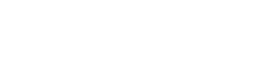
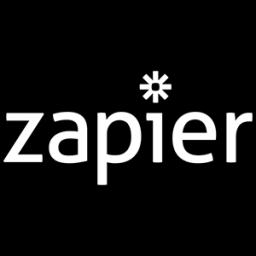
One of the key factors contributing to these discrepancies is the evolution of both the AI models and the benchmarks themselves. When OpenAI conducted their initial tests, they used a pre-release version of o3, which was not the same as the version later tested by Epoch AI. Moreover, the FrontierMath benchmark itself underwent changes, potentially altering the mix of problems or the difficulty level included in the test. As a result, subsequent versions of OpenAI's models, such as o4 and the o3 mini, were able to outperform the original o3 model on the updated benchmark, further emphasizing the dynamic interplay between AI development and benchmarking tests.
Beyond these specific instances, the broader methodology and reliability of AI benchmarks like FrontierMath have been subjects of criticism. Critics argue that benchmarks may suffer from biases embedded in test design and lack standardization across evaluations, which makes it challenging to compare results accurately. Such benchmarks are essential for driving forward AI development, yet they require careful scrutiny and continuous updates to remain relevant and objective. Users and developers alike must navigate these challenges while interpreting benchmark results to gauge true model performance.
Furthermore, the discrepancies observed in AI benchmark results underline the critical role of transparency in the AI industry. With competing claims and different test conditions, it becomes crucial for AI developers and researchers to provide clear, transparent information about the conditions and versions under which a model is tested. This transparency helps stakeholders—from developers to users—understand the real potential and limitations of AI models, thereby promoting trust and fostering a more informed and critical approach to AI development and deployment.
OpenAI o3 Model: Claims vs. Reality
The recent evaluations of OpenAI’s o3 generative AI model revealed significant discrepancies between its claimed performance and the findings of independent testing. Initially, OpenAI asserted that the o3 model successfully solved over 25% of the problems presented by the FrontierMath benchmark, a test known for its challenging mathematical problem sets. However, subsequent evaluations by Epoch AI indicated a performance closer to 10%, highlighting a stark contrast between expected and actual outcomes. This difference has been attributed primarily to changes between the pre-release version of the model and the one eventually tested, along with possible modifications in the FrontierMath benchmark itself. Such deviations underscore the importance of transparency and accuracy in AI performance claims, and serve as a reminder for stakeholders to critically evaluate AI benchmark results when considering technology adoption. More detailed insights can be read in the source article on TechRepublic.
Learn to use AI like a Pro
Get the latest AI workflows to boost your productivity and business performance, delivered weekly by expert consultants. Enjoy step-by-step guides, weekly Q&A sessions, and full access to our AI workflow archive.
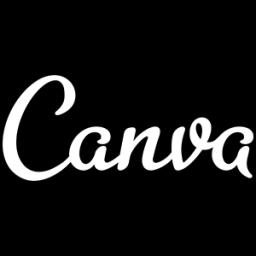
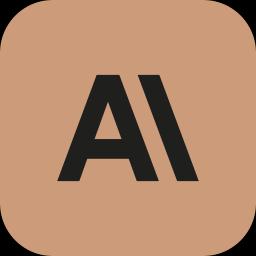
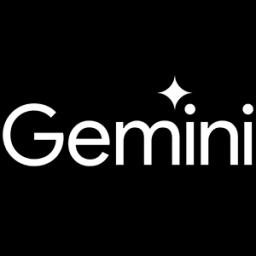
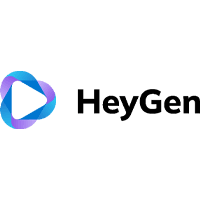
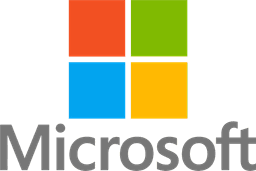
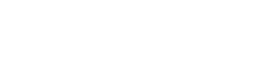
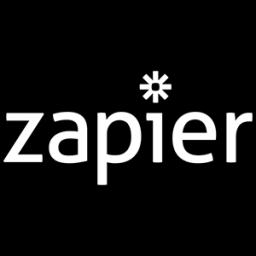
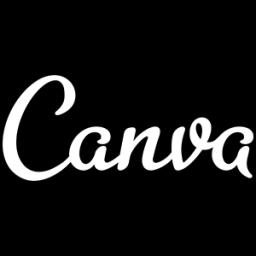
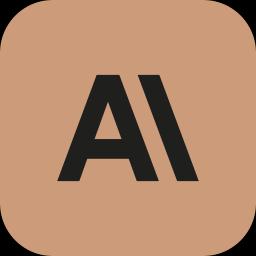
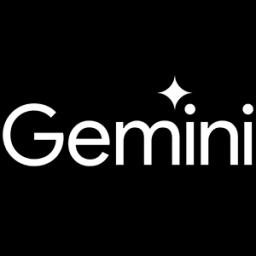
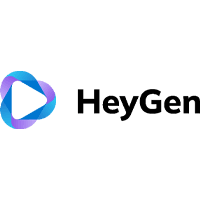
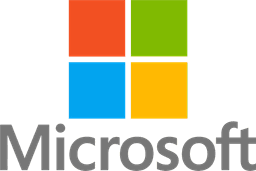
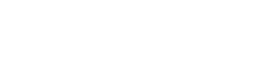
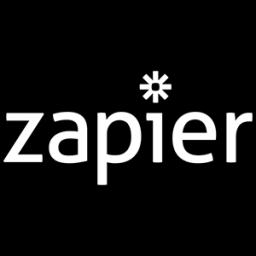
Further complicating the picture, newer iterations of OpenAI’s models, such as o4 and o3 mini, have reportedly outperformed the original o3 on the updated FrontierMath benchmark. Despite these improvements, the incident has spurred widespread discussion about the reliability of AI benchmarks in accurately representing true model capabilities. Critics emphasize that reliance on benchmark scores alone can be misleading due to variabilities in test design and execution. There's a growing call for more standardized and transparent benchmarking processes that fairly evaluate AI models' performances across diverse and realistic tasks. These concerns and insights are well-covered in the article on TechRepublic.
The scrutiny faced by OpenAI in light of these testing discrepancies is only part of a broader issue within the AI community. Models from other companies, such as xAI’s Grok 3 and Meta’s Vanilla Maverick, have similarly been questioned for benchmark score discrepancies, suggesting that this is a widespread problem rather than an isolated incident. Public and expert opinions converge on the need for greater transparency and accountability in AI development to ensure that benchmark claims can be substantiated by consistent and reproducible results. The detailed account of these events can be found on TechRepublic.
Public reactions to the discrepancies in AI model benchmarking have varied widely. Some stakeholders have expressed concerns about potential overstated claims and the need for more transparency in how AI performance metrics are communicated. There is also a broader conversation about re-evaluating the emphasis placed on benchmark performance, advocating instead for assessments of real-world applicability and user-centered design in AI systems. These mixed reactions highlight the complex dynamics of trust and expectation in the burgeoning AI marketplace and can be explored further through reports like those on TechRepublic.
In response to these issues, there are emerging discussions around future implications for the AI industry. Economic considerations include potential increases in the costs of ensuring rigorous testing procedures, which might affect the accessibility of AI research and development for smaller organizations. Socially, there could be an erosion of public trust in AI systems unless greater authenticity and validation in performance claims are established. Politically, this might catalyze the development of regulatory standards for AI benchmarking, pushing for clearer guidelines on how AI’s capabilities are advertised and verified. These aspects are dissected in the context of OpenAI’s challenges in articles such as the one from TechRepublic.
Comparison of AI Models on FrontierMath
The performance of AI models on benchmark tests like FrontierMath provides insights into their capabilities in solving complex mathematical problems. OpenAI's o3 model initially claimed a success rate of over 25% on the FrontierMath benchmark, a figure later contested by Epoch AI's findings of about 10%. This discrepancy underscores the significance of considering both the test conditions and the specific version of the AI model used during evaluation. Benchmark versions, computational resources, and the environment can drastically influence outcomes, as seen in the changes between OpenAI's pre-release and publicly tested versions of o3. To delve deeper into the nuances of these discrepancies, click here.
In understanding how various AI models perform on the FrontierMath benchmark, it is evident that OpenAI's subsequent models, o4 and o3 mini, showed improved performance compared to the original o3 model. This improvement highlights how AI models can evolve and refine their problem-solving abilities over time. Other models also participated in the same benchmark, including xAI's Grok-3 mini and Claude 3.7 Sonnet, each achieving varying levels of success. The diversity in performance among these models not only showcases the competitive nature of AI development but also emphasizes the importance of continuous evaluation and adaptation of these technologies. More details on model comparisons can be found in the TechRepublic article here.
Learn to use AI like a Pro
Get the latest AI workflows to boost your productivity and business performance, delivered weekly by expert consultants. Enjoy step-by-step guides, weekly Q&A sessions, and full access to our AI workflow archive.
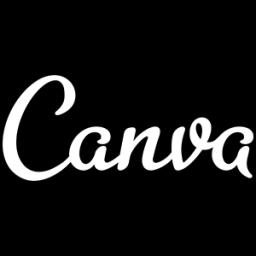
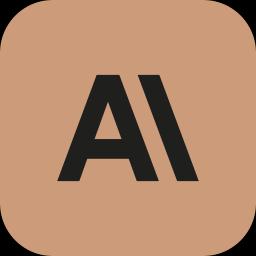
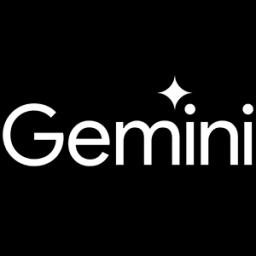
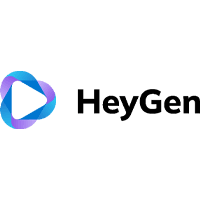
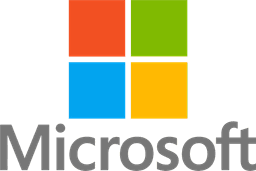
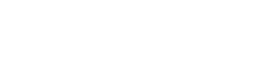
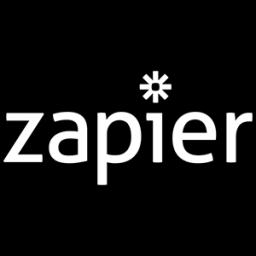
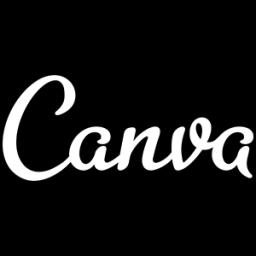
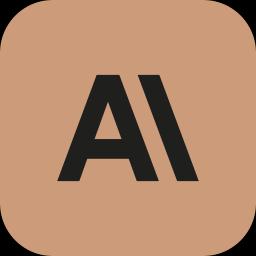
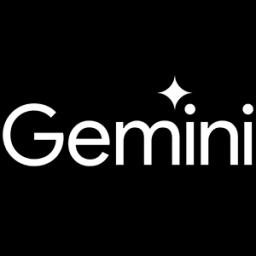
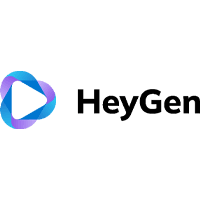
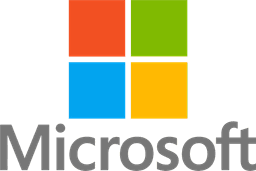
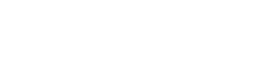
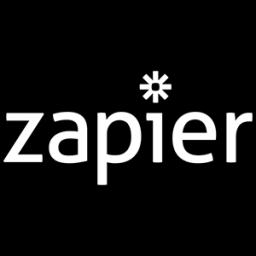
Criticism of AI benchmarking goes beyond mere performance discrepancies, pointing to structural issues within the testing process itself. Critics highlight the potential for bias in test design and the lack of standardization across different evaluations. These factors can distort the representation of an AI model's true capabilities, making it essential to scrutinize not only the scores but also the methodologies behind them. Such challenges have led industry experts and researchers to call for more transparent and consistent benchmarking practices. For further insights into the broader implications of AI benchmarking, refer to this discussion here.
Criticisms and Challenges in AI Benchmarking
AI benchmarking faces multiple criticisms and challenges, particularly in terms of transparency and reliability. Discrepancies, like those observed with OpenAI's o3 generative AI model, exemplify the complexities associated with ensuring accurate benchmarking. Initial claims about the o3 model's performance on the FrontierMath benchmark were significantly higher than what subsequent independent tests indicated, highlighting potential pitfalls in AI performance reporting. These benchmarks, developed to assess AI's proficiency in solving complex mathematical problems, are often susceptible to variances stemming from differences in testing environments or model versions. Furthermore, OpenAI's later models, such as the o4 and o3 mini, showed better performance, yet the initial misreporting underscores the challenge of maintaining consistency and honesty in AI benchmarks (source).
Critics commonly point out that AI benchmarks might unduly emphasize narrow task accuracy, leading to potentially misleading conclusions about a model's overall capabilities. This focus on specific tasks does not necessarily reflect real-world applications, where AI systems might be required to perform a variety of tasks seamlessly. Additionally, the lack of standardized evaluation practices further complicates the benchmarking landscape, making it difficult to compare models objectively. Inconsistent practices in AI benchmarking protocols could lead to ambiguously interpreted results, which skew public and commercial understanding of AI capabilities. These concerns are amplified by commercial interests, as companies may optimize models for specific benchmark outcomes, inflating perceived performance rather than delivering robust all-around capabilities (source).
The incident surrounding OpenAI's o3 model also reflects broader skepticism towards AI benchmarks generated under less-than-transparent circumstances. For instance, different versions of an AI model, like the beta compared to the commercial release, can exhibit markedly different performance levels. The publicized discrepancies in AI model evaluation could result from a number of factors, including evolving benchmark parameters and the use of specialized internal versions during testing. As highlighted by Epoch AI, the methodology and infrastructure used during independent testing differ significantly from those employed by developers in initial stages. These elements contribute to wider industry debates on the reliability of AI benchmarks and stress the need for more transparent and standardized procedures (source).
Ownership and Administration of FrontierMath
FrontierMath is a benchmark commissioned by OpenAI and is expressly owned by the organization. However, the operational aspects of the benchmark are entrusted to Epoch AI, which independently administers it. This division of ownership and administration responsibilities underpins a collaboration geared towards refining the accuracy and credibility of AI performance evaluations, especially in such a specialized field as mathematical problem-solving. The precise nature of this arrangement allows OpenAI to focus on the advancement of AI technologies, while Epoch AI’s independent oversight ensures rigorous monitoring and unbiased testing environments, both crucial for maintaining the integrity of AI benchmarks.
Such a separation of duties is not just procedural but also strategic, as it mitigates potential conflicts of interest that might arise from OpenAI owning the benchmark content while another organization administers it. This collaborative model exemplifies a broader trend in AI research, where checks and balances are institutionalized through shared responsibilities to enhance transparency and reliability. This approach also aligns with the growing demand for clearly defined roles and responsibilities among stakeholders in AI development and benchmarking, highlighting the importance of transparency in performance reporting.
Learn to use AI like a Pro
Get the latest AI workflows to boost your productivity and business performance, delivered weekly by expert consultants. Enjoy step-by-step guides, weekly Q&A sessions, and full access to our AI workflow archive.
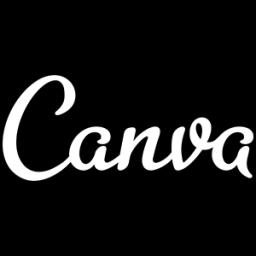
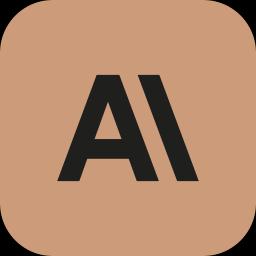
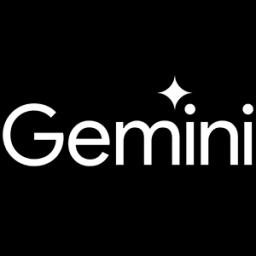
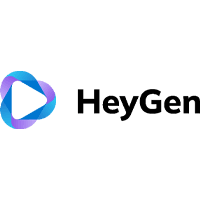
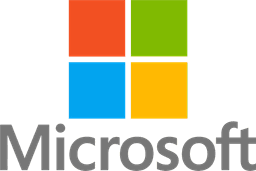
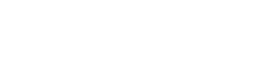
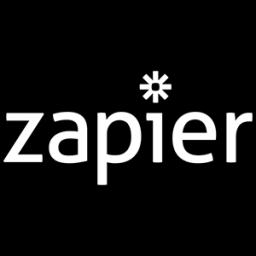
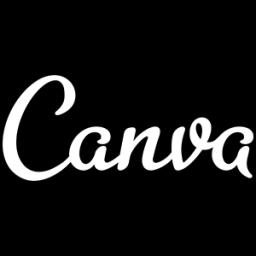
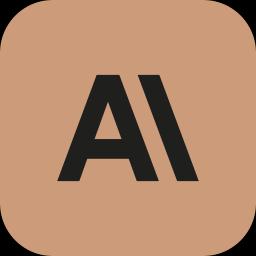
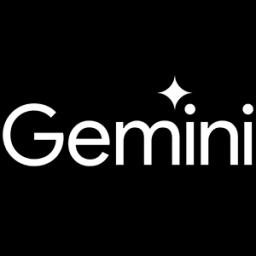
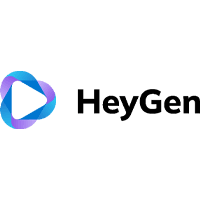
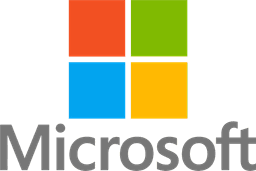
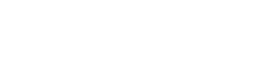
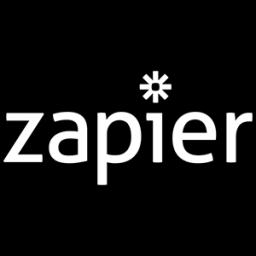
While OpenAI retains legal ownership of FrontierMath, making it the proprietary steward of the benchmark’s content, the active management by Epoch AI ensures that the benchmark evolves with advancements in AI technologies and methodologies. Epoch AI is tasked with not only administering but also potentially evolving the test conditions to reflect the dynamic nature of AI capabilities. This allows for a continuous improvement model where the benchmark remains relevant and challenging. The collaboration emphasizes the importance of evolving benchmarks that can keep pace with rapid technological developments and address industry criticisms regarding transparency and impartiality in testing.
Impact of Benchmark Discrepancies on AI Trust
The discrepancies in AI benchmarks, such as those highlighted by OpenAI's o3 model on the FrontierMath test, underscore the challenges in establishing trust in AI systems. Initially hailed for solving over 25% of the complex mathematical problems in the FrontierMath benchmark, the o3 model's performance was later contested by independent results from Epoch AI, showing closer to 10% accuracy. This gap in performance reports can be attributed to the differences in the model versions tested and the evolving nature of the benchmark itself. Such inconsistencies not only spark scrutiny over the benchmarks but also pose significant questions about the transparency and reliability of AI performance evaluations.
Benchmark discrepancies illuminate a critical issue in the AI industry: the trustworthiness of reported performance metrics. The commercial and reputational stakes of AI technologies drive companies like OpenAI to present their models in the best light possible, which sometimes results in discrepancies between initial claims and subsequent independent evaluations. The FrontierMath case with the o3 model demonstrates this tension, as the independent findings drew considerable attention to the potential biases in benchmark designs and the ways companies might leverage these narratives to suit their strategic interests. Such situations can influence public perception and trust, necessitating a more rigorous and transparent approach to AI evaluations.
Trust in AI is heavily impacted by how performance metrics are communicated and verified. The FrontierMath benchmark's discrepancies, particularly with OpenAI's o3 model, reveal the fragility of relying solely on reported metrics. While newer models like o4 and o3 mini have shown improved performance on updated benchmarks, the initial overstatement of the o3's capabilities could lead to skepticism among developers and the public. These disparities emphasize the need for standardized benchmarking practices and clearer communication from AI developers about the limitations and contexts of their test results, ensuring that the trust placed in AI systems is well-founded and informed.
Reactions and Implications for the AI Industry
The recent discrepancies in the performance reporting of OpenAI's o3 model have stirred considerable reactions and implications within the AI industry. Initially, OpenAI claimed its o3 model solved over 25% of the problems on the FrontierMath benchmark, a complex test designed to challenge AI systems with intricate mathematical problems. However, findings from Epoch AI revealed that o3 achieved only about 10% accuracy []. This revelation has prompted discussions about the integrity and reliability of AI benchmarks, and their impact on the industry's evolution.
One major implication of this discrepancy is the potential harm to the credibility of AI benchmarks in the industry. Benchmarks like FrontierMath are critical for evaluating AI systems' capabilities, but inconsistencies in test versions and performance reporting can raise questions about their reliability. Such events underline the necessity for standardized and transparent benchmarking processes to accurately portray AI models' capabilities and continually foster trust in AI advancements. The concern is not just limited to performance metrics but extends to the entire setup of test conditions [].
Learn to use AI like a Pro
Get the latest AI workflows to boost your productivity and business performance, delivered weekly by expert consultants. Enjoy step-by-step guides, weekly Q&A sessions, and full access to our AI workflow archive.
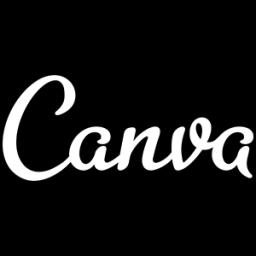
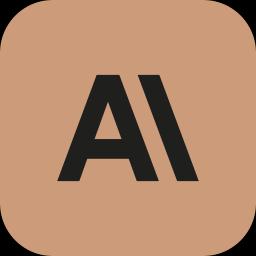
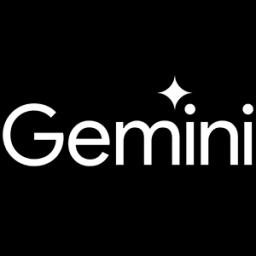
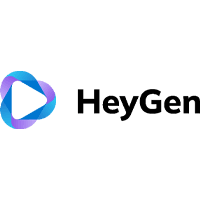
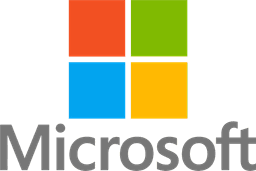
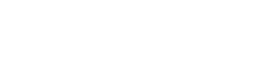
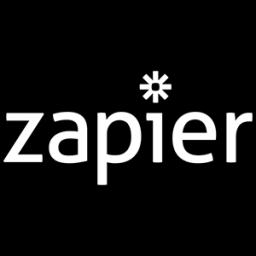
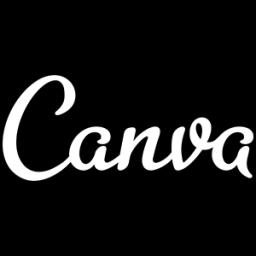
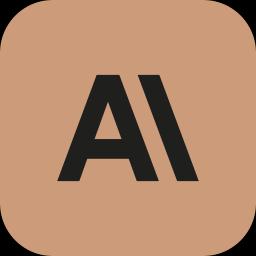
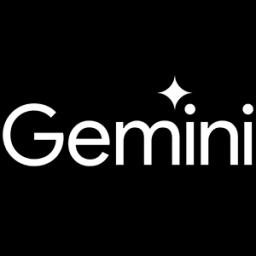
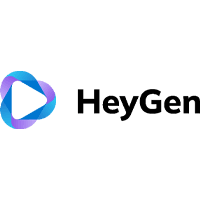
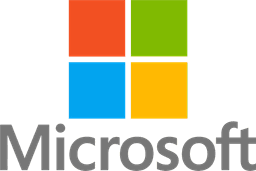
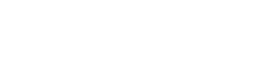
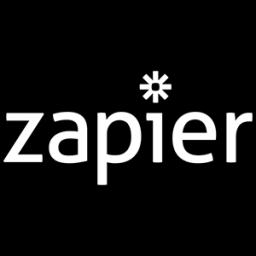
The response from various stakeholders in the industry suggests a push towards more transparency and accountability in AI performance claims. Given that AI models like OpenAI's o4 and o3 mini have set new standards with their improved performances, the industry faces pressure to ensure that reported performance metrics are unequivocally truthful and reflect real-world applications. This necessity is amplified by criticisms highlighting potential biases and non-standardized practices in current benchmarking methodologies [].
Moreover, the fallout from these discrepancies could catalyze changes in how AI technologies are evaluated and their impacts assessed. As AI systems become integral in fields ranging from healthcare to finance, the demand for trustworthy benchmarks that account for broader evaluation metrics rather than narrow, task-specific performances becomes paramount. Falls in public trust due to benchmark discrepancies could slow down the adoption of AI in sensitive sectors, necessitating enhanced efforts towards explainable AI that aligns performance with user expectations and ethical standards [].
Equally significant, these events may drive regulatory bodies to impose more stringent standards on AI performance advertising and benchmarking. Enhanced scrutiny from governments could evolve into policies mandating transparent performance verification, encouraging companies to invest in explainable AI solutions that justify their models' capabilities comprehensively. This shift has substantial economic and political implications, as it may impact innovation dynamics, international competitiveness, and the broader strategic landscape of AI research and deployment [].