A Smarter Way to Train AI Agents
RAGEN Revolutionizes AI Training by Tackling the 'Echo Trap'
Last updated:

Edited By
Mackenzie Ferguson
AI Tools Researcher & Implementation Consultant
RAGEN, a new AI training system developed by experts from Northwestern, Microsoft, Stanford, and others, leverages the StarPO reinforcement learning framework to address persistent issues in AI training. This innovative approach helps AI agents learn from experience without falling into the repetitive 'Echo Trap,' offering a promising future for enterprise AI applications. Tested in symbolic environments like Bandit, Sokoban, and Frozen Lake, RAGEN's open-source platform is setting a new standard in AI reliability and efficiency.
Introduction to RAGEN: Reinforcement Learning Breakthrough
RAGEN marks a significant advancement in the field of reinforcement learning, offering a promising solution to the challenges that come with training reliable AI agents for real-world applications. Developed through a collaboration between leading institutions such as Northwestern University, Microsoft, Stanford, and the University of Washington, RAGEN is built on the innovative StarPO framework. This framework facilitates the learning of AI agents in complex, multi-turn interactive settings, which are essential for applications across various enterprise environments. The breakthrough with this technology lies in its ability to navigate the so-called "Echo Trap," a notorious issue in reinforcement learning where systems can become trapped in loops of repetitive and suboptimal behavior. By addressing this flaw, RAGEN paves the way for more adaptive and intelligent AI agents. For further details, you can explore the full context by visiting the [VentureBeat article](https://venturebeat.com/ai/former-deepseeker-and-collaborators-release-new-method-for-training-reliable-ai-agents-ragen/).
The introduction of RAGEN represents a pivotal change in how AI agents are trained, focusing on creating reliable outcomes in enterprise applications. The central component of this system, the StarPO framework, provides a robust environment for AI agents to learn through structured experiences. The researchers behind RAGEN aimed to overcome the limitations of traditional reinforcement learning models, which often fail in dynamic real-world scenarios.
Learn to use AI like a Pro
Get the latest AI workflows to boost your productivity and business performance, delivered weekly by expert consultants. Enjoy step-by-step guides, weekly Q&A sessions, and full access to our AI workflow archive.
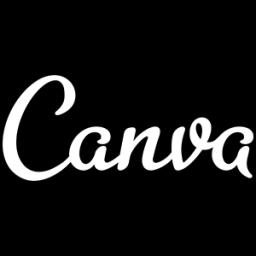
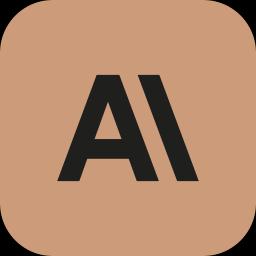
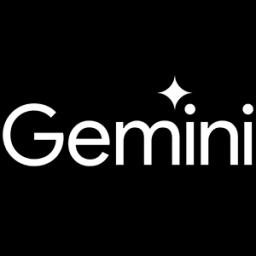
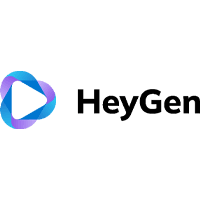
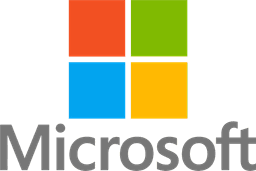
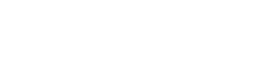
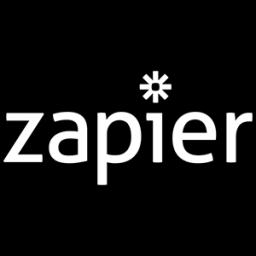
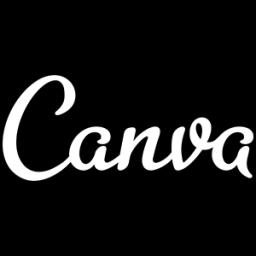
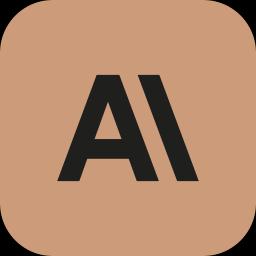
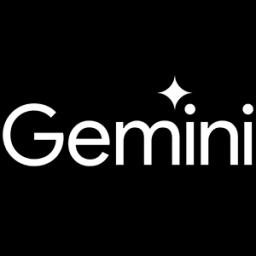
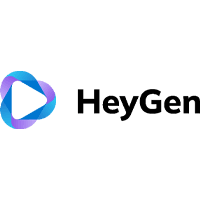
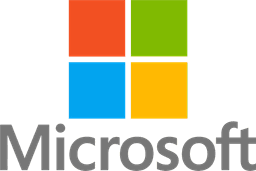
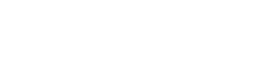
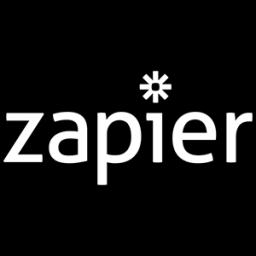
RAGEN’s development used Alibaba’s Qwen language models and demonstrated its capabilities across various symbolic environments including Bandit, Sokoban, and Frozen Lake. These environments were chosen to test different aptitudes - from risk-reward handling to problem-solving and adaptation, showcasing RAGEN's versatility. Furthermore, the stabilization brought by the StarPO-S variant significantly enhances the training process, providing a more consistent learning curve for AI agents, thus ensuring they are more suited for real-world applications.
As an open-source project, RAGEN not only integrates state-of-the-art research but also invites the community to contribute to its development and application, fostering a collaborative approach to tackle the challenges faced in AI training. With the backing of high-profile academic and corporate institutions, RAGEN is well-positioned to become a cornerstone in AI innovation. For more information, you can check out the detailed insights available on [VentureBeat](https://venturebeat.com/ai/former-deepseeker-and-collaborators-release-new-method-for-training-reliable-ai-agents-ragen/).
Understanding the "Echo Trap" in AI Training
The concept of the "Echo Trap" in AI training is a critical challenge within reinforcement learning environments. This phenomenon occurs when reinforcement learning agents favor a repetitive set of actions that initially yielded high rewards, even if those actions become less effective or suboptimal over time. One way to visualize the Echo Trap is to imagine a reinforcement learning model that finds a particular strategy beneficial in initial trials; over time, it overcommits to this strategy, often to its detriment in more dynamic and varied real-world scenarios. Such limitations can significantly hinder an agent's ability to adapt to new and unforeseen challenges, obstructing the evolution of its learning processes toward more optimized solutions. The repetitive behavior induced by the Echo Trap can thus stifle innovation and adaptation in AI agents, making this a subject of ongoing research and concern among AI developers and researchers.
How RAGEN Overcomes the Echo Trap
RAGEN, the innovative reinforcement learning system, presents a compelling breakthrough by addressing the notorious "Echo Trap" prevalent in many AI models. This trap occurs when agents repetitively engage in behaviors that initially seem rewarding but eventually become suboptimal, thereby stalling the learning process and hindering adaptive decision-making [source]. By utilizing the StarPO-S framework, RAGEN effectively sidesteps this issue through advanced techniques such as uncertainty-based rollout filtering, which enriches the agent's ability to differentiate between genuinely optimal actions and those that merely echo previous successes [source].
Learn to use AI like a Pro
Get the latest AI workflows to boost your productivity and business performance, delivered weekly by expert consultants. Enjoy step-by-step guides, weekly Q&A sessions, and full access to our AI workflow archive.
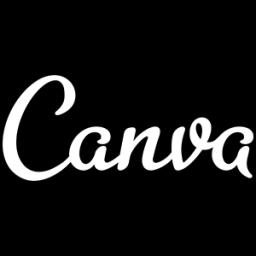
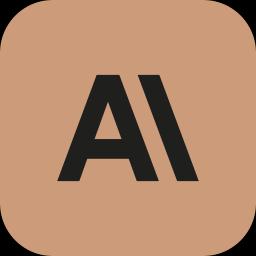
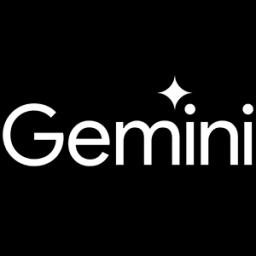
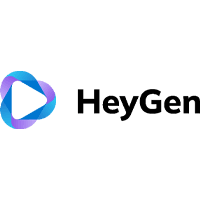
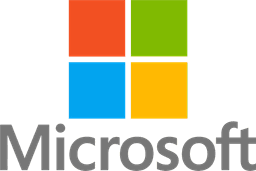
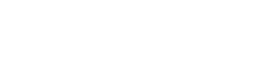
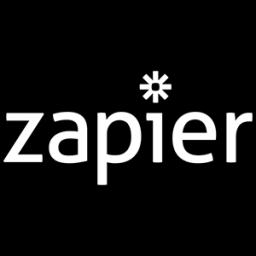
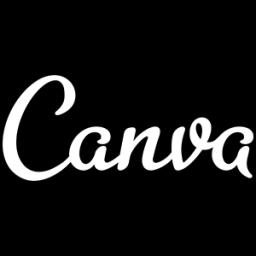
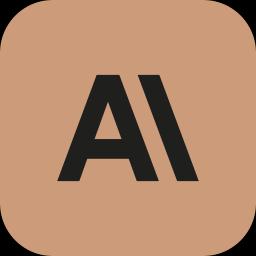
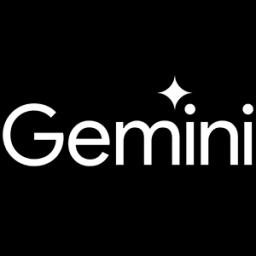
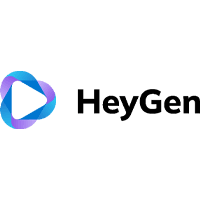
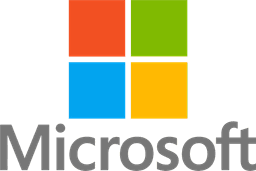
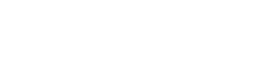
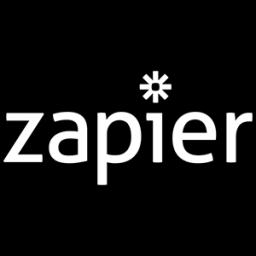
StarPO-S, the cornerstone of the RAGEN system, introduces robustness in training by removing the KL penalty and implementing asymmetric PPO clipping. These methodological innovations ensure that AI agents can explore a broader range of possible actions, thereby reducing the likelihood of falling into repetitive and unproductive behavior loops [source]. Moreover, by providing an open-source platform, RAGEN invites researchers and developers to contribute to and broaden the scope of AI's practical applications, fostering a collaborative environment that could herald unprecedented advancements in AI reliability and adaptability across various industries [source].
The effectiveness of RAGEN is evidenced through its application in testing environments such as Bandit, Sokoban, and Frozen Lake, each presenting unique challenges that simulate real-world scenarios. These symbolic environments test the agents' risk-reward assessment, problem-solving abilities, and adaptive planning skills, thereby serving as an invaluable testing ground for refining AI capabilities [source]. This diverse approach ensures that agents can transition from experimental frameworks to practical applications with greater reliability and effectiveness.
Testing RAGEN: Symbolic Environments Explained
RAGEN, a remarkable advancement in the realm of AI training, particularly emphasizes the importance of symbolic environments in crafting reliable AI agents. Symbolic environments, such as Bandit, Sokoban, and Frozen Lake, serve as meticulously designed arenas where AI agents can hone their skills in reasoning and strategic planning. These environments present varied challenges that require distinct approaches, from risk-reward reasoning in Bandit to the deterministic puzzle-solving in Sokoban, and the need for adaptive planning in Frozen Lake. By testing RAGEN within such diversified symbolic settings, developers aim to enhance the agents' abilities to adapt and learn from experiences, thereby addressing issues like the 'Echo Trap' where traditional reinforcement learning systems may falter by repeating suboptimal actions.
The rationale for deploying symbolic environments in testing RAGEN stems from the need to simulate real-world complexities within a controlled framework. These environments allow researchers to model dynamic and interactive scenarios, crucial for developing AI agents capable of nuanced decision-making. The Bandit problem, for example, emulates scenarios of uncertainty and decision-making under risk, while Sokoban requires the AI to engage in forward-thinking strategies. Frozen Lake, on the other hand, emphasizes the need for adaptability to changing conditions, mirroring real-world environments where AI systems must operate. Such rigorous testing not only validates RAGEN’s mechanisms but also exemplifies how AI can transition from theoretical proficiency to practical application.
Furthermore, the integration of symbolic environments in RAGEN’s testing phase underscores the significance of context in AI training. These environments not only test the AI's problem-solving skills but also capture the essence of contextual learning, which is vital for AI efficacy in real-world applications. The adaptability demonstrated by AI in these environments reflects on its potential utility in diverse sectors, including enterprise scenarios where well-rounded decision-making is paramount. Through this framework, RAGEN illustrates a path forward for AI systems to develop autonomy and resilience, equipping them to handle the complexities of interactive and multifaceted tasks in real-world settings. This approach opens new avenues for AI development, ensuring that systems are not only robust and reliable but also contextually intelligent and adaptable.
In essence, the use of symbolic environments provides a holistic platform to perfect AI agent training, capturing the intricate balance between simplicity and complexity required for effective learning. By harnessing this balance, RAGEN not only trains AI agents to perform tasks with precision but also endows them with the capacity to reason, a trait imperative for scaling the AI’s functionality to real-world enterprise applications. As developers continue to expand these environments, the potential for AI to seamlessly integrate into everyday tasks only becomes more plausible, marking a significant stride toward the future of intelligent agentic systems.
Learn to use AI like a Pro
Get the latest AI workflows to boost your productivity and business performance, delivered weekly by expert consultants. Enjoy step-by-step guides, weekly Q&A sessions, and full access to our AI workflow archive.
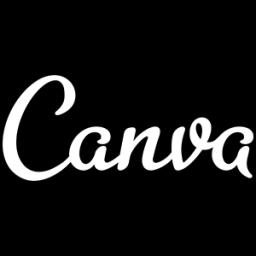
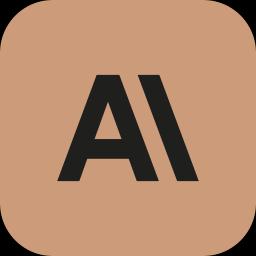
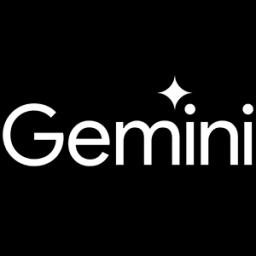
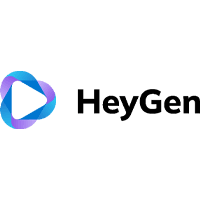
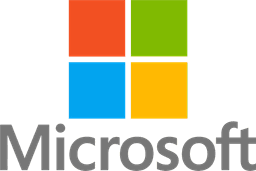
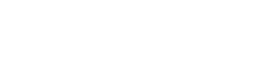
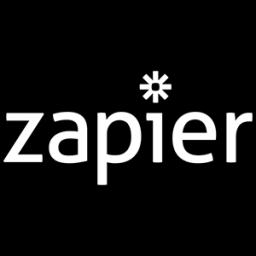
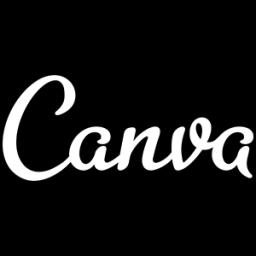
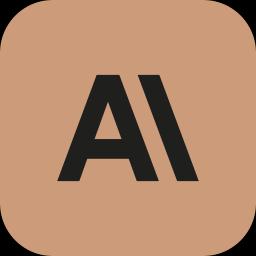
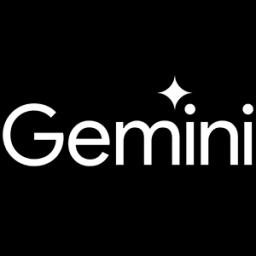
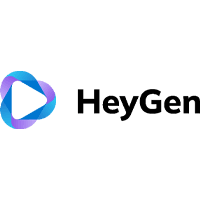
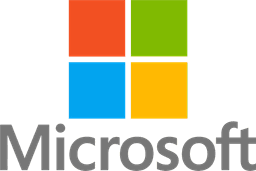
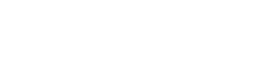
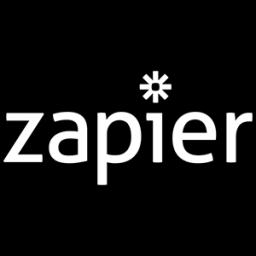
Real-World Applicability of RAGEN
RAGEN's real-world applicability is an exciting frontier for businesses looking to harness AI's potential in more sophisticated ways. By cleverly circumventing pitfalls like the 'Echo Trap,' RAGEN prepares AI agents for dynamic enterprise environments. This is achieved with its innovative use of the StarPO reinforcement learning framework, making AI's integration into complex tasks more reliable and adaptable. As AI technologies rapidly evolve, integrating such systems offers opportunities for streamlining operations and improving productivity across various sectors, from customer service to risk management. The open-source nature of RAGEN further enhances its applicability, inviting collaboration and customization by enterprises to tailor solutions specific to their needs. For instance, industries such as finance and logistics can leverage RAGEN to develop AI systems that are not only responsive but also capable of making informed decisions in real time, advancing the overall reliability of AI deployments. Further exploration and customization could indeed position RAGEN as an invaluable tool for enterprise-level AI solutions. Learn more about RAGEN's capabilities.
Open-Source Innovation: RAGEN's Framework
RAGEN represents a pivotal step in open-source innovation by offering a robust framework for training AI agents, thereby enhancing their reliability in real-world applications. Developed collaboratively by leading institutions such as Northwestern University, Microsoft, Stanford, and the University of Washington, RAGEN utilizes StarPO, a custom reinforcement learning framework . The framework is designed to enable AI agents to learn effectively in dynamic, interactive environments, overcoming the well-known "Echo Trap," where agents might fall into repetitive, suboptimal behavior patterns. With its open-source nature, RAGEN provides a collaborative platform for further innovation and development, offering researchers and developers the opportunity to build upon its foundation and contribute to its evolution.
The open-source nature of RAGEN is crucial in allowing a worldwide community of developers and researchers to contribute to its continuous improvement and adaptation to new challenges in AI training . This democratization of technology enables faster advancements as knowledge and resources are shared openly, fostering a collaborative environment that is essential for tackling complex problems. With experiments conducted across various environments, such as Bandit, Sokoban, and Frozen Lake, RAGEN's applicability extends beyond theoretical frameworks to practical, real-world scenarios where adaptability and decision-making are crucial.
By addressing critical issues such as the "Echo Trap," RAGEN sets a new standard for the development of AI agents capable of high-order reasoning and autonomous learning. This framework is vital for applications necessitating reliable and consistent performance in enterprises and various industries . Through its reinforcement learning advancements, it empowers AI agents not only to act but also to understand the context of their actions in complex situations. As the project evolves, it promises to simplify the integration of AI in enterprise systems, making them more effective and responsive to user needs.
Expert Insights on RAGEN's Impact
RAGEN, the innovative system announced by former DeepSeeker collaborators, heralds a significant shift in the development of reliable AI agents for enterprise-level applications. This new framework, which incorporates advanced reinforcement learning techniques, particularly through its custom StarPO framework, is a breakthrough in tackling common issues faced by AI agents, such as the notorious "Echo Trap." This condition causes AI systems to become trapped in loops of repetitive, ineffective behaviors. By understanding and addressing these challenges, RAGEN offers a promising pathway toward the development of AI that is not only more reliable but also more adaptive to dynamic environments. With tests conducted across various symbolic environments, such as Bandit, Sokoban, and Frozen Lake, RAGEN’s capabilities have been rigorously assessed, paving the way for potential breakthroughs in real-world applications (source).
Experts have noted that RAGEN’s approach significantly contributes to the broader integration of AI systems in enterprise environments. A key strength of RAGEN’s methodology is its ability to manage complete decision sequences and leverage cumulative rewards, which are crucial for developing AI agents capable of nuanced reasoning rather than mere task completion. This is particularly relevant in industries requiring sophisticated analysis and operational functionality, with organizations like Alibaba testing these elements using robust language models. Furthermore, the open-source nature of the project invites substantial community collaboration, sparking further innovations and adaptations across various sectors (source).
Learn to use AI like a Pro
Get the latest AI workflows to boost your productivity and business performance, delivered weekly by expert consultants. Enjoy step-by-step guides, weekly Q&A sessions, and full access to our AI workflow archive.
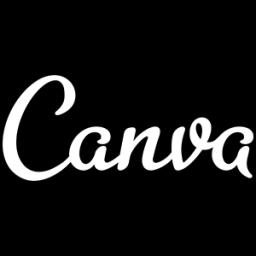
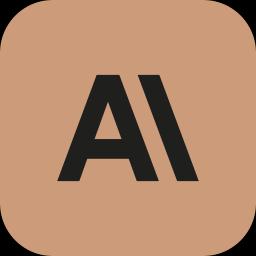
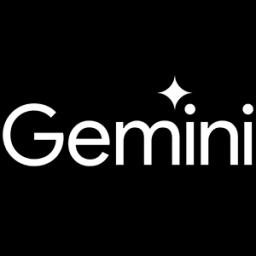
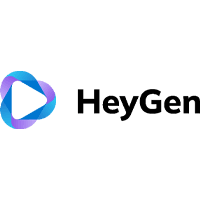
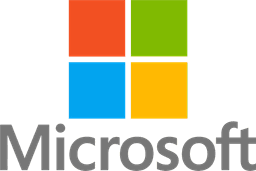
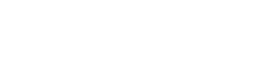
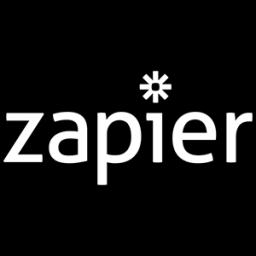
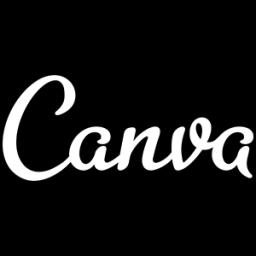
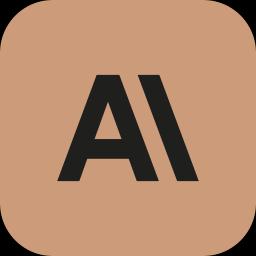
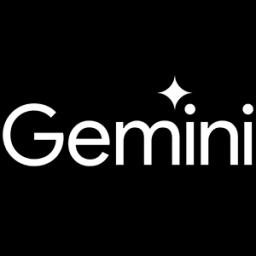
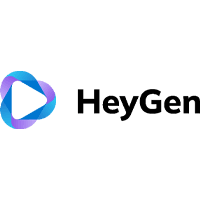
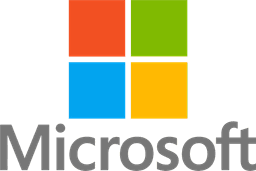
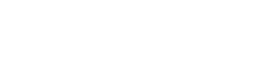
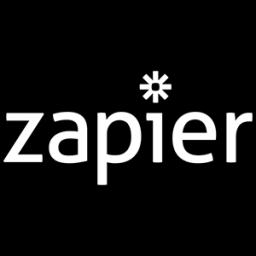
While RAGEN shows promising strides in ensuring AI reliability, experts caution that its transferability to complex, real-world applications remains under scrutiny. The practice of refining reward shaping to maintain task complexity while avoiding training collapse is crucial yet challenging. Particularly, in scenarios like multi-turn dialogues in customer service or invoice processing, further customization of environments and reward systems might be necessary. The ongoing focus on maintaining balance between explicit agent-driven reasoning and adaptive responses is pivotal if RAGEN is to successively scale and generalize beyond its current testing grounds (source).
Public Reactions to RAGEN's Development
The development of RAGEN, an innovative reinforcement learning framework, has sparked a wave of public interest and discussion. As it aims to improve the reliability of AI agents in real-world applications, netizens on platforms like X have been vibrant with opinions ranging from optimism to cautious scrutiny. Enthusiasts commend RAGEN's approach to overcoming the 'Echo Trap,' a common challenge in AI training, which it addresses through its unique StarPO framework. The open-source nature of the project is another aspect that garners widespread support, as it promises greater accessibility and potential for collaborative innovation ().
Despite the excitement, there is a palpable thread of skepticism among the public, particularly regarding RAGEN's ability to replicate its controlled environment successes in the unpredictable landscape of real-world applications. Concerns revolve around whether the same methodologies can be scaled and implemented effectively outside of laboratory conditions. The need for new environmental designs and reward functions tailored to specific enterprise needs raises questions about the system's applicability and versatility in various industries ().
Discussions about RAGEN's broader implications also feature prominently. Proponents see it as a potential game-changer across different sectors, especially in streamlining processes and enhancing the efficiency of AI-led initiatives. However, critics emphasize the importance of additional research and development before RAGEN can be fully integrated into everyday enterprise operations. This cautious approach reflects a broader understanding of the complexities involved in scaling AI technologies for practical use, stressing the balance between innovation and practical feasibility ().
The mixed reactions underscore a general anticipation for what RAGEN could eventually achieve. While it is lauded for tackling longstanding issues in AI training stability and reliability, the pathway to tangible deployment in complex, real-world scenarios is still being mapped. This ongoing dialogue among technology enthusiasts, experts, and the general public signifies not just interest, but also the critical examination needed to responsibly usher in advanced AI systems into broader use ().
Future Implications: Economic, Social, and Political Perspectives
As the advent of advanced AI systems like RAGEN unfolds, the economic implications could be profound. Increased AI reliability, as evidenced by the new STARPO-S framework, may catalyze economic growth by enhancing productivity and reducing costs across various sectors, including finance and manufacturing. However, this technological advancement brings with it the challenge of workforce displacement, as more tasks become automated. Societies will need to implement retraining programs to navigate this shift successfully. With RAGEN being open-source, its accessibility could spur unprecedented innovation, further impacting the economic landscape [VentureBeat](https://venturebeat.com/ai/former-deepseeker-and-collaborators-release-new-method-for-training-reliable-ai-agents-ragen/).
Learn to use AI like a Pro
Get the latest AI workflows to boost your productivity and business performance, delivered weekly by expert consultants. Enjoy step-by-step guides, weekly Q&A sessions, and full access to our AI workflow archive.
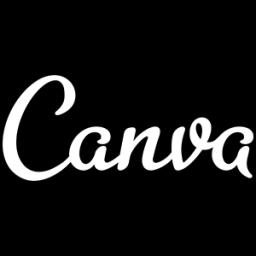
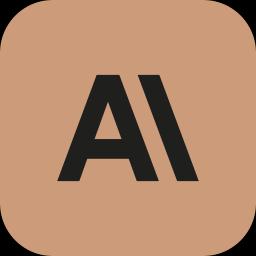
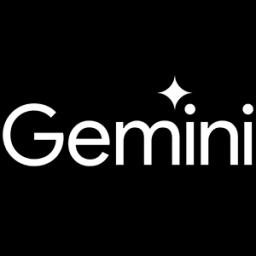
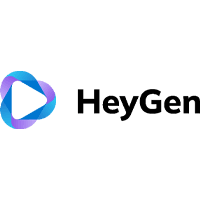
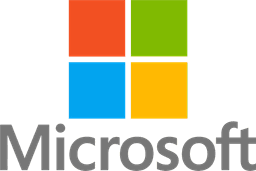
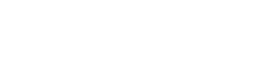
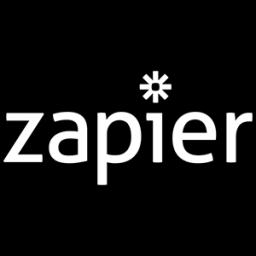
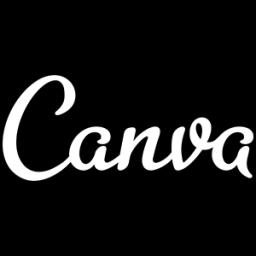
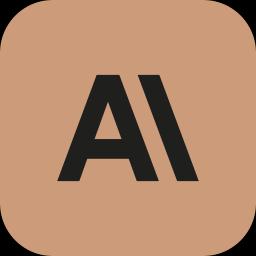
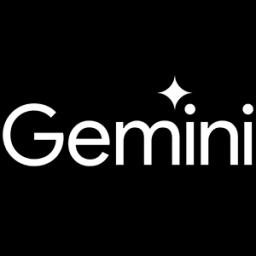
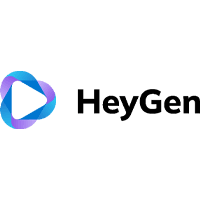
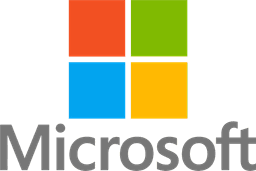
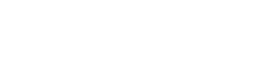
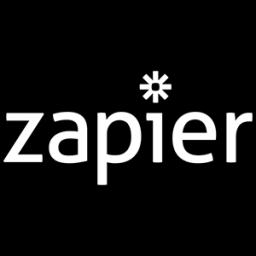
On the social frontier, the rise of RAGEN-trained AI agents could redefine access to essential services, potentially reducing human errors and streamlining efficiency. Yet, there remains the crucial task of addressing inherent biases in AI, which could worsen existing disparities if left unchecked. Ensuring fairness and mitigating bias are paramount to fostering public trust in AI technologies. While increased AI reliability could enhance societal trust, it demands vigilant oversight to prevent misuse and ensure equitable outcomes [VentureBeat](https://venturebeat.com/ai/former-deepseeker-and-collaborators-release-new-method-for-training-reliable-ai-agents-ragen/).
Politically, the emergence of RAGEN represents a call to action for regulatory evolution. Governments may have to establish new frameworks addressing ethical concerns such as job displacement and algorithmic bias. The global nature of AI applications necessitates international collaboration to develop consistent standards. Furthermore, RAGEN’s open-source nature amplifies both innovation and the potential for misuse, pressing the need for sophisticated regulatory mechanisms. Transparency within AI systems will likely invite public scrutiny, demanding accountability and perhaps reshaping governmental approaches to technology oversight [VentureBeat](https://venturebeat.com/ai/former-deepseeker-and-collaborators-release-new-method-for-training-reliable-ai-agents-ragen/).
Despite the promising advancements, uncertainty looms over RAGEN's long-term societal impact. Its effectiveness in more complex, real-world settings remains under research, and scaling these technologies while preserving human oversight is a challenge yet to be resolved. Further investigation into potential biases and economic ramifications is crucial to understand how such intelligent systems will reshape labor markets and societal structures in the years to come [VentureBeat](https://venturebeat.com/ai/former-deepseeker-and-collaborators-release-new-method-for-training-reliable-ai-agents-ragen/).
Conclusion: The Path Ahead for RAGEN
As RAGEN continues to develop, its open-source nature presents both opportunities and challenges for the AI community. By allowing researchers and developers worldwide to contribute, this open-access model facilitates rapid innovation and accelerated growth in AI technology. However, the same openness also demands stringent ethical considerations and robust mechanisms to prevent misuse. The ongoing efforts to improve RAGEN's adaptive capabilities ensure that it remains a pivotal tool for training reliable AI agents, ready to tackle problems in dynamic environments.
Looking forward, the path ahead for RAGEN encompasses a dual focus: enhancing its practical application in real-world enterprise scenarios and refining its ability to adapt across diverse environments. As more businesses seek customized AI solutions, RAGEN's framework offers a flexible platform for developing specialized applications. This adaptability could redefine AI's role across industries, driving efficiency and fostering innovation. The transition from conceptual advancements to tangible benefits in operational contexts remains a focal point for future development.
RAGEN's potential impact on enterprises is profound, especially in sectors that require reliable automation. Its ability to sidestep the common pitfalls of traditional reinforcement learning, such as the Echo Trap, positions it uniquely to offer robust, autonomous agents. For businesses, this means not only reduced operational costs through automation but also increased reliability and performance in AI-driven tasks. As RAGEN scales, its role in enhancing machine learning models could significantly alter the competitive landscape, creating new opportunities and challenges for organizations.
Learn to use AI like a Pro
Get the latest AI workflows to boost your productivity and business performance, delivered weekly by expert consultants. Enjoy step-by-step guides, weekly Q&A sessions, and full access to our AI workflow archive.
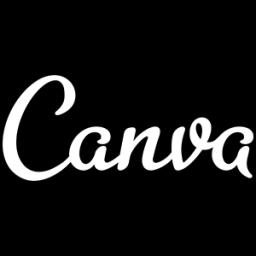
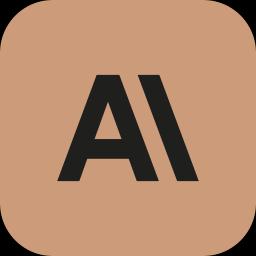
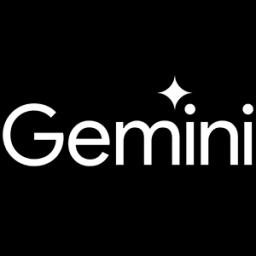
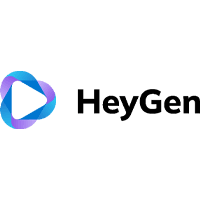
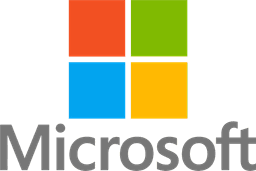
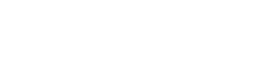
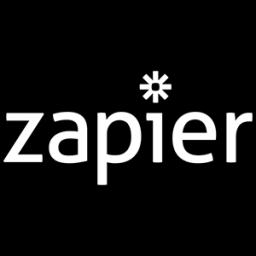
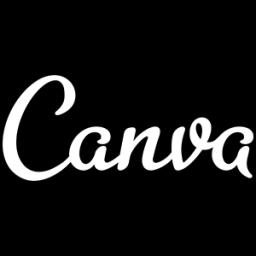
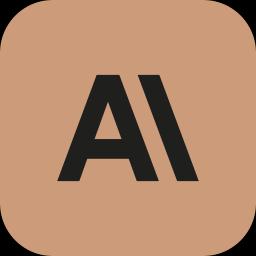
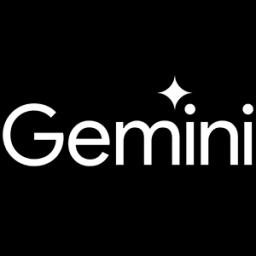
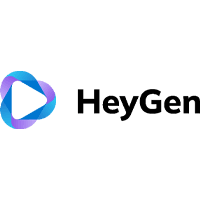
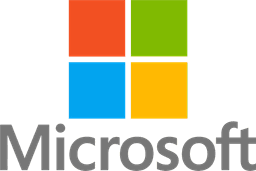
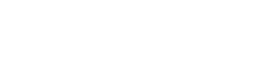
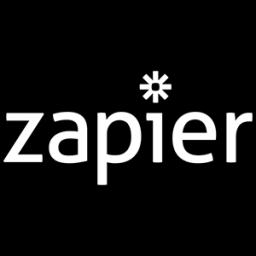
While RAGEN's future appears promising, it is not without uncertainties. The application of AI agents in complex, unpredictable environments will require ongoing research and refinement. Aspects like bias, ethical deployment, and human oversight are crucial areas that need continuous attention. Ensuring that RAGEN remains responsive and ethical is key to achieving its full potential, especially as it moves closer to integration with real-world systems. Collaborative efforts among academia, industry, and policymakers will be essential to navigate these challenges effectively.
As RAGEN's developers press forward, testing its applications across various symbolic environments, such as Bandit and Sokoban, will remain crucial. These tests will help establish benchmarks for the system's performance and its viability in enterprise environments. Furthermore, RAGEN's impact extends beyond technology; its implications for economic, social, and political landscapes are vast. By fostering trust and reliability in AI, RAGEN represents a critical step in the broader acceptance and integration of AI technologies into everyday enterprise operations.