Exploring How AI Pervades Our Online World
Pew Research Sheds Light on AI in Web Browsing: A Methodology Deep Dive
Last updated:

Edited By
Mackenzie Ferguson
AI Tools Researcher & Implementation Consultant
Delve into Pew Research's innovative study that analyzes the browsing habits of 900 U.S. adults to understand the AI landscape online. The methodology involves rigorous steps in participant selection, data collection, AI identification, and relevance classification, despite facing some limitations. Explore what this means for our digital interactions and the future of AI.
Participant Selection Criteria
Participant selection criteria in research studies serve as the foundation for the validity and reliability of the study's findings. In the Pew Research study "What Web Browsing Data Tells Us About How AI Appears Online," the selection of participants was meticulously designed to ensure representativeness and accuracy. Participants were sourced from the KnowledgePanel Digital, a probability-based panel that mirrors the broader U.S. adult demographic. This careful selection method helps ensure that findings can be generalized to the larger population, enhancing the study's relevance ().
To further refine the participant selection, specific criteria were applied. Only those who had previously responded to a pilot survey, remained active in the panel, consented to data sharing, and were active users in March 2025 were included in the study. These stringent requirements ensured that participants were engaged and their browsing behaviors were accurately captured. The sample size, while limited to 900 U.S. adults, was chosen deliberately to maintain a balance between comprehensive data collection and manageable data analysis ().
Learn to use AI like a Pro
Get the latest AI workflows to boost your productivity and business performance, delivered weekly by expert consultants. Enjoy step-by-step guides, weekly Q&A sessions, and full access to our AI workflow archive.
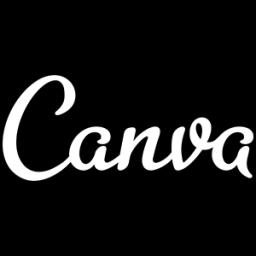
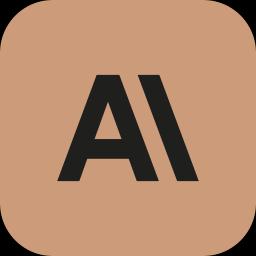
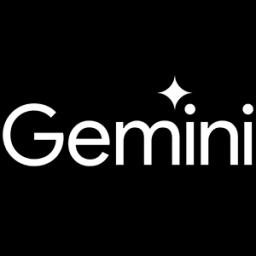
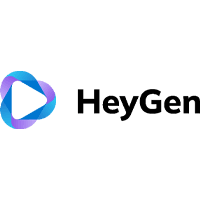
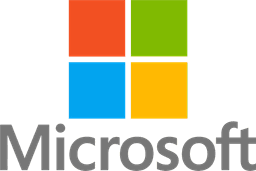
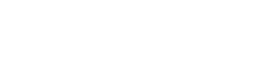
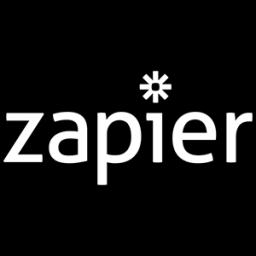
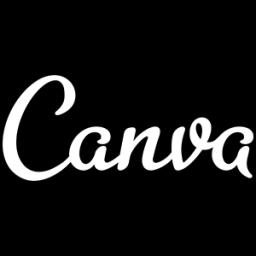
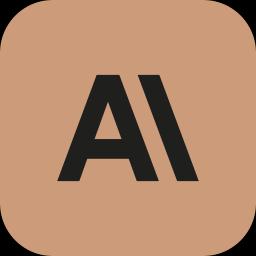
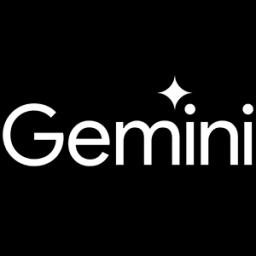
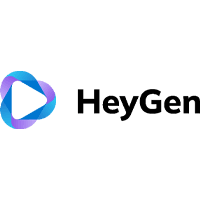
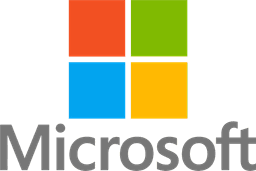
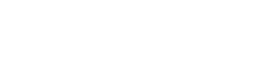
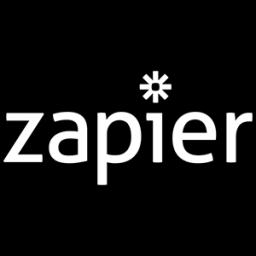
Weighting data collected from participants further contributes to the representativeness of the study. By adjusting the data to reflect the U.S. population accurately, researchers can mitigate biases that may arise from demographic imbalances. This approach is key in making the insights from the study applicable to the real-world context in which AI is encountered on a daily basis. Thus, such rigorous participant selection criteria underscore the study's methodological robustness and its capacity to provide valuable insights into the intersection of web browsing and AI exposure ().
Data Collection and Processing
In their comprehensive report on AI engagement online, the Pew Research Center employs a robust methodology for data collection and processing. By monitoring the browsing behavior of 900 adult participants from the United States, the study provides valuable insights into how AI content is encountered on the internet. All participants were carefully selected from the KnowledgePanel Digital, which ensures a representative cross-section of the U.S. adult population. The data is meticulously collected via the RealityMeter app, providing an authentic glance into real-world web engagement with AI-oriented content. This approach offers a high ecological validity, as noted by experts like Dr. Meredith Broussard from NYU, who praises the reduced bias compared to self-reported surveys .
The process of data collection in the study is both nuanced and intricate, focusing on precise participant monitoring to capture natural browsing habits. With informed consent, the researchers track and analyze a plethora of web interactions, accounting for a wide range of AI exposure patterns online. This data is then weighted to reflect the broader U.S. demographics, enhancing the validity of the findings. However, the methodology's reliance on specific AI-related keywords for webpage identification poses limitations, potentially excluding valuable content that does not feature these terms prominently .
To systematically process the collected data, the study utilizes a logistic regression classifier, refining results to distinguish substantive AI mentions from minor references. This classifier evaluates various criteria, such as keyword density, placement, and page context, thus ensuring only relevant AI-related interactions are examined. While this strategy effectively sharpens the study's focus, experts argue it may inadvertently overlook the broader scope of AI signals present online. The study excluded certain types of pages, like those containing adult content or requiring login credentials, as well as pages unreliably loaded by JavaScript, which could impact outcome comprehensiveness .
Learn to use AI like a Pro
Get the latest AI workflows to boost your productivity and business performance, delivered weekly by expert consultants. Enjoy step-by-step guides, weekly Q&A sessions, and full access to our AI workflow archive.
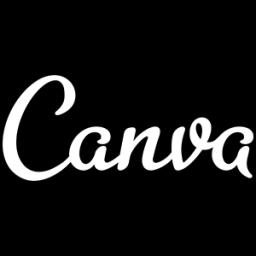
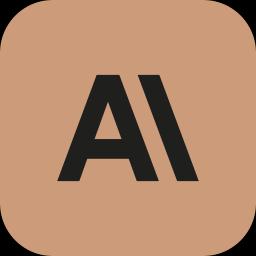
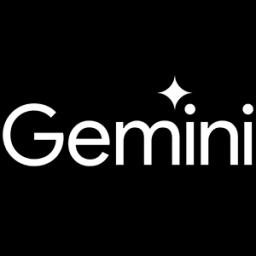
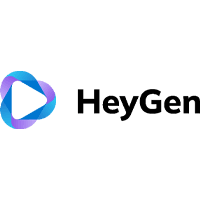
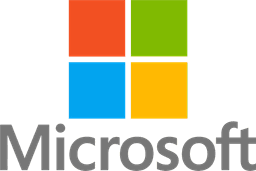
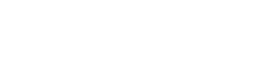
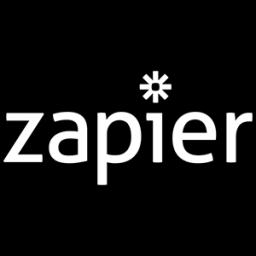
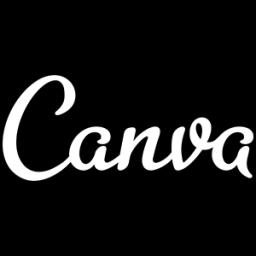
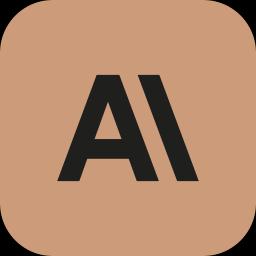
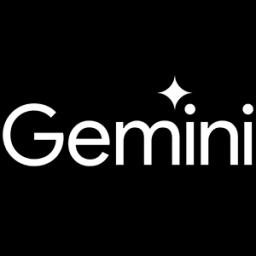
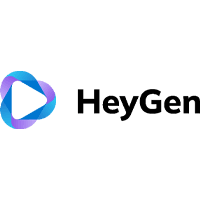
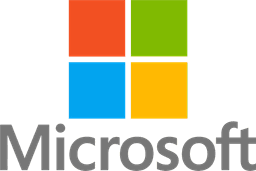
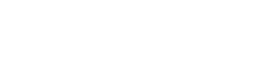
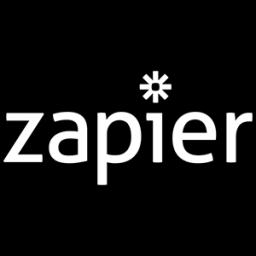
The data processing methodology advances our understanding of AI's digital footprint by aligning structured data collection with intricate analytical tools. However, ethical considerations surrounding privacy remain critical, as outlined by the Electronic Frontier Foundation, accentuating the need for stringent guidelines to safeguard personal data during research analysis. This balance is pivotal, ensuring a reliable yet respectful study of browsing data and shedding light on potential demographic disparities related to AI access and usage .
Through this methodological framework, the Pew Research Center not only captures contemporaneous data on AI presence in online spaces but also contributes significantly to the body of knowledge concerning online behavior studies. By effectively melding demographic insights with AI exposure analysis, the research encapsulates a vital snapshot of current trends while highlighting potential pathways for further longitudinal inquiries into AI's evolving impact on social and media landscapes .
AI Webpage Identification Methodology
The Pew Research Center's examination of webpage identification methodologies centered on understanding AI's online appearance is crucial for setting a benchmark in digital studies. Their approach begins with pinpointing AI-related content through keywords, a foundational step that paves the way for more intricate analysis. By embedding this keyword-based strategy into a logistic regression classifier, the study effectively distinguishes between substantial mentions and fleeting references to AI, thus refining the dataset to reflect genuine exposure to AI discussions. This method not only streamlines the process of identifying relevant digital content but also ensures a higher accuracy in data representation, providing an essential tool for researchers aiming to decode AI's pervasive digital footprint [source].
Furthermore, the dual-step methodology employed by Pew Research underscores advanced data filtering mechanics crucial for addressing the volume and variety of web data. It highlights an innovative path in discerning AI relevance by balancing qualitative keyword presence with quantitative data analytics. Such a refined approach allows researchers to sift through extensive datasets efficiently, focusing on significant AI content that influences public discourse. This meticulous identification process is pivotal in transforming raw data into insightful research findings that can aid policymakers, educators, and enterprises in navigating the complexities of AI integration into daily life. Thus, the methodology stands as a testament to Pew Research's commitment to precision and clarity in social research [source].
Webpage Exclusion Criteria
The Pew Research Center's study on how AI is encountered online implemented several exclusion criteria to ensure data relevance and integrity. Pages deemed unsuitable for analysis included malware sites and those containing adult content, which were excluded due to their inappropriate nature for a general audience. This decision aligns with ethical research standards aimed at minimizing participants' exposure to harmful or offensive material. Moreover, the study omitted productivity tools requiring logins and pages showing a zero-second visit duration. This exclusion was crucial to eliminate potential noise in the data, as these sites might not reflect intentional browsing behavior or could skew the understanding of genuine AI exposure.
Webpages that timed out, returned errors, or had invalid URLs were not included in certain analyses. Such exclusions help maintain the accuracy and reliability of the results by focusing on accessible and functional web content. Similarly, the study avoided analyzing content behind paywalls, dynamic content loaded by JavaScript, and non-text content. This decision stems from a methodological constraint where such content poses challenges in data scraping and processing, potentially leading to incomplete or misclassified data. By setting these criteria, the study aimed to provide a clear, unbiased picture of how U.S. adults encounter AI in their digital lives, focusing on accessible public web content .
Learn to use AI like a Pro
Get the latest AI workflows to boost your productivity and business performance, delivered weekly by expert consultants. Enjoy step-by-step guides, weekly Q&A sessions, and full access to our AI workflow archive.
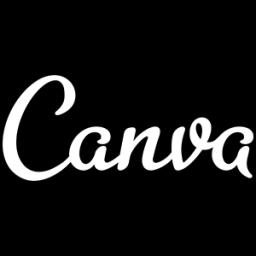
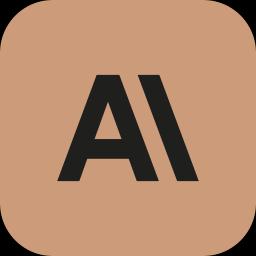
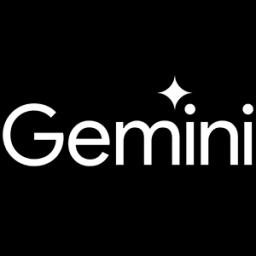
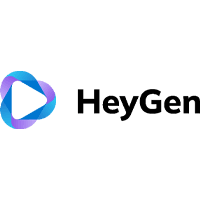
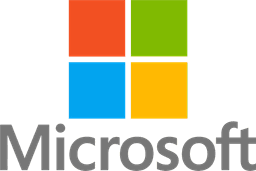
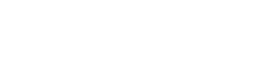
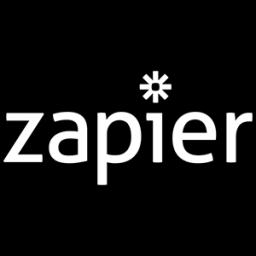
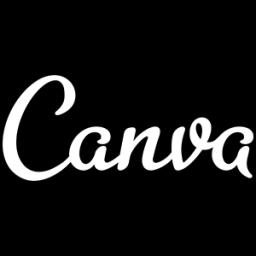
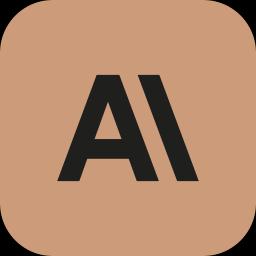
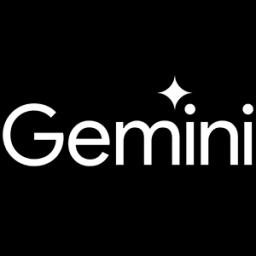
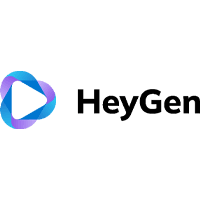
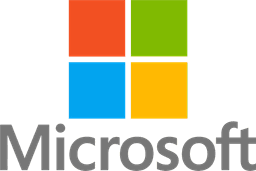
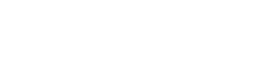
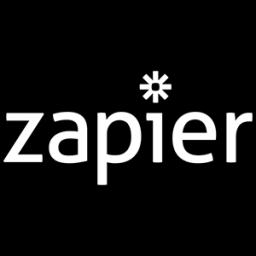
Methodological Limitations
The Pew Research Center's study "What Web Browsing Data Tells Us About How AI Appears Online" features several methodological limitations that need to be considered when interpreting its findings. One significant limitation is the potential loss of dynamic content due to the way browsing data was collected. This can result in missing a significant portion of AI-related interactions that occur through dynamic websites or applications, which aren't fully captured in static webpage analysis. Moreover, content behind paywalls or interactive elements driven by JavaScript might also be excluded from the analysis, possibly impacting the comprehensiveness of the data collected about AI encounters online .
Another major limitation noted in the methodology involves the use of keyword-based identification for AI-related content. While keywords are a practical tool for categorizing data, there's an inherent risk of either missing relevant AI-related webpages or, conversely, misclassifying content where AI may only be mentioned in passing and not substantively discussed. This reliance on keywords might, therefore, limit the accuracy of identifying truly AI-centric webpages, potentially skewing the study’s insights into how AI appears and is represented online .
The study’s sample size and demographic considerations also present limitations in its methodology. Although the dataset is weighted to reflect the U.S. population, the limited sample size of 900 participants might not sufficiently capture the full breadth and diversity of web interactions and the multifaceted nature of AI exposure across different communities. This could lead to potential biases, especially when generalizing findings to the broader population or when making inferences about specific demographic groups' interactions with AI .
Additionally, the exclusion of specific web pages, such as those containing malware, adult content, or those requiring logins and having zero-second visit durations, introduces another layer of potential bias in the data. While these exclusions are necessary for ensuring data quality and participant safety, they might also omit significant contexts where AI-related content could be prevalent, thus narrowing the study’s scope and comprehensiveness .
List of AI Keywords
Artificial Intelligence (AI), a rapidly evolving field, has introduced a myriad of terms and concepts that are now prevalent across various industries and sectors. Understanding AI vocabulary is crucial for comprehending its applications and implications. In the Pew Research Center's study on AI visibility in web browsing data, key AI terms were used to identify relevant online content. These terms, meticulously listed in the study's appendix, serve as a foundation for analyzing AI's digital presence and its impact on user interaction. [0](https://www.pewresearch.org/data-labs/2025/05/23/methodology-metered-data-ai/).
Among the prominent keywords utilized in AI discussions are 'machine learning', 'natural language processing', and 'neural networks'. These terms highlight the foundational technologies that enable AI systems to learn, understand, and mimic human behavior. In addition, keywords like 'algorithm', 'automation', and 'robotics' underline the operational facets of AI technology. The Pew Research study describes how these keywords were instrumental in identifying websites that discuss AI extensively, providing insights into public engagement and understanding of AI technologies online. [0](https://www.pewresearch.org/data-labs/2025/05/23/methodology-metered-data-ai/).
Learn to use AI like a Pro
Get the latest AI workflows to boost your productivity and business performance, delivered weekly by expert consultants. Enjoy step-by-step guides, weekly Q&A sessions, and full access to our AI workflow archive.
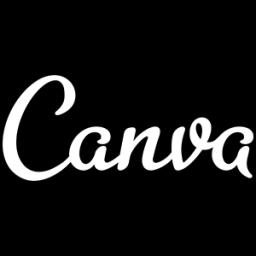
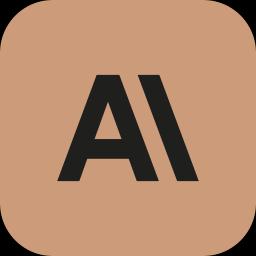
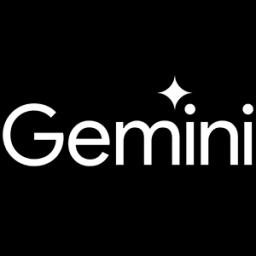
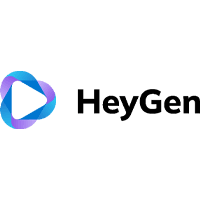
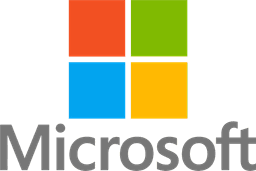
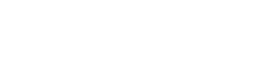
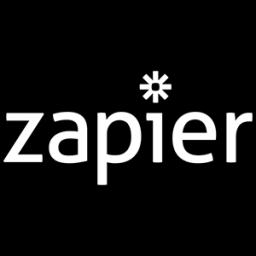
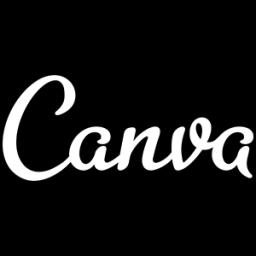
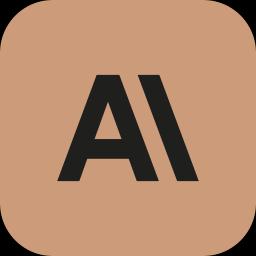
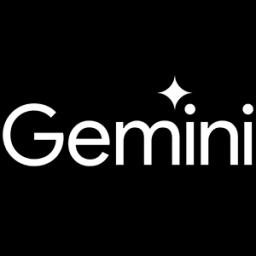
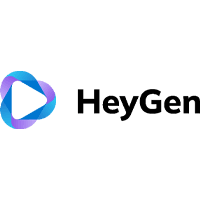
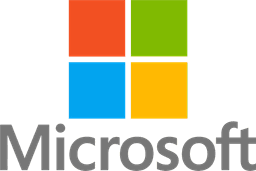
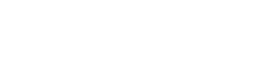
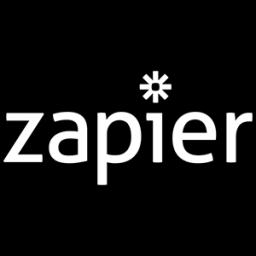
Furthermore, the study included more specific terms such as 'artificial neural network (ANN)', 'deep learning', and 'cognitive computing'. These keywords are vital in understanding advanced AI processes and their applications in various fields like healthcare and finance. The selection of these terms reveals the study's methodological rigor in categorizing and quantifying AI's digital footprint, ensuring that the analysis captures a comprehensive view of AI's role in modern digital life. [0](https://www.pewresearch.org/data-labs/2025/05/23/methodology-metered-data-ai/).
In the context of public perception and technological impact, other terms such as 'AI ethics', 'bias in AI', and 'AI governance' have garnered attention and are frequently mentioned in AI-related discussions. The inclusion of these terms in the Pew Research Center's keyword list reflects the growing societal debate around the ethical implications of AI technologies. These discussions emphasize the necessity for responsible AI development and deployment, echoing concerns about AI's societal influence and governance challenges. [0](https://www.pewresearch.org/data-labs/2025/05/23/methodology-metered-data-ai/).
Lastly, emerging concepts such as 'AI in journalism', 'internet of things (IoT)', and 'digital transformation' were also part of the keyword framework. These terms illustrate the expansive and interdisciplinary nature of AI studies, as the technology continues to reshape not only technical fields but also areas like media, communication, and consumer technologies. The study’s insights into these keywords help track the technology’s evolving impact and underscore the need for ongoing research and dialogue regarding AI's future trajectories. [0](https://www.pewresearch.org/data-labs/2025/05/23/methodology-metered-data-ai/).
Impact of AI on Web Browsing
Artificial Intelligence (AI) continues to revolutionize various online activities, positively impacting web browsing experiences in unprecedented ways. In recent years, AI technologies have been seamlessly integrated into web browsers, enhancing features such as intelligent search and personalized content recommendations. These improvements make the browsing experience not only faster but also more customized to individual user preferences, addressing the need for immediacy and relevance in internet searches. The integration of AI into browsing tools represents a significant shift, offering insights into user behavior and preferences to present content that is more relevant and engaging. As highlighted by recent studies, including one by Pew Research Center, the presence of AI can be seen across many web activities, influencing how information is accessed and understood by diverse audiences. For more insights on AI's transformation of browsing, visit Pew Research and other related publications.
One of the critical uses of AI in web browsing is its ability to improve security and privacy features. Advanced AI algorithms can detect and block harmful content, phishing sites, and malicious advertisements, significantly bolstering the safety of browsers. Moreover, AI's role in ensuring privacy through techniques like differential privacy and intelligent tracking prevention cannot be overstated. These features help maintain users' privacy without compromising on browsing efficiency. As users grow increasingly concerned about their online safety, the implementation of these AI-driven features becomes essential to meet modern security demands. The Pew Research Center's study on AI in web browsing further highlights this need for a secure digital environment. More details on how AI is shaping online privacy can be accessed here.
AI's impact on web browsing also extends to accessibility, enhancing user engagement and inclusivity online. By providing tools such as voice recognition and language translation directly within browsers, AI helps break down barriers for users with disabilities or non-native speakers. This advancement ensures that web technologies are accessible to a broader audience, fostering an inclusive digital space where everyone can participate fully. AI's contribution to improving accessibility is a step towards equal access to information, thereby democratizing the internet. Studies like those by Pew Research underscore the broader societal benefits AI brings to the digital landscape. To explore how AI tools are enhancing web interactions, see more at Pew Research.
Learn to use AI like a Pro
Get the latest AI workflows to boost your productivity and business performance, delivered weekly by expert consultants. Enjoy step-by-step guides, weekly Q&A sessions, and full access to our AI workflow archive.
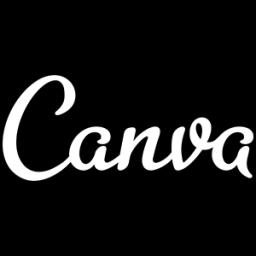
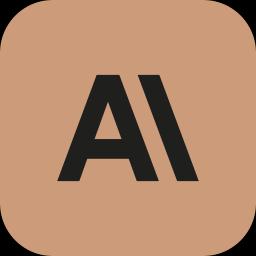
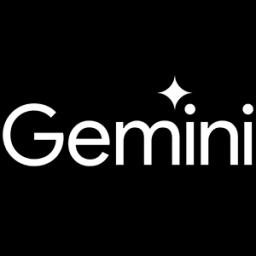
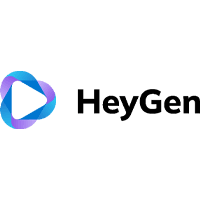
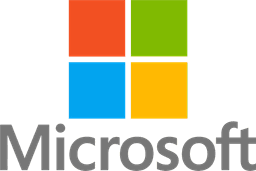
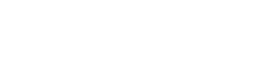
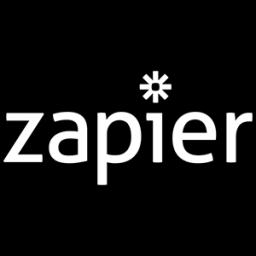
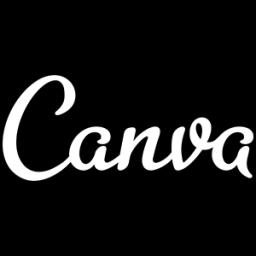
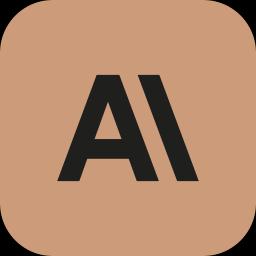
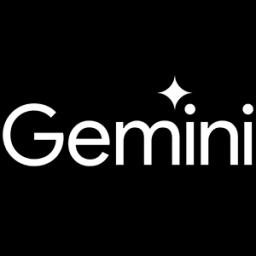
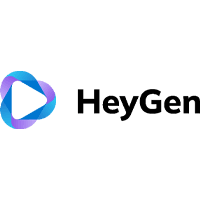
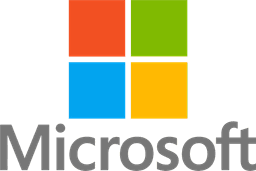
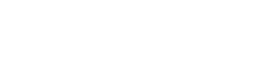
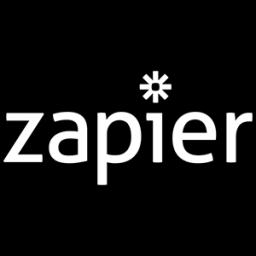
Public Skepticism and Bipartisan Concerns
Public skepticism towards AI, particularly in journalism, is a growing concern that spans across various demographics and political affiliations in the United States. A recent survey by the Pew Research Center found that half of U.S. adults are apprehensive about the negative impacts AI might have on news reporting. There's a fear that AI could compromise the credibility and quality of journalism, with nearly 60% expressing concerns that AI will lead to job losses in the media industry . These worries are not limited to a single political party but are shared among Americans regardless of political leaning, illustrating a rare bipartisan agreement on the issue of AI's potential to influence and possibly erode the integrity of news media. This bipartisan concern highlights the broader anxieties many feel about AI’s role in shaping public discourse and its contribution to the proliferation of misinformation .
In response to these concerns, there's an increasing demand for stringent oversight and regulatory frameworks that govern the use of AI in journalism and media-related technologies. The apprehensions are further exacerbated by studies and reports emphasizing the critical need for improving trust in AI-driven news delivery mechanisms. By adopting more transparent methodologies and integrating human judgment along with AI, news organizations could potentially mitigate public fears and ensure more trustworthy dissemination of information . The insistence from both ends of the political spectrum also suggests an opportunity for policymakers to craft bipartisan solutions that address the ethical utilization of AI in journalism and reinforce public trust in media institutions.
Expert Opinions on Study Methodology
Dr. Meredith Broussard, a data science professor at NYU, highlights the study's ecological validity as a significant strength. By passively observing participants' actual browsing behavior, the study avoids biases typically present in self-reported data. This method provides a more accurate reflection of how people encounter artificial intelligence in their daily online interactions compared to traditional surveys or interviews. Such ecological validity is crucial for understanding the natural context in which AI is integrated into everyday digital experiences. Dr. Broussard emphasizes that this approach allows for insights that more accurately represent user experiences and the genuine pathways through which AI is encountered online. For more information, you can view the detailed findings [here](https://www.nyu.edu/about/news-publications/news/2025/may/pew-research-ai-browsing-data.html).
Dr. Eszter Hargittai, a professor of communication studies at the University of Zurich, points to the study's use of a probability-based sample through the KnowledgePanel as a vital aspect for ensuring generalizability. This approach contrasts with many online studies that often rely on convenience samples, which may not accurately reflect the diverse demographics of internet users. The study's design helps overcome this limitation by employing a methodology that aims to accurately represent the broader U.S. adult population. Dr. Hargittai underscores that the representativeness of the sample is key to drawing conclusions that are applicable to real-world settings. Additional insights about the study's demographic representation can be found [here](https://www.news.uzh.ch/en/articles/media/2025/KI-im-Alltag.html).
An analysis in *Tech Policy Daily* suggests that future research should aim to address the "AI divide," examining how different demographic groups encounter and perceive AI online. The current study provides a foundation, but there is an opportunity to delve deeper into understanding disparities in AI exposure related to factors such as age, socioeconomic status, and education. By expanding the research to include these dimensions, policymakers and technologists can better ensure equitable access to AI technologies and a comprehensive understanding of AI's societal impact. Insights into this analysis can be explored further [here](https://techpolicydaily.com/articles/pew-research-ai-browsing-data-analysis/).
The Brookings Institution emphasizes the need for longitudinal studies to understand the long-term implications of AI exposure on societal attitudes and behaviors. While the Pew Research study offers a snapshot of current AI encounter patterns, it raises essential questions about how these interactions might evolve over time. Understanding these changes is critical for anticipating shifts in public perception and adapting policies to address emerging challenges and opportunities posed by AI. With an eye towards the future, the Brookings Institution discusses potential pathways for research development [here](https://www.brookings.edu/research/how-do-americans-encounter-ai-online-new-insights-from-pew-research-center/).
Learn to use AI like a Pro
Get the latest AI workflows to boost your productivity and business performance, delivered weekly by expert consultants. Enjoy step-by-step guides, weekly Q&A sessions, and full access to our AI workflow archive.
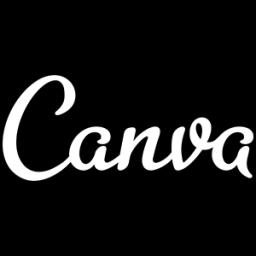
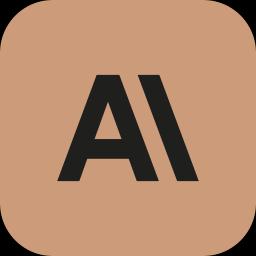
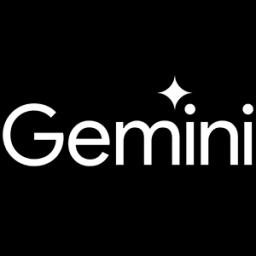
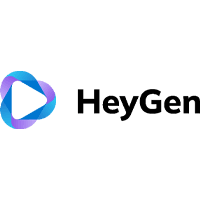
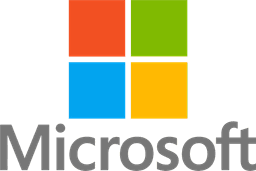
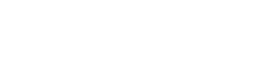
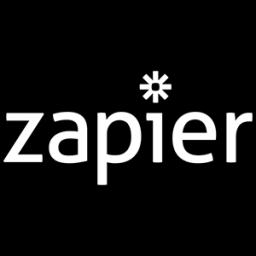
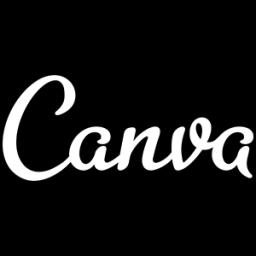
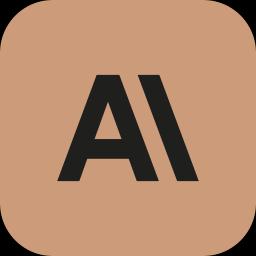
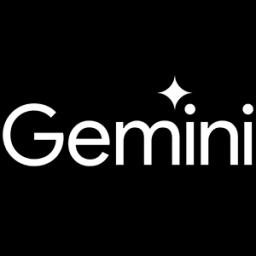
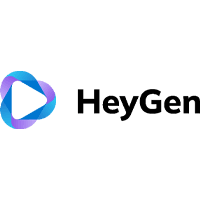
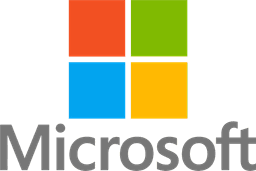
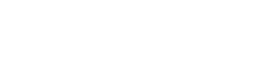
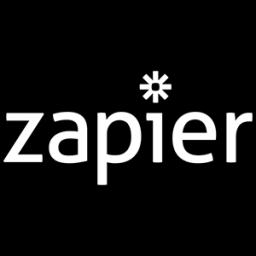
The Electronic Frontier Foundation (EFF) raises important ethical considerations regarding privacy in studies involving browsing data collection. While the Pew study obtained informed consent from participants, EFF highlights the critical need for clear guidelines and robust safeguards to protect individuals' privacy rights. As browsing data can be highly personal, ensuring that such research is conducted ethically is paramount to maintaining participants' trust and safeguarding their personal information. The EFF's insights into privacy implications and ethical considerations can be explored further [here](https://www.eff.org/deeplinks/2025/05/pew-research-study-ai-browsing-data-privacy-implications).
Future Implications of the Study
The Pew Research Center's study on AI encounters via web browsing holds significant implications for the future, particularly in shaping economic, social, and political landscapes. As AI becomes an increasingly integral component of online interactions, this study's methodology highlights potential gaps in economic forecasting. For example, the reliance on keyword identification might not fully capture the complex ways in which AI is integrated into the economy, possibly leading to inaccuracies in predicting market trends and job market shifts. With only 900 participants, the sample size may fall short in representing the diverse economic interactions individuals have with AI, posing a challenge for analysts seeking to gauge AI’s economic footprint accurately.
Socially, the study emphasizes the limitations of using web browsing data alone to understand AI's impact on society. Browsing behavior may not encompass the full spectrum of human interaction with AI, missing out on engagements through mobile applications or interpersonal communication. As a result, societal analyses may overlook key areas where AI influences public life, such as education and healthcare. Additionally, disparities in digital literacy and access to AI-related resources could exacerbate existing social inequalities, leading to a divide where certain segments of the population remain underserved or misinformed about AI's potential benefits and risks.
Politically, the implications are equally profound. The study's insights might skew perceptions of public opinion about AI due to potential sampling biases. By not fully capturing the diversity across demographics, there is a risk that policy recommendations derived from the study fail to adequately reflect the nuanced opinions and experiences of different groups. Furthermore, as AI continues to be a topic of political discourse, its role in campaigning and policymaking becomes more prominent. However, the study's exclusion of untracked devices and social media content limits a thorough understanding of AI's role in shaping political narratives and spreading misinformation. Hence, addressing these gaps is crucial for formulating informed AI governance policies.