The Battle for Developer Hearts Continues
OpenAI's Stronghold Faces a Rising Tide: The LLM Landscape Shift
Last updated:

Edited By
Mackenzie Ferguson
AI Tools Researcher & Implementation Consultant
OpenAI's large language models maintain an 87% usage rate among developers, but the LLM market is rapidly evolving. With 60% of developers switching vendors in the last six months and using multiple providers, alternatives like DeepSeek are gaining traction. DeepSeek now boasts a 29% utilization rate, as Microsoft struggles at 10%. The focus is shifting towards practical AI use cases, cost control, and AI integration in customer-facing applications. Developers are favoring retrieval-augmented generation (RAG) and vector databases over model training.
Introduction
The domain of large language models (LLMs) is experiencing a pivotal shift, as technologies advance and developer behavior evolves rapidly. According to a recent analysis, OpenAI maintains a notable dominance with an 87% usage rate among developers. However, this landscape is far from static. A significant 60% of developers have switched LLM vendors in the last six months, a clear indicator of the dynamic nature of this market [1]. This fluctuation stems from developers actively seeking solutions that align more closely with their specific needs, whether in terms of performance, cost, or unique features not yet addressed by current general-purpose LLMs.
One emerging trend highlighted in the industry is the growing prominence of alternative providers such as DeepSeek. Garnering a 29% adoption rate among businesses, DeepSeek is poised to challenge established leaders like OpenAI [1]. This shift illustrates a broader movement towards diversification in LLM usage, driven by a demand for models that offer specialized capabilities. At the same time, Microsoft's LLMs have been slower to capture wide industry attention, representing just 10% of usage, suggesting potential avenues for improvement and innovation in their offerings.
Learn to use AI like a Pro
Get the latest AI workflows to boost your productivity and business performance, delivered weekly by expert consultants. Enjoy step-by-step guides, weekly Q&A sessions, and full access to our AI workflow archive.
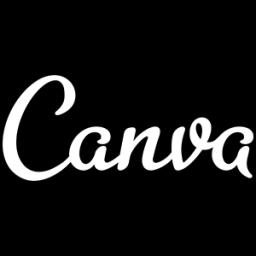
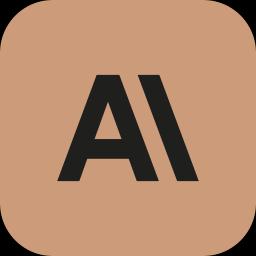
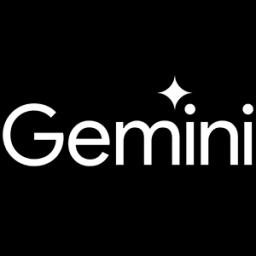
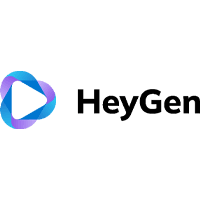
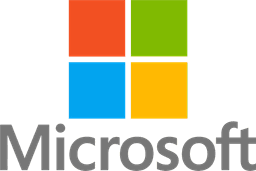
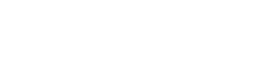
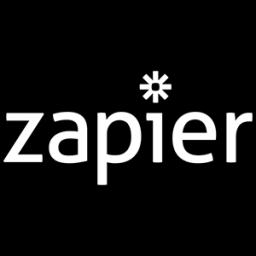
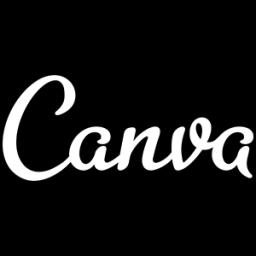
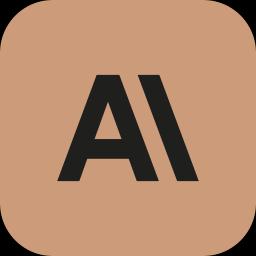
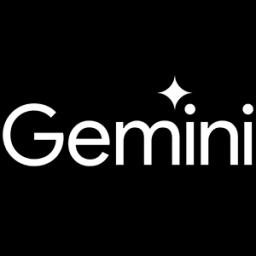
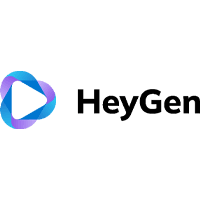
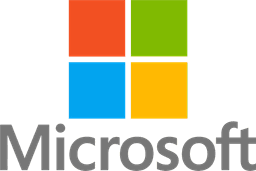
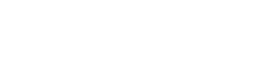
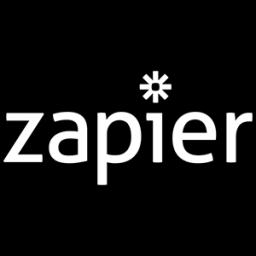
The changing landscape of LLMs also reflects broader trends in AI deployment practices. There is a marked shift away from maintaining large, formal AI teams. Instead, organizations are focusing more on integrating AI seamlessly into their existing workflows, especially within customer-facing applications [1]. This shift is largely motivated by the need to manage costs effectively while maximizing the practical applications of AI technologies.
Interestingly, industry experts have noted a departure from the initial "overhype" surrounding AI technologies. The current focus is more pragmatic, emphasizing the adoption of retrieval-augmented generation (RAG) techniques and vector databases over traditional model training approaches. These tools and techniques provide tangible benefits, including efficiency and cost reduction, which are increasingly valued by businesses looking for solid returns on their technology investments [1].
Public reactions to these shifts in the LLM landscape are varied. While OpenAI’s prevailing influence is acknowledged, the active pursuit of alternatives by a substantial segment of developers signals a cautious approach towards total reliance on a single provider. Enthusiasm for new competitors like DeepSeek is reflected in its growing adoption, which is attributed to its perceived ability to meet demands that others have overlooked [1]. Similarly, the emphasis on implementing practical and cost-effective AI solutions speaks volumes about current market expectations and the pressures faced by businesses today.
OpenAI's Dominance in the LLM Market
OpenAI continues to dominate the large language model (LLM) market, with an impressive 87% usage rate among developers. This dominance is a testament to the effectiveness and appeal of OpenAI's offerings, which are frequently chosen for their robust capabilities and reliable performance. However, the landscape is shifting as new competitors emerge and developers seek varied solutions that cater to specific needs and use cases. The market is seeing a push towards diversification, with 60% of developers switching vendors in the past six months according to a recent report.
Learn to use AI like a Pro
Get the latest AI workflows to boost your productivity and business performance, delivered weekly by expert consultants. Enjoy step-by-step guides, weekly Q&A sessions, and full access to our AI workflow archive.
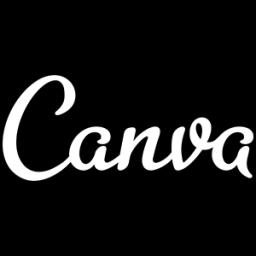
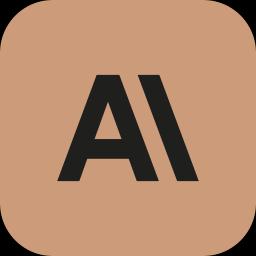
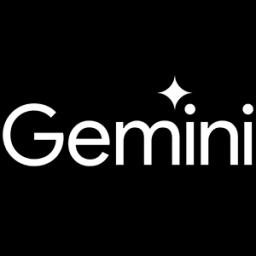
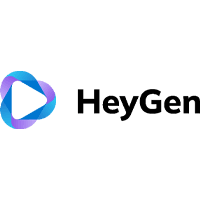
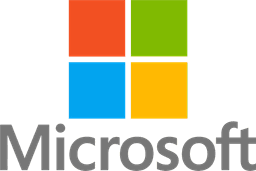
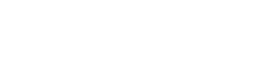
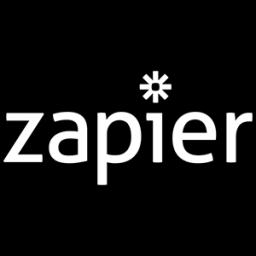
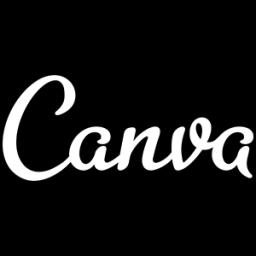
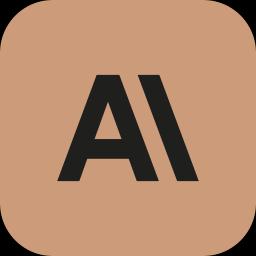
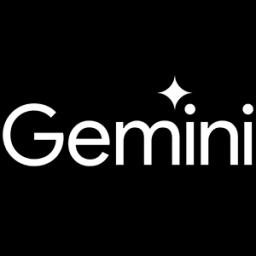
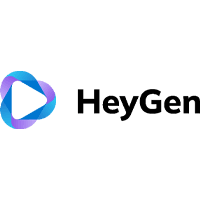
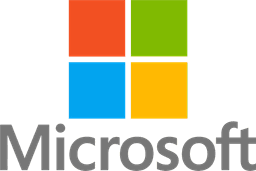
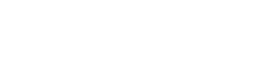
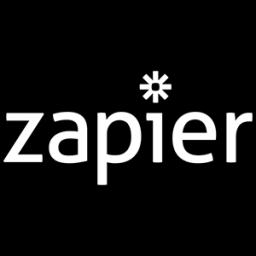
This report indicates that while OpenAI's position is strong, it's not unassailable. Developers are now utilizing an average of two different providers, looking beyond OpenAI for competitive pricing, distinct features, and specialized capabilities. Among the new contenders is DeepSeek, which has rapidly gained a 29% business utilization rate, suggesting its value proposition resonates with companies seeking alternatives to OpenAI for specific tasks. Microsoft’s lagging LLMs, despite the company's established reputation, indicate a challenging environment for even well-known players as they attempt to secure a greater share of this evolving market. This dynamic is captured in the competitive nature of the sector, as developers remain open to exploring and adopting emerging LLM technologies.
The Rise of Competitors: DeepSeek and Beyond
The competitive landscape of large language models (LLMs) is witnessing a remarkable shift, with OpenAI's once unchallenged dominance now facing credible threats from emerging rivals. The rise of DeepSeek as a significant player in the AI sector is noteworthy, with its business adoption rate already reaching 29% . This shift underscores a broader trend where developers are increasingly exploring diverse LLM providers to find solutions tailored for specific needs, such as performance enhancements, cost reductions, and unique capabilities that aren't addressed by standard offerings.
Developers, who once predominantly relied on OpenAI's offerings—with an impressive 87% usage rate—are now diversifying their approach by frequently switching between vendors . The churn, described as 60% within recent months, illustrates a dynamic market where innovation and specialization are valued. DeepSeek's ascent is emblematic of this trend, often attracting developers with its balanced mix of affordability, strong performance metrics, and features fine-tuned to niche requirements.
The impact of DeepSeek extends beyond mere statistics, as it reflects a broader movement in the AI industry towards more pragmatic applications. Businesses are increasingly turning to alternative providers like DeepSeek due to dissatisfaction with the overhyped promises of AI . This shift is shaped by the need for practical, real-world applications and the integration of AI capabilities into customer-facing products, a strategy that emphasizes tangible business benefits and return on investment.
As DeepSeek and similar entrants position themselves as formidable competitors, the market is becoming more competitive, leading to enhanced innovation and potentially more favorable pricing for consumers and businesses alike. This has fostered an environment where retrieval-augmented generation (RAG) and vector databases are preferred over traditional model training, aligning with the industry's push towards efficiency and cost-effectiveness . These developments indicate that while OpenAI continues to lead, the presence of vigorous competition like DeepSeek is catalyzing a transformative period in the LLM domain.
Frequent Switching Behavior Among Developers
Understanding the behavior of developers in frequently switching between large language model (LLM) vendors requires an examination of the current technological landscape and market dynamics. Developers often find themselves in a rapidly evolving ecosystem, driven by the emergence of diverse LLM providers, each offering unique features and capabilities. The demand for specialized solutions that can cater to specific use cases is paramount, compelling developers to explore different vendors. This exploration is propelled by the pursuit of optimized performance, cost-effectiveness, and access to advanced functionalities that meet project-specific needs.
Learn to use AI like a Pro
Get the latest AI workflows to boost your productivity and business performance, delivered weekly by expert consultants. Enjoy step-by-step guides, weekly Q&A sessions, and full access to our AI workflow archive.
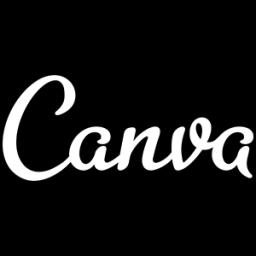
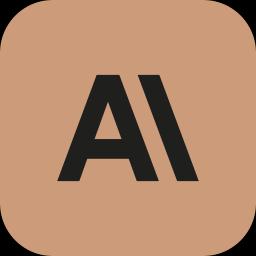
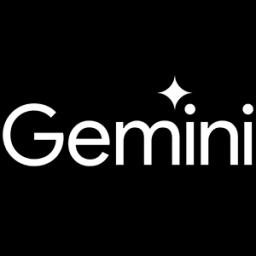
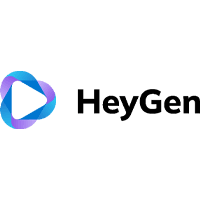
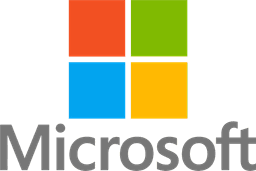
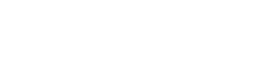
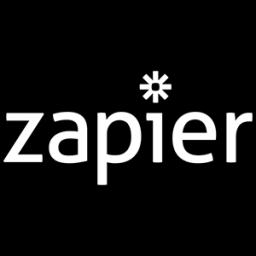
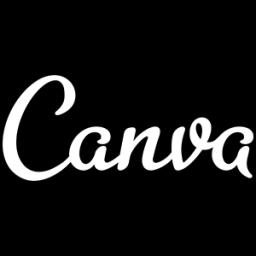
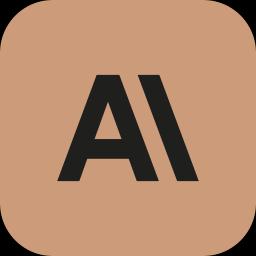
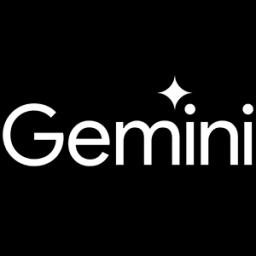
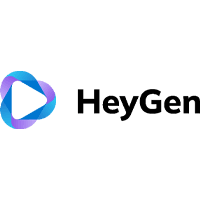
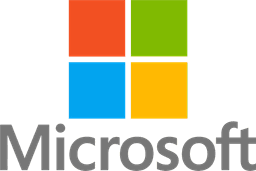
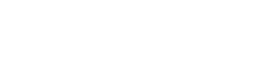
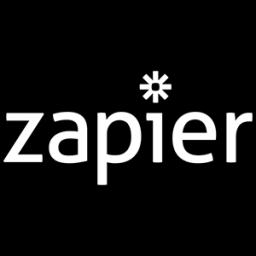
Moreover, the fluid nature of technological advancements in the LLM field encourages developers to experiment with multiple platforms to stay ahead of the curve. Companies such as DeepSeek, with their growing popularity, highlight this dynamic with their increasing business utilization rate of 29% . Meanwhile, traditional giants like Microsoft struggle at a mere 10%, indicating a shift in preferences towards agile and innovative solutions. Such transitions underscore a broader trend of decentralization within the developer community, highlighting a move away from dependency on singular, possibly oversubscribed, platforms like OpenAI.
The frequent switching behavior is also linked to concerns surrounding the 'overhype' that currently permeates the AI industry. Developers are becoming more discerning, often valuing pragmatic and practical applications over experimental and risky endeavors. This trend reflects a growing emphasis on projects that promise clear return on investment, with developers favoring readily deployable AI features over custom model training. As a result, the integration of AI is becoming more aligned with practical business needs, enhancing productivity and ensuring cost-effectiveness, especially as companies move away from formal AI teams to more nimble approaches.
Furthermore, cost management remains a critical factor in the decision-making process. The adoption of retrieval-augmented generation (RAG) and vector databases, over more in-depth model training, illustrates a strategic shift towards tools that are not only cost-efficient but also enhance AI integration into customer-facing applications. This strategy aligns with market demands for seamless and user-friendly interfaces that improve end-user experience. Consequently, developers are empowered to innovate without the financial burden traditionally associated with AI infrastructure.
In conclusion, frequent switching among LLM vendors by developers is not merely a reflection of technological advancement but also a strategic, economic, and experiential phenomenon. The ability to choose from a variety of LLMs allows developers to tailor their tech stack according to specific business and project requirements, ensuring they remain competitive in a fast-paced digital environment. This behavior underscores a significant shift towards a more modular, responsive, and collaborative approach to technology deployment in the AI sector.
Integration of AI into Customer-facing Applications
The integration of artificial intelligence (AI) into customer-facing applications represents a significant shift in how businesses operate and interact with their consumers. As organizations strive to enhance user experiences, AI technologies offer the ability to deliver personalized service, faster response times, and greater efficiency. For instance, leveraging AI in applications enables real-time data processing and personalized recommendations, which can significantly enhance customer satisfaction and loyalty. This capability is increasingly important in a competitive marketplace where differentiation through superior customer service can be pivotal. Moreover, the evolution of retrieval-augmented generation (RAG) and vector databases facilitates the seamless integration of AI, making it possible for companies to harness complex data systems efficiently [1].
In this rapidly evolving AI landscape, the demand for specialized language learning models (LLMs) is rising as businesses seek tailored solutions that meet their unique requirements. OpenAI has been at the forefront, dominating the developer market with their LLMs; however, the trend is shifting as more developers experiment with alternative providers. According to a recent report, 60% of developers have switched LLM vendors in the past six months, underscoring the dynamic nature of the field [1]. New contenders like DeepSeek are emerging, offering competitive business solutions with a 29% adoption rate [1]. These developments highlight the importance of adaptability and the need for platforms that provide not only advanced AI capabilities but also cost-effectiveness and industry-specific features.
Learn to use AI like a Pro
Get the latest AI workflows to boost your productivity and business performance, delivered weekly by expert consultants. Enjoy step-by-step guides, weekly Q&A sessions, and full access to our AI workflow archive.
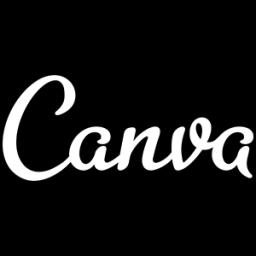
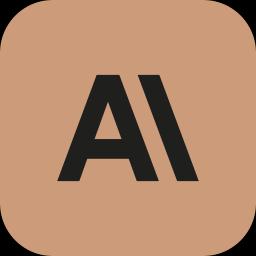
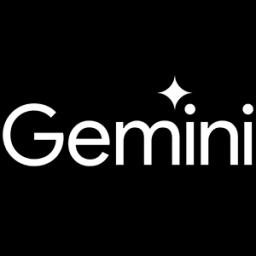
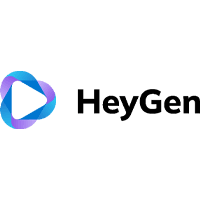
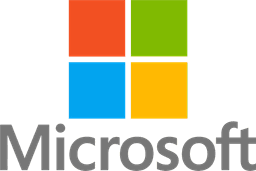
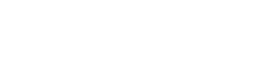
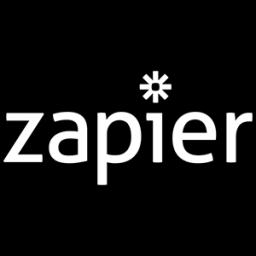
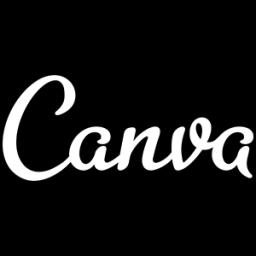
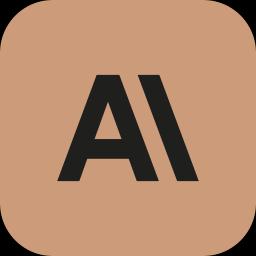
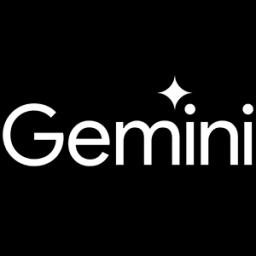
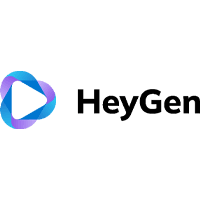
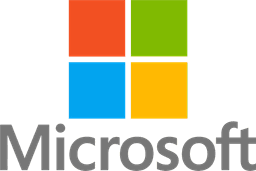
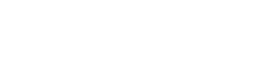
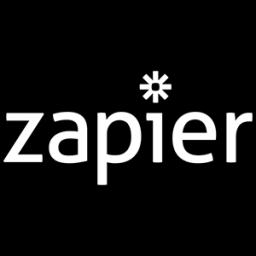
The move towards integrating AI into customer-facing applications also reflects a pragmatic shift in AI adoption strategies. Companies are moving away from investing in large AI teams to focusing on practical use cases that directly impact the bottom line. This approach is driven by the need to manage costs effectively while maximizing the return on investment in AI technologies. For example, instead of developing new AI models from scratch, many organizations prefer to integrate existing solutions, which are readily available and proven effective in delivering results. This strategy aligns with the growing preference for using AI tools that can seamlessly fit into existing business frameworks, enhancing productivity without the need for extensive infrastructure overhaul [1].
As developers continue to explore diverse AI solutions, the market is witnessing a diversification in the types of AI technologies being deployed. This includes a shift towards more flexible and scalable AI solutions that can be easily customized for different applications. In customer-facing scenarios, this flexibility allows businesses to offer unique and innovative experiences that cater to specific consumer needs. Moreover, open-source AI solutions are gaining traction, enabling businesses to fine-tune applications without the constraints of proprietary models. This trend not only fosters innovation but also democratizes AI, making advanced technologies accessible to smaller enterprises and individual developers [1].
The Shift from AI Teams to Practical Use Cases
The landscape of AI is undergoing a significant transformation as organizations shift their focus from building centralized AI teams to integrating practical use cases across various sectors. This transition is largely driven by the realization that the potential of AI extends beyond theoretical frameworks and isolated models, requiring a more hands-on, utilitarian approach. The current focus is on tangible applications that provide measurable value, both in terms of efficiency and cost-effectiveness [1](https://www.techradar.com/pro/openai-continues-to-dominate-ai-llm-among-developers-a-report-finds-but-things-are-changing-fast).
Among the factors contributing to this shift is the increasing demand for AI models that can be seamlessly embedded into existing business processes. Many companies now prefer tools like retrieval-augmented generation and vector databases, which offer immediate usability over traditional model training techniques. This preference highlights a broader trend where businesses are looking for ways to leverage AI without excessive overhead, thus avoiding the burden of maintaining large, specialized teams [1](https://www.techradar.com/pro/openai-continues-to-dominate-ai-llm-among-developers-a-report-finds-but-things-are-changing-fast).
Moreover, the perception of AI being 'overhyped' has led to a more discerning approach towards its deployment. Organizations are increasingly focusing on clear use cases that can deliver direct returns on investment, shedding the inflated expectations that once hovered over AI projects [1](https://www.techradar.com/pro/openai-continues-to-dominate-ai-llm-among-developers-a-report-finds-but-things-are-changing-fast). This pragmatic shift towards integrating AI into customer-facing applications not only helps in enhancing user experiences but also in driving business growth through innovative solutions.
In essence, the movement away from traditional AI teams emphasizes agility and adaptability in AI project implementation. As businesses navigate an ever-evolving technological landscape, the prioritization of practical AI applications underscores the need for sustainable, scalable, and efficient solutions. This focus on real-world utility signifies a profound transformation in how companies perceive and utilize AI, aligning innovation with strategic business goals.
Learn to use AI like a Pro
Get the latest AI workflows to boost your productivity and business performance, delivered weekly by expert consultants. Enjoy step-by-step guides, weekly Q&A sessions, and full access to our AI workflow archive.
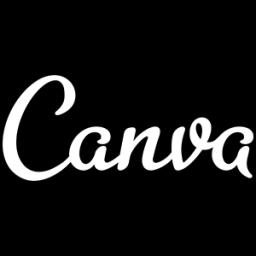
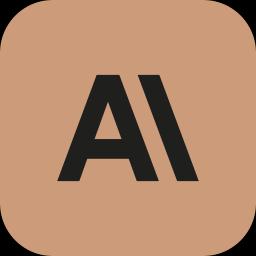
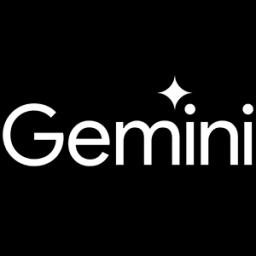
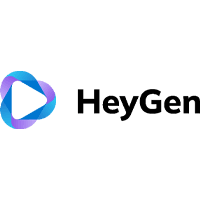
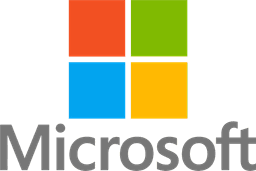
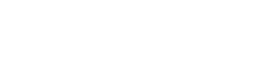
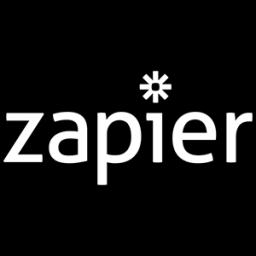
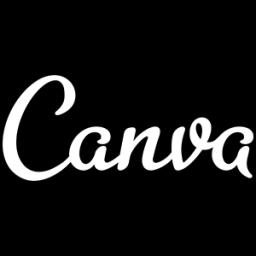
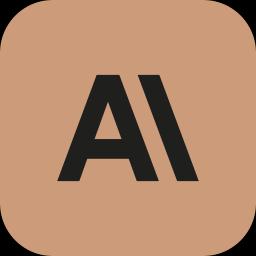
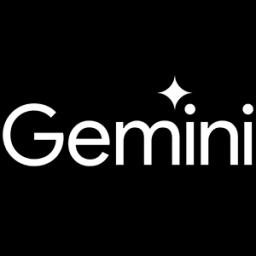
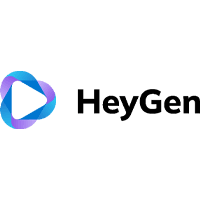
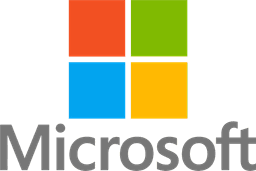
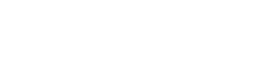
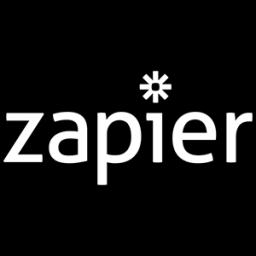
Expert Opinions on the Changing LLM Landscape
The landscape of large language models (LLMs) is undergoing a profound transformation, sparked by the dominance of OpenAI and the subsequent diversification by developers. According to recent insights, OpenAI's models are still widely used, commanding an impressive 87% market share among developers. However, there's a noteworthy shift as 60% of developers have switched vendors in the past six months, often exploring partnerships with at least two providers. This shift highlights a burgeoning need for specialized LLMs that cater to specific tasks or industry requirements, an indicator of the market's evolving dynamics (TechRadar).
The rise of competitors like DeepSeek signifies a diversification in choices available to developers, challenging OpenAI's previously unchallenged position. With DeepSeek's impressive 29% business utilization rate, it has emerged as a substantial player in the LLM market. This is significant in an industry where performance metrics, cost control, and the integration of advanced features are paramount. As developers navigate this competitive environment, their choices are often influenced by the particular needs of their projects, which require flexibility and adaptability from their LLM solutions (TechRadar).
An underlying theme in the changing LLM landscape is the move away from traditional AI teams toward more pragmatic applications of AI. This transition reflects a keen emphasis on practical use cases that yield tangible benefits and return on investment. The preference for retrieval-augmented generation (RAG) and vector databases over traditional model training underscores a shift towards using existing technologies to develop AI solutions. This strategy not only reduces costs but also accelerates deployment times, enabling faster integration of AI features into customer-facing applications (TechRadar).
Despite concerns about the 'overhype' of AI, there remains robust confidence in its potential. Developers are increasingly focusing on AI integration that enhances customer experience and operational efficiency. With the market's current emphasis on cost-effective solutions and clear AI application cases, we are witnessing a concerted effort to balance innovation with practicality. Navigating these changes will require ongoing adaptation from both developers and businesses alike, ensuring that AI's real-world impact is both meaningful and sustainable (TechRadar).
Public Reactions and Sentiments
The current state of the large language model (LLM) market showcases varying public reactions, as both excitement and skepticism surround the advancements in AI technologies. OpenAI has long been a dominant force, with an 87% usage rate among developers, thanks to their innovative and powerful models. However, these developers are increasingly exploring alternative options, as evidenced by the 60% who have switched vendors in the past six months, a shift driven partly by the evolving landscape and options available [source]. This trend is indicative of a broader sentiment that values diversity in choice and adaptability to specific needs, reflecting a pragmatic approach to harnessing AI technology.
Among the alternatives gaining traction is DeepSeek, which has carved out a niche with a 29% adoption rate for business applications. Public perception views DeepSeek as a capable contender to OpenAI, offering performance and features that some developers find more tailored to their specific use cases [source]. The praise for DeepSeek reflects a general openness to embracing new players that bring innovation and customization, factors that are highly valued in the competitive AI arena.
Learn to use AI like a Pro
Get the latest AI workflows to boost your productivity and business performance, delivered weekly by expert consultants. Enjoy step-by-step guides, weekly Q&A sessions, and full access to our AI workflow archive.
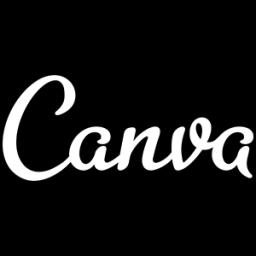
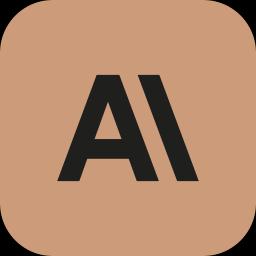
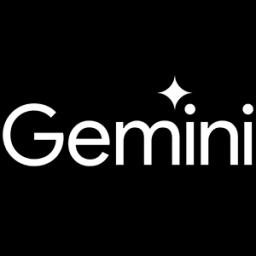
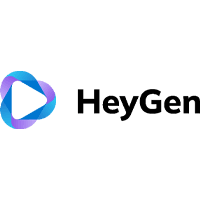
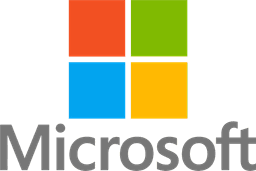
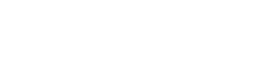
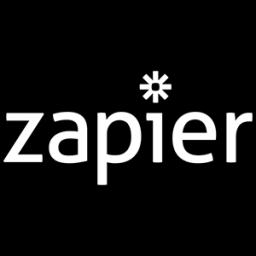
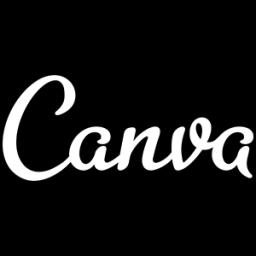
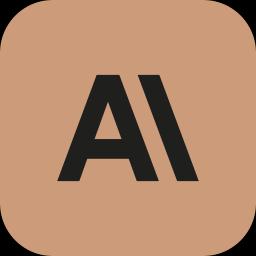
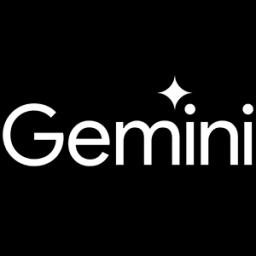
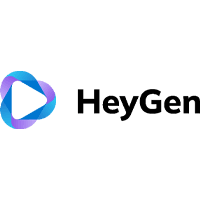
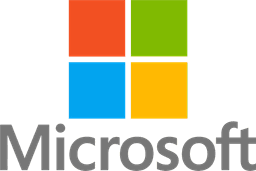
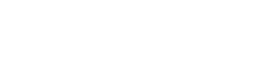
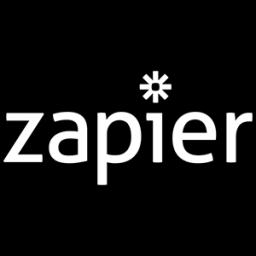
Despite the advancements and positive reception of diverse LLMs, some sectors of the public voice concerns over the "overhype" of AI technologies. Questions about the practicality and realistic applications of AI persist, particularly as companies seek cost-effective methods to integrate AI without bloating their operations [source]. This skepticism is leading to a reevaluation of AI’s role, encouraging businesses to focus on tools that promise tangible returns and seamless integration.
Programmers, in particular, exhibit a mix of enthusiasm and wariness as LLMs continue to transform their industry. Many see opportunities in AI to enhance their work and create innovative solutions. However, there's a wariness about job displacement and the potential ethical challenges posed by these technologies. Such concerns contribute to the nuanced public sentiment where optimism about AI's future is tempered by the need for responsible and equitable deployment [source].
Economic Impacts of a Competitive LLM Market
The competitive landscape of Large Language Models (LLMs) is redefining economic parameters in the tech industry. OpenAI, currently leading with an 87% usage rate among developers, faces intense competition as the market diversifies its options. This shift is catalyzed by developers' pursuit of LLMs that offer superior performance, cost-effectiveness, and tailored functionalities. For instance, DeepSeek, now utilized by 29% of businesses, embodies this trend by presenting itself as a viable alternative to OpenAI's offerings, thanks to its competitive pricing and the specialized features it provides [].
This increased competition is stimulating innovation across the board. With developers frequently switching between providers—60% having done so within the past six months—there is a clear impetus for LLM vendors to continuously enhance their models, pushing boundaries in efficiency and specialization []. Such a vibrant market landscape is expected to drive down costs for LLM services, thus encouraging broader adoption among businesses and creating an environment where small and medium enterprises can compete more effectively.
As the demand for specialized LLMs grows, investment patterns in AI technology are shifting. Companies are increasingly focusing on integrating AI within existing workflows, emphasizing practical, result-oriented applications over speculative AI-centric projects. The move away from large, dedicated AI teams towards the utilization of retrieval-augmented generation (RAG) and vector databases highlights a strategic realignment towards tools that offer immediate utility and ROI, contributing to a more efficient allocation of resources [].
The economic implications of these trends are considerable. The competitive dynamics are likely to foster an era of rapid technological advancement, benefiting consumers through enhanced services and lower costs. Additionally, this environment encourages the democratization of AI technology, allowing more businesses and developers to access and leverage sophisticated AI solutions. This democratization supports innovation across various sectors, driving economic growth and contributing to a more inclusive digital economy [].
Learn to use AI like a Pro
Get the latest AI workflows to boost your productivity and business performance, delivered weekly by expert consultants. Enjoy step-by-step guides, weekly Q&A sessions, and full access to our AI workflow archive.
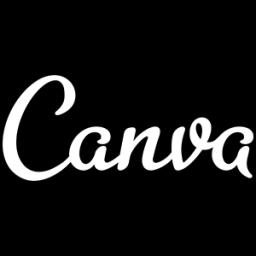
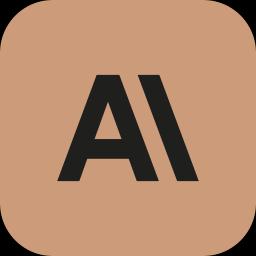
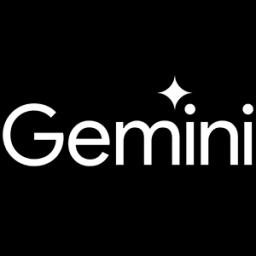
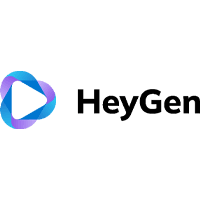
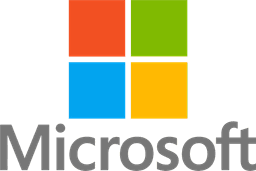
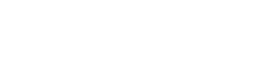
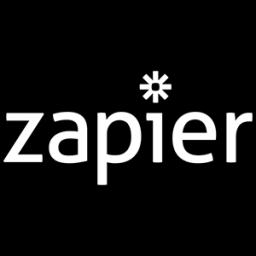
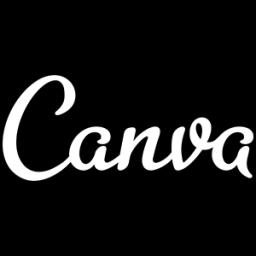
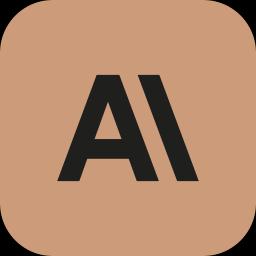
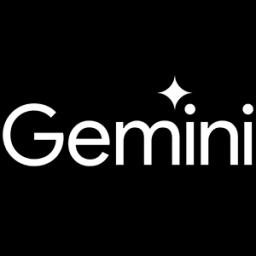
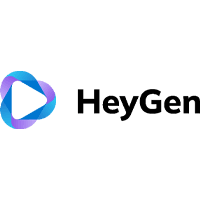
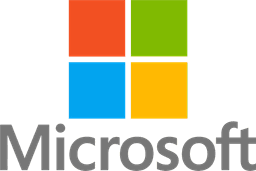
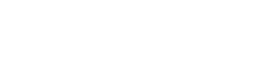
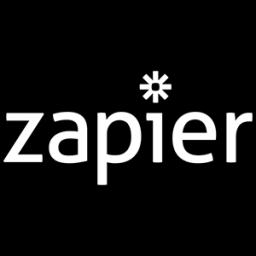
Social Changes Driven by AI Integration
The integration of artificial intelligence (AI) into various sectors is bringing about profound social changes, reshaping how individuals and industries operate in everyday life. As AI systems, such as large language models (LLMs), become more prevalent, businesses and developers are rapidly adapting to new technologies. This shift is driven in part by the accessibility of AI tools that simplify complex tasks and improve efficiency. The widespread use of AI, such as OpenAI's language models, is compelling companies to reconsider traditional structures and roles to incorporate AI-driven solutions effectively. For instance, there is an increasing trend towards integrating AI features into customer-facing applications, enhancing user experiences and optimizing service delivery. This cultural shift is evidenced by the growing percentage of developers using an average of two AI providers, fostering a diversified approach to technology integration [1](https://www.techradar.com/pro/openai-continues-to-dominate-ai-llm-among-developers-a-report-finds-but-things-are-changing-fast).
In the realm of employment and job dynamics, AI's integration is playing a pivotal role in reshaping the labor market. While concerns about automation and job displacement persist, AI is also creating new roles and opportunities that require specialized skills in AI management, development, and integration. This necessitates a workforce that is adaptable and skilled in navigating the nuances of AI technologies. Furthermore, as AI democratizes through open-source models, more individuals and smaller enterprises can harness AI's potential without depending solely on large tech conglomerates. This democratization is poised to unlock a plethora of innovations catering to localized needs and niche markets. Meanwhile, the trend of focusing on practical AI applications is leading organizations to prioritize tools and solutions that offer clear returns on investment, thus fostering a more grounded and cost-effective adoption of AI [1](https://www.techradar.com/pro/openai-continues-to-dominate-ai-llm-among-developers-a-report-finds-but-things-are-changing-fast).
The societal impacts of AI's integration are also visible in the changing expectations and interactions between technology and users. With AI systems increasingly embedded in tools that consumers use daily, such as apps and online services, there is a noticeable shift towards more personalized and efficient service delivery. This integration supports enhanced productivity and offers consumers more tailored experiences, whether in customer service, product development, or personalized marketing strategies. Additionally, societal perceptions are shifting as people become more accustomed to AI's presence in their everyday environment, sparking dialogues about the ethics of AI and the need for responsible implementation. As AI becomes a staple in modern society, it prompts critical reflections on privacy, bias, and decision-making dynamics in digital interactions [1](https://www.techradar.com/pro/openai-continues-to-dominate-ai-llm-among-developers-a-report-finds-but-things-are-changing-fast).
Political and Regulatory Challenges
The rise of Large Language Models (LLMs) like OpenAI's has brought about significant political and regulatory challenges. Governments across the globe are grappling with the need to regulate these technologies effectively. The primary concern lies in balancing innovation with safety, ensuring LLMs do not perpetuate bias or misinformation. Policy makers are faced with the task of creating frameworks that protect public interests while encouraging technological advancement. This delicate balance is crucial as countries compete in the AI race, aiming to secure a foothold in the burgeoning AI-driven economy. Creating and enforcing regulations that are both effective and adaptable to the rapid advancements in AI technology is an ongoing challenge.
Geopolitical tension is another layer of complexity in the evolving LLM sector. As nations invest heavily in artificial intelligence, there is a notable shift towards securing technological supremacy. This competition not only involves countries like the U.S. and China but also tech giants racing to dominate the market. Consequently, this environment poses risks of intellectual property disputes and political conflicts. The geopolitical landscape is further intensified by policies aimed at protecting domestic industries while maintaining open channels for international AI collaboration. The outcomes of these strategies will likely have profound implications on global AI innovation and economic dynamics.
Ethical considerations also play a critical role in shaping the political and regulatory framework for LLMs. Issues of fairness, transparency, and accountability in AI deployment are at the forefront of regulatory discussions. Researchers and policymakers are compelled to develop robust guidelines that ensure ethical AI practices without stifling innovation. As AI technologies become more integrated into societal functions, public trust hinges on transparent operations and the ability to hold AI systems and their developers accountable. Thus, addressing these ethical concerns is paramount to fostering widespread acceptance and utilization of AI technologies.
Learn to use AI like a Pro
Get the latest AI workflows to boost your productivity and business performance, delivered weekly by expert consultants. Enjoy step-by-step guides, weekly Q&A sessions, and full access to our AI workflow archive.
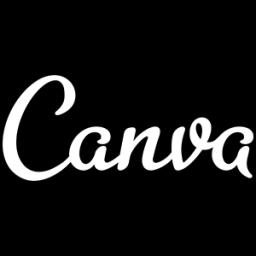
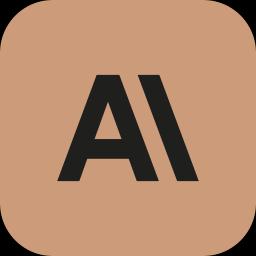
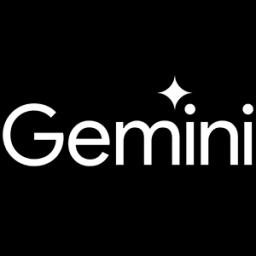
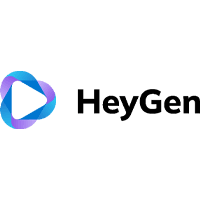
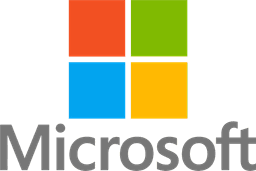
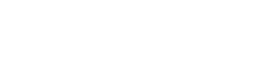
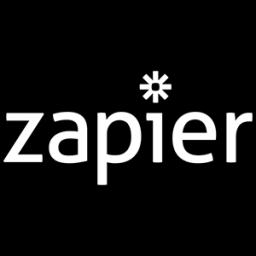
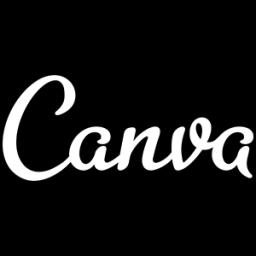
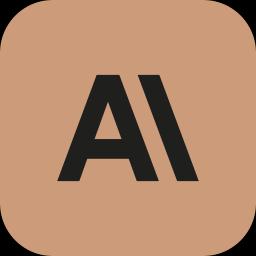
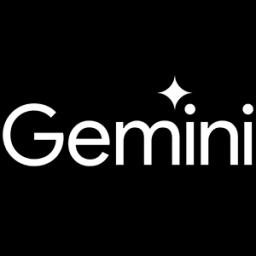
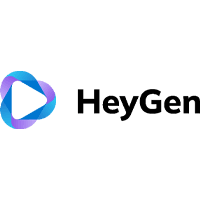
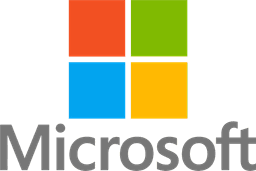
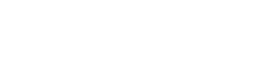
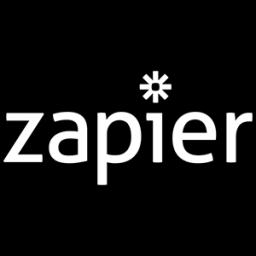
Future Implications and Trends
The rapid evolution of the large language model (LLM) landscape underscores a significant transformation in how businesses and developers engage with artificial intelligence. As OpenAI continues to dominate with its high usage rate, the winds of change bring about noteworthy competitors like DeepSeek, which are gaining traction rapidly. According to a report, 60% of developers have switched vendors in the past six months, illustrating a dynamic shift towards more diverse and specialized LLM applications. This frequency of change among developers reflects a market eager for innovation and tailored solutions that meet industry-specific needs. As companies seek to balance cost, performance, and functionality, the LLM marketplace is set for continued upheaval and growth [source].
One emerging trend in the AI domain is the movement away from traditional, large-scale AI teams towards more nimble and practical applications of AI technology. Businesses are increasingly integrating AI directly into customer-facing applications, a strategic shift motivated by the desire to deliver immediate, tangible value and ensure cost-effectiveness. Technologies such as retrieval-augmented generation (RAG) and vector databases are rising in prominence as they offer more efficient ways to enhance artificial intelligence capabilities without extensive resources or lengthy development times. This pragmatic approach not only aligns with cost-containment strategies but also looks to maximize return on investment by focusing on real-world applications that improve user experience and business outcomes [source].
With the increased integration of AI features into services and products, the implications for the future are expansive, affecting economic, social, and political fields extensively. Economically, the heightened competition due to new players like DeepSeek entering the market is expected to drive down costs and spur innovation, benefitting consumers and accelerating AI adoption at various scales. Socially, this democratization of AI—powered by both proprietary and open-source LLMs—brings advanced technology to a wider audience, enabling broad-scale problem solving and innovation. Politically, this fast-paced technology growth invites a reevaluation of policies, focusing on balancing ethical considerations with technological advancement, as these LLMs offer new capabilities and pose fresh regulatory challenges [source].
The anticipated shifts in the LLM arena are poised to redefine the strategic priorities of businesses worldwide. The demand for specialized LLMs that serve distinct niche markets demonstrates a broader trend towards more personalized and effective AI solutions. Developers and companies are increasingly viewing open-source platforms as viable alternatives that will allow them to customize and refine models to meet their precise needs, fostering a culture of innovation and accessibility. In this changing environment, organizations will refine their resource allocations, investing in impactful, scalable solutions that promise quantifiable improvements in operational efficiency and customer engagement. This landscape encourages a strategic alignment of LLM technology with business objectives, crafting a future where AI becomes deeply embedded in everyday enterprise operations [source].