OpenAI's GPT-5: The Game-Changer We Can't See
OpenAI's Secretive GPT-5 Could Transform the AI Landscape
Last updated:

Edited By
Mackenzie Ferguson
AI Tools Researcher & Implementation Consultant
Rumors are swirling that OpenAI has developed GPT-5 but is keeping it under wraps. The model may be used internally for ‘distillation’ processes to train smaller, optimized models that tackle rising inference costs. By privately leveraging GPT-5, OpenAI avoids triggering a Microsoft partnership clause and may focus on improving efficiency over public model releases.
Introduction
The introduction sets the stage for a discussion surrounding the technological and strategic choices of leading AI companies, specifically OpenAI, and their potential implications on the AI industry. As AI continues to evolve, companies are increasingly faced with critical decisions regarding the deployment and optimization of models like GPT-5. These decisions not only affect the companies' competitive edges but also have far-reaching impacts on industry trends and public perception.
In this context, the introduction outlines the rationale behind OpenAI's decision to keep GPT-5 under wraps. By strategically withholding its latest model, the company aims to tackle the rising costs and complexities associated with large-scale AI deployment. Furthermore, this move allows OpenAI to maintain a competitive advantage by focusing on model distillation for internal improvements, ultimately navigating the intricacies of AI development without triggering significant clauses in existing corporate partnerships.
Learn to use AI like a Pro
Get the latest AI workflows to boost your productivity and business performance, delivered weekly by expert consultants. Enjoy step-by-step guides, weekly Q&A sessions, and full access to our AI workflow archive.
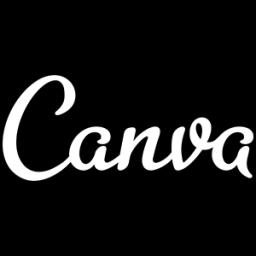
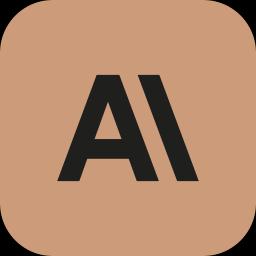
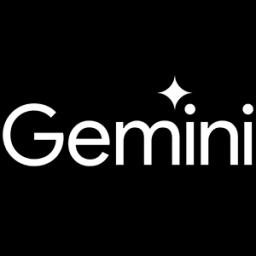
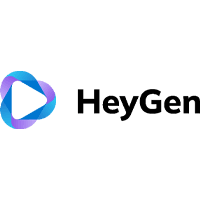
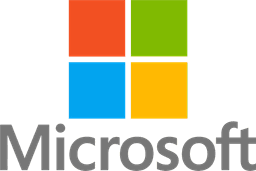
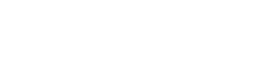
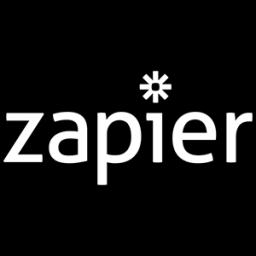
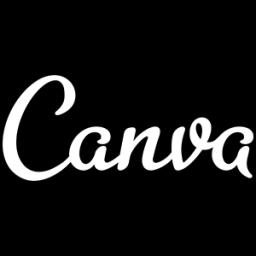
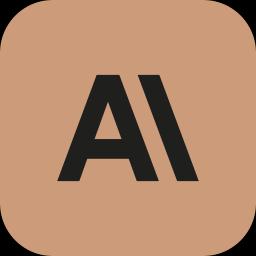
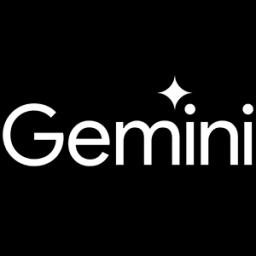
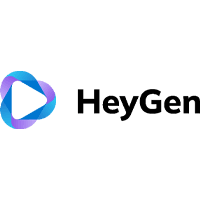
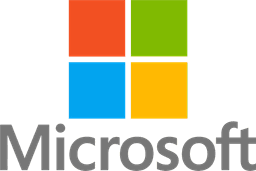
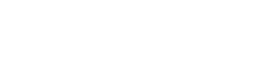
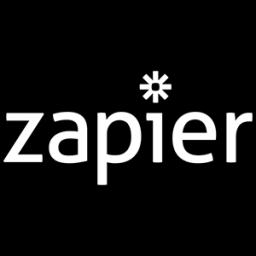
Through this strategic approach, OpenAI, alongside other industry giants like Anthropic and Google's DeepMind, showcases a shift in focus from merely scaling models to enhancing efficiency and addressing environmental concerns. This introductory segment also hints at the tension between maintaining proprietary advantages versus fostering transparency and accessibility in the AI domain, setting the stage for a deeper exploration of how such dynamics are reshaping the landscape of AI innovation and deployment.
The Existence and Purpose of GPT-5
OpenAI's strategic decision to keep GPT-5 under wraps suggests a profound shift in how AI advancements may unfold, prioritizing internal utility over public releases. The rationale behind keeping GPT-5 secret lies in circumventing high inference costs associated with large-scale deployment, thus preserving economic viability. Additionally, controlling the disclosure of GPT-5 avoids any potential disappointment if breakthroughs appear incremental and helps sidestep the 'AGI clause' in OpenAI's agreement with Microsoft.
Evidence hinting at the existence of GPT-5 is backed by noticeable industry trends leaning towards crafting smaller, more efficient models due to the rising scarcity of training data and increasing competitive pressure. OpenAI's stance on model scaling further underscores these insights. By epitomizing a 'teacher-student' approach, the distillation process enhances smaller model performance, aligning with the strategic thrust to streamline computational resource allocation.
The implications of this secrecy are far-reaching. A pivot towards recursive internal improvements rather than public rollouts could signify a new era in AI development centered around enhancing efficiency and model sophistication. However, this might result in reduced public accessibility to the latest AI technologies, possibly recalibrating AI company dynamics towards competitive advantage over sheer openness.
Learn to use AI like a Pro
Get the latest AI workflows to boost your productivity and business performance, delivered weekly by expert consultants. Enjoy step-by-step guides, weekly Q&A sessions, and full access to our AI workflow archive.
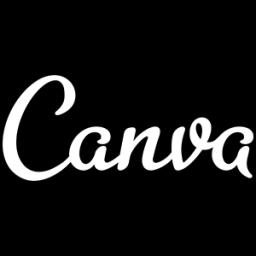
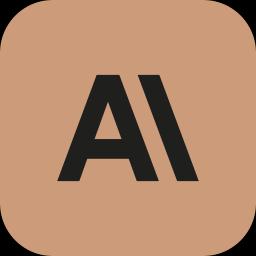
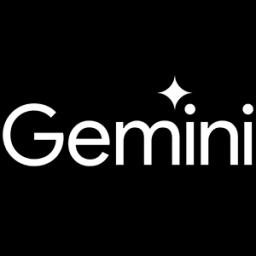
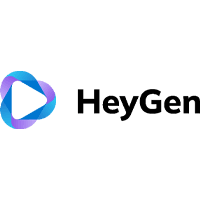
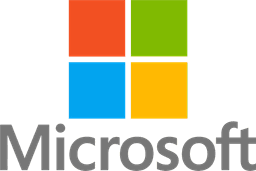
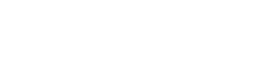
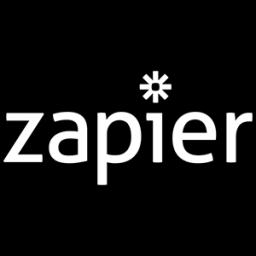
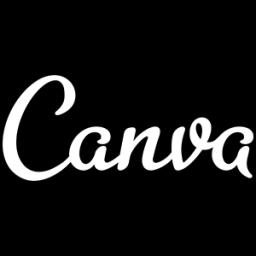
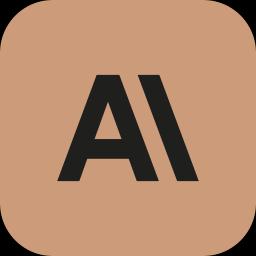
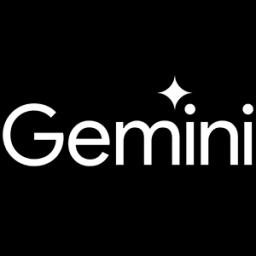
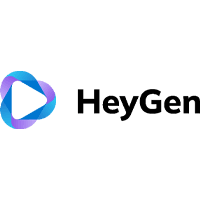
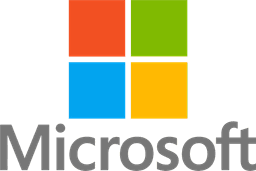
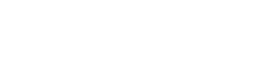
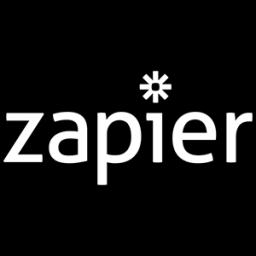
Complementing this secretive stance, other industry players like Anthropic and Meta are similarly honing their strategies for economic and environmental sustainability. Anthropic's advanced Claude models and Meta's energy-efficient training protocols demonstrate significant industry alignment in prioritizing optimization over conventional upscaling. These parallels in strategic initiatives underpin an emerging consensus on redefining AI priorities to maintain competitive edge and environmental responsibility.
Despite these advancements, public opinion seems split. While some users appreciate the strategic acumen of internal model improvements, others raise concerns around transparency and access to state-of-the-art AI capabilities. This dichotomy in public sentiment is accentuated by mounting anxieties over power centralization and declining performance in deployment-ready models, suggesting a growing desire for openness and clarity from leading AI firms.
Looking ahead, the AI sector is poised for further transformation. Closed development practices may drive competitive secrecy, potentially widening the capabilities gap between major AI companies and smaller challengers. Moreover, the emphasis on energy efficiency could catalyze innovations in sustainable AI training, aligning future industry growth with environmental imperatives. Regulatory frameworks will likely tighten as public demand for accountability and transparency continues to rise, reshaping how AI progress is negotiated globally.
Secrecy Surrounding GPT-5
The veil of secrecy surrounding GPT-5 is indicative of strategic maneuvers within OpenAI, reflecting a shift in priorities toward internal improvements over public disclosures. It is believed that OpenAI has already developed GPT-5, opting to use it internally for processes like model "distillation" rather than making it publicly available. This mirrors actions by other industry players, such as Anthropic with its Claude Opus 3.5, emphasizing a trend towards training smaller, more efficient models from larger ones to curb high inference costs.
One of the critical reasons for keeping GPT-5 under wraps involves avoiding the potential activation of the "AGI clause" in OpenAI's partnership with Microsoft. If publicly released, GPT-5 might be perceived as an advancement toward artificial general intelligence (AGI), triggering this clause and possibly leading to stringent scrutiny or changes in partnership dynamics. Thus, OpenAI’s approach ensures a strategic hold, leveraging GPT-5 internally to remain at the forefront of AI development without unleashing unnecessary repercussions.
Additionally, by holding back the public release of GPT-5, OpenAI minimizes the risk of dissatisfaction among stakeholders if the perceived development leap doesn't match public expectations. This controlled release strategy also paves the way for OpenAI to address resources and computational expenses, ensuring that any public deployment aligns with sustainable and economically viable practices.
Learn to use AI like a Pro
Get the latest AI workflows to boost your productivity and business performance, delivered weekly by expert consultants. Enjoy step-by-step guides, weekly Q&A sessions, and full access to our AI workflow archive.
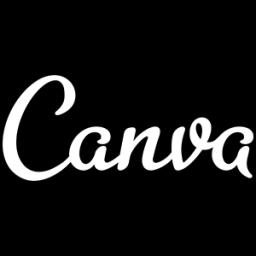
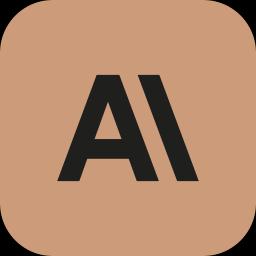
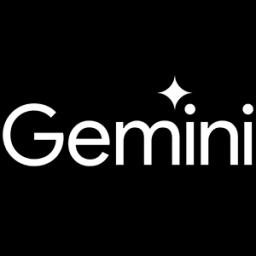
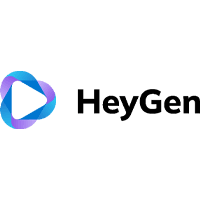
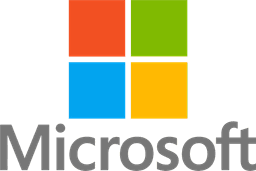
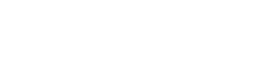
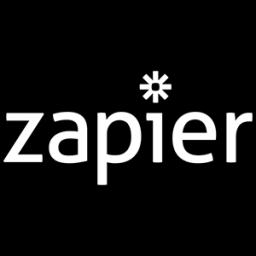
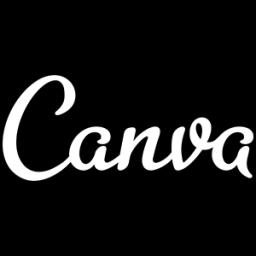
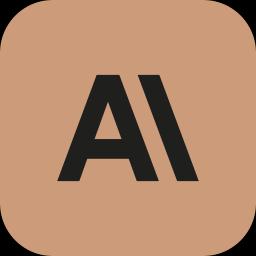
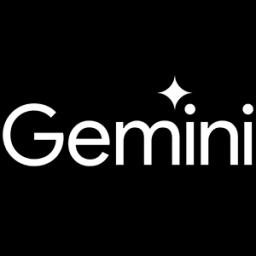
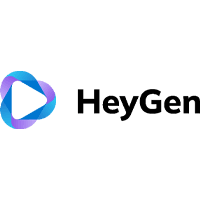
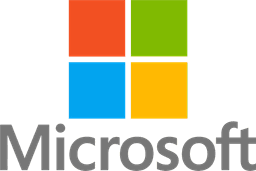
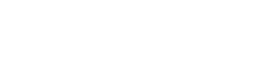
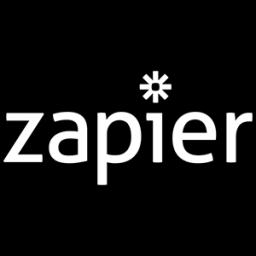
The industry's growing inclination toward internal refinement over public deployment is not isolated to OpenAI. With similar strategies emerging from competitors, this paradigm shift indicates a larger movement within the AI domain where internal efficiency, cost-effectiveness, and strategic advantages take precedence over immediate public accolades or releases. Such measures, while potentially limiting public accessibility to cutting-edge technology, reflect an adaptive approach to the challenges and costs imposed by modern AI infrastructure and capabilities development.
Evidence Supporting GPT-5's Development
OpenAI's development of GPT-5, although not publicly confirmed, is becoming a significant point of discussion in the AI community. The decision to keep GPT-5 behind the curtains aligns with strategic internal developmental goals. By focusing on "distillation," OpenAI aims to use GPT-5 to train smaller, more efficient models, thereby managing resource costs more effectively. This approach mirrors Anthropic's strategy with Claude Opus 3.5, showcasing a broader industry trend towards efficiency over mere scale. Such actions preemptively mitigate activation of the "AGI clause" in OpenAI's partnership with Microsoft, underscoring strategic priorities over disclosure. Moreover, this aligns with a potential industry-wide shift towards prioritizing internal advancements, offering a lens into future AI innovation strategies.
The speculation surrounding GPT-5's existence is bolstered by several indicators within the AI landscape. First, there is an observable trend toward the development of smaller, efficient models across the industry, driven by the scarcity of quality training data. Competitors like Anthropic epitomize this industry shift towards efficiency, reflecting broader movements in AI design frameworks. Additionally, OpenAI's previous assertions concerning model scaling have fueled beliefs that GPT-5 is indeed in development. Despite the lack of public release, these strategies suggest a focus on refining AI capabilities internally before offering them externally, paving the way for sustainable AI evolution without immediately escalating public expectations or resource constraints.
The methodology of "distillation," utilized by OpenAI, involves a sophisticated process where a larger "teacher" model is used to train a smaller "student" model. This enables the harnessing of complex, refined AI knowledge into more compact, economically feasible models, addressing the computational resource and cost challenges inherent to deploying large-scale language models. Through this process, deployment becomes more economically viable, marking a pivotal evolution in how AI models can be rendered both effective and efficient for diverse applications, ultimately ensuring better scalability from an infrastructural and financial perspective.
In terms of implications for AI development, OpenAI’s strategy reflects a significant pivot towards internal recursive improvement, where distilled models enhance core efficiencies quietly rather than showcasing raw size and power to the public. This suggests a reduced public access to the most advanced AI models, but it also indicates a strategic re-alignment towards optimization and efficient use of computational power. This strategic direction may lead to more refined AI functionalities, packaged meticulously to align with market needs and infrastructural capabilities. Such decisions are significant in reshaping future AI developmental priorities and operational strategies across competitive AI markets.
Distillation in AI Models
In recent developments within the AI sector, a strong emphasis has been placed on the concept of 'distillation' in model engineering. This approach, emphasized by leading tech companies such as OpenAI and Anthropic, seeks not only to reduce computational expenses but also to enhance the accessibility of advanced AI capabilities. Distillation involves utilizing a large 'teacher' model, which serves as an extensive reservoir of knowledge and functionality, to guide and train a smaller, more economically viable 'student' model. This method aligns with industry trends prioritizing efficiency and cost-effectiveness over merely scaling the size of AI models.
Learn to use AI like a Pro
Get the latest AI workflows to boost your productivity and business performance, delivered weekly by expert consultants. Enjoy step-by-step guides, weekly Q&A sessions, and full access to our AI workflow archive.
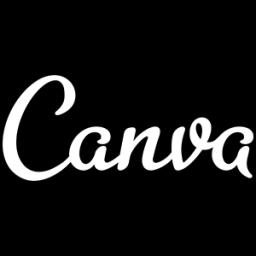
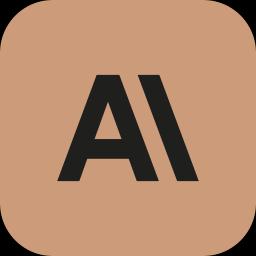
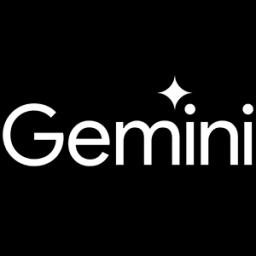
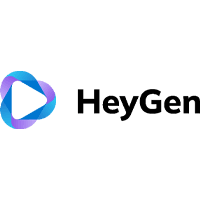
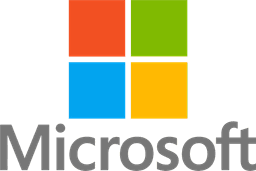
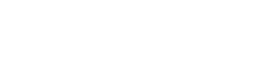
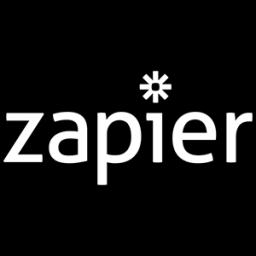
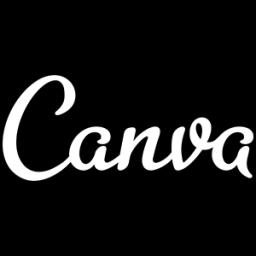
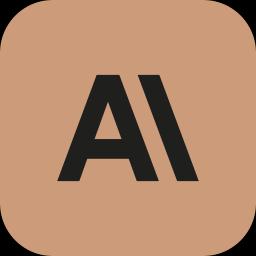
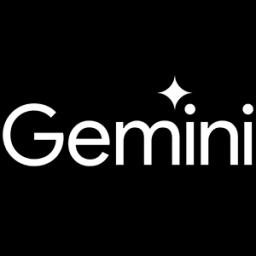
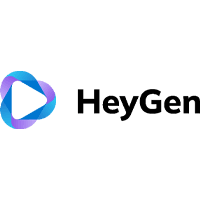
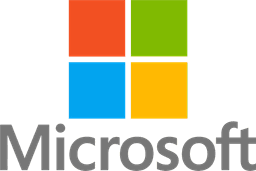
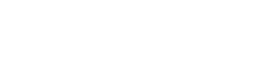
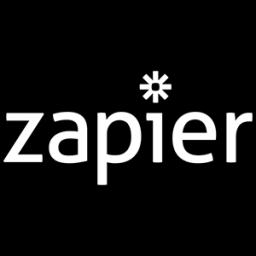
The concept of model distillation is becoming increasingly relevant in discussions about the future of AI development. This is particularly true in scenarios where the financial and environmental costs of running large AI systems are frequently cited as major concerns. By adopting a distillation strategy, companies like OpenAI are able to refine their models internally, potentially making these innovations available for broader use without the substantial overhead that would typically accompany larger models. This not only helps in reducing operational costs but also plays a vital role in minimizing the carbon footprint associated with AI technologies.
Industry leaders are now recognizing the potential of distillation techniques to drive a new paradigm of AI development. The focus is shifting away from releasing the largest models possible towards a more sustainable and strategic approach. By privately deploying advanced models for internal use and knowledge transfer, companies can safeguard intellectual property and maintain a competitive advantage. At the same time, this strategy allows them to continue enhancing AI capabilities without the immediate public scrutiny or expectations that come with high-profile releases. This deliberate choice mirrors broader industry movements towards sustainable technological growth and the responsible management of AI capabilities.
Implications for AI Development
The development of GPT-5 and similar models highlights a strategic shift in the AI industry towards internal advancements rather than immediate public releases. Withholding GPT-5 allows companies like OpenAI to focus on internal "distillation" processes, creating more efficient models with reduced inference costs. This trend reflects a broader industry focus on efficiency and model optimization rather than simply scaling up in terms of raw size. Consequently, AI development is increasingly becoming a competitive endeavor, where strategic considerations may outweigh immediate commercialization. This approach ensures a strategic advantage in model refinement and resource allocation, preparing AI systems to address industry challenges in a financially sustainable way.
This shift towards private development and model distillation raises important considerations for AI transparency and public access. While tech enthusiasts may see strategic logic in refining AI models before public release, there is growing public concern that such practices could set a precedent for restricted access to cutting-edge AI capabilities. This unease is exacerbated by fears of power concentration within a few leading AI companies. Furthermore, societal implications loom large, as the benefits of advanced AI may remain limited to a few stakeholders unless efforts are made to ensure broader accessibility and transparency. As AI continues to evolve, balancing competitive advantage with public interest will be key in shaping the future of AI development.
Related Industry Events
The industry of artificial intelligence is buzzing with new events that directly tie into the ongoing development of language models like OpenAI's alleged GPT-5. Each event reflects a key strategic move in how AI technology is advancing, both in infrastructure and methodology.
Microsoft has recently announced an ambitious expansion of their Azure AI infrastructure, investing $5 billion in new data centers meant to bolster the training and deployment of large language models (LLMs). This investment highlights the industry's shift towards optimizing the scalability and performance of AI models, underlining the importance of dedicated infrastructure in the pursuit of advanced AI applications.
Learn to use AI like a Pro
Get the latest AI workflows to boost your productivity and business performance, delivered weekly by expert consultants. Enjoy step-by-step guides, weekly Q&A sessions, and full access to our AI workflow archive.
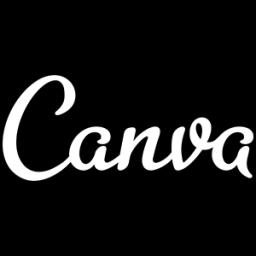
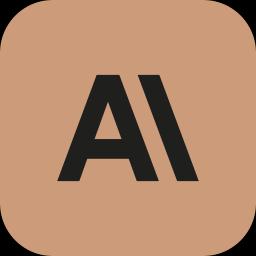
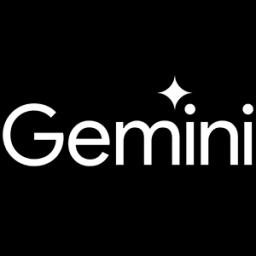
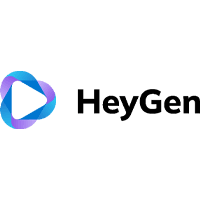
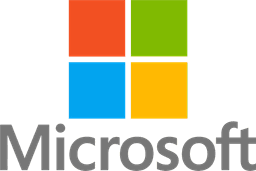
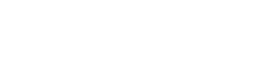
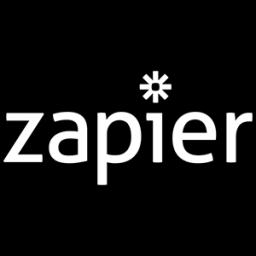
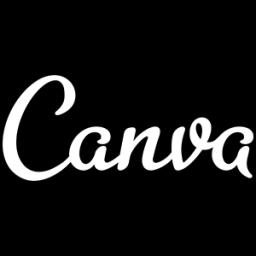
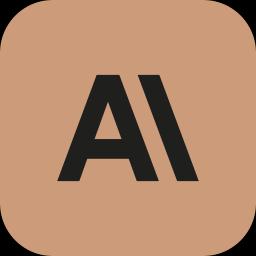
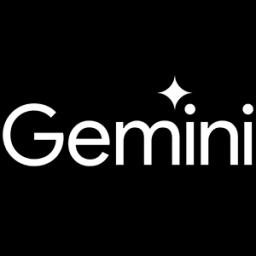
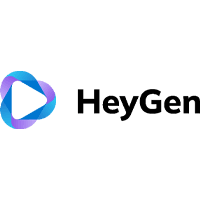
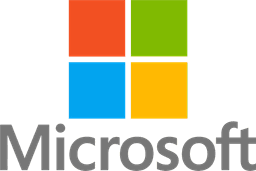
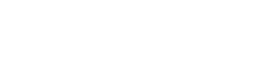
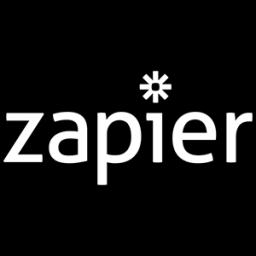
Complementing this move, Google DeepMind has revealed a breakthrough in model compression techniques, which drastically reduce the size of LLMs by up to 80% while retaining 95% of their original performance. This achievement underscores the growing demand for efficient model deployment, as it allows for the practical implementation of AI systems with less computational cost.
Adding to this, Anthropic is gearing up to release Claude 3.0, a language model that promises enhanced efficiency and reduced computational costs. This development signifies a broader industry trend, focusing on internal improvements and cost efficiency prior to public releases. Such strategies are becoming a staple as organizations look to leverage their AI advancements internally before unveiling them to the public.
Meta is also contributing to the sustainable deployment of AI, with its new training protocol that notably cuts energy consumption by 40%. This approach addresses the environmental concerns associated with AI, emphasizing the industry's responsibility to mitigate its ecological footprint while maintaining performance benchmarks in model training.
Each of these moves in the AI sector reflects a concerted effort to not only advance model capacities but to do so within a framework of efficiency, sustainability, and strategic resource allocation. Collectively, they highlight a shift towards more responsible AI development paradigms that prioritize long-term innovation over rapid commercialization.
Expert Opinions on GPT-5
AI experts offer valuable insights into the development and strategic deployment of GPT-5, a hypothetical model developed by OpenAI. Alberto Romero, an AI technology analyst, suggests that OpenAI may already have developed GPT-5 but is choosing to use it internally. He argues that the internal return on investment, especially in enhancing reasoning and agent technologies, outweighs the benefits of releasing it to the public. This internal strategic use aligns with OpenAI's efforts to create more efficient models through distillation processes, where larger models train smaller ones for better performance with reduced costs.
Dr. Dario Amodei, CEO of Anthropic and former VP of Research at OpenAI, points to significant obstacles in the development of GPT-5. He highlights that traditional scaling techniques may no longer be viable, indicating a need for breakthrough architectural innovations rather than merely expanding model sizes. This analysis aligns with the broader industry shift towards model efficiency and optimization over sheer scale.
Learn to use AI like a Pro
Get the latest AI workflows to boost your productivity and business performance, delivered weekly by expert consultants. Enjoy step-by-step guides, weekly Q&A sessions, and full access to our AI workflow archive.
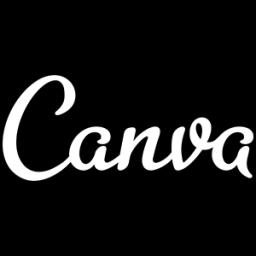
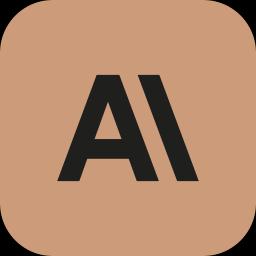
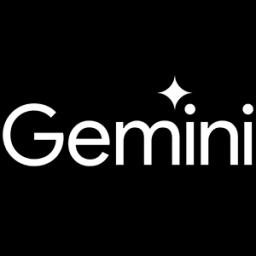
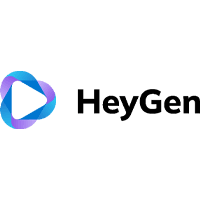
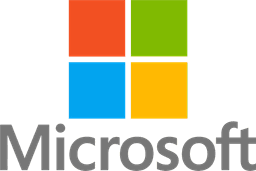
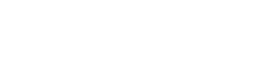
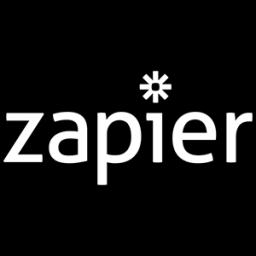
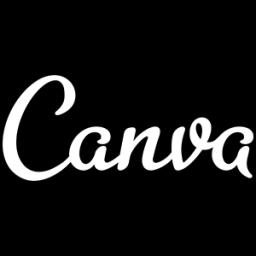
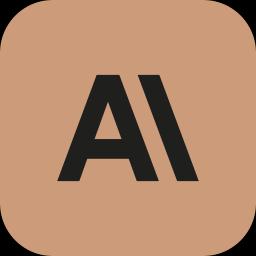
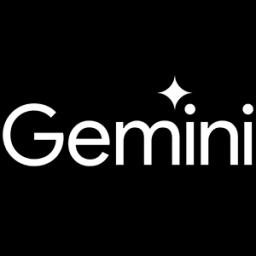
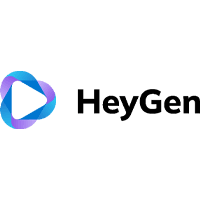
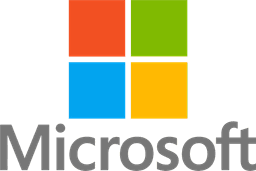
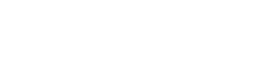
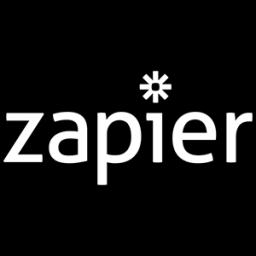
Gary Marcus, a researcher in AI and cognitive science, emphasizes the challenges presented by GPT-5's rumored delay. He notes that these delays underscore a broader industry challenge: the pursuit of reliable artificial general intelligence. According to Marcus, current language model designs might need a fundamental redesign to overcome limitations in reasoning and reliability.
These expert opinions underscore a strategic shift in AI development, focusing more on refining internal capabilities than immediate public releases. This tactic not only prevents potential disappointment due to modest improvements but also strategically positions companies like OpenAI to explore innovative model architectures without the constraints of external pressures or expectations.
Public Reactions to GPT-5
With the advent of GPT-5, public reactions have been mixed, reflecting both enthusiasm and concern. Tech enthusiasts are thrilled by the strategic use of GPT-5 for internal model distillation, applauding OpenAI's approach as a wise move. This group appreciates the focus on enhancing the efficiency and effectiveness of artificial intelligence models, rather than rushing toward a market release.
However, not all feedback is positive. Concerns over transparency have emerged as a significant theme among critics, who argue that withholding GPT-5 sets a precedent for restricted access to advanced AI technologies. These critics worry that such practices could limit public knowledge and cross-industry collaboration, potentially stifling innovation and competitive development processes.
On popular forums like Reddit and OpenAI's community discussions, users express frustration over perceived declines in model quality and responsiveness. Many users speculate that OpenAI's prioritization of internal development over enhancing user experience might have caused these issues, pointing out that the emphasis on strategic advantage could come at the expense of current users' needs.
Social media discussions have further intensified with debates centering around potential executive departures at OpenAI. Speculation about internal instability affecting development priorities has led to a surge of discussions, highlighting concerns about the stability and future direction of AI advancements within the organization.
Learn to use AI like a Pro
Get the latest AI workflows to boost your productivity and business performance, delivered weekly by expert consultants. Enjoy step-by-step guides, weekly Q&A sessions, and full access to our AI workflow archive.
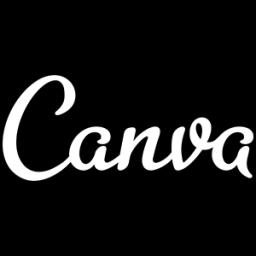
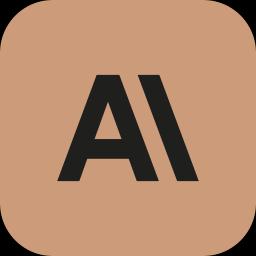
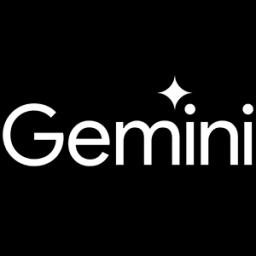
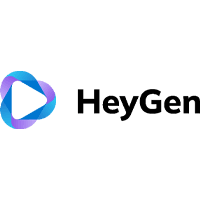
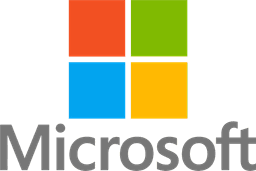
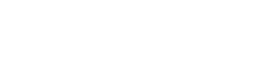
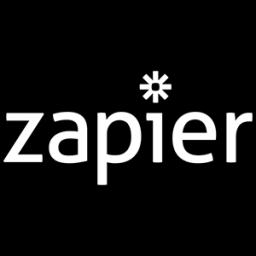
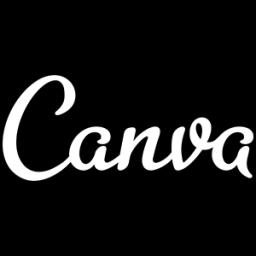
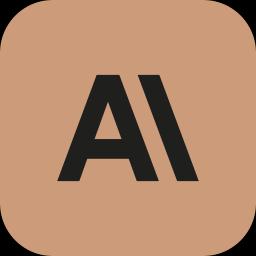
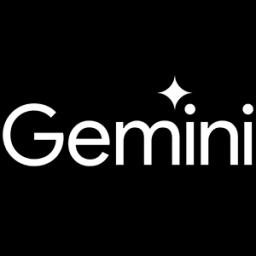
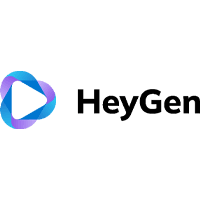
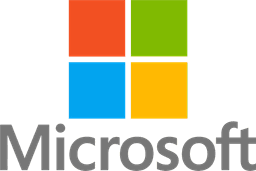
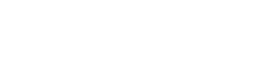
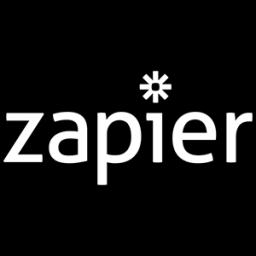
Despite these criticisms, many users recognize the high costs associated with deploying large-scale AI models like GPT-5 and support the idea of focusing on efficiency. This understanding reflects a pragmatic view that emphasizes economic viability and sustainability in advancing AI technologies. Nonetheless, the call for increased transparency remains strong, with public calls for OpenAI to share its developmental roadmap and align with community expectations.
Future Implications of AI Strategy
The strategic move by OpenAI to retain its latest development—GPT-5—confidentially suggests a remarkable transition in the overarching AI strategy landscape. As companies like OpenAI hold their AI advancements for internal use, they potentially redefine the trajectory of technological progress in artificial intelligence. By not making models like GPT-5 publicly available, OpenAI is prioritizing internal efficiency and refinement over widespread deployment. This signals a pivotal shift where the cutting-edge prowess of AI models could remain secluded within corporate frameworks to foster technological advancements and resource optimization.
Reflecting on how this change impacts industry dynamics reveals potential repercussions on competition and innovation. As industry leaders retain their breakthroughs for competitive gains, it might exaggerate disparities between large corporations and smaller entities unable to parallel the resource and financial investments. This encapsulates a forward-looking view where AI progress becomes a game of strategic improvements and efficiency rather than just enlarging models. Such trends reflect broader market consolidation over time due to rising costs associated with training and deploying expansive models.
The approach of withholding significant developments like GPT-5 aligns with broader industry movements towards efficiency and environmental sustainability. Companies are progressively adopting energy-efficient model training, curbing the environmental footprint of AI proliferation. This evolution towards sustainable AI practices will not only address ecological concerns but could also prompt regulatory scrutiny emphasizing accountability and transparency in AI development.
Furthermore, by concentrating on model distillation and energy efficiency, OpenAI and similar organizations are paving pathways for accessible AI deployment through smaller, efficient models. This evolution fosters a nuanced democratization of AI technology, despite limited access to the most advanced versions. While advancing this internal efficiency agenda, AI's role and impact on employment will morph, with job roles shifting from traditional AI development to optimization-focused positions.
Moreover, with leading entities holding onto their advanced developments, academia might experience slower innovation due to reduced open exchanges of advanced methodologies. This proprietary concentration could bottleneck innovation traditionally driven by academic symbiosis, leading to a landscape where AI milestones are benchmarked through optimization and efficiency rather than pure developmental scope. This shift underscores a transformative phase in AI strategy highlighting competitive advantage through technological and operational efficiency.
Learn to use AI like a Pro
Get the latest AI workflows to boost your productivity and business performance, delivered weekly by expert consultants. Enjoy step-by-step guides, weekly Q&A sessions, and full access to our AI workflow archive.
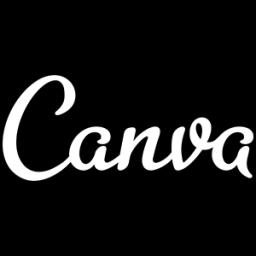
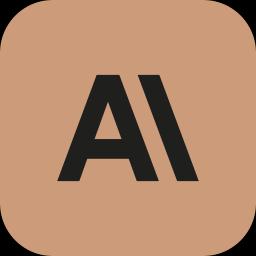
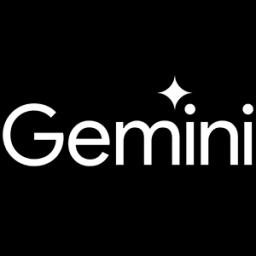
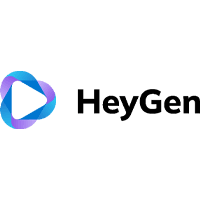
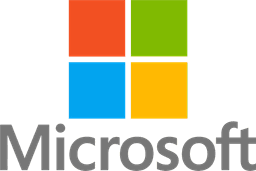
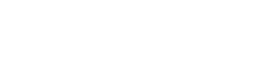
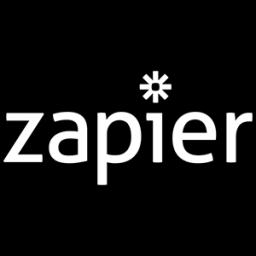
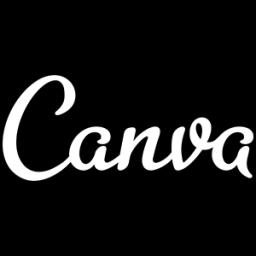
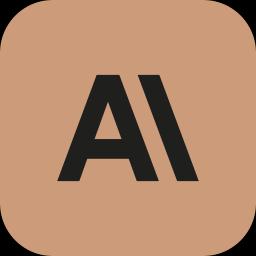
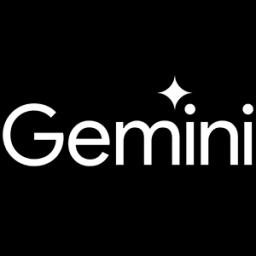
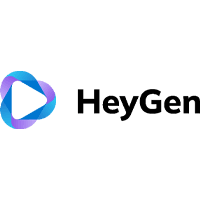
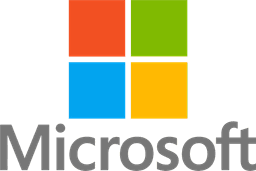
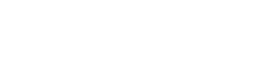
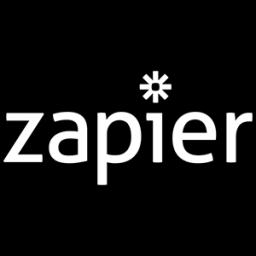
Conclusion
In conclusion, the ongoing conversation about OpenAI's GPT-5 development highlights significant shifts in the AI landscape. With OpenAI likely prioritizing internal use for strategic model distillation over public deployment, the future of AI development may see a trend towards more closed-door advancements. This approach allows organizations like OpenAI to focus on internal efficiencies and tackle rising inference costs, without the immediate pressures of a public release.
The implications of this strategy are profound, indicating a possible industry shift where major AI advancements remain proprietary to maintain competitive advantage. As a result, smaller competitors may find it challenging to keep up with industry leaders, who can invest heavily in infrastructure and innovation. This could lead to further consolidation within the AI sector, where resources and capabilities become concentrated among a few dominant players.
Moreover, the focus on efficiency and internal improvements rather than sheer model size is a pragmatic approach given current trends in AI development. Techniques such as model distillation and energy-efficient training protocols are increasingly becoming central to addressing cost and sustainability challenges, potentially making AI more accessible in the long run through smaller, cost-effective models.
Public sentiment appears divided, with some praising the strategic logic behind OpenAI's apparent restraint while others voice concerns about transparency and access. As advanced AI capabilities remain behind closed doors, calls for greater transparency and accountability in AI development are likely to intensify, potentially ushering in new regulatory measures.
Ultimately, the case of GPT-5 serves as a microcosm of broader industrial shifts where strategic, efficient, and private development may take precedence over immediate public deployment. This strategy not only ensures sustainability but also secures a technological edge that might redefine how AI advancements are pursued, measured, and valued in the coming years.