Cybersecurity Alert
Protect Your AI – New Guidelines Emphasize Data Security!
Last updated:

Edited By
Mackenzie Ferguson
AI Tools Researcher & Implementation Consultant
The latest Cybersecurity Information Sheet (CSI) highlights the best practices to shield data in AI and ML systems, diving into crucial risks like data poisoning and drift, and providing effective strategies to mitigate them.
Introduction to AI Data Security
Artificial Intelligence (AI) has profoundly transformed various aspects of the modern digital landscape, introducing groundbreaking capabilities in areas from healthcare to finance. However, with these advancements comes the critical task of ensuring robust data security, particularly as it relates to AI and machine learning (ML) systems. The integrity, confidentiality, and availability of data are paramount, as deficiencies in these areas can lead to significant vulnerabilities. A comprehensive approach to AI data security involves addressing potential threats throughout the entire AI lifecycle, from data acquisition and preprocessing to model deployment and maintenance.
The importance of data security within AI systems cannot be overstated, as these systems often handle sensitive and personally identifiable information. For instance, in sectors such as healthcare, financial services, and national security, the stakes are extraordinarily high. The Cybersecurity Information Sheet (CSI) highlights essential best practices to safeguard data used in AI and ML, emphasizing the need for a secure data supply chain and strategies to mitigate risks like data poisoning and data drift.
Learn to use AI like a Pro
Get the latest AI workflows to boost your productivity and business performance, delivered weekly by expert consultants. Enjoy step-by-step guides, weekly Q&A sessions, and full access to our AI workflow archive.
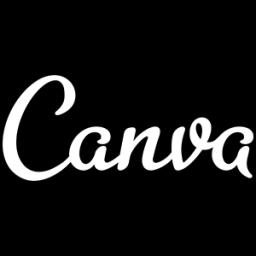
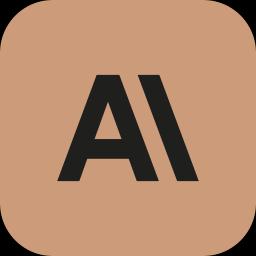
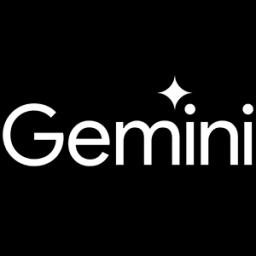
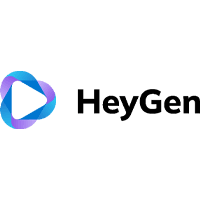
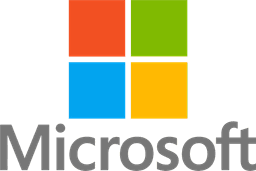
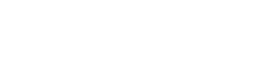
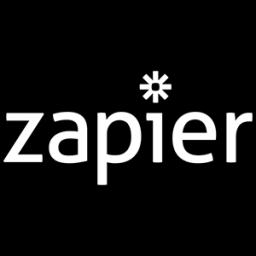
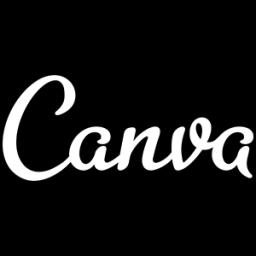
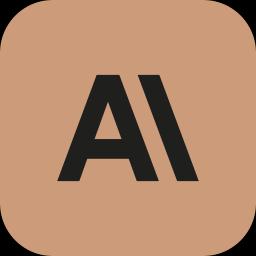
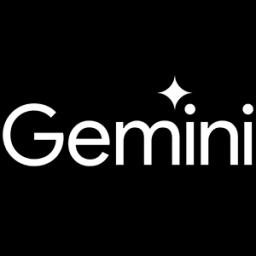
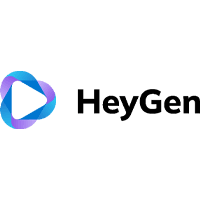
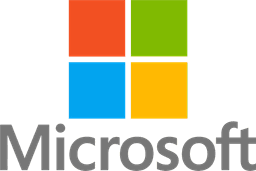
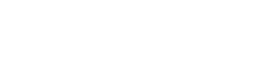
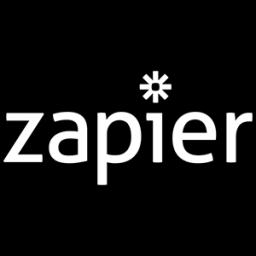
Data provenance plays a crucial role in AI data security, ensuring that the origin and transformation history of a dataset can be traced accurately. By maintaining comprehensive records of data lineage, organizations can effectively track modifications, verify the integrity of data inputs, and comply with regulatory standards. As emphasized in the CSI document, understanding data provenance is essential for identifying unauthorized changes and maintaining the trustworthiness of AI systems.
Furthermore, data integrity must be consistently verified to prevent unauthorized alterations that could compromise model performance. Techniques such as using checksums, cryptographic hashes, and digital signatures are pivotal in this regard, offering mechanisms to ensure data authenticity and detect potential tampering early. This aspect of security is vital, as even minor data errors can propagate through AI systems, leading to substantial deviations in outputs.
In light of increasing cyber threats, organizations are urged to adopt layered security measures. This includes secure data storage and transmission protocols, rigorous data validation processes, and continuous monitoring for anomalies. By investing in these robust security frameworks, businesses can not only protect their AI assets but also maintain competitive advantage by securing consumer trust. The commitment to safeguarding AI data emphasizes a forward-thinking approach, anticipating and mitigating potential security challenges.
Understanding Data Provenance
Understanding data provenance is crucial in the development and maintenance of secure AI systems. Data provenance refers to the comprehensive record of data's origin, where it has traveled, and how it has been altered over time. This concept is essential in AI data security because it enables organizations to trace back to the source of data, ensuring its authenticity and integrity. Having a clear provenance path can reveal unauthorized modifications or data corruption that might otherwise go unnoticed. Such transparency is vital in preventing security breaches and maintaining trustworthiness in AI operations. According to the Cybersecurity Information Sheet (CSI), tracing the full lineage of data helps mitigate risks associated with data supply chain vulnerabilities, which are pivotal in safeguarding AI systems ().
Learn to use AI like a Pro
Get the latest AI workflows to boost your productivity and business performance, delivered weekly by expert consultants. Enjoy step-by-step guides, weekly Q&A sessions, and full access to our AI workflow archive.
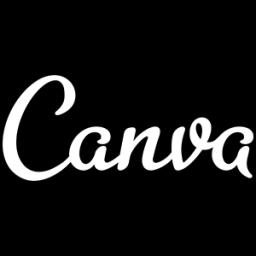
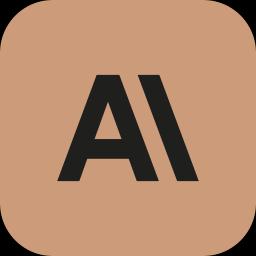
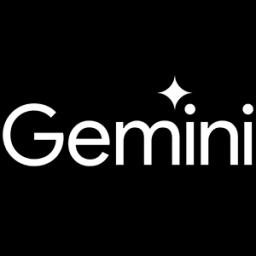
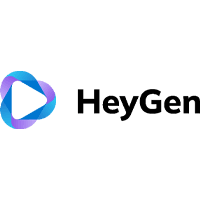
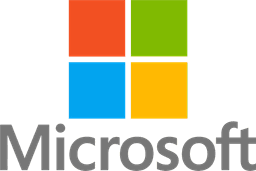
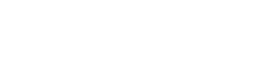
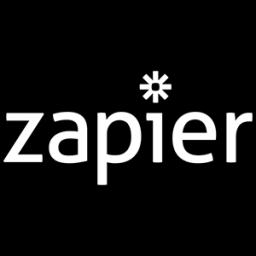
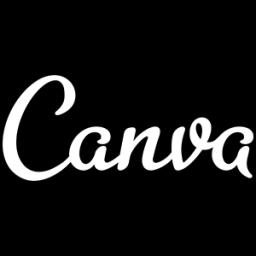
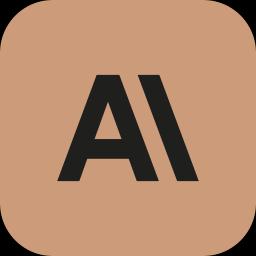
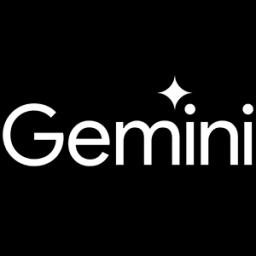
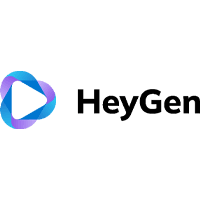
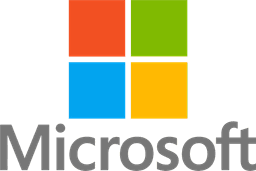
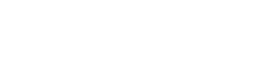
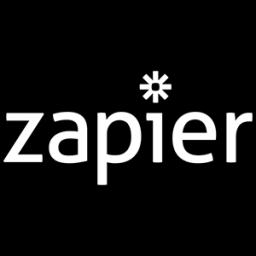
The significance of data provenance extends beyond just tracking the data's journey from source to application; it also plays a vital role in compliance with regulatory standards and promoting ethical data use. Ensuring data provenance involves employing technical measures such as cryptographic techniques to verify the integrity and authenticity of data as it moves across different stages of the AI lifecycle. The CSI document emphasizes maintaining data integrity through various methods, including checksums and digital signatures, which assist in detecting unauthorized data alterations. Leveraging data provenance effectively aligns with best practices for AI and ML systems security by providing necessary transparency and accountability in data handling and processing processes ().
Methods of Ensuring Data Integrity
Ensuring data integrity is a critical component of maintaining the reliability and trustworthiness of AI and machine learning (ML) systems. One effective method for ensuring data integrity involves the implementation of rigorous validation mechanisms at every stage of the data lifecycle. Techniques such as data checksums and cryptographic hashes are employed to verify that data has not been altered during transmission. These methods help in detecting unauthorized changes, providing a level of assurance that data has remained consistent and true to its original form .
Another essential practice in maintaining data integrity involves establishing secure and verified data supply chains. This includes verifying the origins of data sources and ensuring they meet predefined standards for data quality. Data provenance—the tracking of the origin and history of data—is particularly significant, as it allows organizations to trace back the data to its source, facilitating accountability and transparency .
Organizations also focus on securing data through digital signatures and content credentials. By implementing digital signatures, businesses can ensure data authenticity and integrity, as these signatures can reveal unauthorized attempts to alter the data. Furthermore, the use of secure storage solutions is crucial. These solutions protect data at rest and in transit by implementing encryption techniques, thus safeguarding data against potential breaches .
Regular audits and monitoring of AI systems play a vital role in detecting anomalies that may affect data integrity. Continuous monitoring allows organizations to identify data drift—where the statistical properties of data change over time—leading to gradual declines in AI model performance. With proactive monitoring, these systems can adapt by retraining models to accommodate new data patterns, thereby maintaining their accuracy and reliability .
Finally, addressing data poisoning threats—where malicious actors inject false data to influence AI model outcomes—requires robust defensive strategies. These include verifying data from web-scale datasets and employing consensus-based and anomaly detection methods. By implementing these strategies, organizations can mitigate risks and ensure the data's integrity, vital for accurate and trustworthy AI operations .
Learn to use AI like a Pro
Get the latest AI workflows to boost your productivity and business performance, delivered weekly by expert consultants. Enjoy step-by-step guides, weekly Q&A sessions, and full access to our AI workflow archive.
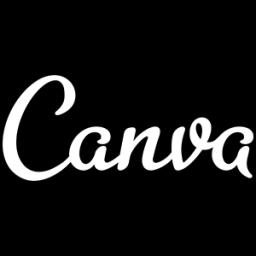
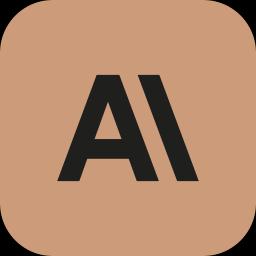
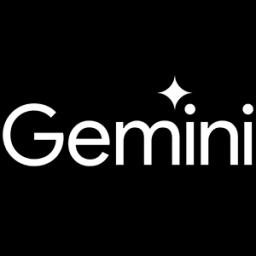
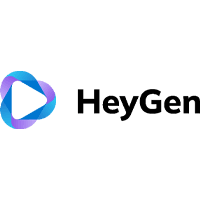
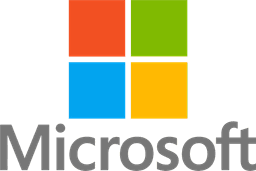
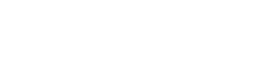
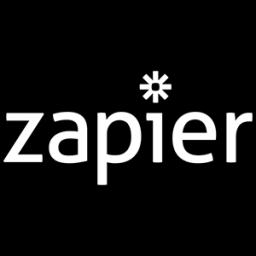
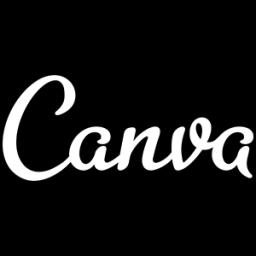
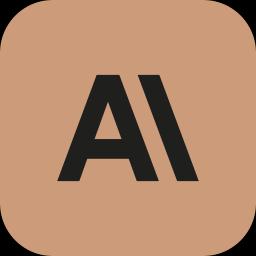
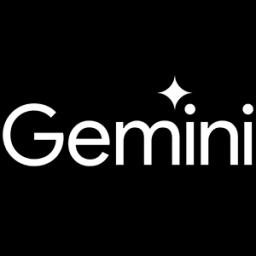
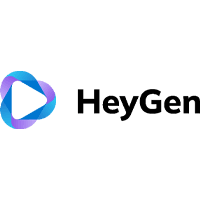
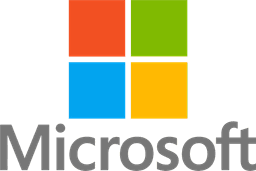
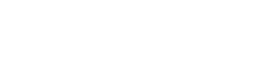
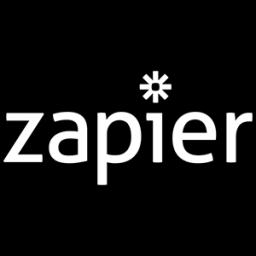
Exploring Data Drift and Its Impact
Data drift presents a significant challenge in maintaining the effectiveness of AI systems. It occurs when there are changes in the underlying data distributions over time, which may lead to a decrease in model performance. As the input data evolves, models trained on previous data sets may become obsolete, thereby affecting their predictions and outputs. The Cybersecurity Information Sheet (CSI) highlights the importance of recognizing data drift as a key risk in AI data integrity. Mitigating its impact involves continuous monitoring of data inputs and regular updates or retraining of AI models to align with new data trends.
The impact of data drift on AI systems is profound, especially in sectors relying heavily on data accuracy, such as finance, healthcare, and autonomous vehicles. In these industries, even minor discrepancies in data can lead to substantial consequences, such as financial losses or safety concerns. Therefore, organizations must employ robust strategies to detect and respond to data drift early. The CSI sheet recommends utilizing anomaly detection tools and maintaining a feedback loop that consistently evaluates model performance against new data. This approach helps in promptly identifying shifts that could affect the system's outputs.
To combat the adverse effects of data drift, organizations are urged to adopt comprehensive data management practices. These include ensuring high-quality data input, implementing rigorous validation protocols, and relying on automated tools that can flag significant variances in historical versus new data sets. As noted in the CSI document, regular model retraining and adjustment to new data characteristics are critical for maintaining AI systems' operational integrity. Consequently, continuous investment in advanced data analytics and monitoring technologies becomes indispensable.
The distinction between data drift and data poisoning is crucial for effective AI system management. While data drift refers to slow and often natural changes in data characteristics over time, data poisoning is a deliberate act to corrupt AI model inputs. As outlined in the document, understanding this difference helps in deploying specific strategies tailored to each threat. Organizations can protect against data drift through dynamic model management, whereas safeguarding against poisoning demands stringent data security measures and validation processes.
The evolving nature of data drift necessitates a proactive stance among AI and ML practitioners, focusing on agility and adaptability of models. By integrating continuous learning frameworks, organizations can enhance model resilience against unforeseen data shifts. This aligns with the strategies highlighted within the CSI guidelines, which advocate for a lifecycle approach to AI data security. Such frameworks not only address data drift but also prepare AI systems to handle other emerging threats effectively.
Mitigating Data Poisoning Risks
Data poisoning poses a significant threat to AI systems by introducing malicious data into the datasets used for training, thus deceiving models and generating faulty outputs [0](https://media.defense.gov/2025/May/22/2003720601/-1/-1/0/CSI_AI_DATA_SECURITY.PDF). This risk underscores the importance of adopting comprehensive strategies to mitigate such threats throughout the AI development lifecycle. By employing stringent data verification techniques, such as source authentication and data integrity checks, organizations can prevent corrupted data from tainting AI models [2](https://outshift.cisco.com/blog/how-to-ensure-ai-data-integrity).
Learn to use AI like a Pro
Get the latest AI workflows to boost your productivity and business performance, delivered weekly by expert consultants. Enjoy step-by-step guides, weekly Q&A sessions, and full access to our AI workflow archive.
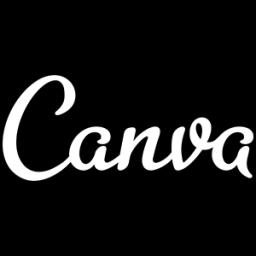
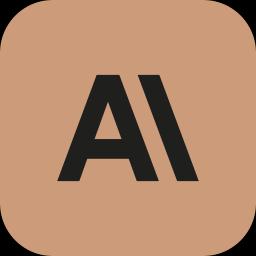
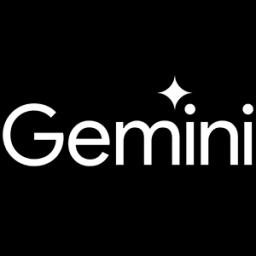
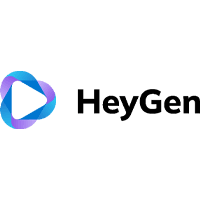
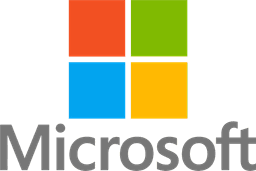
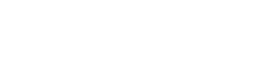
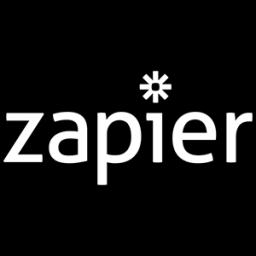
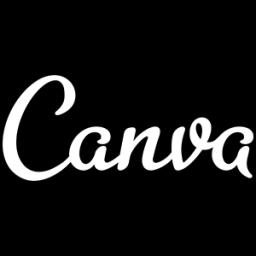
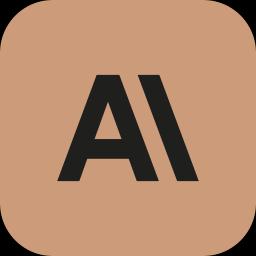
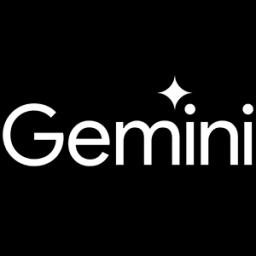
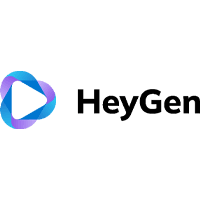
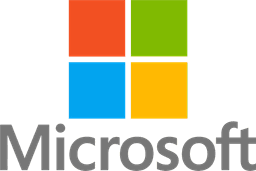
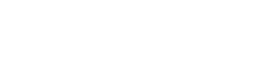
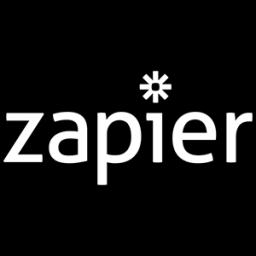
Mitigation begins with securing the data supply chain. It involves verifying the origin and integrity of data through trusted sources and maintaining comprehensive data provenance records. Utilizing digital signatures and cryptographic hashes further ensures that any unauthorized data alterations are promptly identified [2](https://outshift.cisco.com/blog/how-to-ensure-ai-data-integrity). Robust validation protocols, coupled with access controls, can significantly reduce the likelihood of data poisoning attacks on AI systems [3](https://www.sentinelone.com/cybersecurity-101/cybersecurity/data-poisoning/).
Furthermore, incorporating frequent model retraining and continuous monitoring can help identify and correct deviations in model performance that might suggest data poisoning or drift. Anomaly detection systems play a vital role in identifying unexpected behavior in AI outputs, enabling timely interventions [3](https://www.sentinelone.com/cybersecurity-101/cybersecurity/data-poisoning/). These practices, aligned with recommendations from cybersecurity guidelines, are essential to safeguard AI systems from emerging threats [0](https://media.defense.gov/2025/May/22/2003720601/-1/-1/0/CSI_AI_DATA_SECURITY.PDF).
Public and private sector collaboration can enhance data security by sharing insights and developing industry standards for AI data handling. This cooperative approach ensures that the best practices for data verification and security are universally applied, thus minimizing vulnerabilities across different systems and domains [1](https://www.cisa.gov/news-events/news/cisa-and-partners-release-guidance-ai-data-security).
Differences Between Data Drift and Data Poisoning
On the other hand, data poisoning refers to deliberate attacks where adversaries inject malicious data into a training dataset, thereby compromising the integrity and functionality of an AI system. This form of attack can lead to drastic errors in model predictions, as the injected data can bias the model's learning process. A common consequence of a successful data poisoning attack is the model's increased vulnerability to incorrect outputs, which can have severe implications, especially in critical sectors such as healthcare or finance. Effective strategies against data poisoning include implementing rigorous data validation procedures, ensuring data provenance, and using defensive techniques such as anomaly detection and robust training algorithms. The Cybersecurity Information Sheet offers comprehensive strategies for mitigating data poisoning risks.
Recent Developments in AI Data Security
Recent advancements in AI data security focus on enhancing protections for data used in artificial intelligence and machine learning systems. A critical document, the Cybersecurity Information Sheet (CSI), provides a comprehensive guide with best practices for securing AI data, stressing the importance of maintaining security across the entire AI lifecycle (). This includes safeguarding against risks such as data poisoning and data drift, which can significantly affect the reliability of AI systems.
Data security in AI systems is complex due to potential vulnerabilities in the data supply chain. The CSI identifies these vulnerabilities and suggests strategies to mitigate them, such as the use of data provenance techniques. Data provenance, which refers to tracking the origin and changes of data, is a vital aspect of ensuring data integrity in AI. It allows for the identification of unauthorized modifications and verification of data's authenticity ().
Learn to use AI like a Pro
Get the latest AI workflows to boost your productivity and business performance, delivered weekly by expert consultants. Enjoy step-by-step guides, weekly Q&A sessions, and full access to our AI workflow archive.
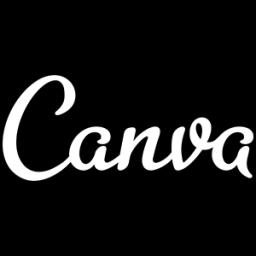
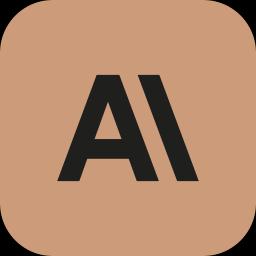
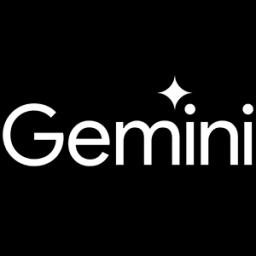
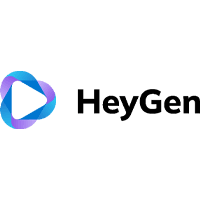
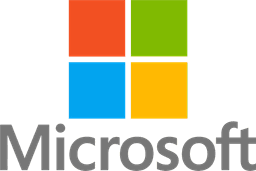
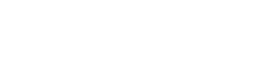
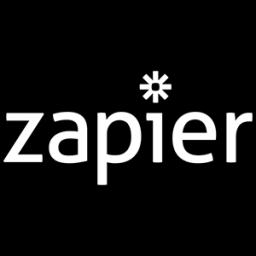
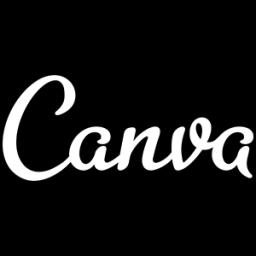
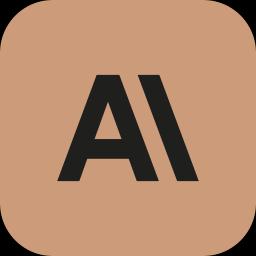
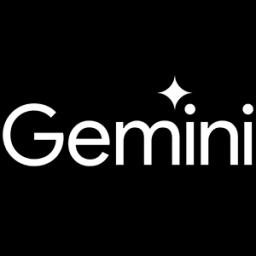
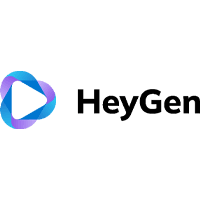
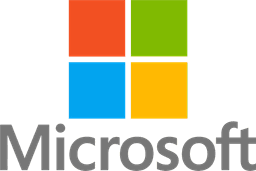
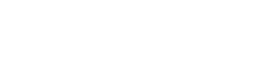
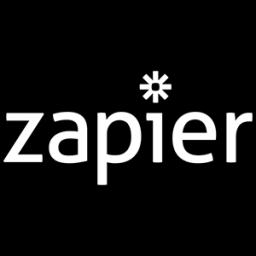
Organizations can face significant risks from data drift and data poisoning. Data drift, the change in statistical properties of data over time, can lead to diminished performance of AI models. In contrast, data poisoning involves intentional manipulation of data to corrupt model outputs, potentially causing significant harm (). Both issues require ongoing monitoring and robust security measures to prevent disruptions to AI operations.
The rising instances of ransomware attacks leveraging AI underscore the evolving landscape of cyber threats. These attacks exploit weaknesses in AI systems' data security, emphasizing the need for enhanced defenses. As AI becomes more embedded in critical infrastructure, safeguarding against such threats becomes paramount. Collaborative efforts, such as those by CISA and international partners, aim to strengthen AI data security protocols ().
Expert perspectives highlight the need for robust security measures throughout the AI lifecycle, including data validation, secure storage, and regular model assessments. Ensuring AI data integrity is crucial, as breaches in security can lead to unreliable outcomes and compromised trust in AI applications (). Effective security practices must be a priority for organizations utilizing AI technologies, with a focus on mitigating risks associated with the data supply chain.
Public awareness of AI data vulnerabilities is likely to grow as data security challenges become more widely understood. This may lead to increased skepticism towards AI technologies, necessitating educational initiatives to improve public understanding and build trust in AI systems. At the same time, regulatory bodies may introduce stricter guidelines to protect sensitive data and ensure AI systems' integrity, which could significantly impact sectors reliant on AI ().
Expert Opinions on AI Security Challenges
AI security presents a myriad of challenges, as attested by experts in the field. A key concern is the integrity and security of data throughout the AI lifecycle—a process that spans data acquisition, preprocessing, model training, to deployment and maintenance. Experts emphasize that any breach at these stages can lead to significant vulnerabilities. As highlighted in a Cybersecurity Information Sheet, data breaches pose substantial threats as they often involve highly sensitive information. The data supply chain, in particular, is susceptible to threats, as contaminated data can lead to tainted AI training processes and erroneous outputs.
One of the critical threats to AI system security is data poisoning, where adversaries deliberately insert harmful data into training datasets. This can severely undermine the system's predictive accuracy, with studies showing that even a small percentage of poisoned data can cause significant disruptions. Experts like those at SentinelOne note that data validation and secure data storage are crucial in countering these attacks. Regular retraining of models and the implementation of anomaly detection methods serve as further measures to safeguard against these insidious threats (Source).
Learn to use AI like a Pro
Get the latest AI workflows to boost your productivity and business performance, delivered weekly by expert consultants. Enjoy step-by-step guides, weekly Q&A sessions, and full access to our AI workflow archive.
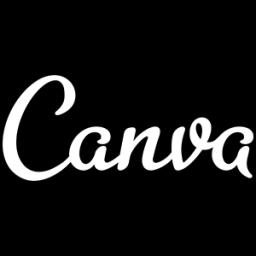
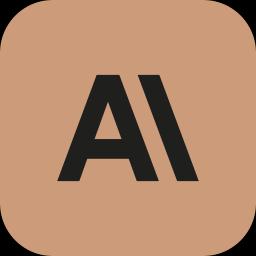
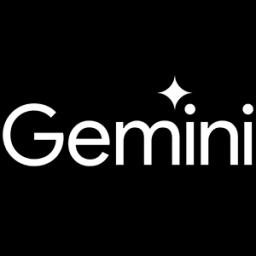
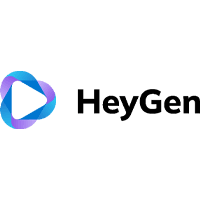
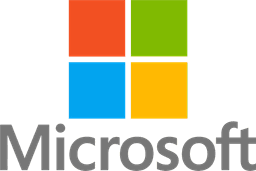
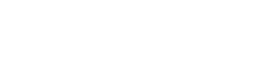
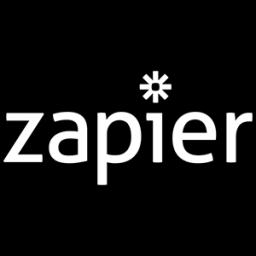
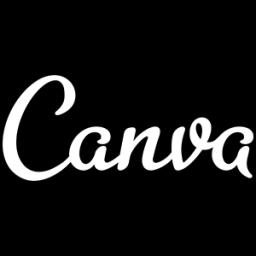
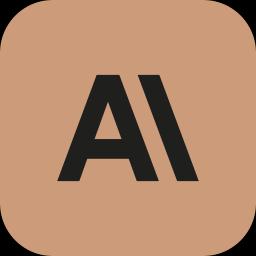
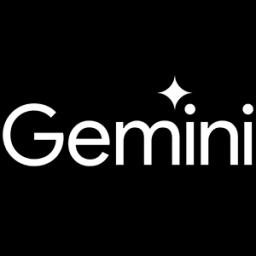
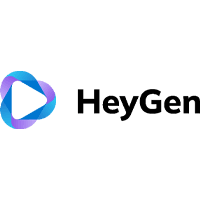
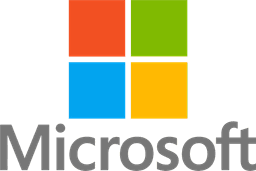
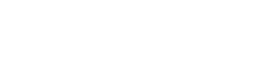
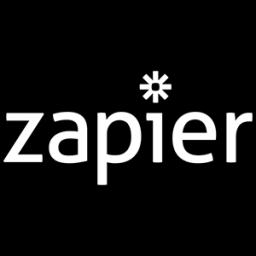
The threat of data drift is another significant concern. As the statistical properties of input data evolve, the performance of AI models can deteriorate. Continuous monitoring and model updating are recommended by cybersecurity professionals to mitigate these risks. Organizations are advised to verify the provenance of their data rigorously, ensuring its integrity and traceability. As outlined by Cisco's experts, employing such vigilant practices can help preserve the quality and reliability of AI outputs (Source).
Increasing awareness of these AI security challenges is leading to significant economic, social, and political shifts. Companies are increasingly investing in robust cybersecurity infrastructures, spurring growth in firms dedicated to AI data protection. Socially, as public understanding deepens regarding issues like data poisoning and drift, there may be a rising skepticism about AI technologies in crucial applications, such as health diagnostics and autonomous vehicles. This warrants educational initiatives to foster public acceptance and trust. Politically, we could see new regulatory frameworks focusing on AI data security to safeguard critical infrastructures. International cooperation may also become pivotal in establishing global AI safety standards, aiming for a coherent response to these emerging threats (Source).
Future Implications of AI Data Security
As AI systems become increasingly integrated into critical aspects of daily life and industry, the future implications of AI data security are vast and complex. A key focus will likely be on ensuring data integrity throughout the AI lifecycle, as outlined in the Cybersecurity Information Sheet on AI data security (). Organizations will need to implement robust data provenance systems to track the origin and modification history of data, thereby mitigating risks associated with tainted data supply chains and potential data breaches.
The economic landscape will experience significant shifts as companies ramp up investments in cybersecurity measures to safeguard AI data. This is set to increase operational costs but will also contribute to the expansion of cybersecurity industries specializing in AI data protection. Policies like those emphasized by CISA in their guidance on AI data security, which include advanced monitoring tools and validation strategies, will be essential ().
Public trust in AI technology, particularly in sectors like autonomous vehicles and healthcare diagnostics, may be challenged by increasing awareness of vulnerabilities such as data poisoning and drift. To counteract potential skepticism, educational initiatives tailored to inform the public about these vulnerabilities and the preventative strategies are crucial. By leveraging insights from articles on integrity threats in AI, businesses can enhance transparency and maintain social acceptance ().
Politically, AI data security is expected to become a key area of focus, prompting governments to introduce more stringent regulations to protect sensitive data and critical infrastructure. This could lead to international cooperation aimed at creating unified global standards for AI safety—to address cross-border data security threats effectively. The need for legal frameworks to ensure accountability and transparency in AI data processes is likely to grow, as pointed out in joint advisories regarding AI data security ().
Learn to use AI like a Pro
Get the latest AI workflows to boost your productivity and business performance, delivered weekly by expert consultants. Enjoy step-by-step guides, weekly Q&A sessions, and full access to our AI workflow archive.
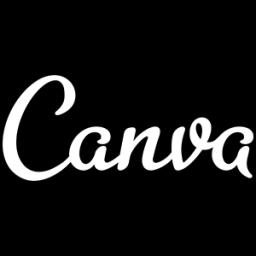
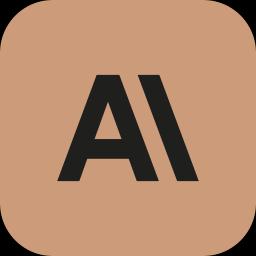
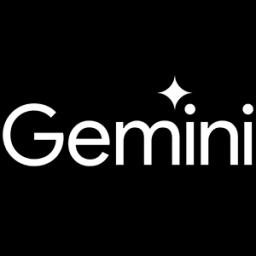
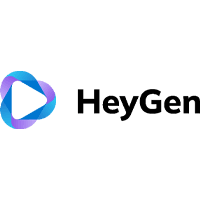
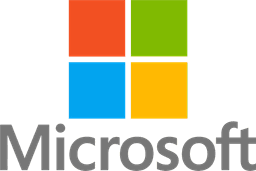
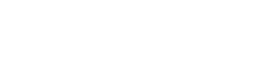
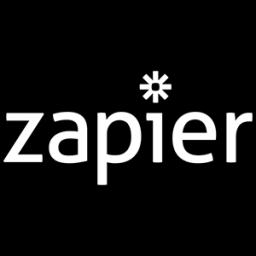
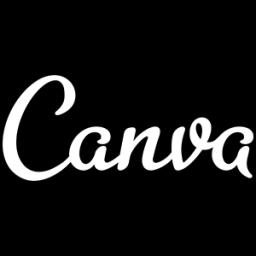
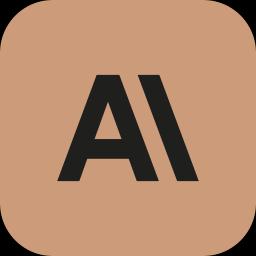
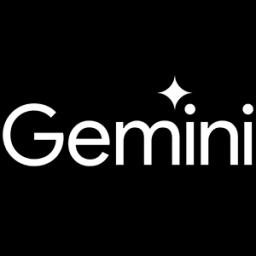
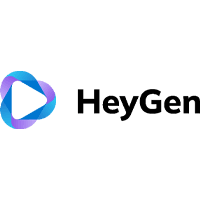
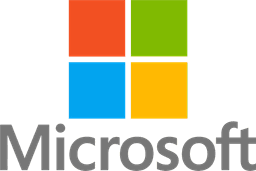
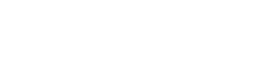
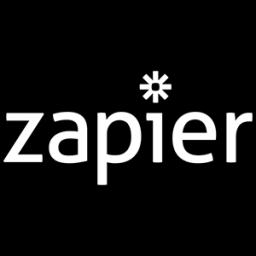
Conclusion: Strengthening AI Data Protections
In the realm of artificial intelligence, safeguarding data integrity is paramount to ensuring reliable and trustworthy outcomes. By strengthening AI data protections, organizations can build confidence in AI systems while mitigating the risks associated with data misuse and adversarial attacks. The Cybersecurity Information Sheet (CSI) underscores the necessity of implementing robust data security measures at every stage of the AI lifecycle—from data acquisition to deployment. By focusing on data provenance, integrity verification, and adaptive monitoring, organizations can shield their AI applications against emerging threats such as data poisoning and data drift. For more comprehensive guidelines, visit the CSI document.
As AI continues to be integrated into diverse sectors, the need for enhanced data security protocols becomes even more critical. Data protections not only preserve the functionality and accuracy of AI systems but also reinforce public trust in their applications, ranging from autonomous vehicles to healthcare solutions. Organizations must prioritize the establishment of comprehensive security frameworks, informed by documents like the Cybersecurity Information Sheet, to maintain competitive advantage and compliance with heightened regulatory demands.
Collaboration across industries and international borders will be essential to foster a unified approach to AI data security. Governments could lead the charge by enacting policies that mandate stringent data protection standards, encouraging innovation in cybersecurity solutions tailored for AI threats. As mentioned in the CSI guidance, continuous investment in security infrastructure and public education on AI vulnerabilities are fundamental to sustaining trust and enabling the future growth of AI technologies.