Turning Threats into Protections
Tenable's Breakthrough: Prompt Injection Hacks Boost MCP Security!
Last updated:

Edited By
Mackenzie Ferguson
AI Tools Researcher & Implementation Consultant
In an innovative twist, Tenable's research reveals how a known AI vulnerability, prompt injection, can now be used as a defensive tool, enhancing the Model Context Protocol (MCP) security landscape. By repurposing this attack vector, organizations can implement robust logging, auditing, and firewalling capabilities for Large Language Models (LLMs) operating over MCP.
Introduction to the Model Context Protocol (MCP)
The Model Context Protocol (MCP) represents a groundbreaking development in the realm of Artificial Intelligence, particularly concerning large language models (LLMs). Developed by Anthropic, MCP serves as a pivotal standard that enables LLMs—such as AI chatbots—to seamlessly integrate and interact with a variety of external tools. This integration allows these models to undertake more complex and practical tasks that go beyond simple conversational interactions. By facilitating direct communication and cooperation between AI models and external systems, MCP enhances the functional capabilities of AI, opening new horizons for their application in diverse industries.
Given the increasing reliance on AI systems, the security of these integrations through MCP is paramount. Prompt injection, traditionally viewed as an AI attack vector, has emerged as a dual-use technique within this context. As detailed in Tenable's research, these techniques can be repurposed for enhancing security measures within the MCP framework. By using prompt injection defensively, security teams can audit and log tool interactions by the LLMs, potentially detecting unauthorized access attempts and fortifying the system's defenses. This proactive approach leveraging prompt injection underlines a novel method of safeguarding AI functionalities, utilizing an attack vector as a security mechanism. For further information on the defensive applications of prompt injection, see [this article](https://cxotoday.com/press-release/tenable-research-shows-how-prompt-injection-style-hacks-can-secure-the-model-context-protocol-mcp/).
Learn to use AI like a Pro
Get the latest AI workflows to boost your productivity and business performance, delivered weekly by expert consultants. Enjoy step-by-step guides, weekly Q&A sessions, and full access to our AI workflow archive.
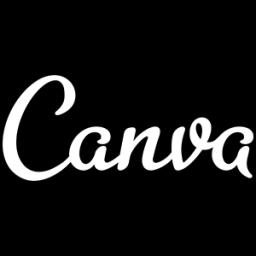
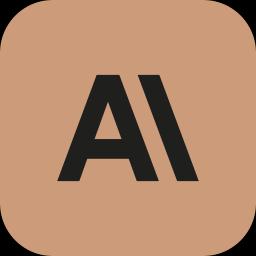
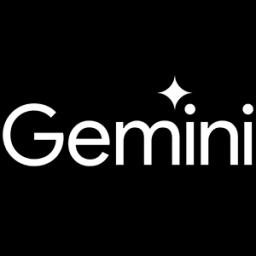
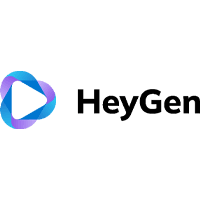
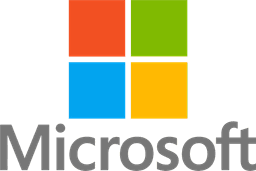
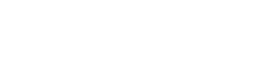
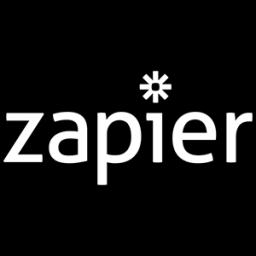
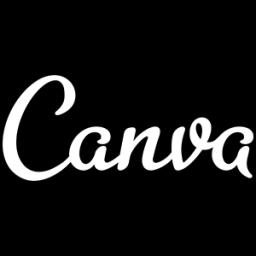
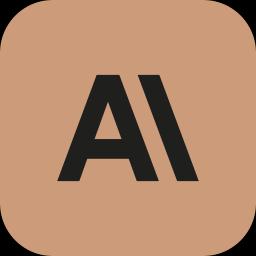
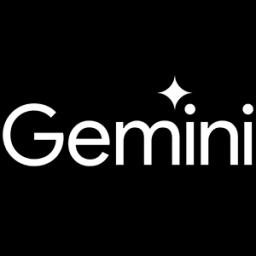
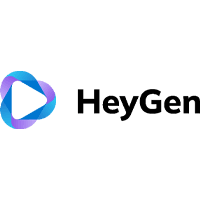
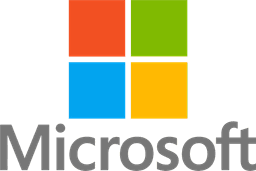
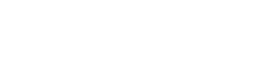
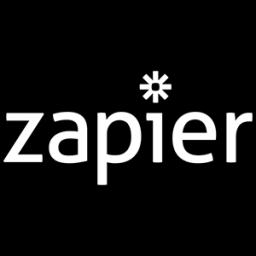
Understanding Prompt Injection as an Attack Vector
Prompt injection as an attack vector represents a significant concern in the landscape of artificial intelligence security. This method involves cleverly crafting input prompts that manipulate large language models (LLMs) into performing unintended or malicious actions, such as bypassing safety protocols or exposing sensitive data. These prompts exploit the inherent flexibility and responsiveness of LLMs, which are designed to process and execute a vast array of instructions as seamlessly as possible. Therefore, when adversaries use knowledge of a model's operational semantics, they can effectively deceive the system into executing commands that it would otherwise consider unsafe. With the growing reliance on AI technologies across various sectors, understanding and mitigating such vulnerabilities is of paramount importance for maintaining robust security frameworks [1](https://cxotoday.com/press-release/tenable-research-shows-how-prompt-injection-style-hacks-can-secure-the-model-context-protocol-mcp/).
Despite its potential for harm, prompt injection is not exclusively a malevolent tool. Recent research has indicated that this technique can be redirected towards defensive measures within AI systems, specifically under the Model Context Protocol (MCP). By utilizing tailored prompts, security teams can audit, log, and control the operations of LLMs, ensuring that only authorized actions and tool calls are executed. This proactive application of prompt injection offers a novel layer of security, transforming a traditionally hazardous tactic into a method of safeguarding against unauthorized access and operations [1](https://cxotoday.com/press-release/tenable-research-shows-how-prompt-injection-style-hacks-can-secure-the-model-context-protocol-mcp/).
The transition of prompt injection from an attack vector to a defensive strategy highlights the dual-use nature of this technique. It showcases the potential to create "firewall" mechanisms that protect LLMs by default, functioning within the MCP framework to prevent malfeasance. This transformation not only underlines the versatility of prompt injection but also emphasizes the evolving landscape of AI security where lines between attack and defense become increasingly blurred. Such developments necessitate a deep understanding of AI operations and behaviors, urging the adoption of custom-tailored solutions to address the specific security needs posed by different models [1](https://cxotoday.com/press-release/tenable-research-shows-how-prompt-injection-style-hacks-can-secure-the-model-context-protocol-mcp/).
Repurposing Prompt Injection for Security
The concept of prompt injection, originally identified as a potential security threat, is now being ingeniously repurposed to enhance security measures within the Model Context Protocol (MCP). This protocol, developed by Anthropic, is pivotal for enabling large language models (LLMs) to seamlessly interact with external tools to execute complex tasks. Traditionally, prompt injection involved malicious entities manipulating AI input to subvert system operations, leading to security breaches or unauthorized actions. However, Tenable Research has highlighted a transformative approach where these very techniques can be harnessed for defensive purposes. By carefully crafted prompts, security teams can monitor, audit, and manage tool interactions within the MCP, thus fortifying the overall security architecture [1](https://cxotoday.com/press-release/tenable-research-shows-how-prompt-injection-style-hacks-can-secure-the-model-context-protocol-mcp/).
Learn to use AI like a Pro
Get the latest AI workflows to boost your productivity and business performance, delivered weekly by expert consultants. Enjoy step-by-step guides, weekly Q&A sessions, and full access to our AI workflow archive.
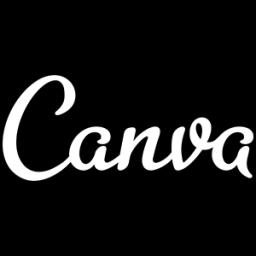
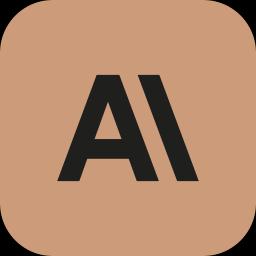
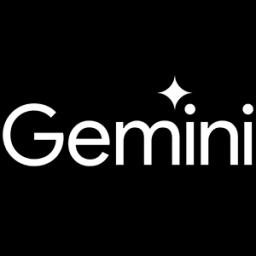
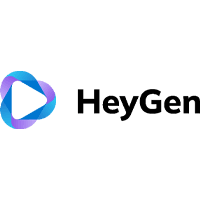
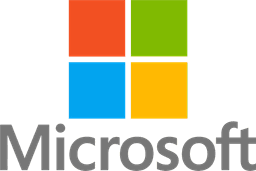
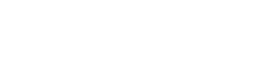
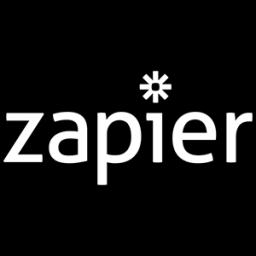
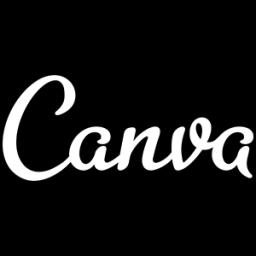
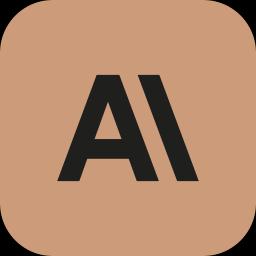
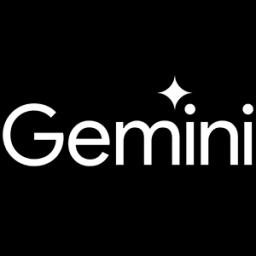
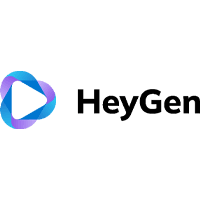
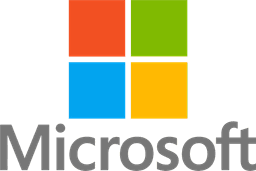
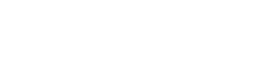
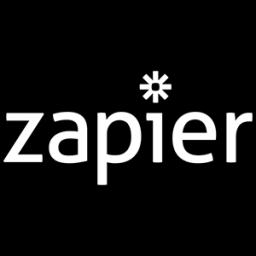
Employing prompt injection as a security ally showcases a remarkable dual-use dynamic that challenges traditional perceptions of AI vulnerabilities. This proactive methodology not only allows for detailed auditing and logging of tool calls but also enables the creation of so-called "firewall tools." These tools operate within the MCP framework to effectively block unauthorized or potentially harmful tool requests, turning an erstwhile attack method into a robust security mechanism [1](https://cxotoday.com/press-release/tenable-research-shows-how-prompt-injection-style-hacks-can-secure-the-model-context-protocol-mcp/).
Despite the promising applications of prompt injection for enhancing security, there are notable challenges related to its deployment across different models. Large language models exhibit varied behaviors when subjected to prompt injections. This variability necessitates model-specific security strategies to ensure efficacy. Furthermore, the inconsistency in LLM responses to these prompts emphasizes the need for comprehensive testing and nuanced understanding of each model’s operational dynamics [1](https://cxotoday.com/press-release/tenable-research-shows-how-prompt-injection-style-hacks-can-secure-the-model-context-protocol-mcp/).
The implications of repurposing prompt injection for security measures extend beyond immediate technological benefits to encompass broader economic, social, and political impacts. Economically, this innovative use of AI could lead to reduced costs in security implementation while catalyzing growth in AI-driven industries. Socially, enhancing AI system reliability fosters greater public trust and encourages integration into daily applications. Nonetheless, there is a risk of these technologies being used for surveillance, which could compromise privacy and instigate ethical debates [1](https://cxotoday.com/press-release/tenable-research-shows-how-prompt-injection-style-hacks-can-secure-the-model-context-protocol-mcp/).
Politically, the adaptation of prompt injection techniques as security protocols may necessitate new regulatory frameworks and could influence international relations, especially if these technologies are perceived as tools for cyber warfare. National policies could shift to embrace or restrict these developments, influencing global AI governance and potentially leading to geopolitical power shifts [1](https://cxotoday.com/press-release/tenable-research-shows-how-prompt-injection-style-hacks-can-secure-the-model-context-protocol-mcp/). Future research is crucial to explore the full spectrum of implications, both beneficial and problematic, that this repurposing might entail, ensuring a balanced approach between innovation and caution [1](https://cxotoday.com/press-release/tenable-research-shows-how-prompt-injection-style-hacks-can-secure-the-model-context-protocol-mcp/).
Variations in Large Language Models (LLMs) Response
Large Language Models (LLMs) present an array of responses due to their underlying diverse architectures, training data, and algorithms. When subjected to similar prompts, LLMs can yield varied outputs, a reflection of each model's unique configuration. This variability necessitates closer examination, especially in scenarios where security and accuracy are paramount. Prompt injection, while traditionally considered a threat, can be paradoxically leveraged to enhance security measures in the Model Context Protocol (MCP). A comprehensive understanding of each model's behavior enables more effective deployment of safeguards and better anticipates areas where vulnerabilities might emerge.
The varied responses from LLMs during prompt injection scenarios reveal that each model interprets inputs differently. This interpretive difference can significantly impact the effectiveness of security techniques. For instance, while some models may consistently adhere to defensive prompts designed to reinforce security protocols, others might bypass or misinterpret these cues, resulting in potential security lapses. Consequently, developers and researchers must devise model-specific strategies that account for these behavioral nuances to ensure robust and secure integration within the MCP framework.
Learn to use AI like a Pro
Get the latest AI workflows to boost your productivity and business performance, delivered weekly by expert consultants. Enjoy step-by-step guides, weekly Q&A sessions, and full access to our AI workflow archive.
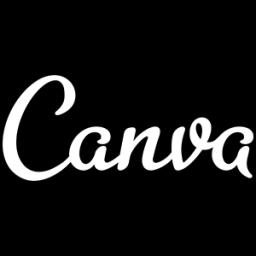
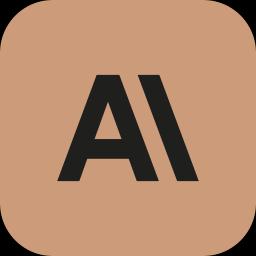
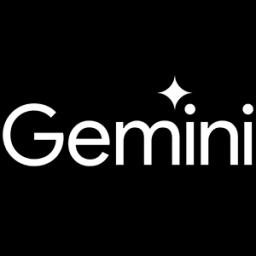
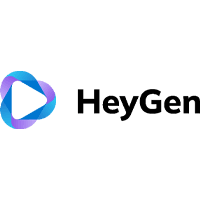
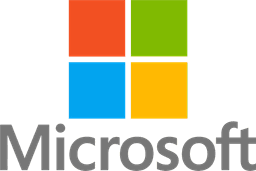
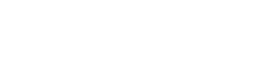
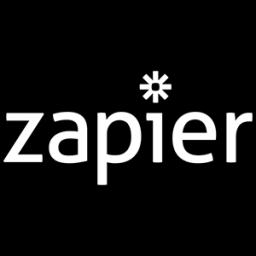
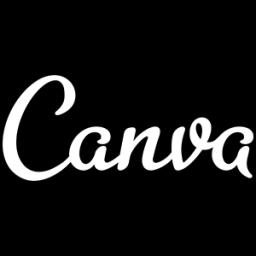
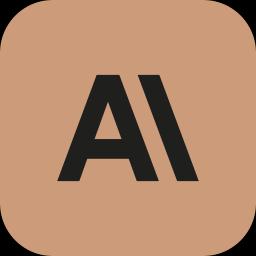
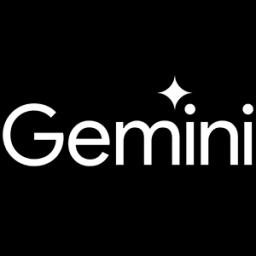
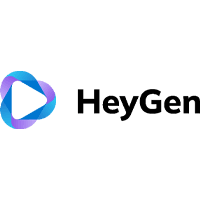
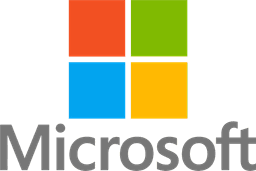
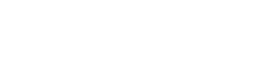
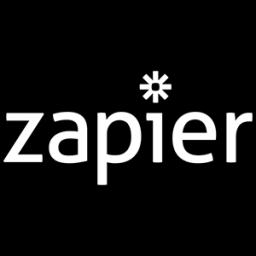
The integration of LLMs into the Model Context Protocol (MCP) highlights a significant challenge posed by the disparate behaviors of these models. As the article from CXO Today illustrates, using prompt injection defensively requires detailed knowledge of each model's operations. This awareness facilitates the creation of tailored security measures, such as auditing and logging, which help prevent unauthorized actions by the LLMs while operating over MCP. Tailoring these approaches to each model ensures that security practices are not only effective but also aligned with the unique responses of each AI system.
The emergence of different LLM behaviors underlines the need for rigorous testing for cybersecurity applications. The research indicates that variations in responses could lead to inconsistent application of security directives across different AI platforms, making it crucial for security teams to conduct thorough evaluations and adjustments. This tailor-made approach, encapsulated by Tenable's findings, prevents oversights and potential exploitation by malicious entities. For MCP to function securely, the safety mechanisms such as "firewall" tools created through defensive prompt injections must be adaptable to each model's response patterns.
As models evolve, the implications of their varied responses become increasingly important. Organizations adopting these models within MCP must remain vigilant, acknowledging that a one-size-fits-all strategy is ineffective. Instead, ongoing research and development should aim to capture the broad spectrum of LLM behaviors to synthesize more intelligent strategies. This vision entails developing a suite of diagnostic tools that assess model performance under different prompts, informing adjustments that bolster security and compliance with rigorous standards tailored to each unique computational entity.
Mitigation Strategies for MCP Vulnerabilities
To effectively mitigate vulnerabilities in the Model Context Protocol (MCP), organizations must implement a wide range of preventive measures. A fundamental strategy involves establishing comprehensive auditing processes that enable the monitoring of interactions within the MCP. Utilizing prompt injection, security teams can log and audit all tool calls made by large language models (LLMs), as highlighted in recent research. This measure not only helps in tracking the actions undertaken but also in identifying and responding to any unauthorized or potentially harmful activities within the system, thereby securing the framework from potential misuse .
Another crucial aspect of mitigating MCP vulnerabilities is the implementation of robust access controls. By enforcing least-privilege access, organizations can ensure that users have only the necessary permissions to perform their tasks, which minimizes the risk of misuse or exploitation of higher-level access capabilities. Moreover, requiring user approvals for tool execution within the MCP environment can serve as an additional safeguard, ensuring that all actions are recorded and scrutinized before they are carried out.
In addition to access controls, defensive prompt injection techniques can be transformed into proactive security measures, akin to firewall tools, within the MCP framework. These techniques can block unauthorized or suspicious tools from being executed, leveraging the same mechanisms employed in malicious attacks to create a defensive posture. By doing so, organizations can prevent unauthorized manipulations of system behavior, thereby maintaining the integrity and security of their AI applications .
Learn to use AI like a Pro
Get the latest AI workflows to boost your productivity and business performance, delivered weekly by expert consultants. Enjoy step-by-step guides, weekly Q&A sessions, and full access to our AI workflow archive.
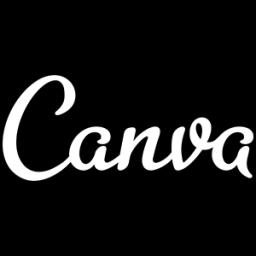
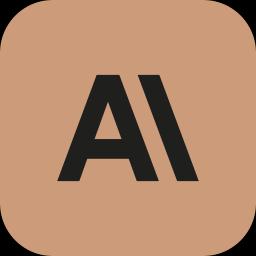
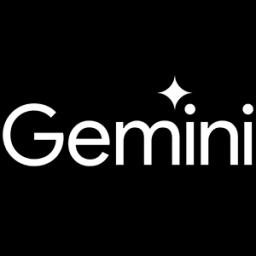
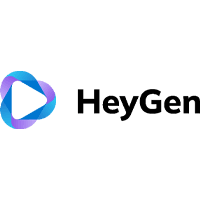
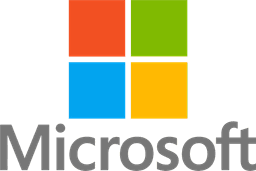
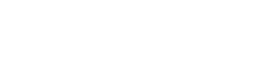
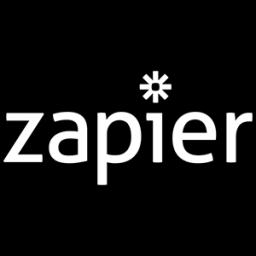
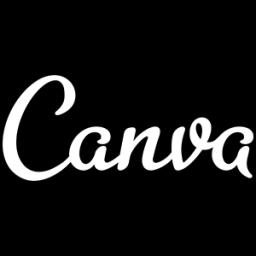
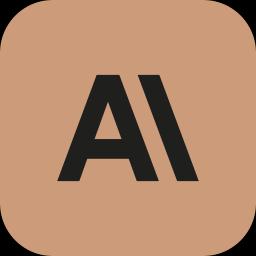
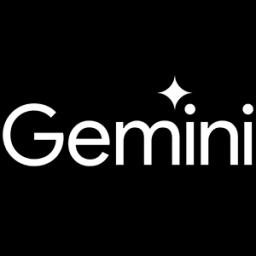
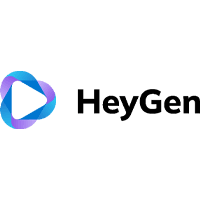
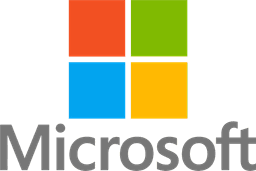
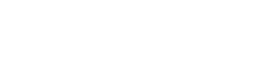
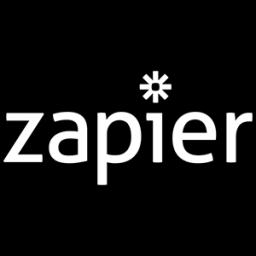
Security measures should also account for the varied behaviors exhibited by different LLMs in response to prompt injections. Inconsistencies in model responses necessitate tailored security strategies for each model type. Security teams need to conduct thorough testing and understanding of each LLM's unique characteristics to implement effective mitigation strategies. This customization ensures that the security measures are not only robust but also adaptable to different AI environments .
Creating Firewall Tools Using Prompt Injection
In a groundbreaking shift, cybersecurity experts are exploring how prompt injection, an entity formerly known for its role in AI security vulnerabilities, can now be utilized as a formidable tool for creating "firewall" solutions within the Model Context Protocol (MCP). This innovative approach, highlighted by Tenable's recent research, demonstrates the dual nature of prompt injection. While traditionally seen as a vector for malicious attacks that could manipulate large language models (LLMs) into compromising actions, this same mechanism can now be harnessed for protective purposes. By repurposing prompt injection, security teams can effectively audit, log, and regulate the interactions within MCP, thus safeguarding AI systems from unauthorized access and potential cyber threats all while maintaining the integrity of AI operations .
Prompt injection techniques, traditionally a concern due to their potential misuse in bypassing AI safety protocols, are undergoing a renaissance in AI security strategy thanks to research such as that from Tenable. By embedding strategically crafted prompts into interactions with MCP, organizations can establish a sophisticated layer of security that functions similarly to a firewall. This method provides real-time monitoring of LLM tool calls, thereby allowing for active prevention of unauthorized tool executions. The idea of transforming an AI vulnerability into a robust security tool illustrates the evolving landscape of cybersecurity, where adaptive strategies are now essential to counteract the dynamic nature of cyber threats .
Furthermore, the variable responses of different LLMs to prompt injections reveal significant implications for security implementations across AI models. The breadth of behavior exhibited by these models necessitates tailored security measures, ensuring that defenses are as dynamic and nuanced as the threats posed. This specificity in approach not only enhances security but also underscores the importance of continued research and development in AI behavior. Indeed, the creation of firewall tools through prompt injection not only serves as a proactive defense strategy but also highlights the potential for widespread application in AI safeguarding, paving the way for increasingly secure digital infrastructures .
The introduction of firewall tools via prompt injection offers a glimpse into the future of AI-driven security frameworks, where the very aspect of AI that was once considered a weak link now becomes a cornerstone of cybersecurity strategy. This transition is more than just a technological evolution; it signifies a shift in perspective within the cybersecurity community, recognizing that the mechanisms of adverse impact can be the very pathways to enhanced protection. Implementing such solutions within the MCP allows organizations to not only detect and deter unsanctioned actions by LLMs but to foster a security environment that naturally adapts to the changing landscape of threats, thus maintaining operational security and efficiency .
Expert Opinions on Prompt Injection and Security
Prompt injection, traditionally viewed as a security threat to artificial intelligence models, can also be harnessed for reinforcing AI security, especially within the Model Context Protocol (MCP). According to a recent report from Tenable, these techniques can be repurposed to serve defensive functions such as auditing, logging, and firewalling tool invocations within MCP. The research suggests a novel angle whereby security teams can insert specific prompts to oversee all transactions initiated by the language models, helping to identify unauthorized or potentially harmful tools.
Learn to use AI like a Pro
Get the latest AI workflows to boost your productivity and business performance, delivered weekly by expert consultants. Enjoy step-by-step guides, weekly Q&A sessions, and full access to our AI workflow archive.
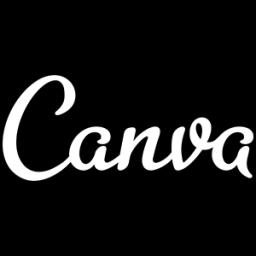
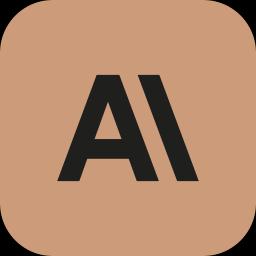
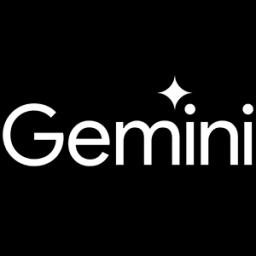
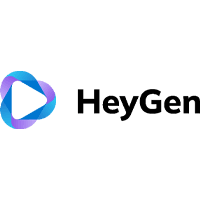
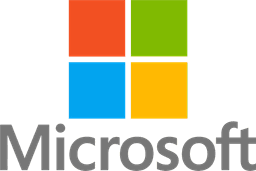
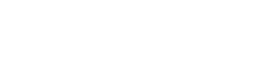
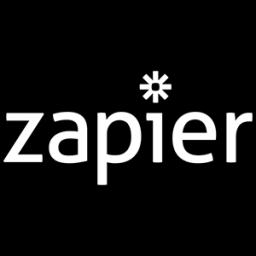
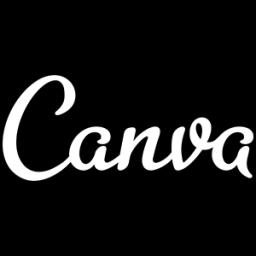
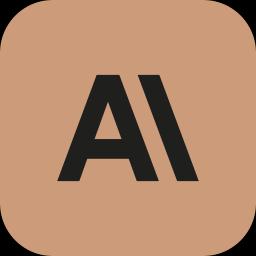
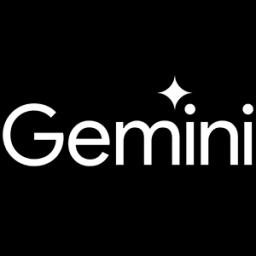
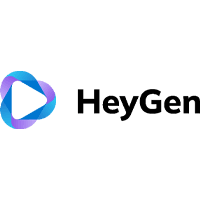
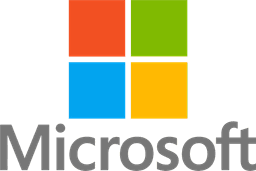
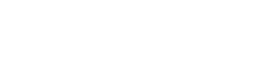
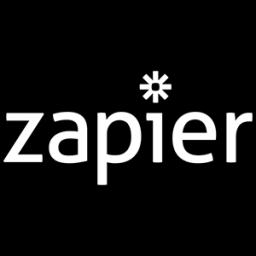
The dual nature of prompt injection is further highlighted by experts who stress its capability for both offense and defense in the AI security landscape. Ben Smith from Tenable noted the potential for prompt injection to act as both a security vulnerability and a robust defensive mechanism. By integrating prompt injection techniques for protections, organizations can bolster their AI models against unforeseen threats. This strategy offers a layered security mechanism that not only guards against traditional prompt injection attacks but also anticipates novel threats.
Additionally, industry leaders like Microsoft are advocating for the use of prompt injection as a proactive measure in securing MCP environments. Their suggested safeguards include AI prompt shields and stringent supply chain security protocols. Through employing advanced machine learning and natural language processing techniques, they aim to differentiate legitimate prompts from malicious ones while reinforcing fundamental security controls like multi-factor authentication and least-privilege access.
In light of these expert opinions, the future of AI security may increasingly lean on the strategic use of prompt injection. The technique is anticipated to play a central role not just in thwarting attacks but also in establishing a comprehensive security framework that can seamlessly adapt to evolving AI threats. By fostering collaborations between AI researchers and security experts, the development of resilient models capable of resisting and effectively countering prompt injection-based exploits appears promising.
Economic Impacts of Defensive Prompt Injection
The economic impacts of defensive prompt injection strategies are poised to revolutionize the cybersecurity landscape, potentially trimming costs associated with traditional security measures and catalyzing a surge in AI adoption. By repurposing this typically exploitative technique, organizations can create more efficient and scalable security solutions that can be integrated seamlessly into existing AI frameworks. Tenable's research illustrates that through techniques such as auditing, logging, and firewalling, AI models operating on the Model Context Protocol (MCP) can be fortified without extensive resource allocation, fostering a more cost-effective approach to security. This shift promises not only operational savings but also stimulates growth by creating a fertile ground for new AI-driven ventures [1](https://cxotoday.com/press-release/tenable-research-shows-how-prompt-injection-style-hacks-can-secure-the-model-context-protocol-mcp/).
Moreover, the advent of defensive prompt injection opens up a burgeoning market for specialized AI security firms. These firms are likely to emerge as leaders in the cybersecurity field, offering cutting-edge solutions that marry traditional security measures with innovative AI-driven approaches. As the demand for these specialized services rises, so too does the need for skilled professionals capable of navigating the intricate dynamics of AI security, potentially leading to a redefinition of cybersecurity roles and reducing the relevance of more conventional positions. This evolution could create a new economic paradigm, characterized by a demand for AI proficiency and expertise in defensive cybersecurity measures, thus reshaping the job market and contributing to economic development [1](https://cxotoday.com/press-release/tenable-research-shows-how-prompt-injection-style-hacks-can-secure-the-model-context-protocol-mcp/).
In addition, the strategic use of defensive prompt injection can also drive investment in AI research and development. Companies looking to harness these advanced security techniques are poised to invest significantly in developing proprietary technology and training employees in AI security protocols. This not only injects capital into the technological sector but also accelerates innovation, as firms compete to offer the most advanced and reliable security solutions. As a result, the entire AI ecosystem may benefit from heightened investment, fostering an environment of constant technological advancement and economic vitality [1](https://cxotoday.com/press-release/tenable-research-shows-how-prompt-injection-style-hacks-can-secure-the-model-context-protocol-mcp/).
Learn to use AI like a Pro
Get the latest AI workflows to boost your productivity and business performance, delivered weekly by expert consultants. Enjoy step-by-step guides, weekly Q&A sessions, and full access to our AI workflow archive.
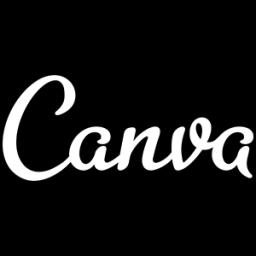
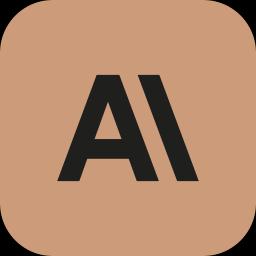
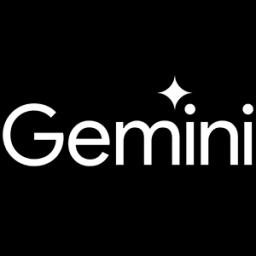
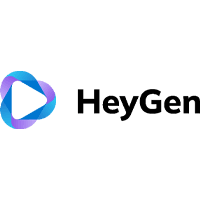
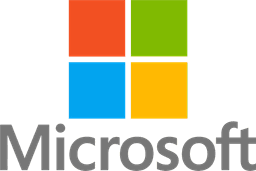
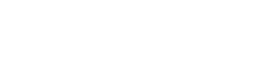
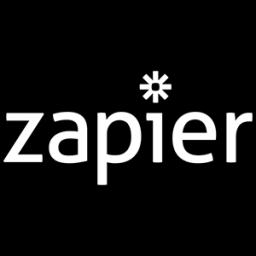
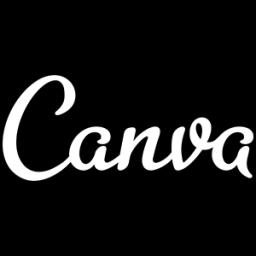
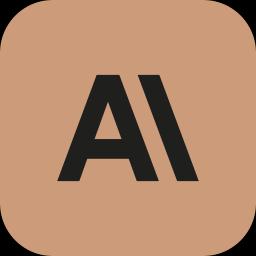
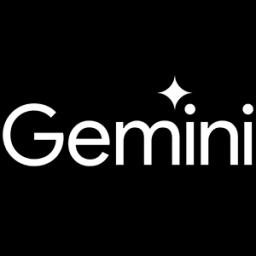
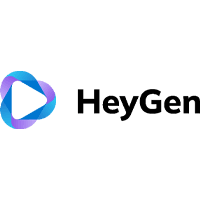
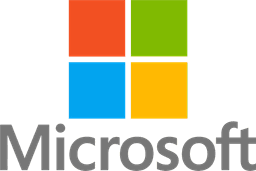
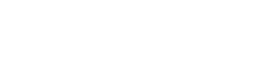
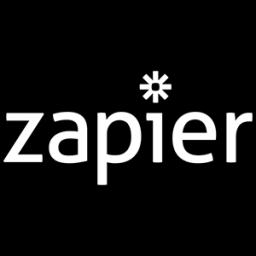
Social and Political Implications
The integration of prompt injection techniques into AI security measures presents significant social and political implications. On a social front, the implementation of these techniques may enhance the trust and reliability of AI systems, thereby fostering greater public confidence and facilitating deeper integration of AI in everyday life. This could have profound effects on society's collective relationship with technology, reshaping how individuals interact with intelligent systems in personal, educational, and professional environments. Nonetheless, the potential for misuse looms large. There is a risk that such techniques could be appropriated for purposes of surveillance or censorship, which would represent a serious intrusion on privacy rights and civil liberties. This dual potential underscores the importance of robust ethical guidelines and oversight to ensure these technologies are used responsibly and for the public good.
Politically, the adoption of prompt injection as a defensive tool within AI security protocols could prompt regulatory actions at both national and international levels. Governments may see the need to regulate these techniques carefully, balancing the potential benefits against risks of abuse. This regulatory stance could significantly influence the trajectory of AI development and deployment across sectors. Moreover, the international landscape could be altered as countries leverage these technologies for cyber warfare or national defense strategies. The offensive and defensive capabilities within the same set of technologies might lead to heightened geopolitical tensions, as nations vie for technological superiority. This scenario underscores the pressing need for international collaboration to establish norms and treaties that govern the safe and equitable use of these emerging technologies.
Uncertainty and Need for Future Research
As we step into a new era marked by unprecedented advancements in AI technology, the unpredictability associated with emerging threats such as prompt injection within the Model Context Protocol (MCP) presents both challenges and opportunities. Current research, including a study by Tenable, has begun to illuminate how these vulnerabilities can be harnessed for security purposes, but the path forward remains clouded with uncertainty. The potential for such techniques to both mitigate and exploit AI vulnerabilities is still largely unexplored, necessitating ongoing research to grasp their full scope and impact .
The limitations in our understanding of AI behavior, particularly the varied responses of different large language models (LLMs) to prompt injection, underscore the need for more nuanced research in this field . Not only is it critical to identify and address existing vulnerabilities, but it is equally important to anticipate the emergence of new threats as AI technology continues to evolve. This calls for a dynamic and iterative approach to research that keeps pace with the rapid advancements in AI capabilities.
It is also crucial to develop comprehensive regulatory frameworks that can effectively govern the use of such sophisticated techniques. These frameworks will need to balance the benefits of defensive prompt injection with the risks, such as potential misuse leading to privacy infringements or other unintended consequences . The adaptability of researchers and policymakers will play a pivotal role in shaping the strategic landscape of AI security, requiring collaborative efforts across academia, industry, and government to ensure these technologies are deployed ethically and effectively.
Learn to use AI like a Pro
Get the latest AI workflows to boost your productivity and business performance, delivered weekly by expert consultants. Enjoy step-by-step guides, weekly Q&A sessions, and full access to our AI workflow archive.
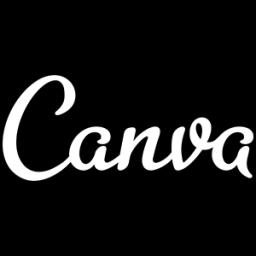
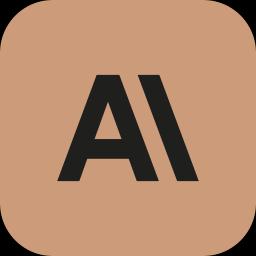
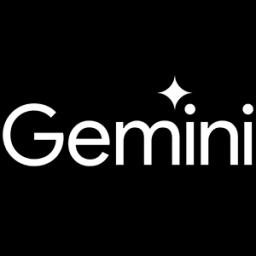
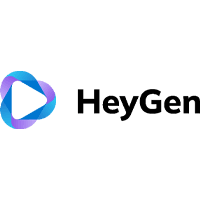
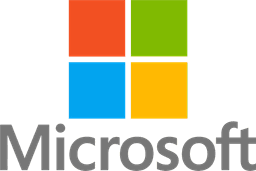
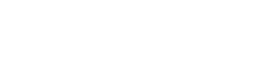
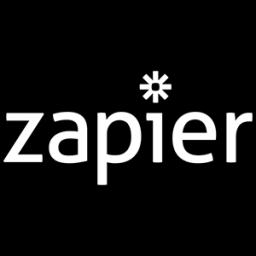
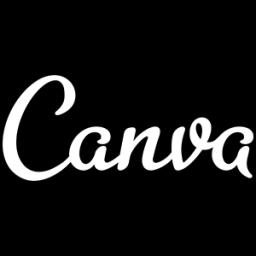
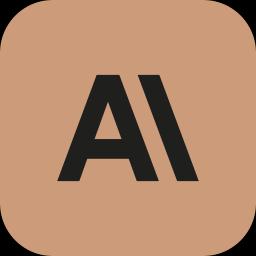
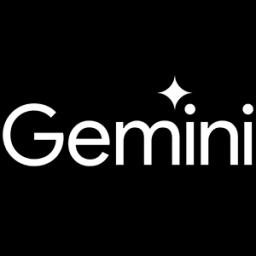
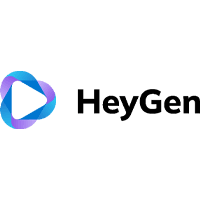
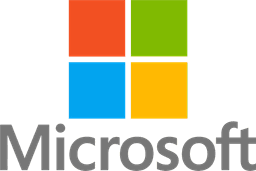
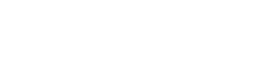
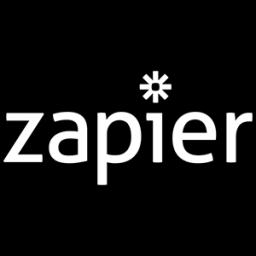
Finally, the trajectory of AI security will rely heavily on technological developments and the vigilance of the research community. The interplay between AI capabilities and security measures is complex, making it imperative for researchers to continuously re-evaluate the effectiveness of current strategies. As the field progresses, the ability to foresee the long-term implications and adapt accordingly will be crucial in safeguarding against both present and future threats .