Databricks Unleashes TAO's Potential
Self-Improving AI: Databricks' TAO Revolutionizes AI Model Performance
Last updated:

Edited By
Mackenzie Ferguson
AI Tools Researcher & Implementation Consultant
Databricks introduces TAO (Test-time Adaptive Optimization), a powerful technique to enhance AI model performance with imperfect data. By leveraging 'best-of-N' selection and a unique reward model, TAO refines AI models with synthetic data, outperforming major competitors like OpenAI on benchmark tests. Discover how this groundbreaking approach could democratize AI and reshape industries.
Introduction to Databricks' TAO
The introduction of TAO is a response to one of the core challenges in machine learning: the scarcity of clean, well-labeled data. Databricks has developed an innovative way to bypass this hurdle, making AI more accessible and effective across various domains. By leveraging TAO, companies no longer need to rely solely on extensive data labeling processes that are both time-consuming and resource-intensive. This new technique, shared openly by Databricks, has sparked discussions on its implications for the future of AI model training and the potential it holds for democratizing artificial intelligence worldwide.
The Challenge of Imperfect Data in AI
In the evolving world of Artificial Intelligence (AI), the utilization of imperfect data poses significant challenges for businesses striving to integrate AI solutions into their operations. Imperfect data, often characterized by noise, inaccuracies, and incomplete datasets, can severely hinder the performance and reliability of AI models. A prominent example of innovation in this domain is Databricks' introduction of Test-time Adaptive Optimization (TAO), which is specifically designed to enhance AI model performance utilizing such imperfect data. By employing TAO, Databricks aims to refine AI models' ability to deliver accurate and reliable outcomes despite the inherent limitations of their training data. As outlined in their recent breakthrough, TAO is particularly pivotal for companies that face difficulties in obtaining high-quality, labeled data for fine-tuning AI models [source].
Learn to use AI like a Pro
Get the latest AI workflows to boost your productivity and business performance, delivered weekly by expert consultants. Enjoy step-by-step guides, weekly Q&A sessions, and full access to our AI workflow archive.
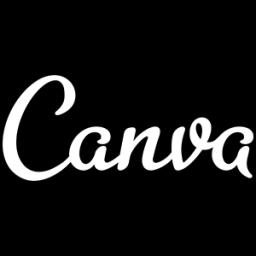
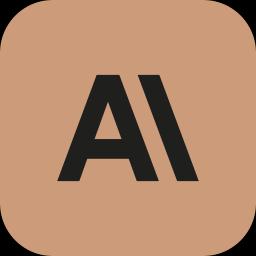
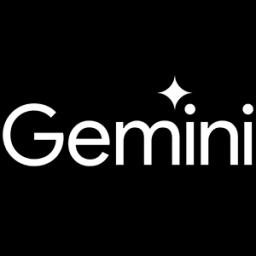
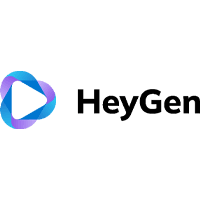
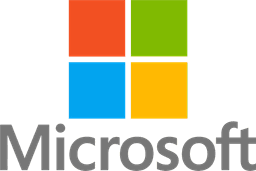
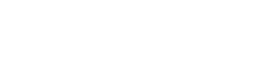
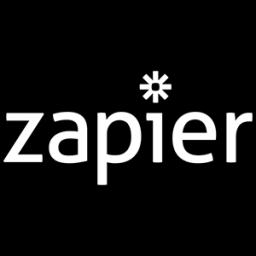
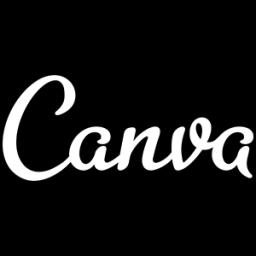
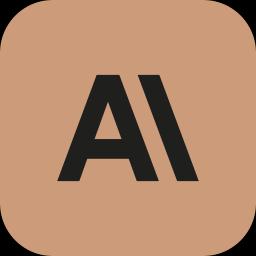
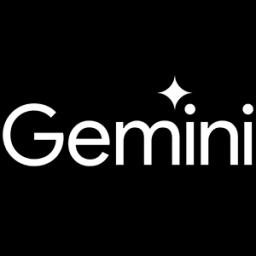
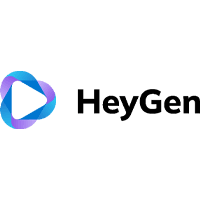
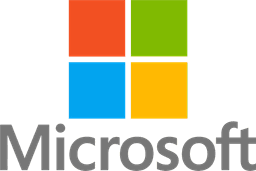
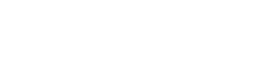
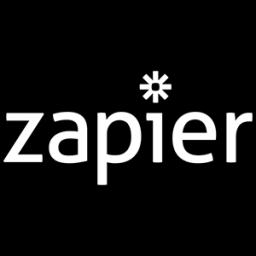
The core challenge with imperfect data lies in its impact on model training and subsequent output accuracy. Traditional AI models often rely on clean, well-labeled datasets to learn efficiently, but many organizations struggle to procure such gold-standard data. This lack of clean data impels the need for innovative techniques like TAO, which utilizes "best-of-N" outputs and a reward model (DBRM) to optimize model performance. These methods offer a novel way of synthetically generating training data, thus overcoming the inadequacies of the available data. The application of TAO has already shown considerable promise, as demonstrated by Databricks with their enhancement of Meta's Llama 3.1B model using the FinanceBench benchmark [source].
Another intriguing aspect of addressing imperfect data is the integration of reinforcement learning and synthetic data generation techniques. While the specific implementation by Databricks through TAO is unique, the underlying concepts have broader implications across the AI industry. The use of reinforcement learning as part of TAO signifies a shift towards more adaptive and self-improving models, even when initial training data is less than ideal. This innovative approach not only boosts model accuracy and performance but also reduces the dependency on exhaustive data labeling, a commonly resource-intensive process. Consequently, Databricks' strategy demonstrates how leveraging imperfect data can revolutionize model development, particularly with its capacity to refine smaller AI models to compete effectively with their larger counterparts [source].
How TAO Works: A Technical Overview
TAO, or Test-time Adaptive Optimization, represents a novel approach by Databricks to address one of the most prevalent challenges in AI development: the scarcity of clean and labeled training data. This technique enhances the adaptive capabilities of AI models by generating synthetic data during the testing phase, improving outcomes even when the input data might be imperfect. Unlike traditional methods that significantly rely on heavily pre-processed data, TAO dynamically adapts by choosing the best output from a set of options, driven by a reward model known as DBRM. This model, trained on human preferences, allows TAO to refine and enhance AI models such as Meta's Llama 3.1B efficiently, as demonstrated in various benchmarks including FinanceBench, where it has outperformed competitive models like OpenAI's GPT-4o and o3-mini. By reducing the reliance on perfect data, Databricks is not only pushing the boundaries of AI fine-tuning but also providing a cost-effective solution for optimizing AI deployments across diverse industries. For additional insights, you can explore the detailed analysis by Wired on the functionality and implications of TAO here.
The "best-of-N" methodology is central to how TAO functions, offering a selection mechanism that outputs multiple predictions or solutions at a test-time scenario. The best output is selected through the use of a reward model that assesses these outputs based on previously gathered human preference data. This innovative approach not only overcomes limitations posed by inadequate data but also optimizes AI performance in a resource-efficient manner. Databricks' implementation of TAO presents a strategic shift towards creating more robust and flexible AI systems, paving the way for groundbreaking applications in sectors like healthcare and finance, where decision-making accuracy is vital. This strategic capability to 'learn as it operates' is pivotal, making it a key differentiator in the competitive AI market landscape. Explore more about how TAO elevates AI tuning here.
Learn to use AI like a Pro
Get the latest AI workflows to boost your productivity and business performance, delivered weekly by expert consultants. Enjoy step-by-step guides, weekly Q&A sessions, and full access to our AI workflow archive.
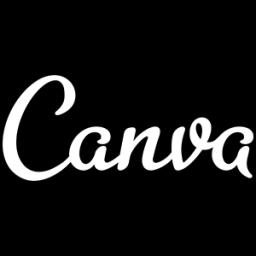
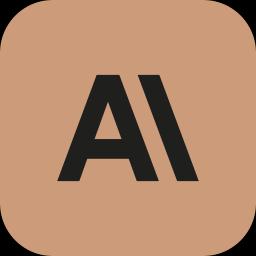
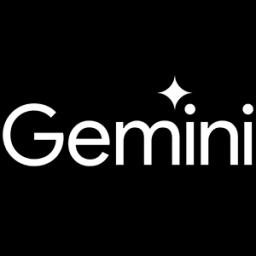
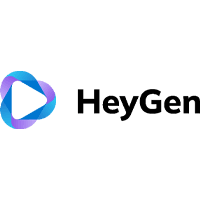
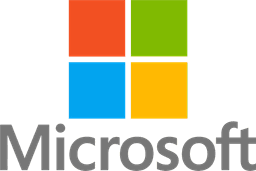
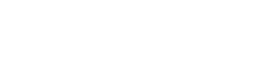
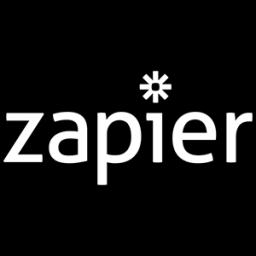
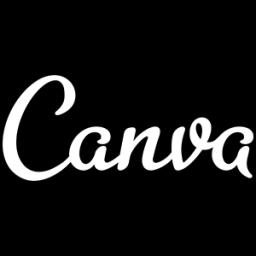
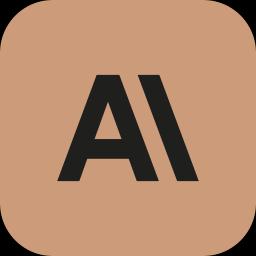
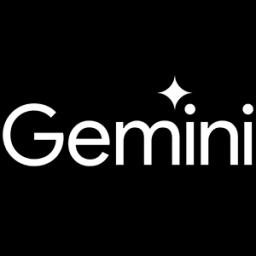
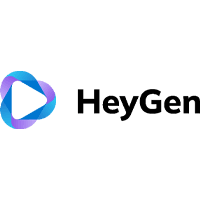
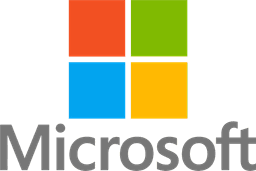
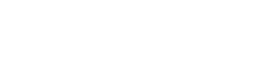
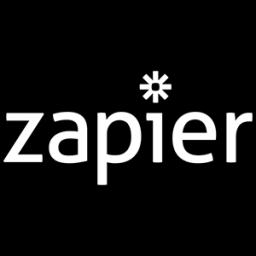
The efficiency of TAO is further substantiated by its performance records. In a competitive evaluation on the FinanceBench benchmark, it has showcased its superiority by enhancing Meta's Llama 3.1B model to outperform leading AI models like OpenAI's GPT-4o. Enabling such enhancements without the need for extensive new datasets limits resource expenditure while boosting the model's efficacy in real-world applications - a feat essentially marked as a game-changer for AI development. The overarching benefits witnessed from employing TAO stretch beyond mere technical improvements, hinting at a larger potential for democratizing AI accessibility. These implications are deeply intertwined with lowering operational costs and raising the bar for smaller organizations to achieve comparable AI-driven results. For more detailed information about TAO's performance and optimization capabilities, refer to this comprehensive article by Wired here.
Comparison with Other AI Techniques: GRPO and LoRA
When comparing TAO to other AI techniques such as GRPO and LoRA, several key differences emerge in their approach and applicability. GRPO, or Gradient-based Reinforcement Policy Optimization, is primarily focused on improving the efficiency and speed of reinforcement learning models by optimizing policies in high-dimensional space. In contrast, TAO (Test-time Adaptive Optimization), as developed by Databricks, emphasizes enhancing AI models when dealing with imperfect data by generating synthetic training datasets. These datasets are refined using a reward model, leading to improved model performance on initial trials. LoRA, or Low-Rank Adaptation, is another distinct technique that deals with efficient adaptation of large-scale language models by reducing the number of trainable parameters. While LoRA is effective in fine-tuning without excessive computational costs, TAO is unique in its ability to self-optimize AI models without requiring traditional data labeling methods [0](https://www.wired.com/story/databricks-has-a-trick-that-lets-ai-models-improve-themselves/).
TAO's innovative approach of "best-of-N" selection distinguishes it from GRPO and LoRA, by selecting the best outputs from multiple model predictions to create superior synthetic data. This process, which incorporates human preference through a reward model (DBRM), provides a robust method to enhance model accuracy with less reliance on extensive data labeling. Unlike GRPO's emphasis on policy optimization for reinforcement learning and LoRA's parameter reduction focus, TAO finds a unique intersection, aiming to optimize models in real-time application scenarios by leveraging existing data imperfections rather than solely refining the algorithmic model itself [0](https://www.wired.com/story/databricks-has-a-trick-that-lets-ai-models-improve-themselves/).
Additionally, the applicability of TAO demonstrates its versatility across various domains, particularly where data quality poses significant challenges. While models like GRPO are best suited for environments where dynamic decision-making is paramount, and LoRA shines in resource-constrained scenarios requiring efficient model scaling, TAO's strength lies in its adaptability to enhance models based on available data conditions rather than computational settings alone. This positions TAO as a pioneer for organizations looking to refine AI models with limited access to pristine, well-curated datasets, providing potentially more realistic and robust solutions in less-than-ideal data environments [0](https://www.wired.com/story/databricks-has-a-trick-that-lets-ai-models-improve-themselves/).
Real-World Applications of TAO
Test-time Adaptive Optimization (TAO), a revolutionary technique pioneered by Databricks, is rapidly finding its way into various real-world applications, transforming how industries utilize AI. This novel method enhances AI model performance by generating synthetic data, which is particularly beneficial for companies struggling with messy or unlabeled datasets. By leveraging TAO, businesses can skip the labor-intensive process of data labeling, making AI more accessible and efficient for tailored industry tasks. For instance, TAO's application in the healthcare sector has notably improved patient management systems by refining predictions based on sparse and noisy data. These advancements allow healthcare professionals to rely on AI for critical decision-making, enhancing patient outcomes and operational efficiency .
Moreover, TAO's integration into financial institutions as part of their AI strategy underscores its versatility and effectiveness. By deploying TAO, financial firms have seen significant improvements in fraud detection algorithms, which now adaptively learn and respond to suspicious activity with greater precision. These improvements not only bolster security measures but also reduce false positives, ensuring smoother operations and heightened customer satisfaction . The use of TAO has demonstrated a substantial enhancement in model reliability and efficiency, as evidenced by its success in optimizing Meta's Llama model, where it surpassed notable benchmarks previously held by leading AI platforms.
Learn to use AI like a Pro
Get the latest AI workflows to boost your productivity and business performance, delivered weekly by expert consultants. Enjoy step-by-step guides, weekly Q&A sessions, and full access to our AI workflow archive.
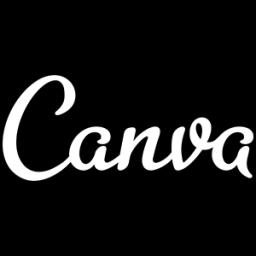
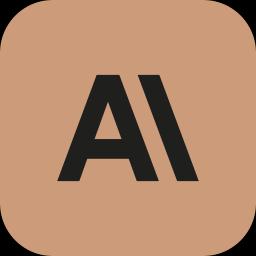
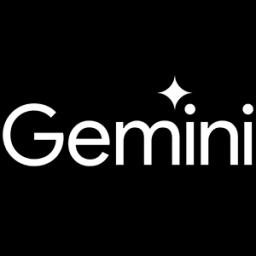
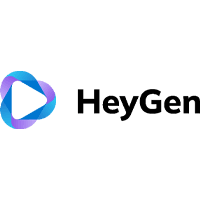
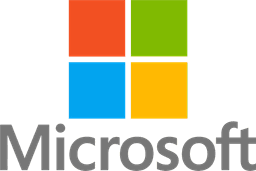
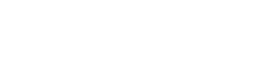
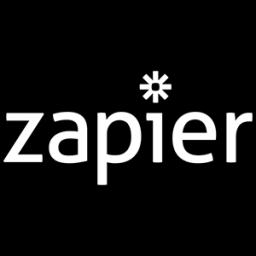
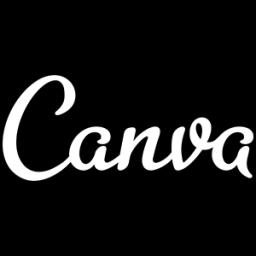
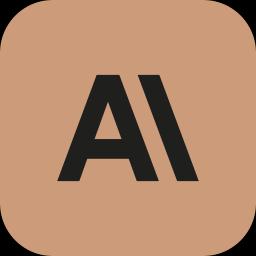
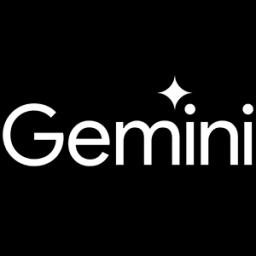
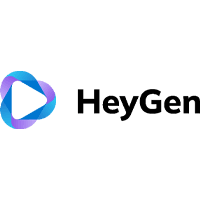
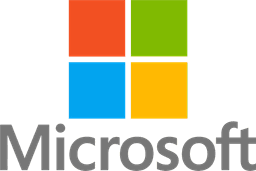
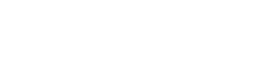
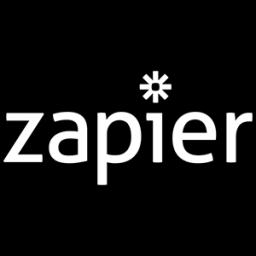
As industries continue to explore AI-driven solutions, TAO emerges as a powerful tool that could fundamentally change operational paradigms. The education sector, for example, benefits from TAO by customizing e-learning platforms that adapt in real-time to each student’s learning pace and style, thus promoting a more personalized educational experience. This adaptability not only caters to individual needs but also increases engagement and learning outcomes. Similarly, in retail and customer service, TAO’s implementation helps businesses predict consumer behavior more accurately, enhancing marketing strategies and customer relations . As the technology continues to mature, its potential to drive innovation across various sectors remains vast, signaling a transformative era for AI applications globally.
Performance Improvement: Case Study with Meta's Llama Model
The application of Databricks' TAO (Test-time Adaptive Optimization) has significantly improved Meta's Llama model's performance, particularly in specialized benchmark tests like FinanceBench, where it rendered superior results compared to some of the leading AI models, including OpenAI's GPT-4o. This accomplishment underscores the efficacy of TAO in refining AI models even when dealing with imperfect data. With TAO, the use of the "best-of-N" selection strategy and the incorporation of human-trained reward models, such as DBRM, to create synthetic training data, has provided a robust means of enhancing existing AI models without relying solely on high-quality, labeled data sets. This innovative approach not only enhances the Llama model's ability to provide accurate results rapidly but also establishes a new standard for AI performance improvement strategies. The involvement of synthetic data significantly aids in overcoming challenges associated with traditional fine-tuning, ensuring more models achieve higher accuracy and precision out of the gate .
TAO's implementation has proven to be particularly beneficial in real-world applications where model reliability and performance are critical. An example can be seen in the health-tracking app realm, where the reliability of AI models is paramount for delivering medically accurate data. TAO has enhanced the reliability of such models, offering solutions that were previously unattainable with underperforming AI. The effectiveness of TAO is evidenced by the considerable performance improvements in Meta's models, which have been shown to outperform several other renowned AI models on specific benchmarks. This demonstrates not only TAO's potential for immediate performance improvements but also its broader applicability in industries where precision and accuracy are non-negotiable .
The success attributed to TAO also hints at a paradigm shift in AI development strategies, where the focus moves from solely relying on traditional data labeling techniques to exploring innovative methods like synthetic data generation. By achieving a substantial performance boost with the Meta's Llama models, Databricks has set a precedent for AI evolution, encouraging other sectors to leverage synthetic data and advanced reinforcement learning techniques to refine and enhance AI models. These advancements are not only reducing the dependency on extensive and often costly data labeling processes but are also providing a pathway towards more agile and adaptive AI systems that can self-improve and adapt to new challenges and environments efficiently .
Potential Limitations and Challenges of TAO
Despite its promise, the Test-time Adaptive Optimization (TAO) technique developed by Databricks poses several limitations and challenges. One major concern is the unpredictability inherent in reinforcement learning, which is a core component of TAO. Models leveraging reinforcement learning, such as those enhanced by TAO, can sometimes produce unexpected results, necessitating careful monitoring and management to ensure reliability and accuracy. This unpredictability might hinder the adoption of TAO in industries where consistent and dependable AI performance is critical [source].
Moreover, the synthetic data generation process, essential for TAO's functionality, may also introduce challenges. Although synthetic data can enhance model performance without the need for extensive real-world data, it requires sophisticated techniques to ensure the data closely resembles actual scenarios. Any deviations could lead to models being trained on imperfect representations, potentially skewing results. Additionally, because TAO relies heavily on synthetic data precision, any shortcomings in synthetic data generation could negatively impact its overall effectiveness, raising concerns about its scalability across different applications and datasets [source].
Learn to use AI like a Pro
Get the latest AI workflows to boost your productivity and business performance, delivered weekly by expert consultants. Enjoy step-by-step guides, weekly Q&A sessions, and full access to our AI workflow archive.
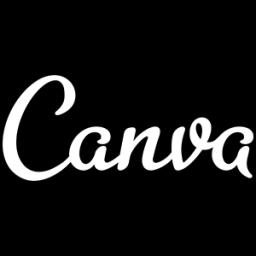
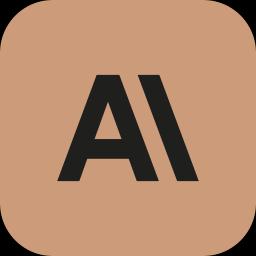
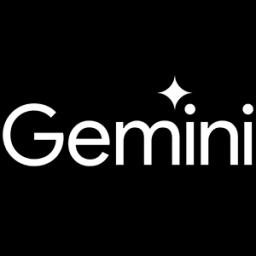
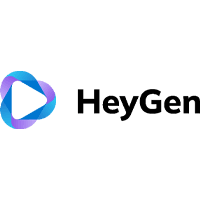
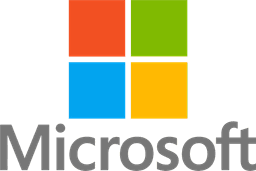
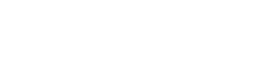
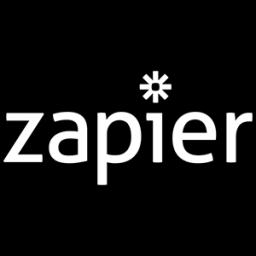
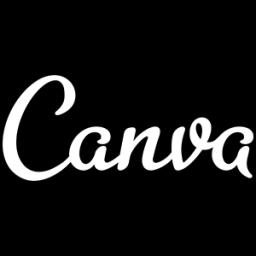
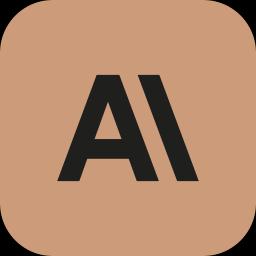
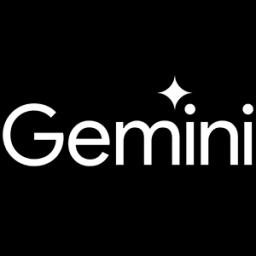
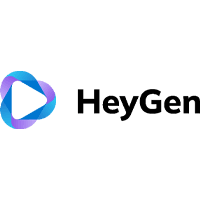
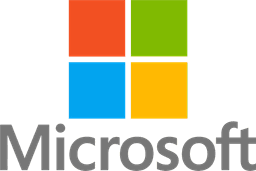
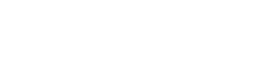
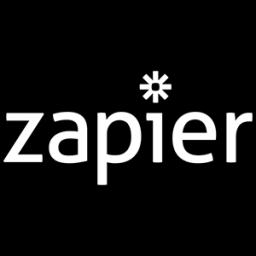
Another limitation of TAO is its resource-intensive nature. Utilizing 'best-of-N' selection and maintaining models capable of refining themselves requires substantial computational resources. This demands significant investment in infrastructure, which may not be feasible for smaller enterprises. Organizations with limited access to computational power may struggle to implement TAO effectively, restricting its benefits to larger businesses with adequate resources. Such requirements may limit TAO's democratizing potential, as the barrier of entry could remain high for under-resourced companies [source].
Furthermore, while TAO has been shown to improve model performance significantly, issues related to data privacy and governance remain unresolved. With TAO's reliance on large volumes of synthetic data and reinforcement learning, there are potential risks concerning data manipulation and privacy violations. Ensuring data integrity and compliance with privacy regulations is paramount as AI technology becomes more prevalent. Developing robust data governance frameworks is essential to address these concerns and foster trust in TAO's implementation across various sectors [source].
Public and Expert Reactions to TAO
The introduction of TAO (Test-time Adaptive Optimization) by Databricks has garnered a range of reactions from both the public and experts in the field of AI. On the public front, the reception is a mix of curiosity and skepticism. Many are intrigued by the claims of TAO's potential to enhance AI model performance without extensive labeled data, which could substantially lower costs and expedite deployment times for AI solutions. News outlets like [Wired](https://www.wired.com/story/databricks-has-a-trick-that-lets-ai-models-improve-themselves/) have highlighted the innovative nature of TAO, suggesting it might lead to a shift in how AI is applied across various industries. However, some commentators on platforms such as LinkedIn have expressed reservations about whether TAO truly represents a novel approach or if it merely builds on existing techniques like GRPO and LoRA, despite the compelling results demonstrated with Meta's Llama 3.1B model.
Expert opinions are also divided, though largely positive. Christopher Amato from Northeastern University has expressed cautious optimism. He acknowledges the scalability TAO offers in data labeling and model improvement, but he also warns about the inherent unpredictability of reinforcement learning systems like those underpinning TAO. His insights indicate a need for careful oversight, particularly when such systems are deployed in environments where decision-making accuracy is paramount. Brandon Cui from Databricks emphasizes TAO's potential to revolutionize how enterprises optimize AI models, underscoring its ability to reduce the dependence on comprehensive data labels, a process traditionally perceived as resource-intensive.
Despite some skepticism, the enthusiasm around TAO is palpable among those who recognize its capability to turn suboptimal datasets into competitive AI model performances. According to [VentureBeat](https://venturebeat.com/data-infrastructure/the-tao-of-data-how-databricks-is-optimizing-ai-llm-fine-tuning-without-data-labels/), the implications of TAO extend far beyond individual AI projects—it could reshape industry standards for model training and AI deployment strategies. The fact that TAO has been able to elevate the performance of AI models like Meta's Llama 3.1B beyond giants like OpenAI's offerings speaks volumes about its technical prowess. Yet, the broader AI community remains vigilant about the method’s broader utility and operational constraints, pushing for comprehensive evaluations in diverse scenarios.
Future Implications: Economic and Social Impact
Databricks' innovative technique, TAO (Test-time Adaptive Optimization), promises to usher in a new era of economic transformation by significantly reducing the hurdles associated with AI integration in businesses. With TAO, firms can enhance their AI models without the need for vast amounts of perfectly labeled data, which is often a costly and time-consuming requirement. This capability not only paves the way for cost reductions but also encourages more rapid innovation across various sectors. By lowering the entry barrier for smaller companies, TAO democratizes access to cutting-edge AI technology, allowing them to compete more effectively with established industry giants. This shift could potentially stimulate job creation in new technology areas, offsetting the displacement effects automation might bring .
Learn to use AI like a Pro
Get the latest AI workflows to boost your productivity and business performance, delivered weekly by expert consultants. Enjoy step-by-step guides, weekly Q&A sessions, and full access to our AI workflow archive.
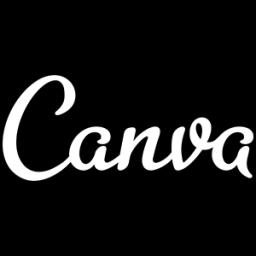
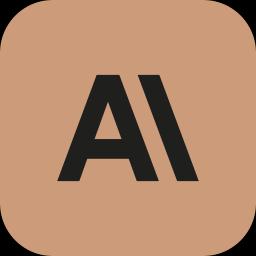
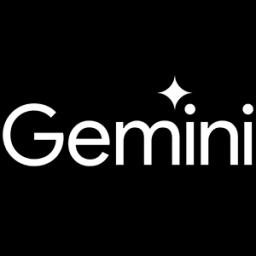
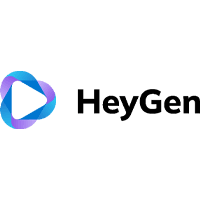
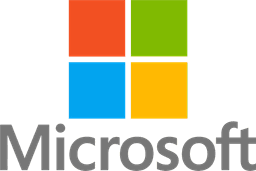
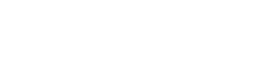
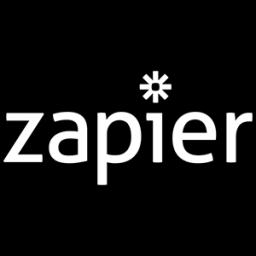
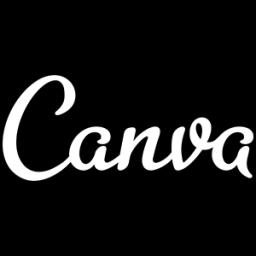
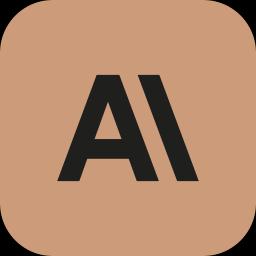
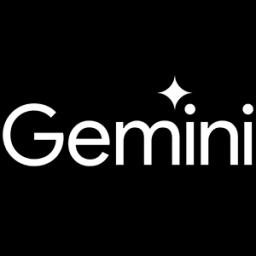
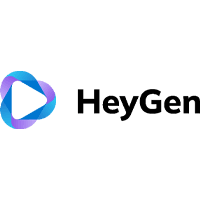
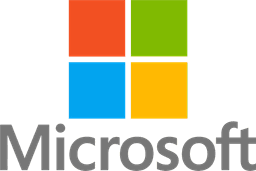
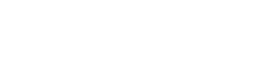
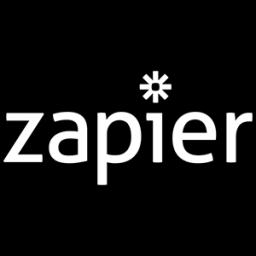
Socially, the implications of TAO extend far beyond economic gains. Automation spurred by improved AI capabilities might cause disruption in certain job markets, necessitating workforce retraining and adaptation. Nonetheless, the technology is poised to generate new employment opportunities in AI development and maintenance. Moreover, as AI becomes more integrated into critical sectors like healthcare and finance, the potential for enhanced services and outcomes will increase. However, these advantages come with pressing ethical considerations. Ensuring AI models are free from bias and maintain transparency and fairness will be critical in mitigating any negative social impacts. In this evolving landscape, maintaining public trust and ethical standards will be as crucial as technological advancement .
Politically, the widespread adoption of TAO raises significant regulatory challenges. Nations will need to craft comprehensive frameworks addressing data privacy, security, and bias inherent in AI algorithms to regulate this growing influence effectively. These regulations will play a vital role in preventing the misuse of AI technologies and mitigating any economic disruptions that might result from widespread automation. Furthermore, as the international race to develop superior AI capabilities intensifies, countries will need to navigate complex geopolitical landscapes to ensure they remain competitive without compromising ethical standards or consumer protections .
Ethical and Political Considerations of TAO
The advent of Databricks' TAO (Test-time Adaptive Optimization) technology raises significant ethical considerations, particularly around data privacy and AI bias. As TAO thrives on imperfect data to refine AI models, it becomes paramount to ensure that this data is not only ethically sourced but also free from biases that could perpetuate or exacerbate existing inequalities. Concerns about data privacy are also amplified, considering the volume of data TAO needs to function effectively. Ensuring transparency in how data is utilized and stored is crucial, as outlined in multiple discussions around AI ethics [source]. Critically, there is a need for comprehensive guidelines and frameworks that can govern the ethical deployment of such technologies, mitigating potential risks.
Politically, the widespread adoption of TAO could drive new regulatory demands. Governments around the world may need to establish regulations to protect data privacy and combat algorithmic bias. This need arises in tandem with the increasing automation of industries, which TAO could accelerate by enabling the more widespread and efficient utilization of AI models even in data-poor environments. Policymakers must consider how to balance the benefits of such technological advancements with potential disruptions in employment and other societal structures, prompting discussions on workforce retraining and educational reforms [source]. Such regulations need to be agile to keep pace with the rapid evolution of AI technologies.
Furthermore, the geopolitical landscape could be reshaped by TAO, as countries vie to either lead or catch up in AI innovation. The competitive edge offered by AI advancements like TAO could widen the technological gap between nations, thereby influencing global economic power structures. This intensified competition necessitates international cooperation to manage the dual-use nature of AI technologies responsibly and to address collective security concerns, such as those related to digital espionage or algorithmic warfare [source]. Ultimately, while TAO presents significant benefits in terms of technological progress, the political ramifications require thoughtful consideration and proactive policy-making to ensure its benefits are broadly shared and its risks mitigated.